ABSTRACT
The fields of applied and translational microRNA research have exploded in recent years as microRNAs have been implicated across a spectrum of diseases. MicroRNA biomarkers, microRNA therapeutics, microRNA regulation of cellular physiology and even xenomiRs have stimulated great interest, which have brought many researchers into the field. Despite many successes in determining general mechanisms of microRNA generation and function, the application of microRNAs in translational areas has not had as much success. It has been a challenge to localize microRNAs to a given cell type within tissues and assay them reliably. At supraphysiologic levels, microRNAs may regulate hosts of genes that are not the physiologic biochemical targets. Thus the applied and translational microRNA literature is filled with pitfalls and claims that are neither scientifically rigorous nor reproducible. This review is focused on increasing awareness of the challenges of working with microRNAs in translational research and recommends better practices in this area of discovery.
Since the discovery of mammalian microRNAs in the early 2000s, there has been a huge effort to understand the functionality of this class of small RNAs. These highly conserved molecules are an essential regulatory element affecting the activation of whole pathways and certainly have a central role in disease processes. Investigators have rapidly explored all manner of ideas related to microRNAs. Much of this work has been elegant, highlighting roles of microRNAs in transcriptional regulation and their potential as biomarkers and therapeutics. Another significant portion of microRNA studies advanced ideas that, although initially intriguing or plausible, have been shown through further, more rigorous study to be of questionable validity. This has created a legacy of ideas that have challenged the clarity of the physiologic roles of microRNAs.
The field of microRNA research is one with unique challenges. The manner of their numerical nomenclature, while highly organized, does not allow an intrinsic understanding of the microRNA's activity or localization in the same manner that gene names can be used. microRNAs can be difficult to assay specifically, as their short length (around 22 nt) and similar sequences can confound hybridization and amplification techniques and can complicate interpretation of cross-species discoveries. Many microRNA assaying techniques are new and not fully characterized, with nonstandard normalization methods, allowing for their misuse or misinterpretation. microRNAs also regulate mRNAs through short (6–8 bp) seed sequences, which by random chance can be found on many genes, making in silico identification of microRNA-regulated pathways possible but also potentially meaningless. All of these challenges have hampered the reproducibility and rigor expected of microRNA experiments, particularly in the translational and applied space. This is important in light of new NIH guidelines to assay rigor and reproducibility for grant funding (http://grants.nih.gov/reproducibility/).
We have evaluated aspects of the microRNA literature, primarily in the translational realm, and have identified both common misconceptions that, in our view, are complicating scientific discovery, and best-practices. It is our hope that by clearly stating these problems related to microRNA studies and suggesting better approaches and examples, a more rigorous and more biologically relevant approach to microRNA research can ensue. Further, we hope to encourage reconsideration of closely held, inaccurate beliefs based on less than rigorous microRNA literature so that the field can advance and the important functions and roles of microRNA can be more firmly established.
Areas of microRNA confusion and pitfalls to avoid
microRNAs are expressed by cells, not tissues
Although this should be obvious, all genes, proteins, microRNAs, and other RNA moieties are expressed by cells. A complex collection of different cell types constitutes a tissue. Thus, macerating a tissue for RNA isolation and discovering the presence of a particular microRNA does not localize it to the most common cell type or the cell type of interest to the investigator. This mistake is common and relates to the anonymity of microRNAs and our current lack of a centralized resource that provides microRNA expression at the cellular level.Citation1-4
Although many microRNAs are ubiquitously expressed, others have expression specificity or marked concentration differences between cell types ().Citation5 While a problem for many cell-specific microRNAs, 2 examples illustrate this point. miR-126 is expressed highly, but not necessarily exclusively, in endothelial cells.Citation6,7 microRNAs miR-451a and miR-144, in a bicistronic cluster, are expressed exclusively in red blood cells (RBCs), while miR-486 is found in RBCs and possibly platelets (Sequence Read Archive [SRA] sample ERR747965). Robust expression of these 4 microRNAs is present in all tissue samples as a result of blood vessels, comprised of endothelial cells containing red blood cells (RBCs), being a constituent of all organs/tissues. While saline flushes can remove most RBCs in laboratory animal tissues, this is not achievable with surgically resected human tissues. However, the extent of rinsing of a tissue, to remove RBCs, may modulate the amounts of these 3 RBC microRNAs and can strongly and unknowingly complicate tissue signals (see point 2).Citation8
Figure 1. microRNA expression is at the cellular level. This schematic representation of an epithelial organ shows shared and unique expression of microRNAs. For ubiquitous microRNAs such as the blue labeled miR-21, the tissue-level signal is a result of expression in almost all cell types. For cell-type restricted microRNAs such as the red labeled miR-150, miR-451a etc. the tissue-level signal is a result of the presence of very specific cell types. Key: epithelial (miR-200b), mesenchymal (miR-143), lymphocyte (miR-150), endothelial (miR-126), red blood cell (miR-451a), macrophage and neutrophil (miR-223).
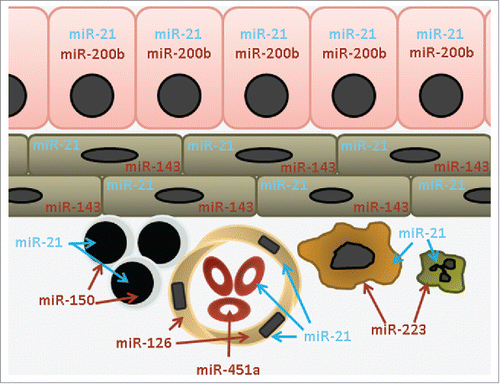
These four microRNAs have been assigned incorrect functional significance in unrelated cell types. Again, because of tissue-level expression data, a miR-126 signal has repeatedly been reported as lower in cancer vs. normal tissue comparisons.Citation9,10 As a result, its levels are then experimentally manipulated, likely inappropriately, in epithelial cancer cell lines, in an effort to determine function.Citation10,11 microRNAs miR-451a and miR-144 have yet to be identified in any non-neoplastic cell type, yet they repeatedly are assigned non-RBC functionality due to tissue level data being confused for cell level expression data ().Citation12-17 miR-486 is also abundant in platelets, but neither RBC nor platelet expression justifies its assigned role in a variety of disease states in which it is unlikely to participate.Citation18,19 Thus it is important to identify the cellular source of a tissue-level microRNA signature, which can be done by checking known datasets,Citation5,20 obtaining cell-specific data from the Sequence Read Archive or Gene Expression Omnibus, or measuring the microRNA by qPCR or northern blot in a cell type of interest.
Table 1. Sampling of studies that assign function to miR-451a in non-red blood cells through cell transfection experiments.
Table 2. Sampling of studies that assign function to miR-144 in non-red blood cells through cell transfection experiments.
Might a normally absent microRNA be “turned on” in a disease state? Although plausible, as discussed in point 4 below, microRNA expression levels appear to be tightly regulated and tend to not have the marked fold changes observed for mRNAs. Thus, if a microRNA is not expressed in a certain cell type in a non-perturbed state, it is unlikely to be significantly increased by perturbation. It is worth noting that cancer-derived cells and cell lines may not express the same microRNAs as a matched native cell due to larger chromosomal structural changes and major pathway alterations. Therefore, caution should be taken in making that comparison. For almost all microRNA studies, it is essential to have definitive knowledge of the cellular origin of the microRNA.
microRNA expression levels will vary in tissue when the cellular composition of the tissue has changed in disease or malignancy
As a correlate to point 1, because many microRNAs have widely variable expression differences between cell types, the ratio of cell types in tissues is important. The cellular composition of tissue changes in most disease states. In cancer, malignant cells replace the native epithelium. In inflammatory diseases, the number of infiltrating leukocytes can increase markedly. If a rare cell type has a 4-fold increase in a tissue, then its cell-specific microRNAs will also increase in a commensurate way (). Several examples from the literature support this underappreciated concept. The Tewari group demonstrated that the plasma expression level of miR-223, a microRNA with exclusivity to neutrophils and monocytes, strongly correlated with the absolute neutrophil count (R = 0.76).Citation21 In a study of inducible colitis, the Zen group showed a strong increase of miR-150 in colons with inflammation.Citation22 miR-150 is highly expressed exclusively in lymphocytes, which are increased multi-fold in colitis. Thus a mere alteration in the ratio of one cell type to another can drive perceived changes in tissue microRNA levels.
Figure 2. Cellular composition is an important driver in changing microRNA signals. Each circle represents a unique cell type making up 1% of a total tissue signal. In the control tissue, 7 different cell types make up 100% of the tissue, with the most abundant cell type (blue) being 40% of the tissue. In disease, the cellular composition can change dramatically and a rare cell type (green) can increase 3-fold. Any cell-specific microRNAs will increase 3-fold, without necessarily altering their expression in the tissue.
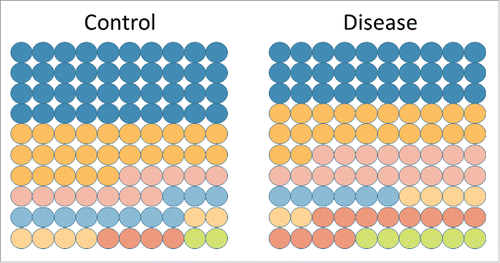
It is not yet possible to deconvolute complex tissues into their intrinsic cell types based on microRNA expression levels alone. To avoid the pitfall of conflating tissue composition and microRNA expression changes, histopathologic examination of case and control tissue can be cross-checked against microRNA expression manuscripts to identify microRNAs that may change only as a result of cellular composition changes. A microRNA level change in tissue does not imply necessarily its up or down regulation. Failure to recognize this cause of expression alteration has probably adversely affected the interpretation of numerous studies.
For the measurement of microRNAs, methodologic details matter
The tools that have been developed to analyze microRNA expression are still being refined, and several technical and methodologic challenges remain. In recent years, for example, small RNA-seq for microRNA (microRNA-seq) has become a major method to analyze microRNA expression. While microRNA-seq is used extensively, it does have its limitations. Here, we will discuss several challenges related to microRNA-seq in general and normalization methods specifically.
microRNA-seq normalization
Currently, most microRNA data sets are normalized to reads per million microRNA reads (RPM). This is similar to Fragments Per Kilobase of transcript per Million mapped reads (FPKM), established for mRNA reads, without a need to normalize for transcript length since mature microRNAs are all ∼17-25bp. Also, since the number of reads can vary >10-fold within/between projects, it is superior to using raw microRNA counts. The fundamental problem with RPM is that it is a dependent normalization value, such that any change in one microRNA's read counts will adjust all other microRNAs values whether or not absolute expression actually changed (). Between random fluctuations in sequencing reads and such a change, one could identify statistically significant microRNA changes that are a feature of other fluctuations. Array and qRT-PCR based methods do not have the same challenge, as these are independent observations.
Figure 3. microRNA-seq normalization (RPM) is a dependent normalization. In this example, a single common microRNA (200,000 RPM by microRNA-seq), identified as green *is experimentally doubled (to 400,000 RPM). No other microRNAs (all other colors) are expected to be altered. However, because all are normalized by RPM, which is dependent of the sum of all reads, every other microRNA will decrease proportionately to account for the increase in the single modulated microRNA.
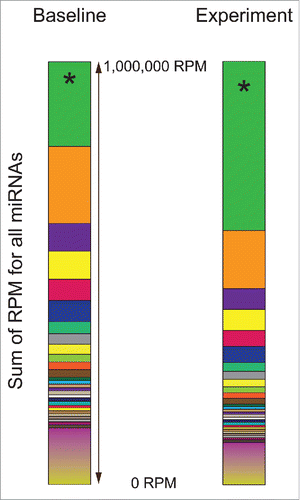
microRNA-seq alignment
A second challenge of microRNA-seq is the diversity of alignment tool methods. These have an outsized effect on which microRNAs are identified and how many reads are called. In a comparison study, we used 8 different tools on the same datasets.Citation23 The differences are dependent upon the library to which the microRNAs are being aligned (genome or hairpin microRNA), the alignment tool (Bowtie, BLASTn, PatMaN), the alignment parameters (allowed mismatches) and handling of reads mapping to multiple locations. For a sample containing 33.23 million reads (SRA sample SRR873410) the methods ranged from discovering 26.24 million microRNA reads down to only 16.39 million microRNA reads. In this same data set, the methods found between 489 and 1,499 microRNAs in total. Thus, differences in alignment tools can significantly impact on the counting of microRNAs in a sample. We found strong agreement between 2 new tools, miRge and Chimira and can recommend them both.Citation23,24
microRNA-seq library preparation
Finally, there are biases in microRNA-seq library preparation, mainly due to challenges of adaptor ligation, GC content, and PCR amplification bias.Citation25-27 The most commonly used method for Illumina sequencing is the TruSeq SmallRNA kit. As an example of the biases that exist, we have found that in almost all examples of microRNA-seq using this method there is a ∼40 fold higher level of miR-143 than miR-145, 2 microRNAs in a bicistronic cluster. However, by other methods including RNA gel blot and qRT-PCR, these levels are significantly more equivalent.Citation28-30 Two studies have demonstrated wide differences in microRNA RPM depending on the use of different library preparation kits (TruSeq small RNA [Illumina], NEXTflex [Bioo Scientific], and NEBNext [New England Biolabs]), with some microRNAs having counts as high as 100,000 RPM by one method and <1,000 RPM by a different method from matched samples.Citation31,32 Thus in comparing studies, it is important to keep these concerns in mind and for all projects within a given laboratory or core sequencing facility be consistent in regards to the preparation method used.
For studies designed to investigate a handful of microRNAs or even hundreds of microRNAs, other methods such as qPCR arrays, hybridization arrays, droplet digital PCR (ddPCR), or NanoString, may be better approaches. In a head-to-head analysis of methods in the miRQC study, the sequencing methods were not superior and, in fact, correlated less-well to the other methods for matched samples.Citation33 The improvements that need to be made for microRNA-seq to become a true gold standard are 1) improvement of library preparation to reduce bias; 2) the use of a consensus method of alignment; and 3) the development of universal internal controls as has recently been proposed.Citation34
Another big methodologic challenge concerns methods to measure microRNAs in biofluids, particularly finding and using appropriate internal or external controls to which the data can be normalized. In the first paper describing circulating microRNAs for cancer detection, the Tewari group used 3 spiked-in C. elegans microRNAs to normalize the data.Citation35 Other groups have tried a variety of methods, including housekeeping-style RNAs with the small nucleolar RNA U6 (RNU6B, U6) being one of them. U6 has been used at least as far back as 2007 to normalize microRNA data, despite evidence showing its poor performance as a normalization tool in some settings.Citation36,37 Whether or not it is the best normalization standard for tissue and cell work is beyond the scope of this commentary, and we recommend several manuscripts on the topic for further information.Citation37-39 However, for serum and plasma, it is likely unacceptable. U6 would be present in serum or plasma only as a result of cell lysis or coagulation, which are variable events and highly influenced by pre-analytical factors (clotting differences, spin speed differences, red blood cell lysis, storage temperature, etc.).Citation40,41
The best approach to normalize serum/plasma microRNA is still open to debate. A microRNA spike-in during the extraction and amplification steps is a current best practice but does not provide biological normalization.Citation42 If the experiment is using an array-based method with hundreds of microRNAs, a global normalization approach may be useful.Citation33,43 Many microRNAs have been tried as “housekeeping genes,” with the most common being miR-16. However, this microRNA has also been reported to vary in many human diseases.Citation5 Thus, while there may not yet be an agreed upon way of doing this normalization in a fluid sample, the main pitfall to avoid is the use of any single RNA species, such as U6, without evidence for its reliability.
Extremely large microRNA fold changes can suggest poor normalization, not interesting biology
Most well-performed studies that have investigated microRNA expression changes in a disease state generally find small (∼1.5-4-fold) changes in microRNA levels.Citation44-46 This may not hold in cancer, where, as described above, genetic alterations may drive some large differences in a microRNA signal. Also, microRNAs may change to a greater extent during development.Citation47 Nonetheless, huge fold differences in microRNAs in somatic adult cells may be due to a methodologic problem, often poor normalization. For example, a 2013 study investigated microRNAs as serum biomarkers for endometriosis.Citation48 Using a TaqMan microRNA array, the investigators found 22 microRNAs that all had >1,000 fold differences between samples. However, this conclusion was based on 2 pooled samples of 10 individuals each. Therefore, any technical variables could not be accounted for or removed. The need to pool samples for such experiments is often based on financial resources, but it carries a high cost of uncontrolled variability. Additionally, a subset of these microRNAs were “confirmed” in a second sample of serum, with normalization to RNU6B, which was described in point 3 as inappropriate as it is not native to serum.
microRNAs must be present to work: The importance of minimal thresholds of expression
Experimental noise—background signal—is all too often misinterpreted as fertile ground for biomarker discovery. A detection or abundance threshold is a must for any profiling study, since undetected features cannot be normalized or assessed for expression differences. Unfortunately, scores of studies have reported putatively significant findings that are simply noise (examples available upon request).
As an example, 2 very closely related reports described differential regulation of microRNAs in brain cells exposed to HIV-1 proteins.Citation49,50 Specifically, 69 microRNAs had a 2-fold change or greater response to treatment in neurons and a neuronal cell line. A reanalysis of the microarray data revealed that only 1 of the 69 microRNAs was expressed above a common detection threshold setting (negative control (background) signal plus 2 standard deviations). Despite this, numerous microRNAs were “confirmed” by quantitative PCR.
It is often obvious if a study has generated seemingly significant findings within the noise of the data. These papers report obscure microRNAs or the anti-sense microRNA (not Argonaute (AGO)-incorporated), which mostly has extremely low levels of mature expression. These papers often claim large fold changes of expression which are beyond the physiologically reasonable changes in disease (see point 4).
The pitfall to avoid is reporting noise as results. Certain basal levels of expression should be adhered to, and the minimal threshold should be reported in the methods section. As stated, 2 standard deviations above background signal, which indicates statistical significance, may be a reasonable cutoff level for hybridization array based data. For microRNA-seq, a rule of thumb is that microRNAs should have values above 100 RPM, with the added caveat that changing levels of one microRNA affects levels of all others (point 2, above). Indeed, microRNAs with <1,000 RPM may not have biologic activity.Citation51 It is also worth determining if the described microRNA is expressed in the cell type of interest (point 1). Failing to account for noise, one will very likely identify a host of obscure microRNAs that have no biologic functionality in the tissue or cell type being evaluated. Such results are unlikely to be reproducible in follow up experiments, and functional studies of these microRNAs are generally artificial.
Too much of a good thing: Supraphysiologic microRNA overexpression can cloud interpretation
The ability to transfect or otherwise introduce microRNAs or their inhibitors into cells is important to understanding the biology and regulatory behavior of this class of RNA. However, one must be aware of potential problems. For example, abnormal AGO loading and formation of high molecular weight aggregates have been reported in transfection experiments,Citation52 while the presence of microRNA inhibitors has been found to directly inhibit qPCR reactions. Length-dependent activation of dsRNA sensor pathways by microRNA mimics (but not by commercially available 21-nucleotide controls) can also cloud interpretation of results.Citation53,54 While exogenous delivery of microRNAs are routinely performed and widely published, just as with mRNA studies, marked overexpression of a microRNA can result in biologically inaccurate discoveries. The recent efforts to understand extracellular vesicle (EV)-mediated transfer of microRNAs through the use of transfection are illuminating.
With the seminal work by Valadi et al, the field of EV microRNA transfer was born.Citation55 There has been a significant effort to understand how microRNAs are packaged (selective versus stochastic) and transferred, and into what cell types they can be delivered to provide paracrine signaling.Citation56-58 Many groups break this latter experiment into 2 parts. First they demonstrate transfer of EV from one cell type to another, often using fluorescent lipid dyes that may themselves form micelles or transfer promiscuously. Then they use a more traditional transfection approach to increase their microRNA of interest in the recipient cell and assess its effects. The problem with this approach is the vastly different uptake of microRNAs via EVs and artificial liposomal systems. As seen in , the ability of recipient cells to uptake microRNA by EV transfer likely differs, by orders of magnitude, to microRNA levels achievable by transfection. This supraphysiologic overexpression of microRNA obtainable by transfection may have different biological functions as described below.
Figure 4. A comparison of exosomal transfer and culture transfection experiments. (A) In in vivo exosomal transfer, donor cells generate exosomes which then enter the circulation or traverse the local environment of extracellular matrix. These exosomes then bind and fuse to recipient cells. The kinetics and specificity needed to target a particular microRNA from a donor cell to be uptaken by a specific recipient cell to sufficiently modulate levels of that microRNA in the second cell type are challenging. (B) In an ex vivo cell transfection experiment, saturating levels of microRNAs in lipid-based systems (or similar) are used to markedly increase the level of the microRNA in a cell, often to supraphysiologic levels.
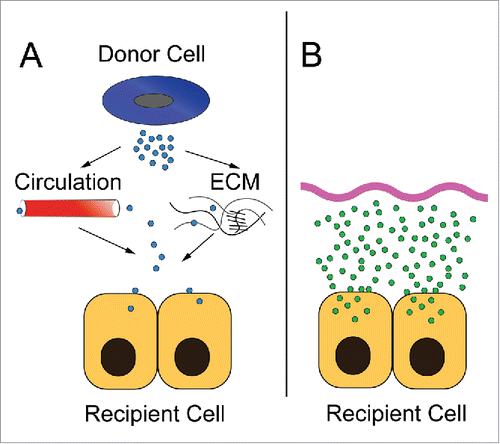
Consider the abundance of microRNAs in EVs in the circulation. Using a published estimate of microRNA copies per exosome,Citation59 and selecting a hypothetical abundant microRNA with a prevalence of 5% of total microRNA, one copy would be expected in every 2400 EVs. This estimate is close to the finding of abundant miRs-223 and −126 at a frequency of one copy per 7,000 and 18,000 blood plasma EVs, respectively.Citation59 Based on our nanoparticle tracking data and published blood EV abundance estimates,Citation60 there may be approximately 3 to 11 trillion EVs in circulation in the average human, with around 1 to 5 billion carrying a copy of our hypothetical microRNA. These “loaded” EVs, though, are part of a system that includes about 25 trillion erythrocytes, 1.5 trillion endothelial cells, 1.5 trillion platelets, and 500 billion lymphocytes (based on estimates from Bianconi E et alCitation61 and Sender, et alCitation62). Even if all blood EVs could somehow be concentrated and delivered to a specific, small cell population (with no uptake by any of the cell types listed above), circulating blood carries only enough EVs to introduce a regulation-relevant thousand or more EVs into only a million or so cells around the same number one might culture in a single well of a 6-well culture plate. In contrast, typical microRNA mimic transfection experiments involve millions of copies per cell, delivered using lipid components optimized for nucleic acid delivery into the cytoplasm of cells.
We do not suggest that EVs have no role in delivering microRNAs in vivo. In tissue, especially at local cell-cell “synapses,” concentrations of EVs might reach levels that are relevant to functional transfer of microRNA. Although much remains to be learned about EV-mediated delivery,Citation63,64 some EVs might also deliver microRNAs in the “right,” i.e., AGO-associated, form to plug into regulatory complexes more efficiently than exogenous synthetic complexes. EV-delivered microRNAs might also accumulate in specific cells over extended periods of time, to be activated under certain stimuli. However, it is clear that there are insufficient microRNA-containing EVs in the entirety of human circulation to achieve the immediate, per-cell exposure levels of typical microRNA transfection experiments, and this must be considered when designing experiments and interpreting results.
How does supraphysiologic overexpression affect the functionality of the microRNA in the cell? Mayya and Duchaine have shown that microRNAs work in a variable dose-response fashion with their mRNA binding sites.Citation65 Thus, the concentration of a microRNA may dictate which genes it regulates. If the microRNA is at a low concentration it may modulate only a subset of genes, whereas at high concentrations, it may find additional mRNA binding partners. Which of these are physiologic partners and which are non-physiologic “off-target” effects are not known but are likely impacting biologic pathways and cell phenotypes in different ways.
A caveat: it is unknown what percent of microRNAs from either method are incorporated into the RISC complex. Interesting work by Thomson et al suggests that only a small percent of transfected microRNAs end up in a potentially functional pool.Citation53So perhaps the discrepancy is not as wide as thought, but this should be clarified before more 2-step exosomal microRNA transfer experiments are performed in this fashion. To avoid this pitfall, one should consider the biological levels of the microRNA on a cell and transfect it accordingly. Supraphysiologic levels could lead to unintended and biologically unlikely regulatory activities. It is also worth noting that experimental expression of a microRNA in a cell type that does not natively express the microRNA, will likely result in a change to the cellular phenotype. This is simply the result of the minimalist requirement of a short (6-8bp) complementary seed sequence to be present on an mRNA that can be bound by abundant microRNAs in a RISC complex. These phenotypes could be interesting and important from a therapeutic angle, but not necessarily biologically relevant.Citation20
The tyranny of numbers: Factors beyond microRNA concentration also affect gene regulation
Even if a microRNA is abundant under physiologic conditions, it might not play an important role in posttranscriptional regulation of specific RNAs. This is because microRNA concentration is only one of several factors that influence the extent of regulation. Even changes in concentration of microRNAs may be inconsequential. A common approach to determining microRNA regulation of a gene in the literature is as follows: 1) profiling reveals that miR-X is upregulated in a disease of interest; 2) an in silico predicted target of the microRNA, Target A, is related to the disease and is downregulated; 3) supraphysiologic miR-X overexpression in a transformed cell line partially silences a reporter with recognition sites from putative Target A; 4) miR-X must regulate Target A. This is often true, however, there are many additional factors that must be considered in microRNA-mediated regulation, particularly due to the supraphysiologic and ex vivo nature of these experiments.
Target levels
Perhaps the most intuitive factor is target concentration.Citation66 A microRNA that increases 2-fold under cell stimulation might not have a proportional effect on a target mRNA with 100-fold greater transcriptional abundance under the same circumstances. Each microRNA species may regulate many messages and the fold expression effect can be diluted out by its affinity to binding partners other than the gene of interest. Nor are mRNAs the only RNAs recognized by microRNAs. microRNAs have been found in RISC in proximity to a variety of RNA molecules, including long noncoding RNAs, tRNAs, even other microRNAs.Citation67,68 Altering the levels of one “competing” target will not necessarily have a strong effect on other targets.Citation69
Interactions
Complicating the issue further is our limited understanding of how microRNAs interact with targets. Canonical, perfect seed matches may be enriched and relatively simple to search for, but they are not the only modes of microRNA:target recognition. Some interactions appear to have no seed-based interaction at all.Citation68 Quantitating and correlating altered microRNA and transcript (or recognition element) levels, then matching them up via bioinformatics approaches, does not determine direct regulation. The cross-linking, ligation, and sequencing of hybrids (CLASH) approachCitation68,70 which binds microRNAs to their targets, does demonstrate a true interaction and should be a more advantageous approach. Unfortunately, it has not been widely adopted and, like earlier methods, requires large amounts of sample to generate small amounts of direct interaction data. More sensitive refinements of CLASH and related methods are needed to assess direct binding and regulation in smaller samples, since the “regulome” for each specific microRNA is likely to be different from one cell type to another and under different conditions. Full and accurate knowledge of the target landscape is essential to understanding effects of microRNAs.
Subcellular location
The subcellular localization of microRNAs strongly affects what putative targets they can regulate. First, the nuclear/cytoplasmic ratio of a specific microRNA will clearly affect its availability for canonical effects on messages. If a microRNA is upregulated during disease, no change in its regulatory influence would be expected if the nuclear ratio also shifted such that most of the “extra” copies were found in the nucleus. Similarly, a tilt toward the cytoplasm for a predominantly nuclear microRNA could lead to increased target regulation even if the overall microRNA level did not change or even declined. Second, location of a microRNA and its cognate mRNA within the cytoplasm might influence whether the message is translated, cleaved, or repressed. It has been proposed, for example, that P bodies are repositories of “inactive” mRNAs.Citation71 Alternative interpretations have been proposed, though. Transcripts of important proteins are held in reserve with microRNAs and translational machinery near synapses, far from the nucleus.Citation72 Upon appropriate signaling, proteins can be made locally and rapidly without waiting for communication with the nucleus.
Active vs inactive microRNA complexes
In our view, one of the potentially most transformative recent insights in the field was reported by La Rocca and colleagues.Citation73,74 In many or most mammalian tissues—and presumably in most cells—microRNAs were found predominantly in a low molecular weight form (inactive complex with AGO) rather than a high molecular weight form (within an active RISC complex and bound to mRNA).Citation74 Complexed with AGO alone, microRNAs might be long-lived, but they have no regulatory influence.Citation73 To regulate, a microRNA:AGO must be incorporated with other proteins into RISC and associated with a target RNA. La Rocca et al. found that the exceptions to the inactive, low mass forms were from high-activity organs such as thymus (immune cell development and maturation) and brain (high energy use). In vitro, cancer cell lines and activated cells also had predominantly high molecular weight complexes. These findings suggest that microRNA-mediated regulation may be rare and inconsequential in most adult cells and is important chiefly in development, proliferation and activation, high metabolic activity, and cancer. In stimulated T cells, compared to resting T cells, redistribution of microRNAs into the high molecular weight form was distinct for each microRNA. Strikingly, La Rocca et al reported a microRNA that declined in overall abundance during T-cell activation yet became a more functional regulator as it was incorporated into active, high molecular weight complexes.Citation74 In future studies, one must now consider not only abundance across the microRNA:target network, but also the activity state of AGO and microRNAs in the cell.
Public datasets and methods – Use! (but with caution)
One of the benefits of our information age and the sharing economy is the number of publically available data sets and tools to evaluate microRNAs. There is significant value in utilizing these tools (). However, caution and skepticism should always be advised as, in general, these materials may not have been developed to address one's exact question.
Table 3. Recommended online microRNA resources.
An important challenge for microRNA bioinformatics is the prediction of mRNA targets. Multiple programs built on different algorithms have been developed, including miRanda, TargetScan, RNA22, and RNAHybrid.Citation75-78 Due to differences in their models, each will predict different gene targets for any given microRNA. While some researchers have focused on any gene that shows up on multiple lists made by the different tools, no consistently significant enrichment of “true” targets was found in a test of this method.Citation79 We further recommend confirming that possible mRNA binding partners are expressed in the appropriate cell type and at a reasonable level relative to the microRNA (see points 1 and 7).
Another approach to finding relevant gene-microRNA interactions is to query “validated” target databases. These tools use the literature and/or public databases to find microRNA-target interactions with experimental support; however, they should be used with caution and at least some fact-checking. We have previously noted the pitfalls of algorithms that apparently use simple text searches,Citation80 such that, e.g., a mention of “β-actin” on page 122 of an edition might be recorded by the algorithm as miR-122 targeting the protein. We have also observed that the number and identity of interactions predicted by some databases can change drastically from month to month, even without version updates, compromising reliability.Citation80 An example of a reliable validated target database is DIANA-TarBase, which is “manually” curated and helpfully provides not only the supporting study or studies for each interaction, but also valuable methods information.Citation81 Even so, each interaction rests on studies that may have their inherent idiosyncrasies. If possible, it is recommended to check the primary sources for interactions of interest.
Related to target prediction and validation, many microRNA studies consist of profiling experiments followed by extensive pathway analysis. These differentially expressed microRNAs are assigned to genes, which are, in turn, assigned to biological pathways. Selected pathways are then discussed in the context of the biological question. This approach involves multiple levels of prediction and potential bias. Algorithms or literature records are used to assign microRNAs to possible targets. Unless gene expression data are available, the relative concentrations (or even presence) of targets are not assessed in the cell or tissue being studied. Pathway analysis introduces a publication bias, relying on published interactions and pathway associations. With much of the field focused on the role of microRNAs in neoplasia, it particularly biases microRNA function into cell proliferation pathways. Finally, identified pathways are integrated into a discussion. What is an appropriate control for a pathway analysis? Would a random selection of microRNAs also yield “significant” pathways that could be rationalized post-hoc? In general, we would suggest that pathway analysis is of limited importance and utility when performed alone. It is useful, in conjunction with other data and analyses to suggest interactions that are experimentally confirmed.
A substantial and growing amount of microRNA expression data is present at the Gene Expression Omnibus (GEO) or Sequence Read Archive (SRA). These data repositories can be used to evaluate microRNA localization (see point 1) or microRNA response to a stimulus. Quality checks should be performed when using public data as we have found them to be variable. For microRNA RNA-seq, the percent of all reads that are called microRNAs is important. In our experience, <25% is poor, 25–50% is likely OK, and >50% is typical of good data. It is also important to have a depth of coverage >1,000,000 microRNA reads for accurate RPM values. For array data, as stated above, first check that signal exceeds background, then ensure that an appropriate global normalization has been performed.
Case studies to identify rigor and reproducibility challenges and successes
Some of the influential manuscripts in the microRNA field represent excellent case studies to help work through the challenges and successes of microRNA research. We present 4 important and often well-cited publications as case studies to demonstrate the challenges in rigor and reproducibility and how to successfully navigate through them.
Case Study 1: In 2007, a major study reported on the downregulation of microRNAs in HIV-1 infection.Citation82 Huang et al's profile of microRNAs in activated and inactivated CD4+ T-cells reported active T cells are better hosts of HIV replication than resting cells. Downregulated microRNAs, the authors reasoned, could be involved in repressing HIV transcripts, so they used target prediction algorithms to identify potential microRNA recognition elements in the 3′ portion of the HIV genome. Five microRNAs that were 2x downregulated and had predicted binding sites in HIV RNA were selected for further investigation (miR-28, miR-125b, miR-150, miR-223, and miR-382). All five microRNAs were found to bind directly to HIV sequences in reporter assays and to have a negative effect on HIV when transfected together into cells.
Numerous subsequent studies have taken these 5 microRNAs to be an exclusive set of reliable “anti-HIV” microRNAs, even though no additional confirmation of results was done and despite identification of many additional candidates according to the supplemental materials of the original study. To our knowledge, there has been no published reproduction of the luciferase and mutation assays to confirm direct targeting of HIV by specific microRNAs. A seminal “guidepost” manuscript, such as this, can sometimes restrict subsequent studies and allow other manuscripts to be published with less-rigorous methodology. For example, a later study used a microarray approach to identify anti-HIV microRNAs that were downregulated by cocaine treatment of CD4+ T-cells.Citation83 The authors reported that the same 5 microRNAs were downregulated by cocaine treatment. However, these data were cherry-picked, as analysis of the raw microarray data demonstrated that every detected microRNA on the array was “downregulated” by cocaine treatment—presumably the result of a dye bias due to improper methods. Essentially, this second paper's findings were accepted as plausible as they were aligned to the earlier study. This story reminds us to carefully interpret these “guidepost” manuscripts and subsequent studies and to maintain a healthy level of skepticism until the data has been rigorously confirmed.
Case Study 2: A recent manuscript is a good example of how to find fluid based microRNA biomarkers in disease. Allen-Rhoades et al. designed a study to identify plasma microRNAs that could detect and monitor childhood osteosarcoma.Citation43 The first component was performed in an osteosarcoma mouse model. After power calculations were performed on how many animals would be required, human osteosarcoma tumors were grown in nude mice with serial plasma collections over time. All plasma samples were treated identically and all aspects of the method were reported including centrifugation speed, time and temperature. RNA extraction was monitored by the addition of synthetic microRNAs that were evaluated by PCR for consistency. microRNAs were initially evaluated by Exiqon array and normalized by global means after removing outliers and delineating a background threshold level. Four microRNAs were selected for follow up based on their fold changes and evaluation of the literature.
Validation of significant microRNA findings were performed in a second set of mice by a qPCR method. The qPCR method was normalized to 3 microRNAs and the synthetic spike-in microRNA determined using the GeNorm and NormFinder algorithms established by the array data.Citation84 Validation confirmed one microRNA with lower signal and 3 microRNAs with increased signal. After validation, human samples from 3 sources were used. This can be less than ideal as different initial preparation and storage methods can alter microRNA expression levels.Citation41 Nonetheless, within the project, these fluids were handled consistently. Global microRNA analysis was replicated in the human sample to find controls appropriate for normalization. Finally, the microRNA signature was replicated in the human subjects by qPCR.
Key strengths of this approach include 1) robust and consistent methods; 2) population sizes based on power calculations; 3) appropriate controls for microRNA expression levels; 4) developing a basal threshold of expression; 5) initial testing in an animal model with significantly reduced signal heterogeneity compared to humans; 6) follow up on both elevated and decreased microRNA signals; 7) validation in human subjects.
Case study 3: Our third case study involves a popular concept, not just one paper: the idea that microRNAs from the diet or other environmental sources (xenomiRs) impact on mammalian cell function. According to the hypothesis, microRNAs from food first survive processing, then pass through the harsh environment of the digestive tract intact, cross the intestinal barrier, enter the blood circulation, and finally, act systemically at zeptomolar to femtomolar concentrations to cause demonstrable phenotypes (see, for example, the concentrations in [85]). The premise of the function of such xenomiRs may come from studies of C. elegans, which takes up dsRNA from its environment and incorporates it effectively into the RNAi pathway.Citation86 However, mammals lack the C. elegans mechanisms for uptake,Citation87 systemic distribution,Citation88 and amplification.Citation89,90
The landmark publication on plant xenomiR uptake reported rice microRNA miR168a was abundant in mammalian serum and plasma and could bind the LDLRAP1 mRNA.Citation91 This finding has now been attributed to artifact or contamination.Citation92-95 Furthermore, artificial overexpression of a foreign microRNA in vitro can always have an effect as described (point 6), but this does not mean it is involved in a natural process. Numerous follow up studies have presented a mixed picture regarding xenomiRs. Many robust uptake experiments using a variety of species and microRNA sources (plant and animal) have found no significant influx of microRNAs into the bloodstream.Citation92,93,96-98 In one human feeding study involving dicots, the most efficiently absorbed microRNA was a monocot microRNA that would not have been present in the food source.Citation99,100 Transgenic mouse studies found no uptake of specific milk microRNAs.Citation101,102 Finally, positive results from a milk intake study involving humansCitation103 could not be validated.Citation104
Exciting and new concepts in the microRNA oeuvre must be supported by rigorous data. When dealing with very low RNA concentrations, one must be on the outlook for laboratory contaminants, nonspecific amplification, sequencing bias, and effects of analysis pipelines, among others, that are more parsimonious explanations for the presence of foreign RNA than hypothetical uptake pathways. For any novel finding, rigorous adherence to optimal methods - adequate controls, sufficient reproducibility (‘n's), and public availability of raw data – is vital to evaluation and general acceptance.
Case Study 4: The Massagué laboratory reported microRNAs miR-126, and miR-335 suppress breast cancer metastasis based on their interpretation of a large number of experiments.Citation105 While this study may be generally correct, there are reasons to be skeptical that these microRNAs represent definitive metastasis suppressors. Their initial study compared highly metastatic clones of the breast cancer cell line MDA-MB-231 to the parent MDA-MB-231 and was performed by TaqMan microRNA assay. They demonstrated higher expression of 8 microRNAs (miR-126, miR-489, miR-127-3p, miR-199a-5p, miR-122, miR-203, miR-206 and miR-335) in the parent MDA-MB-231 cell line. In subsequent publically-available datasets, by Agilent or Affymetrix hybridization microRNA arrays (GEO records GSM1564334 and GSM1571270) or by small RNA-seq (SRA record SRR029132), these microRNAs are either trivially expressed or not expressed in MDA-MB-231. This may indicate different MDA-MB-231 parental clones across these experiments and speaks to the NIH's concern over the identity and validity of cell lines. Or it may indicate interpreting low to negligible expression levels for these microRNAs. miR-126 is well-known to be highly expressed in endothelial cells, miR-122 is well-known to be exclusively expressed in hepatocytes at functional levels, and miR-206 is known to be expressed exclusively in skeletal myocytes (see point 1).Citation7,106 So despite a caveat that malignant cells can have aberrant microRNA expression, it is unlikely MDA-MB-231 gains expression of all 8 of these microRNAs only to lose them during metastasis. Because these microRNAs had such low expression in the parent line, these significant fold-changes could have easily been experimental noise (see point 5) or perhaps differences among quantitative expression platforms (qPCR vs array and RNA-seq).Citation33 To validate the importance of these microRNAs, they overexpressed, by 2-5 log10 fold, 3 of these microRNAs and saw marked reduction of lung colonization. However, supraphysiologic overexpression (see point 6) is likely to be toxic and indeed the measures of anti-metastasis effects (cell proliferation reduction, apoptosis, and reduced migration) are frequently reported with supraphysiologic overexpression of inappropriate microRNAs (). Additionally, they reported low levels of miR-126 and miR-335 corresponded with clinical metastases based on human breast tissue samples. Although it is difficult to know which cell type provided the most miR-335 signal (expression is high in retinal pigment epithelial cells [SRA SRR493011], CD14+ monocytes [SRA SRR527681], and neural stem cells [SRA SRR1988280]) the tissue-level signal for miR-126 would be from endothelial cells and would indicate the vascularity of the tissue and probably not changes in epithelial miR-126 levels (see point 2). The sum of these points is to question the strength of this finding and beware of other studies that reference this paper to suggest miR-126 and miR-335 have a role in epithelial cancer.Citation107
Conclusions
The application of microRNAs to the fields of applied and translational research are exciting with much room for growth and discovery. The points outlined through this manuscript speak to general concerns and successes in how microRNA research is being conducted to achieve high levels of rigor and reproducibility. As we conduct our own research and review that of others, we keep some of these key points in mind:
A strong knowledge of the cellular origin(s) of a microRNA of interest.
Consideration of the caveats to microRNA measuring systems, knowing there is not a single gold-standard for all methods and knowing the complexities of measuring within tissues.
Vigilance to other interpretations of microRNA-based data and willingness to run additional experiments that challenge our preconceptions.
Cognizance that microRNA regulatory roles are more nuanced than just the amount of the microRNA in a cell.
Testing microRNA-RNA interactions in appropriate biological systems and not relying purely on in silico methods.
Striving to achieve the highest level of rigor in our microRNA experiments and be transparent in our science by depositing our raw data to public repositories.
Disclosure of potential conflicts of interest
No potential conflicts of interest were disclosed.
Acknowledgments
The authors thank Dr. Michael Paulaitis for his helpful thoughts on the manuscript. KWW is funded by NIH (NIDA) DA040385 and MKH is supported by the American Heart Association [13GRNT16420015].
ORCID
Kenneth W. Witwer http://orcid.org/0000-0003-1664-4233
Marc K. Halushka http://orcid.org/0000-0002-7112-7389
References
- Gu X, Li JY, Guo J, Li PS, Zhang WH. Influence of MiR-451 on drug resistances of paclitaxel-resistant breast cancer cell line. Medical Sci Monitor 2015; 21:3291-7; PMID:26516138; http://dx.doi.org/10.12659/MSM.894475
- Su Z, Zhao J, Rong Z, Geng W, Wang Z. MiR-451, a potential prognostic biomarker and tumor suppressor for gastric cancer. International J Clin Exp Pathol 2015; 8:9154-60; PMID:26464660
- Liu X, Zhang X, Xiang J, Lv Y, Shi J. miR-451: potential role as tumor suppressor of human hepatoma cell growth and invasion. Int J Oncol 2014; 45:739-45; PMID:24841638; http://dx.doi.org/10.3892/ijo.2014.2446
- Cai SD, Chen JS, Xi ZW, Zhang LJ, Niu ML, Gao ZY. MicroRNA144 inhibits migration and proliferation in rectal cancer by downregulating ROCK1. Mol Med Reports 2015; 12:7396-402; PMID:26458302
- Haider BA, Baras AS, McCall MN, Hertel JA, Cornish TC, Halushka MK. A critical evaluation of microRNA biomarkers in non-neoplastic disease. PLoS One 2014; 9:e89565; PMID:24586876; http://dx.doi.org/10.1371/journal.pone.0089565
- Wang S, Aurora AB, Johnson BA, Qi X, McAnally J, Hill JA, Richardson JA, Bassel-Duby R, Olson EN. The endothelial-specific microRNA miR-126 governs vascular integrity and angiogenesis. Dev Cell 2008; 15:261-71; PMID:18694565; http://dx.doi.org/10.1016/j.devcel.2008.07.002
- McCall MN, Kent OA, Yu J, Fox-Talbot K, Zaiman AL, Halushka MK. microRNA profiling of diverse endothelial cell types. BMC Med Genomics 2011; 4:78; PMID:22047531; http://dx.doi.org/10.1186/1755-8794-4-78
- Civelek M, Hagopian R, Pan C, Che N, Yang WP, Kayne PS, Saleem NK, Cederberg H, Kuusisto J, Gargalovic PS, et al. Genetic regulation of human adipose microRNA expression and its consequences for metabolic traits. Hum Mol Genet 2013; 22:3023-37; PMID:23562819; http://dx.doi.org/10.1093/hmg/ddt159
- Feng R, Chen X, Yu Y, Su L, Yu B, Li J, Cai Q, Yan M, Liu B, Zhu Z. miR-126 functions as a tumour suppressor in human gastric cancer. Cancer Letters 2010; 298:50-63; PMID:20619534; http://dx.doi.org/10.1016/j.canlet.2010.06.004
- Ebrahimi F, Gopalan V, Wahab R, Lu CT, Smith RA, Lam AK. Deregulation of miR-126 expression in colorectal cancer pathogenesis and its clinical significance. Exp Cell Res 2015; 339:333-41; PMID:26455548; http://dx.doi.org/10.1016/j.yexcr.2015.10.004
- Yang Z, Wang R, Zhang T, Dong X. MicroRNA-126 regulates migration and invasion of gastric cancer by targeting CADM1. Int J Clin Exp Pathol 2015; 8:8869-80; PMID:26464628
- Yu L, Yang Y, Hou J, Zhai C, Song Y, Zhang Z, Qiu L, Jia X. MicroRNA-144 affects radiotherapy sensitivity by promoting proliferation, migration and invasion of breast cancer cells. Oncol Rep 2015; 34:1845-52; PMID:26252024; http://dx.doi.org/10.3892/or.2015.4173
- Zhang G, An H, Fang X. MicroRNA-144 regulates proliferation, invasion, and apoptosis of cells in malignant solitary pulmonary nodule via zinc finger E-box-binding homeobox 1. Int J Clin Exp Pathol 2015; 8:5960-7; PMID:26191328
- Xu Z, Ramachandran S, Gunasekaran M, Zhou F, Trulock E, Kreisel D, Hachem R, Mohanakumar T. MicroRNA-144 dysregulates the transforming growth factor-β signaling cascade and contributes to the development of bronchiolitis obliterans syndrome after human lung transplantation. J Heart Lung Transplantation 2015; 34:1154-62; PMID:25979625; http://dx.doi.org/10.1016/j.healun.2015.03.021
- Zhao M, Huang J, Gui K, Xiong M, Cai G, Xu J, Wang K, Liu D, Zhang X, Yin W. The downregulation of miR-144 is associated with the growth and invasion of osteosarcoma cells through the regulation of TAGLN expression. Int J Mol Med 2014; 34:1565-72; PMID:25318625; http://dx.doi.org/10.3892/ijmm.2014.1963
- Gits CM, van Kuijk PF, Jonkers MB, Boersma AW, Smid M, van Ijcken WF, Coindre JM, Chibon F, Verhoef C, Mathijssen RH, et al. microRNA expression profiles distinguish liposarcoma subtypes and implicate miR-145 and miR-451 as tumor suppressors. Int J Cancer 2014; 135:348-61; PMID:24375455; http://dx.doi.org/10.1002/ijc.28694
- Zhu H, Wu H, Liu X, Evans BR, Medina DJ, Liu CG, Yang JM. Role of microRNA miR-27a and miR-451 in the regulation of MDR1/P-glycoprotein expression in human cancer cells. Biochem Pharmacol 2008; 76:582-8; PMID:18619946; http://dx.doi.org/10.1016/j.bcp.2008.06.007
- Sun H, Cui C, Xiao F, Wang H, Xu J, Shi X, Yang Y, Zhang Q, Zheng X, Yang X, et al. miR-486 regulates metastasis and chemosensitivity in hepatocellular carcinoma by targeting CLDN10 and CITRON. Hepatol Res 2015; 45:1312-22; PMID:25655186; http://dx.doi.org/10.1111/hepr.12500
- Pang W, Tian X, Bai F, Han R, Wang J, Shen H, Zhang X, Liu Y, Yan X, Jiang F, et al. Pim-1 kinase is a target of miR-486-5p and eukaryotic translation initiation factor 4E, and plays a critical role in lung cancer. Mol Cancer 2014; 13:240; PMID:25342548; http://dx.doi.org/10.1186/1476-4598-13-240
- Kent OA, McCall MN, Cornish TC, Halushka MK. Lessons from miR-143/145: the importance of cell-type localization of miRNAs. Nucleic Acids Res 2014; 42:7528-38; PMID:24875473; http://dx.doi.org/10.1093/nar/gku461
- Pritchard CC, Kroh E, Wood B, Arroyo JD, Dougherty KJ, Miyaji MM, Tait JF, Tewari M. Blood cell origin of circulating microRNAs: a cautionary note for cancer biomarker studies. Cancer Prev Res (Phila) 2012; 5:492-7; PMID:22158052; http://dx.doi.org/10.1158/1940-6207.CAPR-11-0370
- Bian Z, Li L, Cui J, Zhang H, Liu Y, Zhang CY, Zen K. Role of miR-150-targeting c-Myb in colonic epithelial disruption during dextran sulphate sodium-induced murine experimental colitis and human ulcerative colitis. J Pathol 2011; 225:544-53; PMID:21590770; http://dx.doi.org/10.1002/path.2907
- Baras AS, Mitchell CJ, Myers JR, Gupta S, Weng LC, Ashton JM, Cornish TC, Pandey A, Halushka MK. miRge - A multiplexed method of processing small RNA-Seq data to determine microRNA entropy. PLoS One 2015; 10:e0143066; PMID:26571139; http://dx.doi.org/10.1371/journal.pone.0143066
- Vitsios DM, Enright AJ. Chimira: analysis of small RNA sequencing data and microRNA modifications. Bioinformatics 2015; 31:3365-7; PMID:26093149; http://dx.doi.org/10.1093/bioinformatics/btv380
- Raabe CA, Tang TH, Brosius J, Rozhdestvensky TS. Biases in small RNA deep sequencing data. Nucleic Acids Res 2014; 42:1414-26; PMID:24198247; http://dx.doi.org/10.1093/nar/gkt1021
- Fuchs RT, Sun Z, Zhuang F, Robb GB. Bias in ligation-based small RNA sequencing library construction is determined by adaptor and RNA structure. PLoS One 2015; 10:e0126049; PMID:25942392; http://dx.doi.org/10.1371/journal.pone.0126049
- Backes C, Sedaghat-Hamedani F, Frese K, Hart M, Ludwig N, Meder B, Meese E, Keller A. Bias in high-throughput analysis of miRNAs and implications for biomarker studies. Analy Chem 2016; 88:2088-95; PMID:26760198; http://dx.doi.org/10.1021/acs.analchem.5b03376
- Kent OA, Chivukula RR, Mullendore M, Wentzel EA, Feldmann G, Lee KH, Liu S, Leach SD, Maitra A, Mendell JT. Repression of the miR-143/145 cluster by oncogenic Ras initiates a tumor-promoting feed-forward pathway. Genes Dev 2010; 24:2754-9; PMID:21159816; http://dx.doi.org/10.1101/gad.1950610
- Chivukula RR, Shi G, Acharya A, Mills EW, Zeitels LR, Anandam JL, Abdelnaby AA, Balch GC, Mansour JC, Yopp AC, et al. An essential mesenchymal function for miR-143/145 in intestinal epithelial regeneration. Cell 2014; 157:1104-16; PMID:24855947; http://dx.doi.org/10.1016/j.cell.2014.03.055
- Akao Y, Nakagawa Y, Kitade Y, Kinoshita T, Naoe T. Downregulation of microRNAs-143 and −145 in B-cell malignancies. Cancer Sci 2007; 98:1914-20; PMID:17892514; http://dx.doi.org/10.1111/j.1349-7006.2007.00618.x
- Huang X, Yuan T, Tschannen M, Sun Z, Jacob H, Du M, Liang M, Dittmar RL, Liu Y, Liang M, et al. Characterization of human plasma-derived exosomal RNAs by deep sequencing. BMC Genomics 2013; 14:319; PMID:23663360; http://dx.doi.org/10.1186/1471-2164-14-319
- Baran-Gale J, Kurtz CL, Erdos MR, Sison C, Young A, Fannin EE, Chines PS, Sethupathy P. Addressing bias in small RNA library preparation for sequencing: A new protocol recovers MicroRNAs that evade capture by current methods. Front Genetics 2015; 6:352; PMID:26734062; http://dx.doi.org/10.3389/fgene.2015.00352
- Mestdagh P, Hartmann N, Baeriswyl L, Andreasen D, Bernard N, Chen C, Cheo D, D'Andrade P, DeMayo M, Dennis L, et al. Evaluation of quantitative miRNA expression platforms in the microRNA quality control (miRQC) study. Nat Methods 2014; 11:809-15; PMID:24973947; http://dx.doi.org/10.1038/nmeth.3014
- Locati MD, Terpstra I, de Leeuw WC, Kuzak M, Rauwerda H, Ensink WA, van Leeuwen S, Nehrdich U, Spaink HP, Jonker MJ, et al. Improving small RNA-seq by using a synthetic spike-in set for size-range quality control together with a set for data normalization. Nucleic Acids Res 2015; 43:e89; PMID:25870415; http://dx.doi.org/10.1093/nar/gkv303
- Mitchell PS, Parkin RK, Kroh EM, Fritz BR, Wyman SK, Pogosova-Agadjanyan EL, Peterson A, Noteboom J, O'Briant KC, Allen A, et al. Circulating microRNAs as stable blood-based markers for cancer detection. Proc Natl Acad Sci U S A 2008; 105:10513-8; PMID:18663219; http://dx.doi.org/10.1073/pnas.0804549105
- Corney DC, Flesken-Nikitin A, Godwin AK, Wang W, Nikitin AY. MicroRNA-34b and MicroRNA-34c are targets of p53 and cooperate in control of cell proliferation and adhesion-independent growth. Cancer Res 2007; 67:8433-8; PMID:17823410; http://dx.doi.org/10.1158/0008-5472.CAN-07-1585
- Peltier HJ, Latham GJ. Normalization of microRNA expression levels in quantitative RT-PCR assays: identification of suitable reference RNA targets in normal and cancerous human solid tissues. Rna 2008; 14:844-52; PMID:18375788; http://dx.doi.org/10.1261/rna.939908
- Popov A, Szabo A, Mandys V. Small nucleolar RNA U91 is a new internal control for accurate microRNAs quantification in pancreatic cancer. BMC Cancer 2015; 15:774; PMID:26499892; http://dx.doi.org/10.1186/s12885-015-1785-9
- Schwarzenbach H, da Silva AM, Calin G, Pantel K. Data normalization strategies for microRNA quantification. Clin Chem 2015; 61:1333-42; PMID:26408530; http://dx.doi.org/10.1373/clinchem.2015.239459
- Wang K, Yuan Y, Cho JH, McClarty S, Baxter D, Galas DJ. Comparing the microRNA spectrum between serum and plasma. PLoS One 2012; 7:e41561; PMID:22859996; http://dx.doi.org/10.1371/journal.pone.0041561
- Cheng HH, Yi HS, Kim Y, Kroh EM, Chien JW, Eaton KD, Goodman MT, Tait JF, Tewari M, Pritchard CC. Plasma processing conditions substantially influence circulating microRNA biomarker levels. PLoS One 2013; 8:e64795; PMID:23762257; http://dx.doi.org/10.1371/journal.pone.0064795
- Nair VS, Pritchard CC, Tewari M, Ioannidis JP. Design and analysis for studying microRNAs in human disease: A primer on -Omic technologies. Am J Epidemiol 2014; 180:140-52; PMID:24966218; http://dx.doi.org/10.1093/aje/kwu135
- Allen-Rhoades W, Kurenbekova L, Satterfield L, Parikh N, Fuja D, Shuck RL, Rainusso N, Trucco M, Barkauskas DA, Jo E, et al. Cross-species identification of a plasma microRNA signature for detection, therapeutic monitoring, and prognosis in osteosarcoma. Cancer Med 2015; 4:977-88; PMID:25784290; http://dx.doi.org/10.1002/cam4.438
- Krzeszinski JY, Wei W, Huynh H, Jin Z, Wang X, Chang TC, Xie XJ, He L, Mangala LS, Lopez-Berestein G, et al. miR-34a blocks osteoporosis and bone metastasis by inhibiting osteoclastogenesis and Tgif2. Nature 2014; 512:431-5; PMID:25043055; http://dx.doi.org/10.1038/nature13375
- Ribas J, Ni X, Haffner M, Wentzel EA, Salmasi AH, Chowdhury WH, Kudrolli TA, Yegnasubramanian S, Luo J, Rodriguez R, et al. miR-21: an androgen receptor-regulated microRNA that promotes hormone-dependent and hormone-independent prostate cancer growth. Cancer Res 2009; 69:7165-9; PMID:19738047; http://dx.doi.org/10.1158/0008-5472.CAN-09-1448
- van Rooij E, Sutherland LB, Thatcher JE, DiMaio JM, Naseem RH, Marshall WS, Hill JA, Olson EN. Dysregulation of microRNAs after myocardial infarction reveals a role of miR-29 in cardiac fibrosis. Proc Natl Acad Sci U S A 2008; 105:13027-32; PMID:18723672; http://dx.doi.org/10.1073/pnas.0805038105
- Kuppusamy KT, Jones DC, Sperber H, Madan A, Fischer KA, Rodriguez ML, Pabon L, Zhu WZ, Tulloch NL, Yang X, et al. Let-7 family of microRNA is required for maturation and adult-like metabolism in stem cell-derived cardiomyocytes. Proc Natl Acad Sci U S A 2015; 112:E2785-94; PMID:25964336; http://dx.doi.org/10.1073/pnas.1424042112
- Wang WT, Zhao YN, Han BW, Hong SJ, Chen YQ. Circulating microRNAs identified in a genome-wide serum microRNA expression analysis as noninvasive biomarkers for endometriosis. J Clin Endocrinol Metab 2013; 98:281-9; PMID:23118427; http://dx.doi.org/10.1210/jc.2012-2415
- Chang JR, Mukerjee R, Bagashev A, Del Valle L, Chabrashvili T, Hawkins BJ, He JJ, Sawaya BE. HIV-1 Tat protein promotes neuronal dysfunction through disruption of microRNAs. J Biol Chem 2011; 286:41125-34; PMID:21956116; http://dx.doi.org/10.1074/jbc.M111.268466
- Mukerjee R, Chang JR, Del Valle L, Bagashev A, Gayed MM, Lyde RB, Hawkins BJ, Brailoiu E, Cohen E, Power C, et al. Deregulation of microRNAs by HIV-1 Vpr protein leads to the development of neurocognitive disorders. J Biol Chem 2011; 286:34976-85; PMID:21816823; http://dx.doi.org/10.1074/jbc.M111.241547
- Mullokandov G, Baccarini A, Ruzo A, Jayaprakash AD, Tung N, Israelow B, Evans MJ, Sachidanandam R, Brown BD. High-throughput assessment of microRNA activity and function using microRNA sensor and decoy libraries. Nat Methods 2012; 9:840-6; PMID:22751203; http://dx.doi.org/10.1038/nmeth.2078
- Jin HY, Gonzalez-Martin A, Miletic AV, Lai M, Knight S, Sabouri-Ghomi M, Head SR, Macauley MS, Rickert RC, Xiao C. Transfection of microRNA mimics should be used with caution. Front Genetics 2015; 6:340; PMID:26697058; http://dx.doi.org/10.3389/fgene.2015.00340
- Thomson DW, Bracken CP, Szubert JM, Goodall GJ. On measuring miRNAs after transient transfection of mimics or antisense inhibitors. PLoS One 2013; 8:e55214; PMID:23358900; http://dx.doi.org/10.1371/journal.pone.0055214
- Goldgraben MA, Russell R, Rueda OM, Caldas C, Git A. Double-stranded microRNA mimics can induce length- and passenger strand-dependent effects in a cell type-specific manner. RNA 2016; 22:193-203; PMID:26670622; http://dx.doi.org/10.1261/rna.054072.115
- Valadi H, Ekstrom K, Bossios A, Sjostrand M, Lee JJ, Lotvall JO. Exosome-mediated transfer of mRNAs and microRNAs is a novel mechanism of genetic exchange between cells. Nat Cell Biol 2007; 9:654-9; PMID:17486113; http://dx.doi.org/10.1038/ncb1596
- Das S, Halushka MK. Extracellular vesicle microRNA transfer in cardiovascular disease. Cardiovasc Pathol 2015; 24:199-206; PMID:25958013; http://dx.doi.org/10.1016/j.carpath.2015.04.007
- Tosar JP, Gambaro F, Sanguinetti J, Bonilla B, Witwer KW, Cayota A. Assessment of small RNA sorting into different extracellular fractions revealed by high-throughput sequencing of breast cell lines. Nucleic Acids Res 2015; 43:5601-16; PMID:25940616; http://dx.doi.org/10.1093/nar/gkv432
- Janas T, Janas MM, Sapon K, Janas T. Mechanisms of RNA loading into exosomes. FEBS Letters 2015; 589:1391-8; PMID:25937124; http://dx.doi.org/10.1016/j.febslet.2015.04.036
- Chevillet JR, Kang Q, Ruf IK, Briggs HA, Vojtech LN, Hughes SM, Cheng HH, Arroyo JD, Meredith EK, Gallichotte EN, et al. Quantitative and stoichiometric analysis of the microRNA content of exosomes. Proc Natl Acad Sci U S A 2014; 111:14888-93; PMID:25267620; http://dx.doi.org/10.1073/pnas.1408301111
- Sverdlov ED. Amedeo Avogadro's cry: what is 1 microg of exosomes? BioEssays 2012; 34:873-5; PMID:22815202; http://dx.doi.org/10.1002/bies.201200045
- Bianconi E, Piovesan A, Facchin F, Beraudi A, Casadei R, Frabetti F, Vitale L, Pelleri MC, Tassani S, Piva F, et al. An estimation of the number of cells in the human body. Annals Hum Biol 2013; 40:463-71; PMID:23829164; http://dx.doi.org/10.3109/03014460.2013.807878
- Sender R, Fuchs S, Milo R. Are we really vastly outnumbered? Revisiting the ratio of bacterial to host cells in humans. Cell 2016; 164:337-40; PMID:26824647; http://dx.doi.org/10.1016/j.cell.2016.01.013
- van Dongen HM, Masoumi N, Witwer KW, Pegtel DM. Extracellular vesicles exploit viral entry routes for cargo delivery. Microbiol Mol Biol Rev 2016; 80:369-86; PMID:26935137; http://dx.doi.org/10.1128/MMBR.00063-15
- Tkach M, Thery C. Communication by extracellular vesicles: Where we are and where we need to go. Cell 2016; 164:1226-32; PMID:26967288; http://dx.doi.org/10.1016/j.cell.2016.01.043
- Mayya VK, Duchaine TF. On the availability of microRNA-induced silencing complexes, saturation of microRNA-binding sites and stoichiometry. Nucleic Acids Res 2015; 43:7556-65; PMID:26227970; http://dx.doi.org/10.1093/nar/gkv720
- Seitz H. Redefining microRNA targets. Curr Biol 2009; 19:870-3; PMID:19375315; http://dx.doi.org/10.1016/j.cub.2009.03.059
- Salmena L, Poliseno L, Tay Y, Kats L, Pandolfi PP. A ceRNA hypothesis: the Rosetta Stone of a hidden RNA language? Cell 2011; 146:353-8; PMID:21802130; http://dx.doi.org/10.1016/j.cell.2011.07.014
- Helwak A, Kudla G, Dudnakova T, Tollervey D. Mapping the human miRNA interactome by CLASH reveals frequent noncanonical binding. Cell 2013; 153:654-65; PMID:23622248; http://dx.doi.org/10.1016/j.cell.2013.03.043
- Denzler R, Agarwal V, Stefano J, Bartel DP, Stoffel M. Assessing the ceRNA hypothesis with quantitative measurements of miRNA and target abundance. Mol Cell 2014; 54:766-76; PMID:24793693; http://dx.doi.org/10.1016/j.molcel.2014.03.045
- Helwak A, Tollervey D. Mapping the miRNA interactome by cross-linking ligation and sequencing of hybrids (CLASH). Nature Protocols 2014; 9:711-28; PMID:24577361; http://dx.doi.org/10.1038/nprot.2014.043
- Franks TM, Lykke-Andersen J. The control of mRNA decapping and P-body formation. Mol Cell 2008; 32:605-15; PMID:19061636; http://dx.doi.org/10.1016/j.molcel.2008.11.001
- Schratt G. microRNAs at the synapse. Nat Rev Neurosci 2009; 10:842-9; PMID:19888283; http://dx.doi.org/10.1038/nrn2763
- Olejniczak SH, La Rocca G, Gruber JJ, Thompson CB. Long-lived microRNA-Argonaute complexes in quiescent cells can be activated to regulate mitogenic responses. Proc Natl Acad Sci U S A 2013; 110:157-62; PMID:23248281; http://dx.doi.org/10.1073/pnas.1219958110
- La Rocca G, Olejniczak SH, Gonzalez AJ, Briskin D, Vidigal JA, Spraggon L, DeMatteo RG, Radler MR, Lindsten T, Ventura A, et al. In vivo, Argonaute-bound microRNAs exist predominantly in a reservoir of low molecular weight complexes not associated with mRNA. Proc Natl Acad Sci U S A 2015; 112:767-72; PMID:25568082; http://dx.doi.org/10.1073/pnas.1424217112
- John B, Enright AJ, Aravin A, Tuschl T, Sander C, Marks DS. Human MicroRNA targets. PLoS Biol 2004; 2:e363; PMID:15502875; http://dx.doi.org/10.1371/journal.pbio.0020363
- Agarwal V, Bell GW, Nam JW, Bartel DP. Predicting effective microRNA target sites in mammalian mRNAs. Elife 2015; 4:e05005; PMID:26267216; http://dx.doi.org/10.7554/eLife.05005
- Loher P, Rigoutsos I. Interactive exploration of RNA22 microRNA target predictions. Bioinformatics 2012; 28:3322-3; PMID:23074262; http://dx.doi.org/10.1093/bioinformatics/bts615
- Kruger J, Rehmsmeier M. RNAhybrid: microRNA target prediction easy, fast and flexible. Nucleic Acids Res 2006; 34:W451-4; PMID:16845047; http://dx.doi.org/10.1093/nar/gkl243
- Ritchie W, Flamant S, Rasko JE. Predicting microRNA targets and functions: traps for the unwary. Nat Methods 2009; 6:397-8; PMID:19478799; http://dx.doi.org/10.1038/nmeth0609-397
- Lee YJ, Kim V, Muth DC, Witwer KW. Validated MicroRNA target databases: An evaluation. Drug Dev Res 2015; 76:389-96; PMID:26286669; http://dx.doi.org/10.1002/ddr.21278
- Vlachos IS, Paraskevopoulou MD, Karagkouni D, Georgakilas G, Vergoulis T, Kanellos I, Anastasopoulos IL, Maniou S, Karathanou K, Kalfakakou D, et al. DIANA-TarBase v7.0: indexing more than half a million experimentally supported miRNA:mRNA interactions. Nucleic Acids Res 2015; 43:D153-9; PMID:25416803; http://dx.doi.org/10.1093/nar/gku1215
- Huang J, Wang F, Argyris E, Chen K, Liang Z, Tian H, Huang W, Squires K, Verlinghieri G, Zhang H. Cellular microRNAs contribute to HIV-1 latency in resting primary CD4+ T lymphocytes. Nat Med 2007; 13:1241-7; PMID:17906637; http://dx.doi.org/10.1038/nm1639
- Mantri CK, Pandhare Dash J, Mantri JV, Dash CC. Cocaine enhances HIV-1 replication in CD4+ T cells by down-regulating MiR-125b. PLoS One 2012; 7:e51387; PMID:23251514; http://dx.doi.org/10.1371/journal.pone.0051387
- Andersen CL, Jensen JL, Orntoft TF. Normalization of real-time quantitative reverse transcription-PCR data: a model-based variance estimation approach to identify genes suited for normalization, applied to bladder and colon cancer data sets. Cancer Res 2004; 64:5245-50; PMID:15289330; http://dx.doi.org/10.1158/0008-5472.CAN-04-0496
- Chin AR, Fong MY, Somlo G, Wu J, Swiderski P, Wu X, Wang SE. Cross-kingdom inhibition of breast cancer growth by plant miR159. Cell Res 2016; 26:217-28; PMID:26794868; http://dx.doi.org/10.1038/cr.2016.13
- Timmons L, Fire A. Specific interference by ingested dsRNA. Nature 1998; 395:854; PMID:9804418; http://dx.doi.org/10.1038/27579
- Winston WM, Sutherlin M, Wright AJ, Feinberg EH, Hunter CP. Caenorhabditis elegans SID-2 is required for environmental RNA interference. Proc Natl Acad Sci U S A 2007; 104:10565-70; PMID:17563372; http://dx.doi.org/10.1073/pnas.0611282104
- Feinberg EH, Hunter CP. Transport of dsRNA into cells by the transmembrane protein SID-1. Science 2003; 301:1545-7; PMID:12970568; http://dx.doi.org/10.1126/science.1087117
- Witwer KW, Hirschi KD. Transfer and functional consequences of dietary microRNAs in vertebrates: concepts in search of corroboration: negative results challenge the hypothesis that dietary xenomiRs cross the gut and regulate genes in ingesting vertebrates, but important questions persist. BioEssays 2014; 36:394-406; PMID:24436255; http://dx.doi.org/10.1002/bies.201300150
- Pak J, Fire A. Distinct populations of primary and secondary effectors during RNAi in C. elegans. Science 2007; 315:241-4; PMID:17124291; http://dx.doi.org/10.1126/science.1132839
- Zhang L, Hou D, Chen X, Li D, Zhu L, Zhang Y, Li J, Bian Z, Liang X, Cai X, et al. Exogenous plant MIR168a specifically targets mammalian LDLRAP1: evidence of cross-kingdom regulation by microRNA. Cell Res 2012; 22:107-26; PMID:21931358; http://dx.doi.org/10.1038/cr.2011.158
- Zhang Y, Wiggins BE, Lawrence C, Petrick J, Ivashuta S, Heck G. Analysis of plant-derived miRNAs in animal small RNA data sets. BMC Genomics 2012; 13:381; PMID:22873950; http://dx.doi.org/10.1186/1471-2164-13-381
- Tosar JP, Rovira C, Naya H, Cayota A. Mining of public sequencing databases supports a non-dietary origin for putative foreign miRNAs: underestimated effects of contamination in NGS. RNA 2014; 20:754-7; PMID:24729469; http://dx.doi.org/10.1261/rna.044263.114
- Witwer KW. XenomiRs and miRNA homeostasis in health and disease: evidence that diet and dietary miRNAs directly and indirectly influence circulating miRNA profiles. RNA Biol 2012; 9:1147-54; PMID:22951590; http://dx.doi.org/10.4161/rna.21619
- Dickinson B, Zhang Y, Petrick JS, Heck G, Ivashuta S, Marshall WS. Lack of detectable oral bioavailability of plant microRNAs after feeding in mice. Nat Biotechnol 2013; 31:965-7; PMID:24213763; http://dx.doi.org/10.1038/nbt.2737
- Snow JW, Hale AE, Isaacs SK, Baggish AL, Chan SY. Ineffective delivery of diet-derived microRNAs to recipient animal organisms. RNA Biol 2013; 10:1107-16; PMID:23669076; http://dx.doi.org/10.4161/rna.24909
- Witwer KW, McAlexander MA, Queen SE, Adams RJ. Real-time quantitative PCR and droplet digital PCR for plant miRNAs in mammalian blood provide little evidence for general uptake of dietary miRNAs: limited evidence for general uptake of dietary plant xenomiRs. RNA Biol 2013; 10:1080-6; PMID:23770773; http://dx.doi.org/10.4161/rna.25246
- Receptive to replication. Nat Biotechnol 2013; 31:943; PMID:24213747; http://dx.doi.org/10.1038/nbt.2748
- Liang H, Zhang S, Fu Z, Wang Y, Wang N, Liu Y, Zhao C, Wu J, Hu Y, Zhang J, et al. Effective detection and quantification of dietetically absorbed plant microRNAs in human plasma. J Nutri Biochem 2015; 26:505-12; PMID:25704478; http://dx.doi.org/10.1016/j.jnutbio.2014.12.002
- Witwer KW. Contamination or artifacts may explain reports of plant miRNAs in humans. J Nutri Biochem 2015; 26:1685; PMID:26456566; http://dx.doi.org/10.1016/j.jnutbio.2015.09.004
- Laubier J, Castille J, Le Guillou S, Le Provost F. No effect of an elevated miR-30b level in mouse milk on its level in pup tissues. RNA Biol 2015; 12:26-9; PMID:25763824; http://dx.doi.org/10.1080/15476286.2015.1017212
- Title AC, Denzler R, Stoffel M. Uptake and function studies of maternal Milk-derived MicroRNAs. J Biol Chem 2015; 290:23680-91; PMID:26240150; http://dx.doi.org/10.1074/jbc.M115.676734
- Baier SR, Nguyen C, Xie F, Wood JR, Zempleni J. MicroRNAs are absorbed in biologically meaningful amounts from nutritionally relevant doses of cow milk and affect gene expression in peripheral blood mononuclear cells, HEK-293 kidney cell cultures, and mouse livers. J Nutri 2014; 144:1495-500; PMID:25122645; http://dx.doi.org/10.3945/jn.114.196436
- Auerbach A, Vyas G, Li A, Halushka M, Witwer KW. Uptake of dietary milk miRNAs by adult humans: a validation study. F1000 Res 2016; 5:721; PMID:27158459; http://dx.doi.org/10.12688/f1000research.8548.1
- Tavazoie SF, Alarcon C, Oskarsson T, Padua D, Wang Q, Bos PD, Gerald WL, Massague J. Endogenous human microRNAs that suppress breast cancer metastasis. Nature 2008; 451:147-52; PMID:18185580; http://dx.doi.org/10.1038/nature06487
- McCarthy JJ. MicroRNA-206: the skeletal muscle-specific myomiR. Biochim Et Biophys Acta 2008; 1779:682-91; PMID:18381085; http://dx.doi.org/10.1016/j.bbagrm.2008.03.001
- Zhang J, Du YY, Lin YF, Chen YT, Yang L, Wang HJ, Ma D. The cell growth suppressor, mir-126, targets IRS-1. Biochem Biophys Res Commun 2008; 377:136-40; PMID:18834857; http://dx.doi.org/10.1016/j.bbrc.2008.09.089
- Huang JY, Zhang K, Chen DQ, Chen J, Feng B, Song H, Chen Y, Zhu Z, Lu L, De W, et al. MicroRNA-451: epithelial-mesenchymal transition inhibitor and prognostic biomarker of hepatocelluar carcinoma. Oncotarget 2015; 6:18613-30; PMID:26164082; http://dx.doi.org/10.18632/oncotarget.4317
- Li HP, Zeng XC, Zhang B, Long JT, Zhou B, Tan GS, Zeng WX, Chen W, Yang JY. miR-451 inhibits cell proliferation in human hepatocellular carcinoma through direct suppression of IKK-β. Carcinogenesis 2013; 34:2443-51; PMID:23740840; http://dx.doi.org/10.1093/carcin/bgt206
- Zang WQ, Yang X, Wang T, Wang YY, Du YW, Chen XN, Li M, Zhao GQ. MiR-451 inhibits proliferation of esophageal carcinoma cell line EC9706 by targeting CDKN2D and MAP3K1. World J Gastroenterol 2015; 21:5867-76; PMID:26019450
- Li Z, Wu S, Lv S, Wang H, Wang Y, Guo Q. Suppression of liver receptor homolog-1 by microRNA-451 represses the proliferation of osteosarcoma cells. Biochem Biophys Res Commun 2015; 461:450-5; PMID:25869073; http://dx.doi.org/10.1016/j.bbrc.2015.04.013
- Zhang F, Huang W, Sheng M, Liu T. MiR-451 inhibits cell growth and invasion by targeting CXCL16 and is associated with prognosis of osteosarcoma patients. Tumour Biol 2015; 36:2041-8; PMID:25391425; http://dx.doi.org/10.1007/s13277-014-2811-2
- Kuwabara Y, Horie T, Baba O, Watanabe S, Nishiga M, Usami S, Izuhara M, Nakao T, Nishino T, Otsu K, et al. MicroRNA-451 exacerbates lipotoxicity in cardiac myocytes and high-fat diet-induced cardiac hypertrophy in mice through suppression of the LKB1/AMPK pathway. Circ Res 2015; 116:279-88; PMID:25362209; http://dx.doi.org/10.1161/CIRCRESAHA.116.304707
- Yin P, Peng R, Peng H, Yao L, Sun Y, Wen L, Wu T, Zhou J, Zhang Z. MiR-451 suppresses cell proliferation and metastasis in A549 lung cancer cells. Mol Biotechnol 2015; 57:1-11; PMID:25150396; http://dx.doi.org/10.1007/s12033-014-9796-3
- Grant JS, Morecroft I, Dempsie Y, van Rooij E, MacLean MR, Baker AH. Transient but not genetic loss of miR-451 is protective in the development of pulmonary arterial hypertension. Pulmonary Circulation 2013; 3:840-50; PMID:25006399; http://dx.doi.org/10.1086/674751
- Liu D, Liu C, Wang X, Ingvarsson S, Chen H. MicroRNA-451 suppresses tumor cell growth by down-regulating IL6R gene expression. Cancer Epidemiol 2014; 38:85-92; PMID:24445140; http://dx.doi.org/10.1016/j.canep.2013.12.005
- Li HY, Zhang Y, Cai JH, Bian HL. MicroRNA-451 inhibits growth of human colorectal carcinoma cells via downregulation of Pi3k/Akt pathway. Asian Pacific J Cancer Prevention 2013; 14:3631-4; PMID:23886157; http://dx.doi.org/10.7314/APJCP.2013.14.6.3631
- Liu F, Wang J, Fu Q, Zhang X, Wang Y, Liu J, Huang J, Lv X. VEGF-activated miR-144 regulates autophagic survival of prostate cancer cells against Cisplatin. Tumour Biol 2015; PMID:26566625; http://dx.doi.org/10.1007/s13277-015-4383-1
- Pan HL, Wen ZS, Huang YC, Cheng X, Wang GZ, Zhou YC, Wang ZY, Guo YQ, Cao Y, Zhou GB. Down-regulation of microRNA-144 in air pollution-related lung cancer. Scientific Reports 2015; 5:14331; PMID:26395400; http://dx.doi.org/10.1038/srep14331
- Gao F, Wang T, Zhang Z, Wang R, Guo Y, Liu J. Regulation of activating protein-4-associated metastases of non-small cell lung cancer cells by miR-144. Tumour Biol 2015; PMID:26254097; http://dx.doi.org/10.1007/s13277-015-3866-4
- Lan F, Yu H, Hu M, Xia T, Yue X. miR-144-3p exerts anti-tumor effects in glioblastoma by targeting c-Met. J Neurochem 2015; 135:274-86; PMID:26250785; http://dx.doi.org/10.1111/jnc.13272
- Matsushita R, Seki N, Chiyomaru T, Inoguchi S, Ishihara T, Goto Y, Nishikawa R, Mataki H, Tatarano S, Itesako T, et al. Tumour-suppressive microRNA-144-5p directly targets CCNE1/2 as potential prognostic markers in bladder cancer. Br J Cancer 2015; 113:282-9; PMID:26057453; http://dx.doi.org/10.1038/bjc.2015.195
- Wang W, Zhou X, Wei M. MicroRNA-144 suppresses osteosarcoma growth and metastasis by targeting ROCK1 and ROCK2. Oncotarget 2015; 6:10297-308; PMID:25912304; http://dx.doi.org/10.18632/oncotarget.3305
- Lin L, Zheng Y, Tu Y, Wang Z, Liu H, Lu X, Xu L, Yuan J. MicroRNA-144 suppresses tumorigenesis and tumor progression of astrocytoma by targeting EZH2. Hum Pathol 2015; 46:971-80; PMID:25907866; http://dx.doi.org/10.1016/j.humpath.2015.01.023
- Chen S, Li P, Li J, Wang Y, Du Y, Chen X, Zang W, Wang H, Chu H, Zhao G, et al. MiR-144 inhibits proliferation and induces apoptosis and autophagy in lung cancer cells by targeting TIGAR. Cell Physiol Biochem 2015; 35:997-1007; PMID:25660220; http://dx.doi.org/10.1159/000369755