ABSTRACT
Road traffic noise can adversely impact the health of city residents, particularly when it occurs at night. The objective of this study was to evaluate nighttime traffic ambient noise in Toronto, Canada using measured and model-estimated noise levels. Road traffic noise was measured at 767 locations over 3 seasonal sampling campaigns between June 2012 and October 2013 to fully capture noise variability in Toronto. Temporal and campaign-specific spatial models, developed using the noise measurements, were used to build a final predictive surface. The surface was capable of estimating noise across the city over a 24-hr time frame. Measured and surface-estimated noise levels were compared with guidelines from the World Health Organization and the Province of Ontario to identify areas where noise may pose a health risk. Measured mean nighttime noise in Toronto exceeded World Health Organization (40 dBA) guidelines and mean daytime noise exceeded provincial (55 dBA) guidelines. The final predictive surface, incorporating spatial variables and daily cycles in noise levels, provides noise estimates geocoded for the entire study area. This tool could be used for epidemiological studies and to inform noise mitigation efforts. Based on surface-estimated noise levels during the quietest time of night (2 a.m.–2:30 a.m.), 100% of Toronto has nighttime noise exceeding 40 dBA (mean = 57 dBA, range = 49–110 dBA). A predictive surface was developed to estimate geocoded noise levels and facilitate further study of noise in Toronto. This tool can be used to assess road traffic noise, particularly at night, as an environmental health hazard.
Introduction
Noise is an important risk factor for chronic disease and an emerging global public health problem.[Citation1–3] In addition to directly causing hearing loss, environmental noise is associated with indirect effects such as sleep disturbance,[Citation4,Citation5] depression,[Citation6,Citation7] and cardiometabolic disease.[Citation8–11] Sleep disturbance is a widespread consequence of environmental noise in cities, as nighttime noise is ubiquitous in urban areas and a reduction in sleep quality and quantity occurs at relatively low noise levels.[Citation12,Citation13]
Traffic noise ranks among the top three health hazards contributing to the environmental burden of disease in western Europe, having an impact comparable to that of secondhand smoke.[Citation2] In particular, road traffic is a major source of environmental noise in North American and European cities.[Citation14–17] Noise due to road traffic is expected to increase with the continuing worldwide shift toward urbanization.
To effectively address the threat posed by environmental noise, an assessment of noise levels and their variability within urban areas is needed. Noise prediction models, which estimate noise levels based on relevant environmental characteristics, can characterize spatial and temporal patterns across a city and provide exposure estimates for its residents. Although road traffic noise has received substantial attention in Europe,[Citation2,Citation13,Citation14] it remains poorly characterized in many North American cities. In particular, a comprehensive tool for estimating nighttime noise from road traffic in the City of Toronto is needed to evaluate its impact on city residents.
There is a paucity of predictive models capable of estimating average nighttime noise levels throughout a city, with only one recent study reporting such a model.[Citation18] There also exists a need for a more robust approach to capturing road traffic noise variability in city-wide noise prediction models. Recent modeling efforts have been based on a single seasonal noise measurement campaign[Citation15,Citation16] or have involved the construction of a single model using data from multiple measurement campaigns,[Citation17,Citation19] limiting the capture of between-season noise variability. Another methodological issue that can restrict the capture of city noise variability is the sampling of noise levels at a small number of locations and in a manner not representative of the entire city area. Finally, the absence of a quality control step to ensure the exclusion of noise sources other than road traffic can hinder the effective capture of city road traffic noise variability.
To address these needs, a 2-part investigation of road traffic noise was carried out in Toronto. In the first part, described previously, traffic counts and nearby land use and road type variables were identified as the major factors influencing road traffic noise variability in the city.[Citation20] In the second part, described in this article, noise measurements from 3 seasonal campaigns were used to build a comprehensive predictive surface, allowing the exploration of noise levels across Toronto over a 24-hr time frame. The result is a novel research tool capable of providing spatially and temporally resolved estimates of road traffic noise to assist with evaluating the impact of noise in Toronto.
The objectives of this study were to: (1) measure road traffic noise in Toronto over space and time to optimally capture its variability; (2) construct a predictive surface capable of estimating noise levels across the city at any half-hour period of a 24-hr day; and (3) compare measured and surface-estimated noise levels with guidelines from the World Health Organization (WHO) and Province of Ontario (Ontario Ministry of the Environment and Climate Change) to identify potential noise concerns.
Methods
Study area
The City of Toronto is the largest city in Canada and the fourth largest city in North America, with a population of 2.7 million in 2016 (a 4.5% increase since 2011).[Citation21] Road traffic is the major source of environmental noise in Toronto.[Citation22] Road traffic congestion in Toronto has risen in the last decade[Citation23] and several of the highways crossing the city rank among the busiest roads in North America.[Citation24] Road traffic noise is expected to increase in the city as it and the surrounding region continue to experience rapid population growth.
Road traffic noise measurements
Short-term sampling
To capture spatial noise variability in Toronto, 751 sites were sampled across the city during 3 distinct campaigns: 241 sites between June and August of 2012 (cycle one), 313 sites between November 2012 and January 2013 (cycle two), and 197 sites between June and August 2013 (cycle three) (). Short-term sampling sites were selected to optimally assess the spatial variability of road traffic noise in Toronto. Given the dominant and pervasive contribution of road traffic to noise in cities and the study objective of capturing noise levels throughout Toronto, investigation was focused on road traffic instead of other intermittent and spatially limited urban noise sources (e.g., construction and entertainment). The sampling frame of the Canadian Community Health Survey, a population-based cross-sectional survey,[Citation25] was used to select 6-character postal code centroids across Toronto. Sites were also geocoded to determine their distance to major roads. This approach ensured the probability of selecting a site for sampling was proportional to its population density and proximity to busy roadways. To capture the spatial factors responsible for noise variability across the city, information was gathered on variables related to nearby land use and road types for each short-term sampling site. Further details concerning sample site selection and potential predictor variables are provided in the online supplemental material.
Figure 1. Locations in Toronto where noise measurements were collected. Short-term sampling sites for (A) cycle one, (B) cycle two, and (C) cycle three. (D) Long-term (7-day) sampling sites. For long-term sampling sites: Site 1 = Wentworth Avenue; Site 2 = Barbara Crescent; Site 3 = Dufferin Street; Site 4 = Bowood Avenue; Site 5 = Bloor Street; Site 6 = Queens Quay West; Site 7 = Lawton Boulevard (west side); Site 8 = Lawton Boulevard (east side); Site 9 = Chase Road.; Site 10 = Protea Gardens; Site 11 = Genella Square; Site 12 = Singer Court; Site 13 = Gala Lane; Site 14 = Tullis Drive; Site 15 = Lamb Avenue; Site 16 = Parkview Hill Crescent.
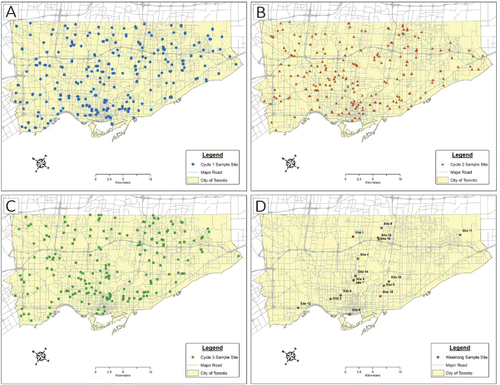
Noise was sampled between 10 a.m. and 5 p.m. during periods of good weather (i.e., the absence of high winds or heavy rain or snow). A-weighted sound pressure levels were measured using a 3M Quest SoundPro Type 1 sound level meter with a Brüel & Kjær 4936 microphone (Supplemental Figure S1). A 3M Quest QC-20 calibrator was used to calibrate the sound meter before and after each sampling period. The sound meter recorded noise levels at 1-sec intervals during each sampling period. In accordance with other recent city-based traffic noise studies, the duration of each sample was 30 min.[Citation15,Citation17,Citation19,Citation26] Each sample was averaged to obtain an equivalent continuous noise level (30-min Leq, dBA). Sites were randomly sampled to minimize the influence of time of day and day of the week on noise measurements. To minimize sound reflection, the sound meter microphone was placed 1.2–1.5 m above the ground and at least 2 m from any barriers.[Citation27] Any intermittent non-traffic noise (e.g., construction, garden work, sirens, etc.) during each sampling period was noted so it could be removed via a data cleaning step. The geographical coordinates of each sampling site were obtained using a Global Positioning System device.
Long-term sampling
To capture temporal noise variability in Toronto, 16 sites were sampled across the city between June 2012 and October 2013 (). Sites were selected based on the availability of consenting volunteers, with sound meters being placed in the backyard or on the balcony or rooftop of their place of residence. Within this convenience sampling approach, sites were chosen to cover as much of the city as possible, including locations representative of different residential and near-road scenarios. For each site, A-weighted sound pressure levels were measured continuously over a period of 7 days. The sound meter, microphone, and calibrator used for long-term sampling were the same as for short-term sampling. Samples were averaged across 30-min time periods to obtain equivalent continuous noise levels (30-min Leq, dBA). Sampling periods were chosen with a preference for periods of good weather and a covered location for sampling. The sound meter was placed inside an environmental enclosure, with the microphone positioned approximately 1.5 m above the ground, balcony floor, or rooftop. The distance from barriers varied considerably between sampling sites (ranging from 1 to ∼5 m).
Data quality
After noise measurements were collected, a thorough quality control step was followed to limit the capture of noise from sources other than road traffic. Intermittent non-traffic noise, identified by referring to site observations recorded during sampling, was removed using a custom cleaning algorithm (Supplemental Figure S2). If measured noise levels were inconsistent with recorded observations for any given site (e.g., an unexplained sharp decline in noise level at the end of the sampling period), the location was resampled. This approach was driven by the assumption that a 30-min sample at a site with no prevailing noise source would demonstrate relatively stable background levels vs. a site with an unexpected noise source that would potentially skew (or bias) measured data.
Data analysis
Temporal modeling
The temporal variability among the 16 long-term noise measurements was initially investigated using boxplots (Supplemental Figure S3) and a plot of noise variation over 7 days (). A daily cyclic pattern and a lack of appreciable day-to-day variation in noise levels were observed, so several linear mixed models were constructed to capture these patterns. Each model contained trigonometric functions (e.g., sine and cosine) at different frequencies to capture the daily cyclic pattern. Random effects with exponential correlation structure were also included to allow for temporal autocorrelation and variation between sampling sites. The model with the smallest Akaike information criterion was chosen as the final temporal model.
Figure 2. Measured and model-estimated temporal noise variation in Toronto during a 7-day week. Shaded regions correspond to nighttime (11 p.m.–7 a.m.). Horizontal lines correspond to the nighttime noise guideline (40 dBA, 8-hr Leq) recommended by the World Health Organization (WHO) for health protection and the daytime road traffic sound guideline (55 dBA, 16-hr Leq) for outdoor living areas recommended by the Ontario Ministry of the Environment and Climate Change (MOECC).
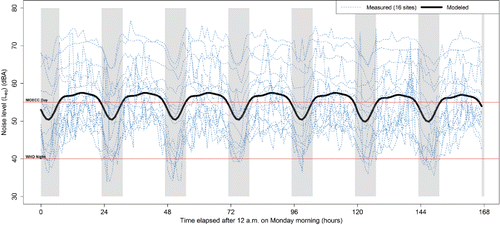
Spatial modeling
For each cycle, several land use regression models were constructed using different variables identified through exploratory analysis as potentially contributing to spatial noise variability. Variables explaining the majority of spatial noise variability were identified from the model with the highest coefficient of determination (R2). These variables were then incorporated into cycle-specific spatial models capable of predicting a continuous noise surface with fast discrete approximation. This strategy ensured the spatial patterns for each cycle were effectively captured. Although the land use regression modeling approach employed in this study did not explicitly account for noise propagation from individual sources, it nevertheless allowed for the inclusion of a wide array of spatial predictor variables (e.g., length of nearby roads and area of nearby land by use type) that indirectly influence noise emission and propagation.[Citation28]
The final cycle-specific predictive models consisted of measured noise levels as the dependent variable, spatial characteristics as the independent variables, and expected noise levels estimated via the final temporal model as offset (spatial predictor variables are listed in Supplemental Table S1). The models assumed the spatial surface had a Gaussian distribution with a Matern correlation structure, in keeping with similar studies.[Citation29] Bayesian inference was made using integrated nested Laplace approximation, permitting rapid and accurate approximation for a continuous Matern surface.[Citation30] All analyses were carried out using R (version 3.1.2).
Predictive surface
For each cycle-specific predictive surface, noise levels between 2 a.m. and 2:30 a.m. were estimated using the final temporal model at a spatial resolution of 5 m x 5 m over the entire city area. According to estimates obtained using the final temporal model (), nighttime noise levels were consistently lowest during this time period. The arithmetic mean across the three cycle-specific surfaces was then used to generate a final predictive surface, visualized as a raster-based surface map (). Combining modeled surfaces to enhance their utility is an approach commonly used in exposure assessment studies.[Citation31]
Figure 3. Nighttime (2 a.m.–2:30 a.m.) noise levels in Toronto. Noise levels (A) on a continuous scale and (B) categorized based on a 55-dBA cut-off, as estimated using the final predictive surface.
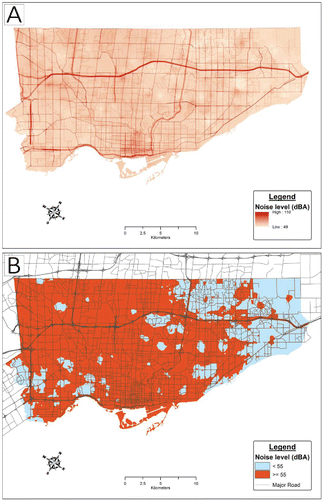
Taking the mean of the 3 cycle-specific surfaces ensured the optimal capture of daytime noise levels based on 30-min short-term samples. Cycles one and two covered different seasons (summer and winter), while cycles one and three collectively characterized a large number of distinct sites across the city during the same season in consecutive years. Each cycle-specific predictive surface was based on a set of predictor variables best suited to that particular cycle. See Supplemental Figure S4 for a flowchart summarizing the stages involved in creating and combining spatial and temporal models to produce the final predictive surface.
Results
Seven-day noise levels exhibited a cyclic pattern over each 24-hr day, with no appreciable difference between weekday and weekend levels (). Noise levels estimated via the final temporal model remained relatively constant during the daytime (7 a.m.–11 p.m., as defined by WHO[Citation13]), peaking in the late afternoon (∼3:30 p.m.). Noise levels declined through the evening and into the night, reaching their daily minimum value in the early morning (∼2:30 a.m.).
At long-term sampling sites, over 90% of measured nighttime (11 p.m.–7 a.m.) 30-min Leq values exceeded the 40 dBA (8-hr Leq) nighttime noise guideline recommended by WHO for the protection of exposed populations from adverse health effects ().[Citation13] In addition, over a third of measured nighttime 30-min Leq values were greater than 55 dBA, the level identified by WHO as being increasingly dangerous for public health due in part to the increased risk of cardiovascular disease.[Citation13] Using the final temporal model, nighttime noise levels were predicted to consistently exceed 40 dBA at all locations in Toronto ().
Table 1. Nighttime and daytime road traffic noise levels reported for North American and western European cities.
At long-term sampling sites, just over half of measured daytime 30-min Leq values exceeded the Province of Ontario guideline of 55 dBA (16-hr Leq) for road traffic noise in outdoor living areas ().[Citation32] Using the final temporal model, daytime noise levels were predicted to consistently exceed 55 dBA at all locations in Toronto (). A 55 dBA limit (16-hr Leq) is also recommended by WHO for protection from serious annoyance in outdoor living areas due to daytime noise exposure.[Citation27] Notably, 14% of measured 30-min Leq values were greater than 70 dBA. According to WHO, whole-day exposure to noise levels in excess of 70 dBA (24-hr Leq) can result in hearing impairment.[Citation27]
The predictive surface developed as part of this study was capable of providing high-resolution noise estimates across the City of Toronto for a particular time of day. The surface is a georeferencing tool by which noise estimates can be linked to other geocoded information (e.g., postal codes for residences, workplaces, and schools). Potential applications include population exposure assessments, investigations into the association between noise and air pollution, and regulatory and planning efforts to address the public health impact of noise.
The spatial models used to build each cycle-specific predictive surface contained variables for nearby land use and road types (Supplemental Table S1). Variables common to all 3 models were industrial area within 100 m, length of arterial roads within 25 m, length of collector roads within 25 m, and length of freeways within 100 m. The coefficients of these shared variables were similar for all 3 models.
To demonstrate the utility of the final predictive surface, it was employed to estimate city-wide noise levels between 2 a.m. and 2:30 a.m. (, ). Nighttime noise levels were at their lowest during this time period. Across the city, estimated 2 a.m.–2:30 a.m. noise levels were consistently greater than the 40 dBA WHO nighttime noise recommendation (i.e., 100% of Toronto residents were exposed to noise levels > 40 dBA). The minimum and maximum surface-estimated noise levels for this time period were appreciably higher than the corresponding measured values.
Discussion
In this study, measured and modeled nighttime and daytime noise levels in Toronto were found to frequently exceed recommended WHO and Province of Ontario guidelines. Based on surface-predicted noise levels for between 2 a.m. and 2:30 a.m., 100% of Toronto residents were exposed to nighttime noise levels outside their dwellings in excess of 40 dBA. This finding indicates that Toronto residents who are chronically exposed to outdoor nighttime noise (e.g., due to leaving windows open or where the build structure provides little attenuation of sound) are exposed to noise at levels capable of negatively impacting their health. The predictive surface represents an effective new tool for estimating city-wide noise levels, based on a large set of noise measurements collected with an eye toward the optimal capture of variability.
The average and range for the nighttime noise levels measured in Toronto were similar to values reported for other cities in North America and western Europe (). This underscores that nighttime noise appears to be a widespread public health issue for cities. While the average and range for nighttime noise levels measured in Montreal, the second-largest Canadian city, closely matched those for Toronto, the mean measured nighttime noise levels reported for Madrid and New York City were higher than the value in Toronto. These differences may reflect Canada-specific city characteristics or the relatively greater population sizes and densities of Madrid and New York City.
On the other hand, the average daytime noise levels measured in Toronto were appreciably lower than values reported for other cities in North American and western Europe (). As was the case for nighttime noise, the minimum measured daytime noise level in Toronto was particularly low compared to other studied cities. This difference cannot be attributed to green space coverage, as public parks and gardens in Toronto make up approximately 13% of the city's land area, a value that is in line with or appreciably lower than the other cities mentioned previously.[Citation33] Instead, the lower daytime noise levels observed for Toronto in this study may be a consequence of short-term measurements not being collected during most of the morning and afternoon rush hours, which are expected to be periods of relatively high daytime road traffic noise.
Only one other recent study reported average nighttime noise levels estimated using a city-wide predictive model. In agreement with the estimates for Toronto from this study, a noise model for London predicted 100% of residents were exposed to a nighttime noise level in excess of 40 dBA ().[Citation18] The minimum estimated noise level was similar for the 2 cities, but the maximum value for this study was considerably larger. For both cities, estimated city noise levels tended to be greater than measured noise levels, with the greatest discrepancies in the London model occurring at sites where traffic flows, and noise levels, were relatively low.[Citation18] A similar effect could explain why the maximum noise level estimated using the predictive surface was so much higher than the measured value. The highest surface-estimated noise levels occurred along major roads (), with lengths of collector roads, arterial roads, and freeways included as predictor variables in all 3 cycle-specific spatial models (Supplemental Table S1).
Given that nighttime and daytime noise levels in Toronto frequently exceeded recommended guidelines for the protection of human health, a response might be required to address this issue. This could include stricter noise regulations, various mitigation strategies (e.g., traffic management and noise-abating alterations to the built environment), and increasing public awareness of and access to information about environmental noise.[Citation27]
The predictive surface enables an improved understanding of road traffic noise across Toronto. It can be used to assign noise level estimates to populations (e.g., linking based on postal code or residential address), enabling research that characterizes the association between noise and adverse health effects as well as the identification of environmental inequities.[Citation34] In addition, the predictive surface can identify spatial and temporal patterns, highlight excessive noise hotspots, and permit the specific examination of noise sensitive areas (e.g., hospitals), informing city-wide efforts (e.g., policy development and urban planning) to reduce or prevent exposure to harmful levels of noise. Furthermore, given the frequent co-occurrence of noise and air pollution, noise level estimates derived from the predictive surface may have some utility as a proxy for air pollution exposure[Citation35] or could be used to address the role of noise as a potential confounding variable in air pollution studies. Finally, the predictive surface could potentially provide a baseline for background road traffic noise when considering city bylaws for other noise sources (e.g., amplified music) and serve as a reference point for evaluating future developments that may be expected to influence city noise levels (e.g., public transit improvements).
In agreement with the predictor variables included in the spatial models underpinning the final predictive surface, recent models have identified road type and land use variables as important predictors of road traffic noise levels.[Citation17,Citation36,Citation37] The lengths of nearby freeways, arterial roads, and collector roads, present as variables in each of the three cycle-specific spatial models, likely served as proxies for road traffic volume, a key determinant of road traffic noise levels. Nearby industrial area was also included in all three spatial models, indicating that although the noise measurements used to build the spatial models predominantly captured road traffic noise, a small proportion of the sampled noise was due to non-traffic sources such as industrial activities. Further, road traffic noise may be greater in industrial areas due to the heavy vehicle traffic associated with many industrial activities.
This study had a number of major strengths. A large number of noise measurements were collected, using a Type 1 sound meter, at various sites covering the entire area of Toronto and reflective of population distribution and proximity to roadways. Models were developed using real-time noise measurements instead of noise levels estimated using proxies such as traffic volume. The final predictive surface was constructed using individual spatial models for three distinct sampling campaigns, which meant assessing between-season noise variability while also ensuring intensive same-season capture of spatial noise variability. The inclusion of a thorough data cleaning procedure minimized the capture of intermittent noise from sources other than road traffic (e.g., lawn mowers). Finally, to reduce the misclassification of land use characteristics at sampling sites and improve understanding of their predictive contributions to the spatial models, nearby total area was used for land use predictor variables instead of simply assigning a classification to each site.
Several limitations should be noted. First, the short-term noise measurements used for spatial modeling were collected for a limited interval of time (a single 30-min period between 10 a.m. and 5 p.m.) and so may not sufficiently capture noise levels throughout the day. To address this issue, noise measurements were collected over different seasons but also over 2 years during the same season, to maximize capture of spatial variability. In addition, a 30-min short-term sampling period was chosen for this study, which was considerably longer than sampling periods used in similar recent studies of urban noise.[Citation15,Citation17,Citation19,Citation26] It should be noted that the short-term noise measurements used to build the spatial component of the predictive surface were not collected during most of the morning and afternoon rush hours, which are expected to be periods of relatively high road traffic noise, nor were they collected at night. An additional campaign of short-term sampling performed during these excluded periods may improve the spatial models underpinning the predictive surface.
Second, a relatively small number of long-term noise measurements were used for temporal modeling. Sound meters needed to be placed in secure locations where they could remain unattended for the entire 7-day sampling period. This required volunteers who could guarantee that the meters would be protected from weather and vandalism. This may limit the extent to which the temporal noise variations observed in this study can be generalized for the entire city, which could impact the validity of the final predictive surface. The final temporal model could be improved by incorporating additional long-term measurements sampled according to a probabilistic methodology, with sound meters potentially being installed on utility poles to ensure their relative security.
Finally, the Province of Ontario recommended guideline referred to in this study was explicitly for outdoor living areas, which include locations not captured by the short-term noise measurements collected in this study. The provincial guideline also specified noise levels be measured 3 m from the building façade and in the middle of the outdoor living area, requirements that were not consistently met in this study due to feasibility constraints. These issues could be resolved by expanding the scope of sampling to include all outdoor living areas and ensuring all sites are sampled in accordance with the guideline.
Looking forward, there are several key applications for the noise prediction tool. First, the construction of predictive surfaces for other Ontario cities would permit a more comprehensive evaluation of urban noise in the province. Second, Toronto-based epidemiological studies could be performed to assess links between noise and adverse health outcomes. Finally, it would be valuable to investigate other potential factors explaining noise variation across Toronto (e.g., traffic composition, forested areas, and meteorological conditions) to augment the predictive surface.
Conclusions
Using an extensive set of noise measurements, a robust noise prediction surface was developed to characterize road traffic noise levels throughout the City of Toronto. This tool provides geocoded noise estimates with potential utility for epidemiological investigations and efforts to mitigate the negative health effects of noise. Considering that measured and surface-estimated nighttime and daytime noise levels across the city frequently exceeded WHO and Province of Ontario recommended guidelines, this study demonstrates that road traffic noise in Toronto is an important public health issue and warrants further investigation.
Supplemental Material
Download MS Word (735.5 KB)Acknowledgments
The authors wish to thank the following individuals who assisted with data collection and preliminary analysis: Chad Shew, Fei Zuo, Fuan Liu, Howard Wu, and Rebecca Hou.
Additional information
Funding
References
- Hammer, M., T. Swinburn, and R. Neitzel: Environmental noise pollution in the United States: Developing an effective public health response. Environ. Health Perspect. 122(2):115–119 (2014).
- Hänninen, O., A. Knol, M. Jantunen, et al.: Environmental burden of disease in Europe: Assessing nine risk factors in six countries. Environ. Health Perspect. 122(5):439–446 (2014).
- World Health Organization: Burden of Disease from Environmental Noise—Quantification of Healthy Life Years Lost in Europe. Bonn, Germany: World Health Organization Regional Office for Europe, 2011.
- Miedema, H.M., and H. Vos: Associations between self-reported sleep disturbance and environmental noise based on reanalyses of pooled data from 24 studies. Behav. Sleep Med. 5(1):1–20 (2007).
- Perron, S., C. Plante, M.S. Ragettli, D.J. Kaiser, S. Goudreau, and A. Smargiassi: Sleep disturbance from road traffic, railways, airplanes and from total environmental noise levels in Montreal. Int. J. Environ. Res. Public Health 13(8):809 (2016).
- Orban, E., K. McDonald, R. Sutcliffe, et al.: Residential road traffic noise and high depressive symptoms after five years of follow-up: Results from the Heinz Nixdorf Recall Study. Environ. Health Perspect. 124(5):578–585 (2016).
- Seidler, A., J. Hegewald, A.L. Seidler, et al.: Association between aircraft, road and railway traffic noise and depression in a large case-control study based on secondary data. Environ. Res. 152:263–271 (2017).
- Babisch, W.: Updated exposure-response relationship between road traffic noise and coronary heart diseases: A meta-analysis. Noise Health 16(68):1–9 (2014).
- Christensen, J.S., O. Raaschou-Nielsen, A. Tjønneland, et al.: Road traffic and railway noise exposures and adiposity in adults: A cross-sectional analysis of the Danish Diet, Cancer, and Health cohort. Environ. Health Perspect. 124(3):329–335 (2016).
- Halonen, J.I., A.L. Hansell, J. Gulliver, et al.: Road traffic noise is associated with increased cardiovascular morbidity and mortality and all-cause mortality in London. Eur. Heart J. 36(39):2653–2661 (2015).
- Sørensen M., Z.J. Andersen, R.B. Nordsborg, et al.: Long-term exposure to road traffic noise and incident diabetes: A cohort study. Environ. Health Perspect. 121(2):217–222 (2013).
- Halperin, D.: Environmental noise and sleep disturbances: A threat to health? Sleep Sci. 7(4):209–212 (2014).
- World Health Organization: Night Noise Guidelines for Europe. Copenhagen, Denmark: World Health Organization Regional Office for Europe, 2009.
- European Environment Agency: Noise in Europe 2014. Copenhagen, Denmark: European Environment Agency, 2014.
- Lee, E.Y., M. Jerrett, Z. Ross, P.F. Coogan, and E.Y. Seto: Assessment of traffic-related noise in three cities in the United States. Environ. Res. 132:182–189 (2014).
- McAlexander T.P., R.R. Gershon, and R.L. Neitzel: Street-level noise in an urban setting: assessment and contribution to personal exposure. Environ. Health 14:18 (2015).
- Ragettli M.S., S. Goudreau, C. Plante, et al.: Statistical modeling of the spatial variability of environmental noise levels in Montreal, Canada, using noise measurements and land use characteristics. J. Expo. Sci. Environ. Epidemiol. 26(6):597–605 (2016).
- Gulliver, J., D. Morley, D. Vienneau, et al.: Development of an open-source road traffic noise model for exposure assessment. Environ. Model. Softw. 74:183–193 (2015).
- Aguilera, I., M. Foraster, X. Basagaña, et al.: Application of land use regression modelling to assess the spatial distribution of road traffic noise in three European cities. J. Expo. Sci. Environ. Epidemiol. 25(1):97–105 (2015).
- Zuo, F., Y. Li, S. Johnson, et al.: Temporal and spatial variability of traffic-related noise in the City of Toronto, Canada. Sci. Total Environ. 472:1100–1107 (2014).
- Statistics Canada: “Population and Dwelling Count Highlight Tables, 2016 Census.” Available at http://www12.statcan.gc.ca/census-recensement/2016/dp-pd/hlt-fst/pd-pl/index-eng.cfm ( accessed February 9, 2017).
- Toronto Public Health: How Loud Is Too Loud? Health Impacts of Environmental Noise in Toronto. Toronto, Canada: Toronto Public Health, 2017.
- McMaster Institute for Transport and Logistics: Congestion Trends in the City of Toronto (2011–2014). Hamilton, Canada: McMaster University, 2015.
- Canadian Automobile Association: Grinding to a Halt: Evaluating Canada's Worst Bottlenecks. Ottawa, Canada: Canadian Automobile Association, 2017.
- Statistics Canada: “Canadian Community Health Survey (CCHS), Cycle 4.1.” Available at http://www23.statcan.gc.ca/imdb/p2SV.pl?Function = getSurvey&Id = 29539 ( accessed January 30, 2017).
- Morelli, X., M. Foraster, I. Aguilera, et al.: Short-term associations between traffic-related noise, particle number and traffic flow in three European cities. Atmos. Environ. 103:25–33 (2015).
- World Health Organization: Guidelines for Community Noise. Geneva, Switzerland: World Health Organization, 1999.
- Mennitt, D., K. Sherrill, and K. Fristrup: A geospatial model of ambient sound pressure levels in the contiguous United States. J. Acoust. Soc. Am. 135(5):2746–2764 (2014).
- Lee, D., and G. Shaddick: Spatial modeling of air pollution in studies of its short‐term health effects. Biometrics 66(4):1238–1246 (2010).
- Rue, H., S. Martino, and N. Chopin: Approximate Bayesian inference for latent Gaussian models by using integrated nested Laplace approximations. J. Royal Stat. Soc. B 71(2):319–392 (2009).
- Parent, M.E., M.S. Goldberg, D.L. Crouse, et al.: Traffic-related air pollution and prostate cancer risk: A case-control study in Montreal, Canada. Occup. Environ. Med. 70(7):511–518 (2013).
- Ontario Ministry of the Environment and Climate Change: “Environmental Noise Guideline (NPC-300).” Available at https://www.ontario.ca/page/environmental-noise-guideline-stationary-and-transportation-sources-approval-and-planning ( accessed January 16, 2017).
- World Cities Culture Forum: “Percent of Public Green Space (Parks and Gardens).” Available at http://www.worldcitiescultureforum.com/data/of-public-green-space-parks-and-gardens ( accessed March 26, 2017).
- Carrier, M., P. Apparicio, and A.M. Séguin: Road traffic noise in Montreal and environmental equity: What is the situation for the most vulnerable population groups? J. Transp. Geogr. 51:1–8 (2016).
- Dekoninck L., D. Botteldooren, L.I. Panis, et al.: Applicability of a noise-based model to estimate in-traffic exposure to black carbon and particle number concentrations in different cultures. Environ. Int. 74:89–98 (2015).
- Ryu, H., I.K. Park, B.S. Chun, and S.I. Chang: Spatial statistical analysis of the effects of urban form indicators on road-traffic noise exposure of a city in South Korea. Appl. Acoust. 115:93–100 (2017).
- Wang, V., E. Lo, C. Liang, K. Chao, B. Bao, and T. Chang: Temporal and spatial variations in road traffic noise for different frequency components in metropolitan Taichung, Taiwan. Environ. Pollut. 219:174–181 (2016).
- Linares C., D. Culqui, R. Carmona, C. Ortiz, and J. Díaz: Short-term association between environmental factors and hospital admissions due to dementia in Madrid. Environ. Res. 152:214–220 (2017).
- Kheirbek, I., K. Ito, R. Neitzel, et al.: Spatial variation in environmental noise and air pollution in New York City. J. Urban Health 91(3):415–431 (2014).