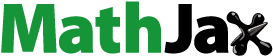
ABSTRACT
RNA-binding protein with multiple splicing (RBPMS) is critical for axon guidance, smooth muscle plasticity, and regulation of cancer cell proliferation and migration. Recently, different states of the RNA-recognition motif (RRM) of RBPMS, one in its free form and another in complex with CAC-containing RNA, were determined by X-ray crystallography. In this article, the free RRM domain, its wild type complex and 2 mutant complex systems are studied by molecular dynamics (MD) simulations. Through comparison of free RRM domain and complex systems, it's found that the RNA binding facilitates stabilizing the RNA-binding interface of RRM domain, especially the C-terminal loop. Although both R38Q and T103A/K104A mutations reduce the binding affinity of RRM domain and RNA, the underlining mechanisms are different. Principal component analysis (PCA) and Molecular mechanics Poisson-Boltzmann surface area (MM/PBSA) methods were used to explore the dynamical and recognition mechanisms of RRM domain and RNA. R38Q mutation is positioned on the homodimerization interface and mainly induces the large fluctuations of RRM domains. This mutation does not directly act on the RNA-binding interface, but some interfacial hydrogen bonds are weakened. In contrast, T103A/K104A mutations are located on the RNA-binding interface of RRM domain. These mutations obviously break most of high occupancy hydrogen bonds in the RNA-binding interface. Meanwhile, the key interfacial residues lose their favorable energy contributions upon RNA binding. The ranking of calculated binding energies in 3 complex systems is well consistent with that of experimental binding affinities. These results will be helpful in understanding the RNA recognition mechanisms of RRM domain.
Introduction
Protein-RNA interactions play essential roles in numerous cellular processes, such as gene expression and its regulation.Citation1 In mammalian cells, more than 1,000 diverse proteins interact with RNA.Citation2 The interactions of proteins with RNA have been generally explained using different types of motifs such as RNA recognition motif, double stranded RNA binding motif, Arginine rich motif, GXXG motif, tetra loops in RNA and so on.Citation3 The RNA-recognition motif (RRM) is the most abundant RNA-binding domain in higher vertebrates, which is present in about 0.5%–1.0% of human genes.Citation4
The first RRM structure was elucidated in 1990 by Nagai et al.Citation5 Over the past 20 years, X-ray crystallographic and NMR experiments promoted our understanding for the RNA-recognition motif and Protein-RNA interactions.Citation6,7 Generally, an RRM consists of 80-90 amino acids with a typical β1-α1-β2-β3-α2-β4 topology, where 4 antiparallel β-strands are packed against 2 α-helices.Citation8 Recently, Farazi et al. studied the transcriptome-wide mRNA targets of the RRM domain of RNA-binding protein with multiple splicing (RBPMS) systematically, and identified RNA targets composed of tandem CAC trinucleotide motifs separated by variable spacer segments.Citation9 Teplova et al. determined the X-ray crystallographic structures of the RBPMS RRM domain in its free form and in complex with CAC-containing RNA ().Citation10 The RRM domain of RBPMS adopts the classical RRM fold. The homodimerization interface of RRM domain is mediated by residues of the first α-helix and adjacent loop region, as well as the loop segment between the second α-helix and the fourth β-strand. The UCAC segment of the RNA is positioned over the 4-stranded β-sheet surface in the RBPMS RRM-RNA complex. These experimental studies provided essential structural bases for understanding the recognition mechanism of RRM domain and RNA. However, several questions still remain unclear. What specific interactions are formed at the interface between RRM domain and RNA? Why and how do the different mutants reduce the binding affinities? What is the relationship between the conformational changes and the interfacial interactions?
Figure 1. Cartoon representation of the free RRM domain (A) and the RNA-binding complex (B) systems. Two RRM domain (chain A and chain B) are colored cyan and blue, respectively. Chain P and chain Q of RNA are colored yellow and orange, respectively. The secondary structure elements are labeled on the free RRM domain. The important RNA-binding residues are highlighted in stick model, and the nucleotides are labeled on the RNA-binding complex.
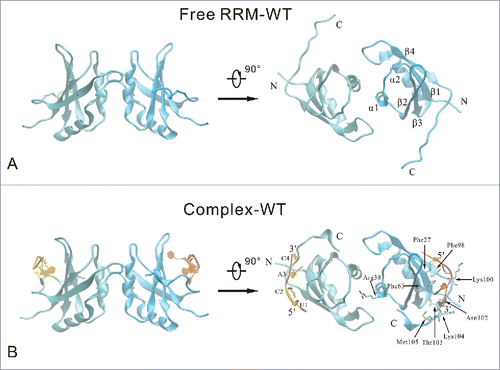
In order to probe the above issues, we applied molecular simulation methods to analyze the structural characters of the RRM domain in free and in complex states. Modern computer simulation methods have become important tools in exploring the recognition mechanism of protein and RNA.Citation11-13 Using NMR measurements, combined with Molecular Dynamics (MD) simulations, Scheiba et al. showed that the C-terminal RNA binding motif of HuR is a multi-functional domain which leads to HuR oligomerization and binds to U-rich RNA targets.Citation14 MD simulations were also employed to investigate the dynamics of the P22 N-peptide-boxB RNA complex and to elucidate the energetic contributions to binding.Citation15 Kormos et al. found that combination of MD simulation and corresponding atomic fluctuation analysis is an effective method for understanding and predicting cooperativity at the molecular level in protein-RNA binding.Citation16 Casu et al. employed heteronuclear, multidimensional NMR and CD spectroscopy as well as MD simulations to investigate the conformational changes of the Rev ARM associated with RNA binding.Citation17 Coarse-grained methods were also carried out to study the direction of functional movements and explain the process of structural rearrangement of Ffh protein and the SRP RNA.Citation18
Here, we used all-atom MD simulation to investigate the free RRM domain, its wild type complex and 2 mutant complex systems. The dynamical properties of RRM domain and RNA were explored by using the dynamical cross-correlated map (DCCM) analysis, principal component analysis (PCA) and free energy landscape (FEL) methods. We analyzed the crucial interfacial interactions of RRM domain and RNA, and explained the loss of binding affinities of 2 mutants relative to the wild type. Finally, we further discussed the possible correlations between the conformational changes of complex structures and the interfacial interactions.
Results and discussion
Comparative analysis of 4 MD trajectories
Four 120 ns MD simulations were carried out for the free RRM domain, its wild type complex and 2 mutant complex systems (R38Q and T103A/K104A). The R38Q mutation is located on the homodimerization interface, whereas the T103A/K104A mutations on the binding interface between RRM domain and RNA. For convenience, the 4 systems were designated as free RRM-WT, complex-WT, complex-R38Q and complex-T103A/K104A, respectively. As shown in , the RMSD values of 4 systems are stable after 20 ns, so the MD trajectories of the last 100 ns are chosen for further analyses. displays the distributional probability of RMSD in 4 systems. The mean values of RMSD are 4.06, 2.72, 4.23 and 3.55 Å for the free RRM-WT, complex-WT, complex-R38Q and complex-T103A/K104A systems, respectively. The complex-R38Q and complex-T103A/K104A systems were mutated from complex-WT system. Therefore, these mutations may cause the simulated complex structures move away from the starting structure. Similarly, the free RRM-WT was modeled from L81M mutant, also showing a relatively higher RMSD value. The previous experiments showed that R38Q mutation reduced binding affinity by 3- to 4-fold, whereas Ala mutations of both Thr103 and Lys104 resulted in undetectable binding.Citation10 Nevertheless, the mean value of RMSD in complex-T103A/K104A system is lower than that in complex-R38Q system. Therefore, it's necessary to analyze the specific flexible regions of these mutant systems.
Figure 2. Comparison of RMSD for free RRM-WT (red), complex-WT (purple), complex-R38Q (blue) and complex-T103A/K104A (yellow) systems. (A) The RMSD values of non-hydrogen atoms versus simulation time. (B) The probability distribution of RMSD calculated from the equilibrium trajectories.
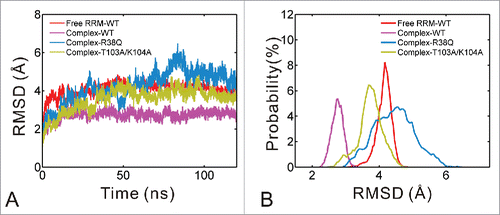
The root mean square fluctuation (RMSF) is usually used to assess the flexibility of different regions. Figs. S1A and S1B show the RMSF values of RRM domain and RNA calculated from the equilibrium trajectories of 4 systems, respectively. The two RRM domains of RBPMS form a symmetrical dimer. The two RNA molecules are bound to RRM domain in the same orientations. The simulation data are similar for these 2 symmetrical groups. Without loss of generality, we choose chain B of RRM domain and chain Q of RNA for analyses. In order to display the hierarchies of flexible regions intuitively, the residues and nucleotides in Fig. S2 were colored according to their RMSF values. As shown in Fig. S1A, most regions of the RRM domain in complex-R38Q system possess higher RMSF values than those in free RRM-WT, complex-WT and complex-T103A/K104A systems. Especially, the regions with higher RMSF values are around residues Arg38, Ala80 and the C-terminal loop. These regions are mainly located at the homodimerization interface (Fig. S2C). It indicates that R38Q mutation is unfavorable to the dimerization of RRM domain. Interestingly, both free RRM-WT and complex-T103A/K104A show higher RMSF values around Thr103. It implies that without RNA binding or mutations of the RNA-binding residues will destabilize the interfacial residues. Thus, RNA binding will facilitate the stability of the residues at the RNA-binding interface. As shown in Fig. S1B, the RMSF values of RNA in 2 mutant complex systems are both higher than those in complex-WT system. The profile of RMSF curve of complex-R38Q system is still similar to that of complex-WT system. The nucleotide U1 has higher RMSF value, but the subsequent CAC trinucleotides are relatively stable. Nevertheless, the complex-T103A/K104A system shows higher RMSF values at the last 2 nucleotides A3 and C4. These results imply that the R38Q and T103A/K104A mutations may change the dynamical properties of the RRM domain and the RNA, respectively.
Dynamical cross-correlated map analysis of RRM domain and RNA
The dynamical properties of RRM domain and RNA can be detected by dynamical cross-correlated map. According to Equation Equation1(1)
(1) , the correlated fluctuations of RRM domain and RNA in complex-WT, complex-R38Q and complex-T103A/K104A systems are graphically presented in . Positive correlations are mapped in the upper left triangle while negative correlations are mapped in the lower right triangle. Deeper color indicates more correlated (or anticorrelated).
Figure 3. Dynamical cross-correlated map of complex-WT (A), complex-R38Q (B) and complex-T103A/K104A (C) systems. Positive correlations and negative correlations are mapped in the upper left triangle and lower right triangle, respectively. Deeper color indicates more correlated (or anti-correlated). Both x and y axes of the map are residue indices in RRM domain (chain B) and nucleotide indices in chain Q of RNA. In curve of complex-WT, RNA shows positive correlations with the antiparallel β-sheet at residues Phe27, Phe65 and Phe98-Met105.
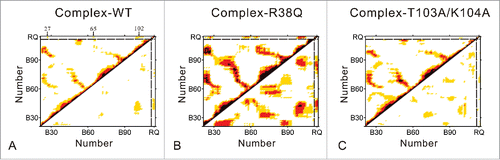
In the map of complex-WT system (), some positive correlations were observed between the antiparallel β-sheet of RRM domain. RNA shows positive correlations with the antiparallel β-sheet in RRM domain, especially residues Phe27, Phe65 and Phe98-Met105. These residues are found by the previous experiments to form interfacial hydrogen bonds and intermolecular stacking interactions for RNA recognition.Citation10 Meanwhile, RNA exhibits negative correlations with the 2 α-helices. Nevertheless, as shown in , the positive and negative correlations are increased remarkably in the map of complex-R38Q system. This result is mainly caused by the large movements of complex-R38Q system. Fortunately, RNA also remains its positive and negative correlations, which are similar to those in complex-WT system. For complex-T103A/K104A system (), the internal correlated values of RRM domain are between those of complex-WT and complex-R38Q systems. It implies that the correlated motions of RRM domains in complex-T103A/K104A system are more remarkable than those in complex-WT system but weaker than those in complex-R38Q system. However, the positive and negative correlations between RRM domain and RNA are decreased in the complex-T103A/K104A system, which indicates that the correlated motions between RRM domain and RNA are reduced in this mutant system. The decreasing correlated motions imply that T103A/K104A mutations may weaken the interactions between RRM domain and RNA.
Motion mode analysis of 4 systems
To further explore the direction of dynamical motion, both PCA and FEL were carried out for the 4 MD trajectories. shows the average structures and the first motion modes (PC1) of 4 systems. As shown in , the homodimerization interface is stable in the free RRM-WT system, but the C-terminal loop has remarkable motions. The C-terminal loop in the free RRM-WT system even shows a moving trend to the conformation in the complex-WT system. In the complex-WT system (), the RRM domain is relatively stable, including the C-terminal loop. This comparison implies that RNA binding stabilize the C-terminal loop conformation in RRM domain. Nevertheless, the nucleotide U1 in RNA has remarkable movements in the complex-WT system, which is consistent with the above RMSF analysis. As shown in , the 2 RRM domains exhibit large opposite motions in complex-R38Q system. It implies that R38Q mutation may induce the separation of the RBPMS homodimer. However, the average structure of RNA in complex-R38Q system is similar to that in complex-WT system. In contrast, as shown in , the T103A/K104A mutations obviously adopt the distinct average structure in comparison to complex-WT system and change the moving trend of RNA.
Figure 4. First slowest motion modes of free RRM-WT (A), complex-WT (B), complex-R38Q (C) and complex-T103A/K104A (D) systems. The average structure is depicted with tube model. RRM domain and RNA are colored blue and orange, respectively. The motion modes are shown as cone model and colored red. The length of cone is positively correlated with the motion magnitude, and the orientation of cone indicates the motion direction.
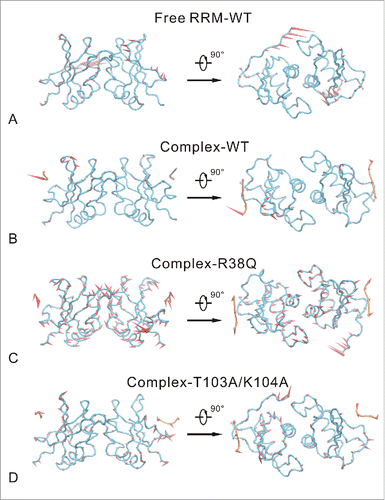
Fig. S3 shows the first 50 eigenvalues obtained from the diagonalization of the covariance matrix. The eigenvalues of 4 systems quickly decrease and converge to zero with the increasing of eigenvector index. The first 2 principal components (PC) capture 50.9%, 20.9%, 59.6% and 39.4% of the system's variance for free RRM-WT, complex-WT, complex-R38Q and complex-T103A/K104A systems, respectively. The complex-WT system does not have remarkable motions on the backbone. Its motion are mainly the localized random motions, so the fraction of first 2 PCs are only around 20%. In contrast, the free RRM-WT, complex-R38Q and complex-T103A/K104A systems possess specific motion modes (see ), so the first 2 PCs capture higher fraction of the system's variance.
As shown in , the free energy contour maps are constructed for 4 systems at 298 K with deeper color indicating the lower energy. According to the above stability analyses, it is found that the free RRM-WT, complex-R38Q and complex-T103A/K104A systems could adopt conformational states wider than those for complex-WT system. Therefore, as show in , the PC1 and PC2 motion modes of these 3 systems span larger ranges than those of the complex-WT system. The free energy landscapes of the 4 systems generally can be divided to 2 distinctive local basins. The two minima correspond to the conformations from the former and latter 50 ns of equilibrium trajectories, respectively. Fig. S4 shows the representative structures in the 2 local energy basins compared with the crystal structure. For free RRM-WT (Fig. S4A), the C-terminal loop in the representative structures has remarkable movements and shows similar structure to that in the complex-WT system. As shown in Fig. S4B, the nucleotide U1 in RNA exhibits the movement away from the RRM domain in the 2 representative structures of complex-WT. In complex-R38Q system (Fig. S4C), 2 RRM domains move far away from each other, but the RNA conformations are also similar to those in the complex-WT system. Different to complex-R38Q system, the RNAs in the representative structures of complex-T103A/K104A system undergo large conformational changes at nucleotides A3 and C4 (Fig. S4D).
Figure 5. Free energy contour map vs. the principal components PC1 and PC2 for free RRM-WT (A), complex-WT (B), complex-R38Q (C) and complex-T103A/K104A (D) systems. Deeper color indicates lower energy. The distinctive local basins are denoted as B1 and B2, respectively. B1 and B2 basins correspond to the conformations from the former and latter 50 ns of equilibrium trajectories, respectively.
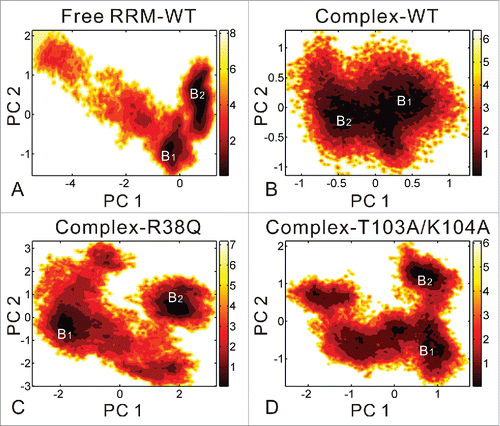
The conformational changes of RRM domain and RNA are related to the rearrangement of interactions, so we further analyzed the detailed interactions in the complex systems below.
Comparison of interactions in 3 complex systems
The binding energies of the 3 complex systems were calculated by g_mmpbsa and shown in . The ranking of calculated binding energies is well consistent with that of experiment binding affinities.Citation10 The complex-WT system has the lowest binding energy, whereas the complex-T103A/K104A system possesses the highest binding energy. The van der Waals energy, the polar and nonpolar solvation energies of complex-R38Q system are similar to those of complex-WT system, but its electrostatic energy is slightly higher than that of complex-WT system. The loss of electrostatic energy may be induced by the fluctuation of RRM domain. Nevertheless, in the complex-T103A/K104A system, all of the van der Waals energy, the electrostatic energy, the polar and nonpolar solvation energies are higher than those of complex-WT system. It implies that T103A/K104A mutations may break most of the interactions in the interface between RRM domain and RNA. MM-PBSA method is widely applied to calculate absolute binding affinities.Citation19,20 However, MM-PBSA results may be influenced by system-dependent properties, such as the features of the binding site, the extent of protein and ligand conformational relaxation upon association, and the protein and ligand charge distribution. The current implementation of the MM-PBSA method within g_mmpbsa does not include the calculation of entropic terms, and therefore in principle it is unable to give the absolute binding energy. In this work, the conformational entropy may be indirectly reflected by the probability distribution of RMSD (see Fig. S1B). The conformational flexibility of T103A/K104A system is slightly higher than that of complex-WT system, and the R38Q system possesses the highest conformational flexibility in the 4 systems. The mutations of R38Q and T103A/K104A systems will cause the increase of conformational entropy, which could be favorable for binding. Nevertheless, the cross-correlated map analysis implies that the increased conformational flexibility of T103A/K104A system is not related to protein-RNA interactions. Therefore, these increased conformational flexibility could not make a favorable contribution to RNA binding.
Table 1. Comparison of binding energy components between RRM domain and RNA in 3 complex systems.
In order to investigate the detailed interactions between RRM domain and RNA, we used the energy decomposition strategy to analyze the contribution of each residue or nucleotide (). In three complex systems, 11 residues in RRM domain contribute unfavorable energies for RNA binding. These residues are mainly located on the loop structure or the regions far away to the RNA binding interface. In complex-WT system, residues Arg24, Phe27, Lys36, Arg38, Arg45, Lys48, Lys56, Lys60, Phe65, Arg71, Lys78, Arg85, Arg95, Ala99, Lys100, Thr103, Lys104, Met105, Lys107 and Lys109 make favorable contributions for RNA binding. These interfacial residues are also reported by the previous experiments,Citation10 which may form hydrogen bonds or intermolecular stacking interactions with RNA. As shown in , the favorable contributions of residues Arg38 and Met105 are lost in complex-R38Q system. Similarly, the favorable contributions of residues Thr103-Met105 are disappeared in complex-T103A/K104A system (). Moreover, T103A/K104A mutations decrease the favorable contribution of residue Phe65, which is identified by previous experiments to make the crucial intermolecular stacking interactions with A3.Citation10 In 3 complex systems, all of 4 nucleotides make favorable energy contributions in the energy decomposition of RNA (). The three nucleotides CAC contribute most of the binding energies, so these 3 nucleotides are more stable than U1 in the representative structures shown in Fig. S4. Nevertheless, the contributions of trinucleotides CAC are decreased in the 2 mutant systems, especially the contributions of nucleotides A3 and C4 in complex-T103A/K104A system.
Figure 6. Binding energy decomposition of complex-WT (A-B), complex-R38Q (C-D) and complex-T103A/K104A (E-F) systems. Energetic contributions of residues in RRM domain are shown in the left figures (A), (C) and (E). Energetic contributions of nucleotides in RNA are shown in the right figures (B), (D) and (F). Energies are given as kilojoules per mole. Error bars represent the standard deviations of the energies.
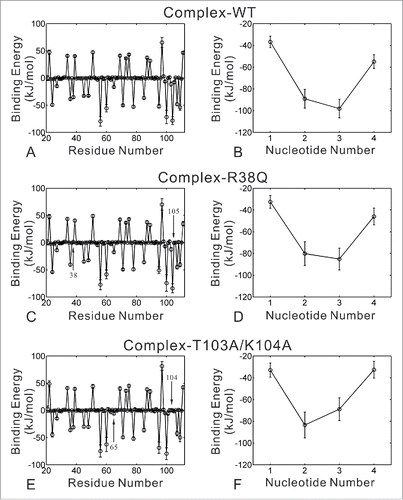
To understand the detailed interactions, we further analyzed the hydrogen bonds between RRM domain and RNA. The hydrogen bonds formed by RRM domain and RNA with occupancy over 10% for complex-WT, complex-R38Q and complex-T103A/K104A systems are shown in . In complex-WT system, high occupancy hydrogen bonds mainly formed between RRM domain and trinucleotides CAC. The nucleotide U1 does not make any high occupancy hydrogen bonds with RRM domain. As shown in , these high occupancy hydrogen bonds are labeled in 2 representative structures of the complex-WT system. Eight hydrogen bonds are maintained in the 2 representative structures. Only nucleotide C2 alternates the hydrogen bonds with Lys100-NZ by atoms O1P and O2P (). These high occupancy hydrogen bonds may contribute to stabilize the orientations of intermolecular stacking interactions between RNA and RRM domain (residues Phe27 and Phe65). Compared to complex-WT system, most of hydrogen bonds (8 out of 10) are remained in complex-R38Q system, except 2 hydrogen bonds Lys100-NZ:Cyt2-O2P and Met105-N:Cyt4-O2 (see the hydrogen bonds highlighted in bold). Therefore, consistent to the energy decomposition, the favorable contribution of residue Met105 is lost in complex-R38Q system. Notably, only one native hydrogen bond is remained in complex-T103A/K104A system between C2 and Phe98, and the other 8 hydrogen bonds are non-native. This result implies that T103A/K104A mutations break most of the high occupancy hydrogen bonds in the interface between RRM domain and RNA, which could results in complete loss in binding affinity.
Figure 7. The crucial interactions of RRM domain and RNA in 2 representative structures of the complex-WT system. The interfacial hydrogen bonds and intermolecular stacking interactions formed by nucleotides C4, A3 and C2 are shown in the first representative structure (A-C) and the second representative structure (D-F), respectively. RRM domain and RNA are depicted with cartoon (blue) and ribbon (orange) models, respectively. Atoms involved in interfacial hydrogen bonds and intermolecular stacking interactions are shown in stick model.
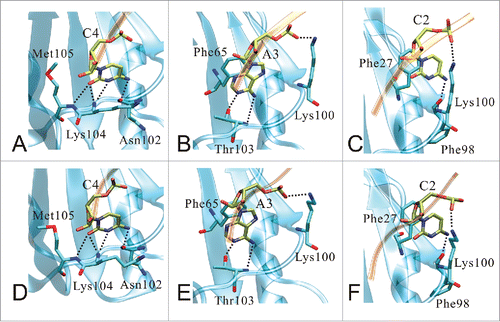
Table 2. The hydrogen bonds formed by RRM domain and RNA with occupancy over 10% for 3 complex systems.
From the above analyses, we discussed the recognition mechanism of the RRM domain and RNA. Through the comparison of free RRM-WT and complex systems, it's found that the RNA-binding interface of free RRM domain is not stable, especially the C-terminal loop. The C-terminal loop in free RRM-WT even adopts the conformation similar to that in the complex-WT system. The previous studies also found that the C-terminal loop is often directly involved in the recognition of the target RNA molecule and are necessary for their ‘positive discrimination’.Citation8,21 Similarly, our study also found that the structure of the C-terminal loop is stabilized by the specific RNA binding in the complex-WT system. The binding affinity between RRM domain and RNA is mainly contributed by the trinucleotides CAC. The nucleotide U1 does not form any high occupancy hydrogen bonds and moves far away from the RRM domain in the representative structures of complex-WT. Both R38Q and T103A/K104A mutations reduce the RNA-binding affinities of RRM domain, but the underling mechanisms are different. R38Q mutation causes the large movements of 2 RRM domains and induces the separation of RBPMS homodimer. Several experimental data proposed that multiple RRMs will help achieving higher affinity and specificity than the isolated RRM.Citation4,22 In this work, although the R38Q mutation does not directly act on the RNA-binding interface, some interfacial hydrogen bonds (Lys100-NZ:Cyt2-O2P and Met105-N:Cyt4-O2) are weakened because of the large fluctuations of RRM domains. Then, the calculated binding energy is increased in complex-R38Q system. In contrast, T103A/K104A mutations mainly change the dynamical properties of RNA. Hydrogen bonds and intermolecular stacking interactions were found to contribute to sequence-specific RNA recognition and binding affinity.Citation23 T103A/K104A mutations break most of high occupancy hydrogen bonds in the interface between RRM domain and RNA. Thus, many key interfacial residues, including Thr103-Met105 and Phe65, lose their favorable energy contributions for RNA binding. The energy decomposition also implies that the intermolecular stacking interactions between A3 and Phe65 could be weakened. The correlated motions between RRM domain and RNA are decreased, and large conformational changes are taken place at nucleotides A3 and C4. Therefore, the complex-T103A/K104A system possesses the highest binding energy and its binding affinity is undetectable. Our study shows that the binding affinity of RRM domain and RNA is influenced by many reasons, such as the homodimerization of RRM domain or the crucial interactions on the RNA-binding interface. Likewise, if the binding affinity of RRM domain and RNA need to be improved, it will be possible to mutate those residues that contribute unfavorable energies.
Conclusions
In this work, MD simulation was applied to investigate the dynamical properties and crucial interactions of RRM domain and RNA. The comparative analysis of MD trajectories indicates that the RNA binding increases the stability of RRM domain, but residue mutations of RRM domain induce the fluctuation of complex systems. The calculated binding energies based on MM/PBSA agree well with the binding affinities. R38Q mutation causes large fluctuations of 2 RRM domains and induces the separation of RBPMS homodimer. These fluctuations of RRM domains weaken the favorable contribution of residue Arg38 and Met105, which makes slight influence on the RNA binding. In contrast, the RBPMS homodimer is relatively stable in the T103A/K104A system, so its global fluctuation values are lower than those of R38Q system. However, T103A/K104A mutations break most of high occupancy hydrogen bonds in the interface between RRM domain and RNA, and large conformational changes take place at nucleotides A3 and C4. These fluctuations go against RNA binding directly, so the binding energy of complex-T103A/K104A system increases remarkably and its binding affinity is completely lost. This study explains the reduced binding affinity caused by different mutations and provides useful insights into the RNA recognition mechanisms of RRM domain.
Systems and methods
Simulation protocols
The RRM domain (residues Glu21-Val111) of human RBPMS in the free state and the RNA-binding state were built from X-ray crystallographic structures (PDB code: 5CYJ and 5DET).Citation10 According to the experimental reference, the free RRM domain is L81M mutant. In order to be consistent with the complex structure, the M81 in the free RRM domain are mutated back to L81 in this work. In addition, there are 2 RNA chains in the experimental complex structure, including chain P and chain Q. Chain P is 4-nt RNA, and chain Q is 5-nt RNA. In order to keep consistence, we retained 4-nt RNA in both chains. Besides the wild types of free RRM domain and RRM-RNA complex, we also modeled 2 mutant systems for comparison with the experimental data. The mutations of residues in the 2 mutant systems were R38Q and T103A/K104A, respectively. The mutations of these residues were performed using CharmmGUI.Citation24
Then, 4 independent molecular dynamics simulations were performed using the NAMD 2.10 programCitation25 with the CHARMM 36 all-atom force field.Citation26,27 In each simulation, the initial structure was solvated in a cubic periodic water box with the edges of the box at least 12 Å from any parts of solutes. The four simulation systems (free RRM-WT, complex-WT, complex-R38Q and complex-T103A/K104A) contained 18924, 17533, 17540 and 17556 TIP3P water molecules with a total of 59814, 55885, 55892 and 55922 atoms, respectively. Sodium and chloride ions were added to each system to get a final ion concentration of 150 nM. The SHAKE algorithm was used to constrain the covalent bonds involving hydrogen atoms.Citation28 The Particle Mesh Ewald (PME)Citation29 summation algorithm was used to calculate the long-range electrostatic interactions. The cut-off of non-bonded interactions was set as 12 Å. Each system was energetically minimized with 20000 steps. Then, all backbone atoms of solutes were restrained with a harmonic constraint of 0.1 kcal·mol−1·Å−2 and the system was slowly heated up from 0 to 298 K over a period of 1.0 ns. Finally, the non-constrained MD simulation was performed at constant pressure (1 atm) by Langevin piston methodCitation30 and constant temperature (298 K) by Langevin dynamicsCitation31 for 120 ns. For each system, the MD integration step was set as 2 fs, and one snapshot was sampled every 1000 steps. Total 60000 conformations were collected for further analyses. The analyses of trajectories including RMSD and RMSF values were performed with VMD 1.9.2.Citation32 RMSD values were calculated over the non-hydrogen atoms of RBPMS RRM domain and RNA, and RMSF values were measured based on the Cα atoms of RRM domain and C5' atoms of RNA.
Dynamical cross-correlated map analysis
Dynamical cross-correlated motions play an important role in the recognition and biological function of a bio-macromolecular system.Citation16,33 In MD simulations, the extent of cross-correlated fluctuations between atoms can be described by the covariance matrix .Citation34 The element
in the matrix is defined as:
(1)
(1) where
(
) is the displacement vector corresponding to
th(
th) atom of the systems, and
indicates an ensemble average. The cross-correlated values range from −1 to 1. Positive values represent positively correlated movements (the same direction), and negative values imply anti-correlated movements (the opposite direction). The higher the absolute value of
is, the more correlated (or anti-correlated) the 2 atoms (or residues) are. In this work, the covariance matrix for all Cα (protein) and C5'(RNA) atomic fluctuations was extracted from the MD trajectory by using the Gromacs 4.5 package.Citation35
Principal component analysis
Principal component analysis (PCA) is widely applied in MD simulations to extract the slow and global functional motions of bio-molecules by using the dimensional reduction method.Citation36,37 On the basis of the above dynamical cross-correlated analysis, the covariance matrix is diagonalized to obtain the orthogonal eigenvectors and the corresponding eigenvalues. The eigenvectors are also called the principal components (PCs), which indicate the directions of the concerted mot ions. The first few PCs usually represent the slow and global functional motions of a bio-molecular system.Citation34,38 The corresponding eigenvalues describe the magnitude of the motions along the direction. In this work, PCA was performed with Gromacs 4.5 packageCitation35 to obtain the global functional motions in the 4 systems.
Free energy landscape
Free energy landscape (FEL) is widely used to identify the dominant conformational states during the biological process.Citation38,39 The relative free energy between 2 states is calculated by(2)
(2) where
is the Boltzmann constant,
is the absolute temperature,
is the reaction coordinate, and
is the probability distribution of system along the reaction coordinate. The unit of the free energy landscapes is kT, where k is the Boltzmann constant and T is absolute temperature. On the basis of the above PCA data, the first 2 PCs are chosen as the reaction coordinates
and
.Citation34,40 Then, the 2-dimensional free energy landscapes are constructed from the joint probability distributions
. In the FEL, the local basins usually represent the conformational ensemble in the relatively stable states, and the barriers indicate the transient states.Citation41
Analysis of the interfacial interactions
The interfacial interactions between RRM domain and RNA are dominated by the hydrogen bonds and intermolecular stacking interactions.Citation10 In this article, the hydrogen bonds were calculated by VMD 1.9.2Citation32 with a distance cut-off value of 3.5 Å and an angle cut-off value of 35°.Citation42 The relative binding energy between RRM domain and RNA were calculated by g_mmpbsa.Citation43 It implements the Molecular mechanics Poisson-Boltzmann surface area (MM-PBSA) approach to estimate interactional free energies. This tool can calculate molecular mechanics potential energy (include both electrostatic and van der Waals interactions) and free energy of solvation (include polar and nonpolar solvation energies). It also has been used to estimate the energy contribution per residue or nucleotide to the binding energy.
Disclosure of potential conflicts of interest
No potential conflicts of interest were disclosed.
Supplemental_Data.zip
Download Zip (11.8 MB)Funding
This work was supported by National Natural Science Foundation of China (31200990), Special Program for Applied Research on Super Computation of the NSFC-Guangdong Joint Fund (the second phase), Science and Technology Planning Project of Guangzhou (1563000117), National Supercomputer Center in Guangzhou and supercomputer resources at Jiangsu University of Technology.
References
- Riley KJ, Steitz JA. The “Observer Effect” in genome-wide surveys of protein-RNA interactions. Mol Cell 2013; 49:601-4; PMID:23438856; http://dx.doi.org/10.1016/j.molcel.2013.01.030
- Jankowsky E, Harris ME. Specificity and nonspecificity in RNA-protein interactions. Nat Rev Mol Cell Biol 2015; 16:533-44; PMID:26285679; http://dx.doi.org/10.1038/nrm4032
- Nagarajan R, Chothani SP, Ramakrishnan C, Sekijima M, Gromiha MM. Structure based approach for understanding organism specific recognition of protein-RNA complexes. Biol Direct 2015; 10:8; PMID:25886642; http://dx.doi.org/10.1186/s13062-015-0039-8
- Clery A, Blatter M, Allain FHT. RNA recognition motifs: boring? Not quite. Curr Opin Struct Biol 2008; 18:290-8; PMID:18515081; http://dx.doi.org/10.1016/j.sbi.2008.04.002
- Nagai K, Oubridge C, Jessen TH, Li J, Evans PR. Crystal structure of the RNA-binding domain of the U1 small nuclear ribonucleoprotein A. Nature 1990; 348:515-20; PMID:2147232; http://dx.doi.org/10.1038/348515a0
- Daubner GM, Clery A, Allain FHT. RRM-RNA recognition: NMR or crystallography … and new findings. Curr Opin Struct Biol 2013; 23:100-8; PMID:23253355; http://dx.doi.org/10.1016/j.sbi.2012.11.006
- Ferré-D'Amaré AR. Use of the spliceosomal protein U1A to facilitate crystallization and structure determination of complex RNAs. Methods 2010; 52:159-67; PMID:20554048; http://dx.doi.org/10.1016/j.ymeth.2010.06.008
- Muto Y, Yokoyama S. Structural insight into RNA recognition motifs: versatile molecular Lego building blocks for biological systems. Wiley Interdiscip Rev-RNA 2012; 3:229-46; PMID:22278943; http://dx.doi.org/10.1002/wrna.1107
- Farazi TA, Leonhardt CS, Mukherjee N, Mihailovic A, Li S, Max KEA, Meyer C, Yamaji M, Cekan P, Jacobs NC, et al. Identification of the RNA recognition element of the RBPMS family of RNA-binding proteins and their transcriptome-wide mRNA targets. RNA 2014; 20:1090-102; PMID:24860013; http://dx.doi.org/10.1261/rna.045005.114
- Teplova M, Farazi TA, Tuschl T, Patel DJ. Structural basis underlying CAC RNA recognition by the RRM domain of dimeric RNA-binding protein RBPMS. Q Rev Biophys 2016; 49:e1; PMID: 26347403; http://dx.doi.org/10.1017/S0033583515000207
- Tuszynska I, Matelska D, Magnus M, Chojnowski G, Kasprzak JM, Kozlowski LP, Dunin-Horkawicz S, Bujnicki JM. Computational modeling of protein-RNA complex structures. Methods 2014; 65:310-9; PMID:24083976; http://dx.doi.org/10.1016/j.ymeth.2013.09.014
- Puton T, Kozlowski L, Tuszynska I, Rother K, Bujnicki JM. Computational methods for prediction of protein-RNA interactions. J Struct Biol 2012; 179:261-8; PMID:22019768; http://dx.doi.org/10.1016/j.jsb.2011.10.001
- Dieterich C, Stadler PF. Computational biology of RNA interactions. Wiley Interdiscip Rev-RNA 2013; 4:107-20
- Scheiba RM, Ibanez de Opakua A, Diaz-Quintana A, Cruz-Gallardo I, Martinez-Cruz LA, Martinez-Chantar ML, Blanco FJ, Díaz-Moreno I. The C-terminal RNA binding motif of HuR is a multi-functional domain leading to HuR oligomerization and binding to U-rich RNA targets. RNA Biol 2014; 11:1250-61; PMID:25584704; http://dx.doi.org/10.1080/15476286.2014.996069
- Bahadur RP, Kannan S, Zacharias M. Binding of the bacteriophage P22 N-peptide to the boxB RNA motif studied by molecular dynamics simulations. Biophys J 2009; 97:3139-49; PMID:20006951; http://dx.doi.org/10.1016/j.bpj.2009.09.035
- Kormos BL, Baranger AM, Beveridge DL. Do collective atomic fluctuations account for cooperative effects? Molecular dynamics studies of the U1A-RNA complex. J Am Chem Soc 2006; 128:8992-3; PMID:16834346; http://dx.doi.org/10.1021/ja0606071
- Casu F, Duggan BM, Hennig M. The arginine-rich RNA-binding motif of HIV-1 Rev is intrinsically disordered and folds upon RRE binding. Biophys J 2013; 105:1004-17; PMID:23972852; http://dx.doi.org/10.1016/j.bpj.2013.07.022
- Chang S, He HQ, Hu JP, Jiao X, Tian XH. Network models reveal stability and structural rearrangement of signal recognition particle. J Biomol Struct Dyn 2012; 30:150-9; PMID:22702726; http://dx.doi.org/10.1080/07391102.2012.677765
- Genheden S, Ryde U. The MM/PBSA and MM/GBSA methods to estimate ligand-binding affinities. Expert Opin Drug Discov 2015; 10:449-61; PMID:25835573; http://dx.doi.org/10.1517/17460441.2015.1032936
- Hou T, Wang J, Li Y, Wang W. Assessing the performance of the MM/PBSA and MM/GBSA methods. 1. The accuracy of binding free energy calculations based on molecular dynamics simulations. J Chem Inf Model 2011; 51:69-82; PMID:21117705; http://dx.doi.org/10.1021/ci100275a
- Deka P, Rajan PK, Perez-Canadillas JM, Varani G. Protein and RNA dynamics play key roles in determining the specific recognition of GU-rich polyadenylation regulatory elements by human Cstf-64 protein. J Mol Biol 2005; 347:719-33; PMID:15769465; http://dx.doi.org/10.1016/j.jmb.2005.01.046
- Cienikova Z, Jayne S, Damberger FF, Allain FHT, Maris C. Evidence for cooperative tandem binding of hnRNP C RRMs in mRNA processing. RNA 2015; 21:1931-42; PMID:26370582; http://dx.doi.org/10.1261/rna.052373.115
- Chen Y, Varani G. Engineering RNA-binding proteins for biology. Febs Journal 2013; 280:3734-54; PMID:23742071; http://dx.doi.org/10.1111/febs.12375
- Jo S, Kim T, Iyer VG, Im W. CHARMM-GUI: A web-based graphical user interface for CHARMM. J Comput Chem 2008; 29:1859-65; PMID:18351591; http://dx.doi.org/10.1002/jcc.20945
- Phillips JC, Braun R, Wang W, Gumbart J, Tajkhorshid E, Villa E, Chipot C, Skeel RD, Kalé L, Schulten K. Scalable molecular dynamics with NAMD. J Comput Chem 2005; 26:1781-802; PMID:16222654; http://dx.doi.org/10.1002/jcc.20289
- Lee J, Cheng X, Swails JM, Yeom MS, Eastman PK, Lemkul JA, Wei S, Buckner J, Jeong JC, Qi Y, et al. CHARMM-GUI input generator for NAMD, GROMACS, AMBER, OpenMM, and CHARMM/OpenMM simulations using the CHARMM36 additive force field. J Chem Theory Comput 2016; 12:405-13; PMID:26631602; http://dx.doi.org/10.1021/acs.jctc.5b00935
- Brooks BR, Brooks CL, Mackerell AD, Nilsson L, Petrella RJ, Roux B, Won Y, Archontis G, Bartels C, Boresch S, et al. CHARMM: The biomolecular simulation program. J Comput Chem 2009; 30:1545-614; PMID:19444816; http://dx.doi.org/10.1002/jcc.21287
- Ryckaert J-P, Ciccotti G, Berendsen HJC. Numerical integration of the cartesian equations of motion of a system with constraints: molecular dynamics of n-alkanes. J Comput Phys 1977; 23:327-41; http://dx.doi.org/10.1016/0021-9991(77)90098-5
- Darden T, York D, Pedersen L. Particle mesh Ewald: an N.Log(N) method for Ewald sums in large systems. J Chem Phys 1993; 98:10089-92; http://dx.doi.org/10.1063/1.464397
- Martyna GJ, Tobias DJ, Klein ML. Constant pressure molecular dynamics algorithms. J Chem Phys 1994; 101:4177-89; http://dx.doi.org/10.1063/1.467468
- Berendsen HJC, Postma JPM, van Gunsteren WF, DiNola A, Haak JR. Molecular dynamics with coupling to an external bath. J Chem Phys 1984; 81:3684-90; http://dx.doi.org/10.1063/1.448118
- Humphrey W, Dalke A, Schulten K. VMD: visual molecular dynamics. J Mol Graph 1996; 14:33-8, 27–8; http://dx.doi.org/10.1016/0263-7855(96)00018-5
- Velazquez HA, Hamelberg D. Conformational selection in the recognition of phosphorylated substrates by the catalytic domain of human Pin1. Biochemistry 2011; 50:9605-15; PMID:21967280; http://dx.doi.org/10.1021/bi2009954
- Maisuradze GG, Liwo A, Scheraga HA. Relation between free energy landscapes of proteins and dynamics. J Chem Theory Comput 2010; 6:583-95; PMID:23620713; http://dx.doi.org/10.1021/ct9005745
- Van der Spoel D, Lindahl E, Hess B, Groenhof G, Mark AE, Berendsen HJC. GROMACS: fast, flexible, and free. J Comput Chem 2005; 26:1701-18; PMID:16211538; http://dx.doi.org/10.1002/jcc.20291
- Wan H, Hu JP, Tian XH, Chang S. Molecular dynamics simulations of wild type and mutants of human complement receptor 2 complexed with C3d. Phys Chem Chem Phys 2013; 15:1241-51; PMID:23229122; http://dx.doi.org/10.1039/C2CP41388D
- Chang S, Hu JP, Lin PY, Jiao X, Tian XH. Substrate recognition and transport behavior analyses of amino acid antiporter with coarse-grained models. Mol Biosyst 2010; 6:2430-8; PMID:20838682; http://dx.doi.org/10.1039/c005266c
- Chang S, He HQ, Shen L, Wan H. Understanding peptide competitive inhibition of botulinum neurotoxin a binding to SV2 protein via molecular dynamics simulations. Biopolymers 2015; 103:597-608; PMID:26178648; http://dx.doi.org/10.1002/bip.22682
- Voelz VA, Dill KA, Chorny I. Peptoid conformational free energy landscapes from implicit-solvent molecular simulations in AMBER. Biopolymers 2011; 96:639-50; PMID:21184487; http://dx.doi.org/10.1002/bip.21575
- Papaleo E, Mereghetti P, Fantucci P, Grandori R, De Gioia L. Free-energy landscape, principal component analysis, and structural clustering to identify representative conformations from molecular dynamics simulations: the myoglobin case. J Mol Graph 2009; 27:889-99; PMID:19264523; http://dx.doi.org/10.1016/j.jmgm.2009.01.006
- Lavi A, Ngan CH, Movshovitz-Attias D, Bohnuud T, Yueh C, Beglov D, Schueler-Furman O, Kozakov D. Detection of peptide-binding sites on protein surfaces: The first step toward the modeling and targeting of peptide-mediated interactions. Proteins 2013; 81:2096-105; PMID:24123488; http://dx.doi.org/10.1002/prot.24422
- Babu MM, Singh SK, Balaram P. A C-H center dot center dot center dot O hydrogen bond stabilized polypeptide chain reversal motif at the C terminus of helices in proteins. J Mol Biol 2002; 322:871-80; PMID:12270720; http://dx.doi.org/10.1016/S0022-2836(02)00715-5
- Kumari R, Kumar R, Lynn A. g_mmpbsa—A GROMACS Tool for high-throughput MM-PBSA calculations. J Chem Inf Model 2014; 54:1951-62; PMID:24850022; http://dx.doi.org/10.1021/ci500020m