Abstract
The uncertainty factor concept is integrated into health risk assessments for all aspects of public health practice, including by most organizations that derive occupational exposure limits. The use of uncertainty factors is predicated on the assumption that a sufficient reduction in exposure from those at the boundary for the onset of adverse effects will yield a safe exposure level for at least the great majority of the exposed population, including vulnerable subgroups. There are differences in the application of the uncertainty factor approach among groups that conduct occupational assessments; however, there are common areas of uncertainty which are considered by all or nearly all occupational exposure limit-setting organizations. Five key uncertainties that are often examined include interspecies variability in response when extrapolating from animal studies to humans, response variability in humans, uncertainty in estimating a no-effect level from a dose where effects were observed, extrapolation from shorter duration studies to a full life-time exposure, and other insufficiencies in the overall health effects database indicating that the most sensitive adverse effect may not have been evaluated. In addition, a modifying factor is used by some organizations to account for other remaining uncertainties—typically related to exposure scenarios or accounting for the interplay among the five areas noted above. Consideration of uncertainties in occupational exposure limit derivation is a systematic process whereby the factors applied are not arbitrary, although they are mathematically imprecise. As the scientific basis for uncertainty factor application has improved, default uncertainty factors are now used only in the absence of chemical-specific data, and the trend is to replace them with chemical-specific adjustment factors whenever possible. The increased application of scientific data in the development of uncertainty factors for individual chemicals also has the benefit of increasing the transparency of occupational exposure limit derivation. Improved characterization of the scientific basis for uncertainty factors has led to increasing rigor and transparency in their application as part of the overall occupational exposure limit derivation process.
INTRODUCTION
The use of uncertainty factors (UFs) in establishing exposure limits extends back at least as far as 1954, when Lehman and Fitzhugh(Citation1) proposed a 100-fold factor (which they referred to as a “margin of safety”) for extrapolating from animal toxicity data to safe levels of human exposure to food additives and pesticide residues. The UF concept is integrated into health risk assessments for all aspects of current public health practice, including in deriving health-based occupational exposure limits (OELs). The concept has widespread application, but the nomenclature for UFs varies among organizations—other terms for this concept include safety factors or assessment factors.(Citation2,Citation3) Despite the widespread acceptance of the UF approach for risk assessment, implementing the concept varies significantly among organizations. In addition, there are unique aspects of occupational risk assessment that impact UF application. Methods for establishing OELs were initially proposed decades ago and have seen ongoing modification and application.(Citation2,Citation4–Citation8) In these articles, the authors briefly described the sources of uncertainty in the limit-setting process and suggested possible default UFs, but none presented specific scientific support for the UFs recommended for establishing OELs.
The conceptual basis for the application of UFs is that chemical toxicity usually follows a predictable pattern with increasing dose—from no significant effects, to minimal effects (not necessarily adverse) within the range of biological compensation, to clearly toxic effects, and finally to overt disease and/or death.(Citation9) The use of UFs is predicated on the assumption that sufficiently reducing exposure from levels known to be toxic will yield an exposure level that is safe for at least the great majority of the exposed population, including vulnerable subgroups. Organizations that publish OEL values differ in how to approach chemicals with a toxic mode of action (MOA) that suggests the lack of a biological threshold. This issue is most commonly encountered in the context of genotoxic carcinogens and mutagens, which are frequently considered to be exceptions to the general rule that a safe dose exists for most toxicants. Such chemicals are often treated as posing some degree of excess risk—though possibly very slight—at any dose greater than zero. In many organizations, development of acceptable exposure levels for chemicals that lack a biological threshold is based on dose-response modeling and low-dose extrapolation rather than application of UFs.(Citation10) The field is moving to increased use of mode of action analysis to evaluate implications of the nature of the toxic response for its likely dose-response behavior. The result is an integrated risk assessment approach for cancer and non-cancer endpoints and use of methods that best reflect biological understanding.(Citation11) Whether for cancer or non-cancer endpoints, considering UFs is often a critical step in OEL development.
This article describes the application of the UF approach for OEL setting and the issues encountered. Key points of emphasis covered in this manuscript include the following.
Risk assessment organizations generally agree in the areas of variability and uncertainty addressed in setting OELs. However, the quantitative application of UFs and the level of documentation provided in an OEL rationale vary widely.
The current standards of practice for setting OELs are changing with a general shift toward including chemical-specific adjustments and increasing documentation and transparency of the UF selection.
An understanding of the sources of variability and uncertainty addressed in an OEL are essential to its informed use and interpretation by the risk manager.
Application of advances in toxicology, including results of new testing strategies beyond traditional animal testing, is having a growing impact in informing mode-of-action and dose-response assessments, including in quantitative UF application.
APPLICATION AND DESCRIPTION OF UNCERTAINTY FACTORS
Uncertainty Factors Application for Occupational Exposure Limit Derivation
UFs enter the OEL process after a point of departure (PoD) has been determined,(Citation12) and prior to establishing the OEL. The PoD refers to the dose or concentration from the health effects study that is the starting point for extrapolation to the OEL. Regardless of whether the PoD represents a no-effect level (e.g., a no-observed-adverse-effect level [NOAEL] or benchmark dose [BMD]) or an effect level (e.g., a lowest-observed-adverse-effect-level [LOAEL]) in the exposed population or animal dose group, in general there will be some uncertainty in how the PoD relates to the true no-adverse-effect level in the broader working population. This is particularly evident when the PoD is based on animal data. The application of UFs is intended to result in an OEL that is protective of all adverse chemical-related effects.
For the purposes of calculation, health-based OELs are derived by dividing the PoD for the critical effect by various uncertainty or adjustment factors to extrapolate to the “true” no-effect level in the worker population of interest (Equation (1)). In general, the OEL can be derived with greatest confidence from a high quality epidemiology or controlled inhalation exposure study in human volunteers. However, the PoD is typically derived from a single key study of a relatively small number of animals or humans, possibly via another route of exposure.(Citation12) For purposes of this discussion, the PoD is defined as a mg/kg dose, because oral-dosing data are more commonly available than inhalation data. If other units are used (such as mg/m3 exposure concentration), or if the PoD is related to local or site-of-entry effects, dose unit adjustments may be required.(Citation13) UFs have been defined for each of the main sources of uncertainty typically considered. These factors are multiplied together to form a composite uncertainty factor (UFC) that is used in the denominator of the general equation used to set health-based limits.
Equation (1) Calculation of an OEL for systemic effects
Basis for Individual Uncertainty Factors
Regardless of the organization involved, there are common considerations related to biological variability and database uncertainty that all, or nearly all, OEL-setting organizations consider. highlights the common considerations using the nomenclature of EPA,(Citation14) which will be carried forward in this article. The specific nomenclature used and specific individual factors and their default values vary among organizations (). Note that most expert committees do not publish specific information on the values they assign to UFs, if any are explicitly used.(Citation15,Citation16)
Table 1 UFs Used in OEL-setting, and the Rationale for Their Use
Table 2 Default Uncertainty/Assessment Factors for WorkersA
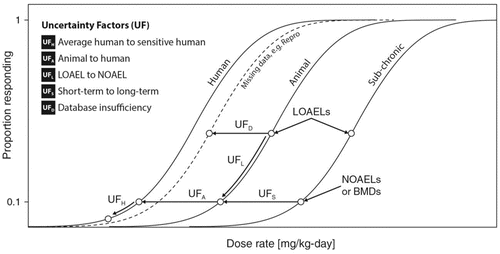
A conceptual visualization of each area of uncertainty is shown in . Here, UFs are seen as differing extrapolations, either among dose-response curves for different types of data, or between different points along the same dose response curve. Two of the UFs (UFA and UFH) deal specifically with biological variability. In the context of biological variability, research to gain more information helps to characterize the inherent variability and may increase or decrease the value of the factor needed. For example, UFA accounts for uncertainty in extrapolating from experimental animals to human toxicity when the PoD is based on animal bioassay data. This adjustment to the PoD allows for the possibility that the human response may occur at a lower dose than the animal response. Similarly, UFH allows for variation in kinetics and dynamics in the dose-response relationship for humans, adjusting the PoD estimate from the average human to a sensitive or susceptible human subpopulation. Together these two factors have the effect of converting a NOAEL in animals to a NOAEL for the sensitive human population. In many cases, there are other inadequacies in the source data for the PoD and the resulting uncertainties are addressed using three additional factors, UFL, UFS, and UFD. Jointly, these three factors account for the possibility that a lower PoD would have been found if more complete data had been available. Reducing these uncertainties by applying more data will provide a better PoD and reduce overall uncertainty. UFL is applied to extrapolate from a LOAEL to a NOAEL within the same dose-response curve. UFS shifts the PoD from a short-term study to a lower value, which might be observed given a longer study. UFD is intended to adjust for missing data (such as the lack of developmental or reproductive toxicity assays) that might have identified more sensitive endpoints. Each of these areas of uncertainty is detailed below.
Addressing Sources of Uncertainty Using Science-based Adjustment Factors
Dourson and Stara,(Citation17) Naumann and Weideman,(Citation18) and Dourson et al.(Citation19) summarized the scientific bases for the uncertainty factors discussed below. The use of UFs is a scientific judgment based on a policy decision to determine a safe concentration/dose. This judgment should be based on a systematic approach. Toxicological expertise is normally required to determine the appropriate UFs to apply, and alternative factors may be used if supported by the available data for a specific chemical and a sound scientific rationale. For this reason, to achieve robust derivation of an OEL, full consideration must be given to the underlying rationale for and intent of each key area of uncertainty, along with how it can be best addressed with the available data. UFs discussed below address the major sources of variability and uncertainty when setting safe levels of exposure for humans. Note that the values may differ depending on the segment of the population being protected. Reference doses (RfDs) established by the U.S. Environmental Protection Agency (EPA) are designed to protect the whole population, including the young, elderly, and infirm, throughout their entire lifetime. OELs are intended to protect workers, who are generally considered healthy adults, during their working lifetime. They should also protect the offspring of workers and not lead to premature illness or death beyond working life.
Animal-to-Human Uncertainty Factor
The animal-to-human uncertainty factor (UFA) is used to extrapolate from an animal-based PoD to estimate the corresponding PoD for the “average” human. Application of this factor takes into account the possibility that humans may respond at a lower dose than the animals used in the critical toxicity study. This assumption is viewed as precautionary, because humans can be equally sensitive or even less sensitive than animals for a given response. This factor is used in the absence of data to demonstrate these latter possibilities, and replacement with data is preferred, when possible. A default uncertainty factor as high as 10 is typically used in the absence of chemical-specific data;(Citation19) see below for further information.
The UFA is often conceptualized as being composed of a sub-factor for toxicokinetic (TK) differences between species (i.e., differences in the internal dose to the target tissues for toxicity), and a sub-factor for toxicodynamic (TD) differences in the sensitivity (i.e., differences in the response of the target tissue to a given internal dose). This division of the UFA into sub-factors differs among organizations. Notably, the WHO applies a sub-factor of 4 for toxicokinetics and 2.5 for toxicodynamics;(Citation20,Citation21) while the EPA typically uses equal sub-factors of √10, or approximately 3.(Citation22) The TK sub-factor may require adjustment under certain circumstances, such as when inhalation dosimetry models are used or when the PoD is related to direct contact (portal of entry) effects. This adjustment might also be relevant for the interindividual TK sub-factor (discussed below).
Recognizing that interspecies differences reflect both toxicokinetics and toxicodynamics opens the possibility of refining this factor with known differences in physiology. One approach to address TK differences is through allometric scaling. Allometric scaling provides an estimate of differences in physiological parameters and basal metabolism (and therefore chemical metabolism and elimination) based on ratios of body weight or surface area. An FDA guidance document on setting doses in initial clinical trials includes species-specific extrapolation factors for estimating human-equivalent doses, based on allometric scaling.(Citation23) These are sometimes used for setting OELs for pharmaceuticals. The use of allometric scaling based on body surface area, or body weight, to the 2/3 power (BW0.67) provides a human-equivalent dose used to improve clinical trial safety.(Citation23) These species-specific values range from 2 (dog) to 12 (mouse), due to differences in body surface area.(Citation24,Citation25) The EPA uses a factor that more closely aligns with interspecies differences in physiological parameters and metabolism. Allometric scaling using the body weight to the ¾ power (BW0.75) results in species-specific factors that range from 1.5 (dog) to 7 (mouse).(Citation26) An analysis by Bokkers and Slob(Citation27) suggests that an additional UF of 3.1 should be applied to the allometrically scaled dose, based on the 95th percentile of the allometric extrapolation factor. Vermeire et al.(Citation3) reached a similar conclusion. Although not specifically attributed to toxicodynamics by the authors, this additional UF would be numerically similar to the addition of a TD sub-factor to the allometrically scaled TK sub-factor. A recent analysis by Escher et al.(Citation28) supports the use of allometric scaling for interspecies extrapolation, but argues that the remaining uncertainties may be due to factors other than toxicodynamics. In practice, there is no consensus on the need for an additional sub-factor to address toxicodynamic differences, after applying the factor based on these allometric scaling methods.
There are additional advanced dosimetry techniques for refining the UFA, typically for replacing the TK portion of this factor with data based on physiological differences among species.(Citation13) For assessments based on a PoD adjusted using inhalation dosimetry, another consideration is whether the methods address all key aspects of TK variability. For example, methods that rely only on predicted deposition fractions or surface concentrations, may not fully address other kinetic processes related to metabolism and elimination. In part, such considerations can be addressed using advanced approaches that estimate the pertinent target tissue dose. A recently published physiologically-based pharmacokinetic (PBPK) model for diacetyl(Citation29) illustrates the potential for such models to replace default TK assumptions for interspecies scaling; the model indicates that the lower respiratory tract of a worker exposed to diacetyl may receive exposures up to 40-fold greater than the lower respiratory tract of a rat inhaling the same concentration.
Interindividual (Human) Variability Uncertainty Factor (UFH)
The interindividual (human) variability uncertainty factor (UFH) accounts for general physiological and metabolic variation within the human population and the possibility that there may be sensitive subpopulations due to variations in age, sex, genetic susceptibility, pre-existing diseases, etc. In general, the OEL is intended to protect nearly all workers, but may not always be protective of the most susceptible individuals in the population. For example, Johansson et al.(Citation30) showed that experimental data on asthmatics generally have not been explicitly considered when setting OEL values; however, this has changed with several recent ACGIH TLV®s. A complication to defining UFH is that the fraction of workers “nearly all” refers to is not well documented in the OEL methods or derived OELs published to date. A key consideration is the relative size of the subpopulation that might be affected. A second consideration is the severity of effects that might occur in subpopulations that have a specific unique susceptibility if exposed at the OEL. The possibility for severe reactions would favor coverage by the OEL. Alternatively, the severity of effect along with residual uncertainties regarding susceptible subpopulations may be addressed by addition of a modifying factor (see below).
The UF for interindividual variability is used to adjust PoD values derived from either animal or human data. In the case of human data, it may be the only UF that is required. A default UF as high as 10 is typically used for the general population in the absence of chemical specific data(Citation19) (see below for further information). Like the UFA, the UFH is often conceptualized as being composed of two sub-factors, with one sub-factor for TK differences between individuals and the other sub-factor for differences in suceptibility at a given tissue dose (i.e., TD differences). Unlike the UFA, the TK and TD sub-factors of the UFH are generally assigned numerical values of √10, or approximately 3.(Citation20–Citation22)
The scientific basis for the UFH has been reviewed.(Citation17–Citation19,Citation31–Citation34) It has been noted that a TK sub-factor of √10 may not be adequate for all groups of the population.(Citation32) A TK sub-factor of √10 has been shown to be adequate for ≥ 99% of healthy adults when polymorphic pathways are not involved; however, sensitive sub-groups may require a larger TK sub-factor.(Citation35) It has also been argued that an overall UFH of less than 10 should be adequate for setting OELs, on the grounds that workers are expected to be less heterogeneous than the general population because they do not include the very old and very young, and they are not exposed for an entire lifetime.(Citation36,Citation37) Epidemiological studies of working populations generally find that, in the absence of excess exposures to toxicants, workers are healthier than the general population. This is known as the “healthy worker effect,” which is well established in occupational epidemiology.(Citation38,Citation39) However, working populations may also include asthmatics, atopic individuals, pregnant women, older workers, and others who may be more susceptible than the average member of the population.
Genetic and epigenetic factors can be an important driver for variability in response to chemicals. This has been well described in the context of variability in metabolism of pharmaceuticals. Implications for similar considerations for occupational chemical exposures are also an important variable in setting OELs, since one of the primary UFs is assigned to address human variability. An approach for systematic consideration of genetic and epigenetics is discussed in detail by Schulte et al.(Citation40)
The European Chemicals Agency recommends(Citation41) a factor of 5 to address interindividual variability in workers and a factor of 10 for the general population when establishing derived no-effect levels (DNELs) (see ). The basis for this distinction is not well documented, although the literature on chemical-specific adjustment factors (CSAF), as discussed below, seems to support this dichotomy. For example, a reanalysis of the TK data for pharmaceutical active ingredients (APIs) analyzed by Naumann et al.(Citation42) and Silverman et al.(Citation43) show that the mean ratios of the bimodal CSAFs for elderly or diseased subpopulations are a factor of 2.2 higher compared with the healthy populations for the same APIs. If similar toxicodynamics are assumed, a 2-fold reduction in the default UFs of 10 for interindividual variability appears justified for workers.
It is a valid scientific question whether a full default value of 10 for the UFH is appropriate to account for variability in susceptibility for working populations, and in the absence of a definitive scientific rationale, organizations may well establish differing policies on this point. The rationale for determining this relies on information on the underlying basis for susceptibility to the specific effects caused by the chemical under study. The types of considerations that are embedded in the selection of UFH include the following.
Is the underlying basis for susceptibility more or less prevalent in the worker population than in the general population?
Is there a basis for assuming that a working population would experience more severe or less severe toxic effects than the general population?
LOAEL-to-NOAEL Uncertainty Factor (UFL)
When the key study used to derive an OEL includes a LOAEL but not a NOAEL, the preferred procedure is to calculate a benchmark dose (BMD) to use as the PoD for extrapolation.(Citation12) The benchmark dose (lower confidence limit), or BMDL, typically approximates the NOAEL for standard study designs and is used similarly to the NOAEL as a PoD. If a NOAEL or BMDL is used as the PoD, a value of 1 is typically applied for this area of uncertainty in health risk assessments,(Citation14,Citation41) since the goal of the extrapolation is to estimate a concentration that is not associated with adverse effects. However, some datasets may be unsuitable for benchmark dose estimation. If an OEL is based on the LOAEL as the PoD for extrapolation, an additional UF (UFL) should be applied to estimate a point on the dose-response curve where no effect would be expected to occur.
Some judgment may be required in selecting an appropriate UFL. Dourson and Stara(Citation17) conducted an empirical analysis of LOAEL:NOAEL ratios, and they found that 96% of the cases they considered fell within a factor of 10. Naumann and Weideman(Citation18) noted that the average LOAEL:NOAEL ratio is approximately 3, based on analyses of a large number of good quality toxicology studies.(Citation44–Citation46) Thus, the choice of a default value for the UFL may depend, to some extent, whether organizational policy favors an upper-bound estimate of risk or a central tendency estimate. These analyses do have the limitation of analyzing NOAEL and LOAEL ratios, which are pre-defined by study dose-spacing for individual studies. This is an inherent challenge in the PoD selection, and is the reason BMD methods are generally preferred.(Citation12)
In choosing a value other than the default value, several considerations are relevant. The most common basis for using a different value is the severity of the effect at the LOAEL. In addition, some OEL setting processes also incorporate the use of a lowest-observed-effect level (LOEL) as the basis for the PoD. For example, for setting exposure guides for pharmaceutical agents, the most sensitive effect is often based on a pharmacological effect (which is the often the intended effect for patients). However, for workers, such effects are candidates for the PoD, since the effects are not intended outside of therapeutic treatment. In this light, pharmacological effects are typically viewed as “adverse,” although clinical study documentation often refers to them as a LOEL rather than a LOAEL. Whether the critical effect is termed a LOAEL or LOEL, the key determinates of UFL selection remain the same (effect severity and dose-response slope). Dourson and Stara,(Citation17) Naumann and Weideman,(Citation18) and Dourson et al.(Citation19) agree that the severity of the effect at the LOAEL should enter into the choice of UFL, on the grounds that an effect of minimal toxicological severity, such as fatty liver, suggests that the LOAEL is likely to be close to the NOAEL, while a more severe effect, such as hepatic necrosis, suggests that the LOAEL may be further from the NOAEL. Other considerations can include response incidence, the slope of the dose-response curve, and the MOA (anticipated dose-response behavior). Any residual uncertainties can be addressed with the modifying factor (see below).
Shorter-Term-to-Longer-Term Uncertainty Factor (UFS)
OELs are intended to protect workers who are exposed for an entire working lifetime, which for animal studies are usually equated to a chronic bioassay (e.g., 2 years for mice and rats). If the PoD is based on sub-chronic data, then the UFS is applied to adjust for the lack of adequate chronic exposure data based on the consideration that the OEL is intended to be protective of a working lifetime. The default value of the UFS has been the subject of some debate in the literature. Dourson and Stara(Citation17) argued for a default factor of 10, citing studies that indicated a 10-fold factor would equal or exceed the observed ratio of subchronic-to-chronic effect levels 96% of the time. Naumann and Weideman(Citation18) advocated a default UFS value of 3, noting that reviews of a large number of high quality toxicology studies suggest that a factor of 3 is typically sufficient to account for the possibility that a lower PoD would have resulted if longer-term studies were conducted.(Citation47–Citation52) Dourson et al.(Citation19) advocate a more flexible approach in which a UFS of 10 is seen as “a loose upper bound estimate,” which may be reduced if the chemical-specific data suggest that a smaller UFS is warranted.
Assessing the appropriate value of UFS requires evaluating whether the critical effect that is the basis of the PoD would be expected to increase in either incidence or severity, or to occur at a lower dose, if the study duration was increased. It is possible that a reversible acute effect may be experienced on a chronic basis without any increase in severity. The progression or lack of progression of toxicity from short-term studies to longer studies, such as a 2-week study compared with a 90-day study, may provide some data relevant to assessing the UFS. The MOA may also provide relevant information. A full assessment of MOA is based on whether the dose would have likely accumulated with longer-term exposure (i.e., TK considerations) and whether effects would accumulate and become more severe with longer-term exposure (i.e., TD considerations). For example, a chemical that produces damage via a reactive metabolite (which does not accumulate in the body) and does not show evidence of a progression of damage over time may merit a reduced UFS. Sensory irritant effects are generally not expected to progress with longer exposure duration, and OELs for these are often assigned a UFS of 1. Chemicals studied for durations longer than the typical 3-month sub-chronic study, but less than chronic duration (e.g., a 6-month or 1-year study) may also merit a UFS < 10. The choice of the UFS for a particular chemical must include consideration of both the quality of the available data and the MOA of the chemical.
Database Inadequacy (incomplete data) Uncertainty Factor (UFD)
An additional UF may be required if the overall toxicity database is incomplete, and significant data gaps suggest the possibility that a lower PoD would be identified for unstudied toxic endpoints. Such considerations often arise if data are available from only one species, or if developmental and reproductive toxicity studies have not yet been performed. Although not all OEL-setting organizations include an explicit UFD, this factor is intended to account for the possibility of finding a lower PoD once the additional studies are performed. Dourson et al.(Citation53) reviewed chronic toxicity data for pesticides and concluded that an adequate database would consist of 2-year studies in the rat and dog, plus reproductive and developmental studies in the rat. It is unusual to have 2-year dog study data for OEL setting purposes; 2-year studies in mice and rats are more common. The available data suggest a UFD of 3–10 for database inadequacy may be needed if an OEL is based on datasets that lack longer-term studies in two species plus reproductive and developmental studies.(Citation19)
The decision to apply a UFD should be based on considering the specific types of data available for the chemical in question and judging the likelihood that additional studies would reveal additional toxicity. In some cases, existing data may suggest the possibility of an effect that can only be reliably tested using a special study design that has not been conducted. For example, indications from standard study designs of concerns related to neurological effects or effects on the immune system might suggest the need for special functional or endpoint-specific studies. If absent, such data gaps are often used to support a larger UFD. It is not unusual in OEL-setting to have the benefit of human studies, which though inadequate for establishing an OEL due to lack of reliable exposure measurements, may shed light on the hazards associated with the chemical. For example, a dataset lacking reproductive and developmental studies in animals may be considered inadequate if the available human data suggest a potential reproductive hazard, but adequate (UFD = 1) if the human data are sufficient to rule out concern for reproductive or developmental toxicity.
A common data gap in setting OELs is the absence of toxicity data from exposure by the inhalation route; many OELs are derived based on oral-dosing studies. This reflects testing designs for actual end-product use scenarios where ingestion and skin application dominate. There are scientific principles that underpin the circumstances when route-to-route extrapolation is biologically justifiable.(Citation54) However, examining the uncertainty generated by such route-to-route extrapolations is necessary. Common considerations include the degree to which an observed systemic toxicity effect is likely to be more sensitive than portal of entry effects. This is a major concern because a very common endpoint for current OELs for industrial chemicals is respiratory tract irritation.(Citation55) In some cases the potential for such effects might be inferred from irritation assays tested via other routes, Citationalthough relying on correlations between endpoints such as dermal irritancy and irritation of the respiratory tract is not ideal. The need for adjustments for route-dependent bioavailability is also considered. In some cases adjustments for this consideration can be handled via application of dosimetry adjustments or through the use of absorption factors in the OEL equation.(Citation5,Citation13,Citation56) When there are a priori concerns regarding differences in bioavailability via different routes, and data are not available to address quantitative adjustments for route considerations, the UFD might be increased for this purpose. Alternatively, residual uncertainties regarding bioavailability can be addressed with the modifying factor.
The Composite Uncertainty Factor (UFC)
The value of typical defaults used varies among organizations. Organizations that derive environmental standards in the United States to protect the general population (e.g., EPA reference doses [RfDs] and Agency for Toxic Substances and Disease Registry [ATSDR] minimal risk levels [MRLs]), tend to favor order of magnitude-based adjustments of 1 or 10, using an intermediate value of 3. Note that in such cases the value of 3 represents one-half of the log10 unit (3.16 rounded to 3) as the minimum increments that are used for the UF adjustments to reflect the level of precision for such an approach. Many European agencies also use a value of 10 to account for a full area of uncertainty, but they tend to favor intermediate values of 2 and 5 in their calculations. In some cases, other alternative defaults are provided for specific data extrapolations (e.g., the assessment factors used for the derived-no-effect level [DNEL] under chemical registration requirements in the EU).
After the numerical values of the various UFs have been determined, they are then multiplied to yield the overall composite UF, UFC. For example, default values of 4 for UFA and 10 for UFH would be multiplied to yield a UFC of 40, which would then be applied to the PoD as described above. Note that although the value of 3 is used in place of 3.16 during the discussion of default UFs, caution should be applied when multiplying UFs of 3 together. For example, when multiplying two UFs of 3 together, where each factor represents a half-log adjustment (e.g., 3.16 × 3.16), the product will be 10, not 9. This is also illustrated by multiplying three UFs of 3, that is, 3 × 3 × 3 will equal 30, not 27.
Assuming default values for the UFA, UFH, UFS, and UFL, the numeric value of the UFC could conceivably be very large. Although this multiplicative approach to setting the UFC would be correct if the various UFs were strictly independent of one another, it has been argued that the various UFs are not entirely independent and that simply multiplying default factors of 10 can lead to double-counting of some sources of uncertainty. Reducing some of the UFs to adjust for the presumed double-counting has been proposed(Citation57) and verified empirically by Swartout et al.(Citation58) Current EPA guidelines(Citation22) for applying UFs for determining reference doses for the general population also assume that an overall UFC of 10,000 is likely to be more than adequately protective, and therefore UFC is limited to a value of 3,000 even if four full areas of uncertainty are present (i.e., the UFA, UFH, UFS, and UFL are all assigned values of 10). Moreover, if five full areas of uncertainty are present, it is likely that the data are not sufficient to derive an exposure limit with adequate confidence.
Probabilistic approaches for defining combinations of UFs have also been proposed.(Citation58–Citation60) In general, these lead to smaller values of the total UFC than those obtained by multiplying the default values of UFs. Most recently, Hasegawa et al.(Citation61) proposed an alternative set of default UFs, based on probabilistic combinations of empirically-derived UFs. These alternative UFs are in some cases larger than the usual 10-fold default UFs, but in other cases less than 10. Although these probabilistic combinations of UFs have to date not attained wide regulatory acceptance, they may provide a framework for refining existing practices for establishing the overall UFC. The impacts of probabilistic UFs in interpreting occupational risk are further described by Waters et al.(Citation62)
The nature and level of documentation of the UF application in deriving the OEL is highly variable among the organizations that publish OELs. The OEL documentation published by leading organizations for Threshold Limit Values (TLV®), Workplace Environmental Exposure Levels (WEELs), and values published by the EU Scientific Committee on Occupational Exposure Limits (SCOEL) describe the rationale for the OEL, noting considerations and uncertainties. An explicit description of the individual UFs or values used in deriving the OEL is not provided, although many cases the composite is given. In contrast, risk values such as environmental or emergency airborne limits established by most government organizations (e.g., EPA Reference Concentrations [RfC], Acute Emergency Guideline Levels [AEGL], limits derived by U.S. state agencies; and EU DNEL values including for occupational scenarios) have a documented uncertainty factor approach, and the values applied to derive a given value are typically enumerated. There are merits and limitations of each approach for calculating a composite UF and enumerating specific default component subfactors () and hybrid approaches have also been proposed.(Citation63) However, overall the trend is toward increased documentation (at least qualitatively) of the UF choices in the rationale for the OEL.
Table 3 Merits and Limitations of Alternative UF Documentation Approaches
Toxicokinetic Adjustment Factor
As an alternative to using dosimetry models to modify the PoD,(Citation13) there are additional adjustments that modify the denominator of the OEL equation that some organizations use to reflect specific toxicokinetic considerations. For example, ECHA has predefined assessment factors for correction of the PoD to consider differences in dosing route and the exposure route for the worker exposure scenario.(Citation41) Two other common adjustments applied by some organizations (and in particular the pharmaceutical sector) reflect route-specific bioavailability and extrapolation to address steady-state internal dose conditions.(Citation5) In certain cases, route-to-route extrapolation might be appropriate when attempting to derive an OEL from a study conducted by a route (e.g., oral) that is different from the potential route of exposure (i.e., inhalation). Although direct studies using the relevant route of administration are normally expected, extrapolation using an additional adjustment factor (e.g., bioavailability correction factor) may be useful to ensure adequate protection in certain situations.
Naumann and Weideman(Citation18) and Naumann et al.(Citation56) provided the background on the use of bioavailability correction factors and guidance on when and how they should be applied for OEL development. Absent a validated TK model or a risk assessment requiring the use of a predetermined default, the technical approach most commonly employed for the bioavailability adjustment reflects a ratio of route-specific bioavailability data.(Citation5,Citation64) When the adjustment factor is placed in the denominator of the OEL equation it can be calculated as:
For example, if an OEL were derived based on a critical effect derived from an oral dosing study with a systemic bioavailability of 10% and the bioavailability by the inhalation route was 100%; the resulting bioavailability adjustment factor would be 1.0/0.1 or a factor of 10. This adjustment, therefore, serves as an estimate of the inhalation equivalent to the oral PoD on a systemic dose basis, i.e., the OEL is lowered by this factor.
In practice, such adjustments for bioavailability often have significant limitations based on simplifying assumptions that must be made in the absence of route-specific bioavailability data. Several limitations to the application of such a factor include:
The adjustment is not applicable for OELs based on effects at the portal of entry—in such cases extrapolation across routes is not recommended.
The adjustment relies on reliable estimates of systemic bioavailability. Surrogates for total systemic dose (e.g., penetration rates) are not adequate metrics for systemic dose since they do not take into account other toxicokinetic properties such as local or hepatic first-pass metabolism.
If the form of the chemical that causes toxicity (e.g., parent or metabolite) is not known then the bioavailability adjustments based on parent compound may not reflect actual route-specific differences in toxic potency. This consideration is common for chemicals that undergo significant hepatic first-pass metabolism following oral dosing.
Unless inhalation is the primary route of exposure of concern for consumer or environmental exposures for a chemical, TK data following inhalation is not likely to be available. Thus, the adjustment may be based on empirical bioavailability estimates for the dose route for the critical study that served as the PoD, but the bioavailability for inhalation (the route of interest for the OEL) must be estimated. One approach to address this limitation is to assume 100% inhalation bioavailability (for most small organic molecules) as a precautionary assumption or to estimate a value based on properties such as partition coefficients.
Estimating inhalation bioavailability for large molecules (e.g., protein therapeutics) adds additional complexity that needs to take into account factors such as molecular weight, the presence of specific receptors that facilitate epithelial transport, and bioavailability from the gastrointestinal tract following mucociliary clearance and swallowing.
Additional factors may be needed to adjust OELs because of the potential for bioaccumulation with repeated exposure. For chemicals with long elimination half-times, plasma levels increase with repeated exposure until they reach steady state. The OEL established using a NOAEL from a short-duration study would need to be adjusted downward by a factor corresponding to the ratio of the plasma level following a short-term exposure (duration equivalent to the study used to identify the PoD) to the expected plasma level at steady state following long-term exposure. This adjustment is of specific utility in setting OELs for pharmaceuticals where single dose or short-duration clinical studies are often available. OELs derived from animal toxicology studies typically require repeat-dose studies where steady-state kinetics apply before an OEL would be derived. This bioaccumulation adjustment is not necessary if the OEL is established using studies of sufficient length such that the steady state at the maintenance dose is achieved, because any accumulation has already been taken into account under such conditions.
Modifying Factor (MF)
This factor adjusts for uncertainties not addressed by the UFs described above, and it allows for explicitly incorporating scientific judgment, especially when there are multiple reviewers. A modifying factor may also be considered if there is a need to address residual uncertainties not covered by the other factors. This factor also allows for scientific judgment to be applied to address the overall quality of the database and relevance of available studies to human risk assessment. Additional factors of <1 to 10 may be used in these cases.(Citation19) Explicitly incorporating a modifying factor (MF), along with the rationale for including it, may promote increased transparency of the decision-making process, leading to the final OEL. However, current EPA guidance is to forego the specific use of this factor and incorporate the uncertainties it addresses within another factor, such as UFD.(Citation22)
Modifying factors may be used to make further adjustments that the reviewer deems necessary to cover situations that other factors do not clearly or adequately address. Some considerations that are often cited for application of a MF include the following.
The slope of the dose-response curve for the critical effect.
The choice of the critical effect.
The severity of the effect.
Route-to-route extrapolation.
Identification of susceptible subpopulations.
Clinical significance of the critical effect.
Reversibility of the critical effect.
Overall quality of the database.
Relevance of the critical effect to workers.
Similarity or differences with related chemicals.
Lack of independence between individual uncertainty factors.
Chemical-Specific Adjustment Factors (CSAFs) in OEL Setting
Default uncertainty factors, by definition, should be used only in the absence of relevant data. Chemical-specific adjustment factors (CSAFs) are intended to replace the default factors where TK and TD data are available, according to the scheme proposed by Renwick(Citation65–Citation67) and adopted by the World Health Organization/International Programme on Chemical Safety.(Citation21,Citation68)
CSAFs can be used to replace default uncertainty factors to establish safe levels of exposure whenever sufficient chemical-specific data are available. The Renwick scheme provides a framework for incorporating chemical-specific TK and TD data when deriving regulatory and internal health-based limits, such as acceptable daily intake values, tolerable intake values, reference doses/concentrations, and occupational exposure limits. Renwick(Citation67) listed a number of considerations for use of chemical-related data, including whether the chemical itself or a metabolite is the active species, the relevance of the TK or TD data to the critical endpoint, and how representative the data are of the human population. Meek et al.(Citation69) have also proposed guidelines on the use of data-derived adjustment values to replace default uncertainty factors when sufficient chemical-specific data are available.
The distinction between uncertainty and variability should be emphasized. CSAFs are used to characterize the “variability” in TK and TD in a relevant subset of the intended population. To the extent that this subset is representative of the overall population and provides quantitative information on differences (or similarities), the level of “uncertainty” should be reduced considerably. The risk assessors/risk managers should feel more confident that the health-based limit they derive will provide adequate protection for even the most susceptible individuals. There may be residual uncertainties; however, these would obviously be less than when no data are available on potentially important differences. By focusing on TK and TD data for potentially susceptible subpopulations, the risk assessor consciously evaluates data for the segment of the population in greatest need of protection.
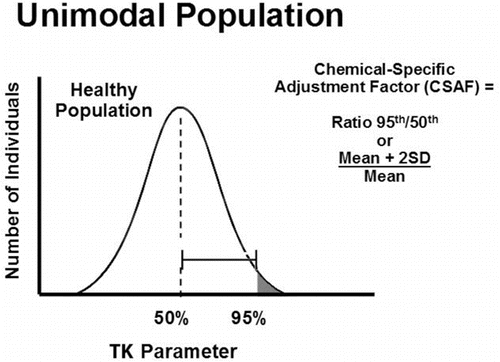
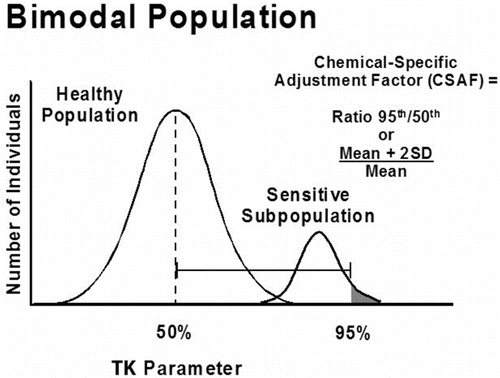
Within the CSAF scheme, interindividual differences are assessed using several TK and TD parameters indicative of systemic exposure and pharmacologic activity of the chemicals evaluated. The TK parameters initially chosen as measures of internal dose were peak plasma concentration (Cmax), area under the curve of blood concentration by time (AUC), steady-state plasma concentration (SS), and elimination or clearance rates. The first two, Cmax and AUC, are considered the best indicators of body burden and systemic exposure because they are direct measures of the amount of chemical in the blood, although clearance rates are also commonly used metrics in the CSAF method.
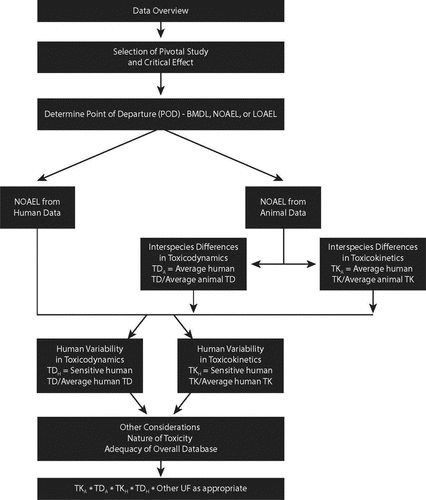
The general guidelines(Citation21,Citation69) for evaluating TK and TD data include some important considerations for choosing CSAFs. For assessing interindividual differences in TK, (1) the TK parameter used should be directly related to the critical effect (e.g., AUC vs. Cmax), (2) the data should be generated with the dose and route most relevant to the recommended health-based limit, and (3) data from human subjects should be representative of the expected variability in the population being protected (workers, in this case age 18–65), including any sensitive subpopulations. For assessing interindividual differences in TD, (1) the measured endpoint must be directly related to the critical effect used to derive the recommended exposure limit, (2) the data must include a quantitative comparison of the response in tissues at a similar level of response from average and sensitive humans, and (3) a sufficient number of individuals must be included to define interindividual variability. The WHO/IPCS guidance documents (Citation21,Citation68) provide examples of how CSAFs are derived for all four sub-factors.
The method for estimating interindividual differences and deriving CSAFs relies on the ratio of the tail of the distribution to the central tendency for the TK or TD parameter of interest. The approach is based on the premise that if a subpopulation (e.g., tail of the distribution) is sufficiently different (i.e., more susceptible based on significantly higher Cmax or AUC values for a given dose), their level of exposure needs to be adjusted downward to conform to the normal (average) healthy individual. Where two or more distinct subpopulations exist, the ratio of the upper tail of the most sensitive subpopulation over the mean of the healthy population is used to derive an appropriate adjustment factor. This is illustrated graphically in (transformed unimodal distribution) and (transformed bimodal distribution).
In practice, evaluating interindividual differences typically relies on papers presenting only summary statistics (e.g., mean and standard deviation) for TK and/or TD parameters. A CSAF is calculated by dividing the mean plus two standard deviations by the mean (Mean + 2SD)/Mean). Occasionally, standard errors need to be converted to standard deviations by multiplying the former by √N.
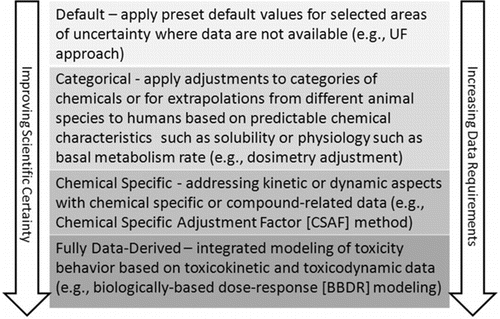
A case study was published to illustrate how CSAFs can be used to derive occupational exposure limits.(Citation70) The case study involved timolol maleate, a non-selective beta-adrenergic blocking agent, and it shows how a polymorphism in oxidative metabolism by CYP2D6 with two distinct phenotypes (i.e., poor and extensive metabolizers) was evaluated to take this potential susceptibility into account when establishing the OEL. The prevalence of poor metabolizers (up to 9% of selected subpopulations) is high enough to indicate careful consideration of this potential susceptibility when establishing the OEL for timolol maleate. A chemical-specific adjustment factor (CSAF) for kinetics of 9.8 was calculated for this bimodal distribution by dividing the value corresponding to two standard deviations above the mean AUC value for poor metabolizers by the mean AUC for the extensive metabolizers, (MeanPoor + 2SD)/MeanExt), as shown in . When combined with a CSAF for dynamics of 1.2, this yields a composite CSAF of 12 to address human variability in sensitivity. The CSAF was applied to the extrapolated no-effect level for clinically significant cardiovascular effects (with correction for oral bioavailability) to establish an occupational exposure limit (OEL) for timolol maleate that is expected to be protective of nearly all workers, including those who may be poor metabolizers.
It is important to note that use of CSAFs does not automatically imply a reduced “safety factor” used in setting health-based limits. Sometimes the available data for a given chemical suggest a high level of interindividual variability. Note that, in the example above, the composite uncertainty factor derived using the CSAFH-TK combined with the default for toxicodynamics (CSAFH-TD) of 3.2 would have been 31, which is 3-fold higher than the default value of 10. The overall process for applying the CSAF approach in the context of setting exposure limits is shown in .
CONCLUSION
The application of UFs in deriving OELs has evolved considerably in recent decades. Although originally seen as a somewhat arbitrary safety factor, based primarily on professional judgment, application of UFs has moved towards an increasingly strong dependence on scientific evidence —first in regard to scientifically-based defaults,(Citation17,Citation18,Citation31,Citation71) and more recently in developing chemical-specific values.(Citation21,Citation42,Citation43,Citation70,Citation72) Increasingly, it is the application of chemical-specific data that is seen as the preferred “default” method, with the application of standard values of UFs limited to those situations in which an OEL must be derived in the absence of chemical-specific data. Thus, there is a preferred hierarchy of UF approaches (). Despite this trend for the majority of industrial chemicals detailed TK data to support CSAF calculation may not be available.
This article outlines the scientific basis for the most commonly used UFs, (i.e., the UFs for animal-to-human extrapolation, intra-individual variability, extrapolation from shorter-term to longer-term exposures, extrapolation from a LOAEL to a NOAEL, and adjustments for an incomplete database). Because the application of scientific judgment cannot always be avoided in the OEL-setting process, such judgments should be open and transparent, and they should systematically evaluate key areas of uncertainty, incorporating chemical-specific data when available, and clearly documenting the rationale for final decisions that are used in the OEL. The science of UF application is continuing to improve. Advancements in the scientific development of UF application include the following:
greater expectation for transparency and description of the basis for UF selection, including the quantitative values that were applied;
improved application and access to more robust methods (e.g., CSAFs and bioavailability correction factors) because tools are better, access to data is greater, and familiarity with methods is increasing;
an understanding of the basis for human variability in sensitivity (e.g., genetics and epigenetics), and more complete consideration in OEL setting, with a goal of moving toward fully data-derived methods of OEL derivation; and
application of advances in toxicology, including computational toxicology approaches to refine UF values in a quantitative manner.
ACKNOWLEDGMENTS
The authors thank the following for their helpful comments and suggestions on earlier drafts: Ed Sargent, Linda Schenk, Paul Schulte, and Christine Sofge.
REFERENCES
- Lehman A.J., and O.G. Fitzhugh: 100-Fold margin of safety. Assoc. Food Drug Off. U. S. Q. Bull. 18:33–35 (1954).
- Fairhurst S.: The uncertainty factor in the setting of occupational exposure limits. Ann. Occup. Hyg. 39:375–385 (1995).
- Vermeire, T., H. Stevenson, M.N. Pieters, M. Rennen, W. Slob, and B.C. Hakkert: Assessment factors for human health risk assessment: a discussion paper. Crit. Rev. Toxicol. 29(5):439–490 (1999).
- Zielhuis, R.L., and F.W.van derKreek: The use of a safety factor in setting health based permissible levels for occupational exposure. I. Int. Arch. Occup. Environ. Health 42:191–201 (1979).
- Sargent, E.V., and G.D. Kirk: Establishing airborne exposure control limits in the pharmaceutical industry. Am. Ind. Hyg. Assoc. J. 49(6):309–313 (1988).
- Agius, R.: Occupational exposure limits for therapeutic substances. Ann. Occup. Hyg. 33:555–562 (1989).
- Galer, D.M., H.W. Leung, R.G. Sussman, and R.J. Trzos: Scientific and practical considerations for the development of occupational exposure limits (OELs) for chemical substances. Regul. Toxicol. Pharmacol. 15:291–306 (1992).
- Nielsen, G.D., and S. Øvrebø: Background, approaches and recent trends for setting health-based occupational exposure limits: A minireview.Regul. Toxicol. Pharmacol. 51:253–269 (2008).
- Henschler, D.: Evaluation of adverse effects in the standard-setting process. Toxicol. Lett. 64–65 Spec No:53-7 (1992).
- National Institute for Occupational Safety and Health (NIOSH): NIOSH Criteria for a Recommended Standard: Occupational Exposure to Respirable Coal Mine Dust. Cincinnati, OH: U.S. Department of Health and Human Services, Centers for Disease Control and Prevention, National Institute for Occupational Safety and Health, DHHS (NIOSH) Publication No. 95-106 1995.
- National Research Council (NRC): Science and Decisions: Advancing Risk Assessment. Washington, D.C.: National Academies Press 2009.
- Wheeler, M.W., R. Park, A.J. Bailer, and C. Whittaker: Historical context and recent advances in exposure-response estimation for deriving occupational exposure limits. J. Occup. Environ. Hyg. 12(S1):S7–S17.
- Kuempel, E., L. Sweeney, J. Morris, and A. Jarabek: Advances in inhalation dosimetry models and methods for occupational risk assessment and exposure limit derivation. J. Occup. Environ. Hyg. 12(S1):S18–S40.
- Environmental Protection Agency (EPA): Methods for Derivation of Inhalation Reference Concentrations and Application of Inhalation Dosimetry. Washington, D.C.: Office of Health and Environmental Assessment. EPA/600/8-90-066F 1994.
- Haber, L., and A. Maier: Scientific Criteria Used for the Development of Occupational Exposure Limits for Metals and Other Mining-Related Chemicals.Regul. Toxicol. Pharmacol. 36:262–279 (2002).
- Deveau, M., C.-P. Chen, G. Johansen, et al.: The global landscape of occupational exposure limits—Implementation of harmonization principles to guide limit selection. J. Occup. Environ. Hyg. 12(S1):S127–S144 (2015).
- Dourson, M.L., and Stara, J.F.: Regulatory history and experimental support of uncertainty (safety) factors. Regul. Toxicol. Pharmacol. 3:224–238 (1983).
- Naumann, B.D., and P.A. Weideman: Scientific basis for uncertainty factors used to establish occupational exposure limits for pharmaceutical active ingredients.Hum. Ecol. Risk Assess. 1:590–613 (1995).
- Dourson, M.L., S.P. Felter, and D. Robinson: Evolution of science-based uncertainty factors in noncancer risk assessment. Regul. Toxicol. Pharmacol. 24:108–120 (1996).
- World Health Organization, International Programme on Chemical Safety (WHO/IPCS): Assessing Human Health Risk of Chemicals: The Derivation of Guidance Values for Health-Based Exposure Limits. Environmental Health Criteria 170. Geneva: World Health Organization, International Programme on Chemical Safety, 1994.
- World Health Organization, International Programme on Chemical Safety (WHO/IPCS): Chemical-Specific Adjustment Factors for Interspecies Differences and Human Variability: Guidance Document for Use of Data in Dose/Concentration Assessment. IPCS Harmonization Project Document No. 2, Geneva: World Health Organization, International Programme on Chemical Safety, 2005; . http://whqlibdoc.who.int/publications/2005/9241546786_eng.pdf (accessed May 6, 2010).
- Environmental Protection Agency (EPA): A review of the Reference Dose (RfD) and Reference Concentration (RfC) processes. Risk Assessment Forum. EPA/630/P-02/002F, December 2002.
- Food and Drug Administration (FDA): Guidance for Industry: Estimating the Maximum Safe Starting Dose in Initial Clinical Trials for Therapeutics in Adult Healthy Volunteers. Center for Drug Evaluation and Research (CDER), 2005; http://www.fda.gov/cder/guidance/index.htm (accessed October 18, 2011).
- Davidson, I.W.E., J.C. Parker, and R.P. Beliles: Biological basis for extrapolation across mammalian species. Regul. Toxicol. Pharmacol. 6:211–237 (1986).
- Mordenti, J., and W. Chappell: The use of interspecies scaling in toxicokinetics. In: Toxicokinetics and New Drug Development, A. Yacobi, J.P. Skelly, and V.K. Batra (eds.). New York, Pergamon Press, 1989. pp. 42–96.
- Environmental Protection Agency (EPA): Recommended Use of Body Weight ¾ as the Default Method in Derivation of the Oral Reference Dose, Office of the Science Advisor, Risk Assessment Forum. Washington, D.C.: U.S. Environmental Protection Agency (2005).
- Bokkers, B.G.H., and W. Slob: Deriving a data-based interspecies assessment factor using the NOAEL and the benchmark dose approach. Crit. Rev. Toxicol. 37:355–373 (2007).
- Escher, S.E., M. Batke, S. Hoffmann-Doerr, H. Messinger, and I. Mangelsdorf: Interspecies extrapolation based on the RepDose database—A probabilistic approach. Tox. Lett. 218:159–165 (2013).
- Gloede, E., J.A. Cichocki, J.B. Baldino, and J.B. Morris: A validated hybrid computational fluid dynamics-physiologically based pharmacokinetic model for respiratory tract vapor absorption in the human and rat and its application to inhalation dosimetry of diacetyl. Toxicol. Sci. 123(1):231–246 (2011).
- Johansson, M., G. Johanson, and M. Öberg: How are asthmatics included in the derivation of guideline values for emergency planning and response? Regul. Toxicol. Pharmacol. 63:461–470 (2012).
- Calabrese, E.J.: Uncertainty factors and interindividual variation. Regul. Toxicol. Pharmacol. 5:190–196 (1985).
- Renwick, A.G., and N.R. Lazarus: Human variability and noncancer risk assessment—An analysis of the default uncertainty factor. Regul. Toxicol. Pharmacol. 27:3–20 (1998).
- Dourson, M.L., G. Charnley, and R. Scheuplein: Differential sensitivity of children and adults to chemical toxicity: II. Risk and regulation. Regul. Toxicol. Pharmacol. 35:448–467 (2002).
- Institute for Environment and Health (IEH): Variability and Susceptibility in Human Response to Occupational Exposure to Chemicals in the UK. Report R13. Leicester, UK: Medical Research Council Institute for Environment and Health, 2002.
- Falk-Filipsson, A., A. Hanberg, K. Victorin, M. Warholm, and M. Wallén: Assessment factors—Applications in health risk assessment of chemicals. Environ. Res. 104:108–127 (2007).
- Schenk, L., and G. Johanson: Use of uncertainty factors by the SCOEL in their derivation of health-based occupational exposure limits. Crit. Rev. Toxicol. 40:791–798, 2010.
- Scientific Committee on Occupational Exposure Limits (SCOEL): Methodology for the Derivation of Occupational Exposure Limits. Scientific Committee on Occupational Exposure Limits (SCOEL). Key Documentation (version 7 (2013); http://ec.europa.eu/social/BlobServlet?docId=4526&langId=en (accessed September 10, 2013).
- Monson, R.R.: Observations on the healthy worker effect. J. Occup. Med. 28:425–433 (1981).
- Li, C.-Y., and E.-C. Sung: A review of the healthy worker effect in occupational epidemiology. Occup. Med. 49(4):225–229 (1999).
- Schulte, P.A., C.Whittaker, and C.P. Curran: Considerations of using genetic and epigenetic Information in occupational health risk assessment and standard setting. J. Occup. Environ. Hyg. 12(S1):S69–S81.
- European Chemicals Agency (ECHA): Guidance on Information Requirements and Chemical Safety Assessment; Available from http://echa. europa. eu/) (2008).
- Naumann, B.D., K.C. Silverman, R. Dixit, E.C. Faria, and E.V. Sargent: Case studies of categorical data-derived adjustment factors. Human Ecol. Risk Assess. 7(1):61–105 (2001).
- Silverman, K.C., B.D. Naumann, D.J. Holder, et al.: Establishing data-derived adjustment factors from published pharmaceutical clinical trial data. Human Ecol. Risk Assess. 5(5):1059–1089 (1999).
- Weil, C.S.: Statistics vs. safety factors and scientific judgment in the evaluation of safety for man. Toxicol. Appl. Pharmacol. 21:454–463 (1972).
- Lewis, S.C.: Reducing uncertainty with adjustment factors. In: Improvements in quantitative noncancer risk assessment (A symposium overview). Fund. Appl. Toxicol. 20:1–14 (1993).
- Abdel-Rahman, M.S., and A.M. Kadry: Studies on the use of uncertainty factors in deriving RfDs. Human Ecol. Risk Assess. 1(5):614–624 (1995).
- Weil, C.S., and D.D. McCollister: Relationship between short- and long-term feeding studies in designing an effective toxicity test. Agr. Food Chem. 11(6):86–491 (1963).
- McNamara, B.P.: Concepts in health evaluation of commercial and industrial chemicals. In New Concepts in Safety Evaluation, M.A. Mehlman, R.E. Shapiro, and H. Blumenthal (eds.). Washington, D.C.:Hemisphere, 1976. pp. 61–140.
- Woutersen, R.A., H.P. Til, and V.J. Feron: Sub-acute versus sub-chronic oral toxicity study in rats: comparative study of 82 compounds. J. of Appl. Toxicol. 4(5):277–280 (1984).
- Lewis, S.C., J.R. Lynch, and A.I. Nikiforov: A new approach to deriving community exposure guidelines from “no-observed-adverse-effect levels.” Regul. Toxicol. Pharmacol. 11:314–330 (1990).
- Lewis, S.C., and C.S. Nessel: Extrapolating subchronic test results to estimate chronic no-observed-adverse-effect levels: Factors of 10 are larger than necessary. Toxicologist 14(1):401 (1994).
- Kadry, A.M., G.A Skowronski, and M.S. Abdel-Rahman: Evaluation of the use of uncertainty factors in deriving RfDs for some chlorinated compounds. J. Toxicol. Environ. Health 44:83–95 (1995).
- Dourson, M.L., L.A. Knauf, and J.C. Swartout: On Reference Dose (RfD) and its underlying toxicity data base. Toxicol. Industr. Health. 8(3):171–189 (1992).
- Interdepartmental Group on Health Risks from Chemicals (IGHRC): Guideline on Route-to-Route Toxicity Data when Assessing Health Risks of Chemicals. Published by the Institute of Environment and Health, 2006; http://ieh.cranfield.ac.uk/ighrc/pdf/cr%20reports/cr12%5b1%5d%5b1%5d.pdf (accessed September 29, 2015).
- Gaffney S.H., and D.J. Paustenbach: A proposed approach for setting occupational exposure limits for sensory irritants based on chemosensory models. Ann Occup Hyg. 51:345–356 (2007).
- Naumann, B.D., P.A. Weideman, R. Sarangapani, S. Hu, R. Dixit, and E.V. Sargent: Investigations of the use of bioavailability data to adjust occupational exposure limits for active pharmaceutical ingredients. Toxicol. Sci. 112(1):196–210 (2009).
- Calabrese, E.J. and C.E. Gilbert: Lack of total independence of uncertainty factors (UFs): Implications for the size of the total uncertainty factor. Regul. Toxicol. Pharmacol. 17:44–51 (1993).
- Swartout, J.C., P.S. Price, M.L. Dourson, H.L. Carlson-Lynch, and R.E. Keenan: A Probabilistic Framework for the Reference Dose (Probabilistic RfD). Risk Anal. 18:271–282 (1998).
- Gaylor, D.W., and R.L. Kodell: Percentiles of the product of uncertainty factors for establishing probabilistic reference doses. Risk Anal. 20:245–250 (2000).
- Pelekis, M., M.J. Nicolich, and J.S. Gauthier: Probabilistic framework for the estimation of the adult and child toxicokinetic intraspecies uncertainty factors. Risk Anal. 23:1239–1255 (2003).
- Hasegawa, R., M. Hirata-Koizumi, M.L. Dourson et al. : Proposal of new uncertainty factor application to derive tolerable daily intake. Regul. Toxicol. Pharmacol. 58:237–242 (2010).
- Waters, M., L. McKernan, A. Maier, M. Jayjock, V. Schaeffer, and L. Brosseau: Exposure estimation and interpretation of occupational risk: Enhanced information for the occupational risk manager. J. Occup. Environ. Hyg. 12(S1):S99–S111.
- National Institute for Occupational Safety and Health (NIOSH): Current Intelligence Bulletin 66: Derivation of Immediately Dangerous to Life or Health (IDLH) Values. Cincinnati, OH: US Department of Health and Human Services, 2013. Centers for Disease Control and Prevention, National Institute for Occupational Safety and Health, DHHS (NIOSH) Publication 2014–100.
- Vermeire, T., M. Pieters, M. Rennen, and P. Bos: Probabilistic Assessment Factors for Human Health Risk Assessment–A Practical Guide. RIVM report 601516 005/TNO report V3489. National Institute of Public Health and the Environment (RIVM) (in cooperation with TNO Nutrition and Food Research), Bilthoven, The Netherlands, 2001.
- Renwick, A.G.: Safety factors and establishment of acceptable daily intakes. Food Add. Contamin. 8(2):135–150 (1991).
- Renwick, A.G.: Data-derived safety factors for the evaluation of food additives and environmental contaminants. Food Add. Contam. 10(3):275–305 (1993).
- Renwick, A.G.: Subdivision of uncertainty factors to allow for toxicokinetics and toxicodynamics. Human Ecol. Risk Assess. 5(5):1035–1050 (1999).
- World Health Organization, International Programme on Chemical Safety (WHO/IPCS): Guidance Document for the Use of Data in Development of Chemical Specific Adjustment Factors (CSAFs) for Interspecies Differences and Human Variability in Dose/Concentration Response Assessment. Geneva: World Health Organization, International Programme on Chemical Safety, 2001; available at http://www.who.int/ipcs/methods/harmonization/areas/uncertainty/en/ (accessed August 9, 2014).
- Meek, B., E. Ohanian, A. Renwick, et al.: Guidelines for application of chemical-specific adjustment factors (CSAFs) in dose/concentration-response assessment. Comm. Toxicol. 7:575–590 (2001).
- Naumann, B.D., D.G. Dolan, and E.V. Sargent: Rationale for the chemical-specific adjustment factors used to derive an occupational exposure limit for timolol maleate.Hum. Ecol. Risk Assess. 10:99–111 (2004).
- Hattis, D., L. Erdreich, M. Ballew: Human variability in susceptibility to toxic chemicals–a preliminary analysis of pharmacokinetic data from normal volunteers. Risk Anal. 7(4):415–426 (1987).
- Sargent, E.V., B.D. Naumann, D.G. Dolan, E.C. Faria, and L. Schulman: The importance of human data in the establishment of occupational exposure limits. Hum. Ecol. Risk Assess. 8(4):805–822 (2002).
- Naumann, B.D., P.A. Weideman, R. Dixit, S.J. Grossman, C.F. Shen, and E.V. Sargent: Use of toxicokinetic and toxicodynamic data to reduce uncertainties when setting occupational exposure limits for pharmaceuticals. Hum. Ecol. Risk Assess. 3(4):555–565 (1997).