ABSTRACT
MiRNAs play a central role in physiological and pathological processes. Both for the biological understanding and for their clinical application, it is essential to understand the interaction of miRNAs and their targets. Target identification largely hinges on in-silico prediction, which requires a complete consideration of miRNA binding sites within the UTRs of target genes. Here, we show that 5-mer sites might also play an essential role for human miRNA-target binding. We implemented and employed an algorithm to all pairs of 2,588 human miRNAs annotated in miRBase and the 3′ UTRs of 16725 genes (>43 million combinations). Our in-silico analysis showed a highly significant enrichment (p = 1.4 × 10−69) of 5-mer binding sites in 3′ UTRs across all experimentally validated miRNA-target gene pairs. We next confirmed the central role of 5-mer binding sites by reporter assays and demonstrated that two non-canonical 5-mer sites of miR-34a in the 3′ UTR of T-cell receptor alpha (TCRA) have a significantly stronger influence on its posttranscriptional regulation than the canonical binding sites. These observations indicate an essential role of 5-mer binding sites for the miRNA targeting in human cells.
Introduction
Since the onset of molecular genetics, a major research focus was on the mechanisms underlying regulation of transcription and translation and their alterations of these processes in human diseases [Citation1]. The discovery of micro(mi)RNAs by Lee et al. in Caenorhabditis elegans in 1993 has opened the view for a new layer of regulatory molecules [Citation2]. The crucial role of miRNAs in posttranscriptional regulation processes has been recognized not least by the analysis of several key miRNAs including the let-7 family and their role in regulating genes during developmental processes. There is, however, an insufficient understanding on how exactly small non-coding RNAs orchestrate the human transcriptome.
Since their first description, numerous miRNAs have been identified by various approaches including complementary metal-oxide-semiconductor (CMOS) based assays [Citation3] or immunoassays [Citation4], RT-qPCR and on a larger scale by microarray assays and high-throughput sequencing. An overview of the different experimental approaches used for miRNA identification and verification is given by Mestdagh et al [Citation5]. The various approaches have led to 28,645 miRNAs including 2,588 human mature miRNAs that are presently deposited in miRNA Database miRBase (Release 21, http://www.mirbase.org) [Citation6].
MiRNAs play not only a central role in physiological processes like cellular signaling, cell migration, and apoptosis but also in numerous pathological processes. Altered miRNA expression profiles have for example been reported for most cancer tissues including the most lethal cancer types like lung cancer [Citation7], breast cancer [Citation8], and brain cancer [Citation9]. Besides the cancer tissues, altered miRNA profiles occur also in whole peripheral blood of patients with rather different diseases like myocardial infarction [Citation10], lung cancer [Citation11], multiple sclerosis [Citation12,Citation13], melanoma [Citation14], ovarian cancer [Citation15], chronic obstructive pulmonary disease [Citation16], glioblastoma [Citation17], and Alzheimer disease [Citation18]. In a comparative study on 1,368 patients including 1,049 genome-wide miRNA profiles we identified 34 blood born miRNAs dysregulated in human pathologies [Citation19]. Despite the large number of miRNAs associated with diseases by basic research studies, there are a rather limited number of clinical trials focusing on microRNAs (ClinicalTrials.gov).
Both for the biological understanding of miRNAs and for their clinical application towards a better prognosis, monitoring and diagnosis, it is essential to understand the interaction of miRNAs and their target transcripts. In general, the repression of protein translation by miRNAs is mediated by binding of a miRNA to a target sequence termed seed region, which is mostly localized within the 3′ UTR of mRNA sequences. Besides targeting of 3′ UTRs, evidence exists that miRNAs may also bind within the 5′ UTR or the ORF of a transcript [Citation20]. The recording of species specific targetomes including the human targetome, is far from being completed, and represents a major challenge in RNA biology. While miRNA:mRNA duplexes are largely paired in a perfect manner in plants, the prediction of the target regions is especially demanding in animals due to the possibility of an imperfect complementarity between miRNA and mRNA [Citation21,Citation22]. In addressing these challenges, a number of methods have been developed to identify miRNA targets in animals. These tools encompass applications of machine learning models, artificial neural networks, and Bayesian learning using different input features. These features include expression values of miRNA and target transcripts, accessibility of target mRNAs, and minimum free energy of a predicted duplex [Citation23–Citation25] to infer tentative miRNA-target pairs [Citation26,Citation27]. In addition, targetomes of individual miRNAs are well suited for simple interaction analyses based on graph and network methods as implemented in miRTargetLink [Citation28] (https://ccb-web.cs.uni-saarland.de/mirtargetlink/).
The most common approach in target prediction analysis is based on miRNA seed sequences (nucleotides 2–7 of a miRNA) and their complementary canonical binding sites [Citation29]. As the study of Bartel et al. demonstrates, the regulatory potential of different kinds of binding sites (8-mer to 6-mer) results in a hierarchy of site efficacy: 8-mer >> 7-mer-m8 > 7-mer-A1 >> 6-mer > no site [Citation29]. With regards to shorter k-mer sites, 5-mer sites have been suggested to regulate target genes in Drosophila if compensatory 3′ pairing between a miRNA and its target is observed [Citation30].
In our previous studies, we highlighted the central role of miR-34a in T cell receptor signaling via regulation of five PKC family members [Citation31]. MiR-34a is dys-regulated in numerous tumors types like cancers of brain, lung, pancreas, colon, esophagus, and liver [Citation32–Citation38]. As a tumor suppressor miRNA, miR-34a regulates a variety of oncogenic targets genes, for example MET proto-oncogene (MET), sirtuin 1 (SIRT1), Notch-1 and CD44 molecule (CD44) [Citation39–Citation43]. Elevated miR-34a expression levels were reported in serum, plasma and whole blood samples of cancer patients [Citation44–Citation46]. Recently, we identified miR-34a as most up-regulated miRNA in CD3+ T cells of lung cancer patients targeting five protein kinase C family members including PRKCA, PRKCB, PRKCE, PRKCH and PRKCQ [Citation31]. Besides the central role of these PKC isozymes in carcinogenesis, these miR-34a target genes are involved in cell signaling through the immunological synapse (IS) of T cells downstream of the T cell receptor (TCR) [Citation47–Citation51].
Here, we analyzed the binding of miR-34a within the TCRA gene by in-silico prediction and experimental validation. To this end, we applied an in-silico k-mer count based approach and dual luciferase assays to show that 5-mer binding sites, without extensive pairing at the 3′ end of a miRNA, can also play a crucial role in posttranscriptional regulation of target genes in human.
Results and discussion
Canonical sites are not sufficient to down-regulate TCRA
Within the TCRA-3′ UTR two canonical binding sites with a length of six and seven nucleotides were identified by in-silico prediction. To validate the binding and repression of TCRA by miR-34a, we amplified nucleotides 96–497 of the TCRA 3′ UTR. The amplified sequence was cloned into the pMIR-RNL-TK reporter vector, transfected in HEK 293T cells together with the miR-34a expression plasmid, and used in a dual luciferase reporter assay. To verify the effect of miR-34a binding to its target sites in the TCRA 3′ UTR, each of the binding site was mutated separately and in combination. In these experiments, the luciferase activity was however not significantly increased for TCRA reporter plasmids that contained single mutated canonical binding sites compared to the not mutated functional sites. When both canonical binding sites were simultaneously mutated, the luciferase activity was slightly elevated. Nevertheless, the luciferase activity of the latter experiment was still being far lower than the activity of the control experiments and far beyond everything we would consider as an effective binding. Since the repression of TCRA by miR-34a seems to be central according to our systems biology analysis but the experiments modifying the canonical binding sites only showed minor effects, we performed a very detailed computational analysis of the seed region of miR-34a and the TCRA 3′ UTR. As additional factor besides the two previously known canonical binding sites, we identified two 5-mers in the 3′ UTR that could potentially bind the miR-34a seed ().
Figure 1. Schematic diagram of the reporter gene vector of TCRA. The location of the predicted binding sites and the non-canonical 5-mer binding sites of miR-34a-5p in the TCRA 3′ UTR are shown. Additionally, the diagram displays the sequences of the predicted binding sites and the non-canonical 5-mer binding sites of miR-34a-5p as well as the mutated binding sites (underlined letters).
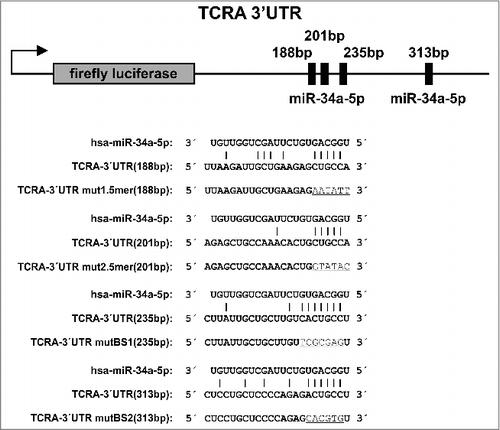
Enrichment of 5-mer sites in experimentally validated miRNA target gene pairs
We asked whether the 5-mer sites are specific for the 3′ UTR of TCRA and miR-34a or whether other validated miRNA gene pairs show similar patterns. To analyze the role of 5-mer binding sites in regulating the translation of target genes systematically, we performed a binding site enrichment analysis. In detail, we utilized all human miRNAs from miRBase v21 [Citation6] to extract the contiguous 5-, 6-, 7-, and 8-mers sequences as detailed in Material and Methods. Next, we extracted human 3′ UTR regions annotated in Ensembl (http://www.ensembl.org) 52. We then computed the number of mapped 5-, 6-, 7- and 8-mers for each possible miRNA – gene pair and intersected each of these pairs with the validated human interactions deposited in miRTarBase (http://mirtarbase.mbc.nctu.edu.tw), TarBase (http://diana.imis.athena-innovation.gr/), miRecords (http://c1.accurascience.com/miRecords/) and a large CLASH data set [Citation53–Citation56]. In total, there were 57 million combinations of pairs between the 2,588 human miRNAs and the UTRs of 16,725 human genes. The bar plot in presents the average count ± standard deviation for the number of k-mer (k from 5 to 8) sites in miRNA-gene pairs that are not known to be targets, those that are considered as weak evidence targets (identified e.g. by microarray experiments) and those that are considered as strong evidence targets (identified e.g. by reporter assays). For all k-mers, we observed an increased count from not-known targets over weak-evidence to strong-evidence targets. The enrichment of 5-mer sites in validated targets using experimental techniques with strong evidence was highly significant (p = 1.4 × 10−69). These results suggest that 5-mer target sites are present in 3′ UTRs of target transcripts and may have a more important role for regulating gene expression than previously assumed.
Figure 2. Number of interactions using different seed lengths and validation groups. Shown is the mean ± standard deviation of the matching miRNA-target transcript counts for each of the different k-mers (k from 5 to 8) as seed. For each k-mer length, the observed interactions are grouped, from left to right, into interactions for which no validation was known, “weak targets” identified by e.g. microarray analysis, and “strong targets” identified by e.g. luciferase reporter assays according to miRTarBase v7, TarBase v6, miRecords v4, and a large CLASH dataset [Citation52-Citation55]. The significant p-value (p = 1.4 × 10−69) between the mean of the observations in the blue (no validation) and yellow (strong evidence) group for 5-mers supports our conclusions that these small non-canonical binding sites might have a biological function in human.
![Figure 2. Number of interactions using different seed lengths and validation groups. Shown is the mean ± standard deviation of the matching miRNA-target transcript counts for each of the different k-mers (k from 5 to 8) as seed. For each k-mer length, the observed interactions are grouped, from left to right, into interactions for which no validation was known, “weak targets” identified by e.g. microarray analysis, and “strong targets” identified by e.g. luciferase reporter assays according to miRTarBase v7, TarBase v6, miRecords v4, and a large CLASH dataset [Citation52-Citation55]. The significant p-value (p = 1.4 × 10−69) between the mean of the observations in the blue (no validation) and yellow (strong evidence) group for 5-mers supports our conclusions that these small non-canonical binding sites might have a biological function in human.](/cms/asset/7ebe12fa-3e70-4f2f-9c85-98973759da6b/krnb_a_1462652_f0002_oc.jpg)
Experimental validation of the effects of 5-mer sites in TCRA
To experimentally confirm the role of 5-mer binding sites, we performed reporter assay experiments. To understand the influence of single sites and cooperative effects we had to carry out experiments for all of the 16 possible combinations (no site mutated, 4 single sites mutated, 6 combinations of two sites mutated, 4 combinations of three sites mutated, all 4 sites mutated,). lists all combinations of mutations in the 3′ UTR ordered by increasing effect sizes according to altered binding capacities. Surprisingly, the effect of both single mutated 5-mer binding site was already stronger as compared to the single- and even to the combined effect of the mutated canonical binding sites. The same strong biological effect of the 5-mer binding sites was also evident in the analysis of two simultaneously mutated 5-mer binding sites, showing significantly higher effects as the single 5-mer, the single canonical and the combined mutation of the two canonical sites. Consistent with this observation are the results of experiments with three mutated binding sites and one intact binding site. In each of these assays, we observed the strongest effects on the luciferase activity for assays that contained two mutated 5-mer binding sites among the three mutated sites. The strongest effect, which comes nearest to the controls i.e. the reporter plasmid without insert or with four mutated binding sites, was found for a combination of the two mutated 5-mer and the mutated first adjacent canonical binding site. As for the remaining combinations that contained each a single mutated canonical binding site together with a single mutated 5-mer binding site, we found effects that were mostly stronger than the effects of single mutated canonical binding site but less strong than the effects of any combination that contained two mutated 5-mer binding sites. The co-transfection of wildtype TCRA-reporter vector with miR-34a led to a significant decrease of the luciferase activity to 40.3% (p < 0.001). The over-expression of miR-34a likely impacts the activation and functionality of T cells via decreased levels of TCRA, which is part of TCR-CD3E complex [Citation57,Citation58] and which is essential for the initiation of the adaptive immune response by binding to the peptide-bound major histocompatibility complex (pMHC) [Citation59]. Notably, TCRA deficient mice show a defective thymic regulatory T cell (Treg) selection and an impairment of the Treg function [Citation60].
Figure 3. Dual luciferase reporter gene assay of TCRA 3′ UTR. HEK 293T cells were transfected with the indicated combinations of empty plasmids, reporter gene constructs and miRNA-34a expression vectors. 48h after transfection the cells were lysed and the luciferase activity was measured. The luciferase activity of the control vector experiment was set to 100%. The results represent the mean of four independent experiments carried out in duplicates. Three asterisks correspond to p < 0.001, two asterisks to 0.001 < p < 0.01 and NS. to not significant. Data are represented as mean±SEM. The schematic illustration on the right side shows the location of the miR-34a binding sites for each reporter construct respectively. Green boxes correspond to wild type (wt) binding sites whereas red boxes indicate mutated binding sites. The labels on top of this illustration indicate which column corresponds to which functional binding sites of miR-34a.
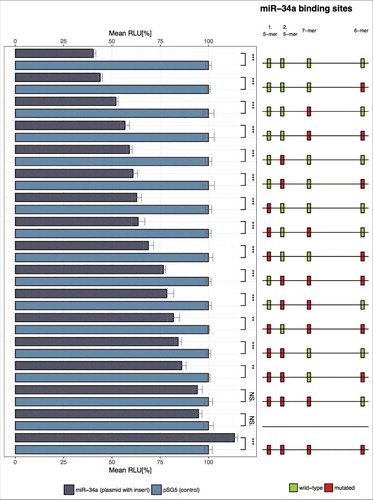
Conclusion and outlook
In sum, our results demonstrate that 5-mer binding sites can have a substantial influence on transcript abundance of target genes that might even outreach the impact of canonical binding sites. Such 5-mer sites are significantly enriched in known and even validated miRNA-target gene pairs. To prevent counting 5-mers that might arise as evolutionary remnants of longer seed matches, i.e. a longer k-mer with one or two point mutations, our k-mer selection procedure allows up to 2 mismatches, strategically precluding these cases. Besides longer k-mers, also shorter fragments, specifically 4-mers, are potentially enriched in 3′ UTRs of target genes. Whether these are artifacts or could also add to the gene targeting has to be investigated in thorough experiments. The here described findings challenge both the concept of a single predominant binding site for miRNAs within the 3′ UTRs of genes and the concept of an undisputed prevalent role of canonical binding sites of the miRNAs. Although to prove the generality of target gene regulation via 5-mer sites further large-scale experimental validation is needed, adding the knowledge of the 5-mer sites has a significant potential to improve current computational and machine learning miRNA – gene interaction predictors.
Methods
Cell lines, tissue culture
The human HEK 293T cell line was purchased from the German collection of microorganisms and cell cultures (DSMZ) and authenticated by STR DNA typing. HEK 293T cells were cultured in DMEM (Life Technologies GmbH, Darmstadt, Germany) with 10% Fetal bovine serum (Biochrom GmbH, Berlin, Germany) and Penicillin (100 U/mL), Streptomycin (100 μg/mL).
Dual luciferase reporter assays
For the dual luciferase assays, 6.5 × 104 HEK 293T cells were seeded out per well of a 24-well plate. After 24 hours the cells were transfected with 0.2 μg reporter construct and 0.8 μg miRNA expression plasmid per well using PolyFect transfection reagent (Qiagen, Hilden, Germany). The transfection was carried out corresponding to the manufacturer's protocol. Two days after transfection HEK 293T cells were lysed and measured according to Dual Luciferase® Reporter Assay System protocol (Promega, Mannheim, Germany).
Plasmids
The pSG5-miR-34a expression vector was cloned as described previously [Citation31]. Nucleotides 96–497 of the TCRA 3′ UTR (GenBank: X02592.1) were PCR amplified using specific primers from Jurkat cDNA as template. The TCRA 3′ UTR amplicon was cloned into the pMIR-RNL-TK vector using the SpeI, SacI restriction sites. This vector was described in Beitzinger et al. [Citation61]. The hsa-miR-34a-5p binding sites in the TCRA 3′ UTR were mutated applying site-directed mutagenesis with the QuickChange II Site-Directed Mutagenesis Kit (Agilent Technologies, Santa Clara, United States) using specific primers. The primers used for amplification of the TRCA 3′ UTR and the mutation of the reporter constructs respectively are shown in supplementary Table 1.
Data analysis
Statistical analysis of the luciferase assays was carried out with SigmaPlot 10 (Systat, Chicago, USA) applying Student's t-test.
Bioinformatics analysis
An overview of our workflow is illustrated in . For the binding site enrichment analysis, we downloaded all human miRNAs from miRBase v21 [Citation6] and extracted the contiguous 5-, 6-, 7-, and 8-mers sequences starting at the second position from the 5′ end. We also collected human 3′ UTR regions currently annotated in Ensembl (http://www.ensembl.org 52) and manually curated by the havana project. To acquire the respective target sequences we used these regions in combination with the Ensembl human reference genome (GRCh38) as input to the bedtools “getfasta” [Citation62] routine. From this we built a bowtie index and mapped the extracted, contiguous miRNA k-mers to each UTR sequence using bowtie v1.1.2 [Citation63]. During the computation of alignments, zero mismatches, i.e. a perfect matching for 5-mers, up to one mismatch for 6- and 7-mers, and up to two mismatches for 8-mers were considered. After a stringent k-mer selection process (), the remaining matches were processed in SAM/BAM format using Samtools [Citation64]. As a result, we summarized the counts in a table providing for each possible miRNA – UTR pair the number of mapped 5-, 6-, 7- and 8-mers. Finally, we intersected each of these pairs with the validated interactions known from the miRTarBase v7, TarBase v6, miRecords v4, and a large CLASH dataset from which 26163 weak, and 8248 strong annotations could be acquired [Citation52–Citation55]. To identify significant differences between the strongly or weakly validated targets and no validation at all we performed an unpaired Student's t-test assuming unequal variance between these categories, for each k-mer size respectively.
Figure 4A. Illustration on the underlying algorithm to detect miRNA k-mer enrichments in possible target sequences. Human mature miRNA 5p/3p sequences were collected from miRBase v21, while 3′ UTRs for human were acquired from Ensembl Release 91 [Citation51]. After extracting and mapping the k-mers with up to 2 mismatches for each possible binding site, the best k-mer was selected. Subsequently, for each miRNA and gene pair for which at least one valid k-mer existed we counted the number of 5-, 6-, 7-, and 8-mers. Finally, all pairs were annotated with their experimental status using validated interactions from miRTarbase v7, TarBase v6, miRecords v4, and a custom CLASH dataset [Citation52-Citation55].
![Figure 4A. Illustration on the underlying algorithm to detect miRNA k-mer enrichments in possible target sequences. Human mature miRNA 5p/3p sequences were collected from miRBase v21, while 3′ UTRs for human were acquired from Ensembl Release 91 [Citation51]. After extracting and mapping the k-mers with up to 2 mismatches for each possible binding site, the best k-mer was selected. Subsequently, for each miRNA and gene pair for which at least one valid k-mer existed we counted the number of 5-, 6-, 7-, and 8-mers. Finally, all pairs were annotated with their experimental status using validated interactions from miRTarbase v7, TarBase v6, miRecords v4, and a custom CLASH dataset [Citation52-Citation55].](/cms/asset/a3e01ca1-76d3-4d98-9b22-48fb3ec8d0d6/krnb_a_1462652_f0004a_oc.jpg)
Figure 4B. Rules for selecting the best k-mer for each possible binding site between a miRNA and a target sequence based on k-mer alignments are depicted. In case 1 the longest k-mer, in this case a 7-mer, forms the best match since no mismatches occurred and no better, i.e. longer, alignment was found. For case 2 the shown 7-mer is still the best k-mer match since a mismatch (shown in red) occurred inside the 7-mer but not at the leftmost end. In case 3 the 6-mer is selected as best matching as the 7-mer has a non-valid mismatch according to the table of allowed mismatches and the mismatch occurred at the leftmost position of the 7-mer. All other occurring alignment cases can be lead back to any of the three cases and treated analogously.
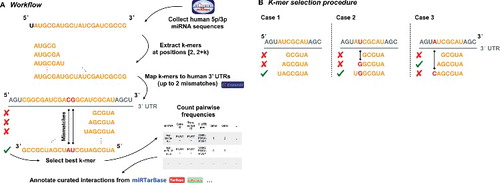
Disclosure of potential conflicts of interest
No potential conflicts of interest were disclosed.
Acknowledgments
We appreciate the financial support of the European Union and the Michael J. Fox Foundation.
Additional information
Funding
References
- Lee TI, Young RA. Transcriptional regulation and its misregulation in disease. Cell. 2013;152:1237–51.
- Lee RC, Feinbaum RL, Ambros V. The C. elegans heterochronic gene lin-4 encodes small RNAs with antisense complementarity to lin-14. Cell. 1993;75:843–54.
- Hofmann S, Huang Y, Paulicka P, et al. Double-Stranded Ligation Assay for the Rapid Multiplex Quantification of MicroRNAs. Anal Chem. 2015;87:12104–11.
- Kappel A, Backes C, Huang Y, et al. MicroRNA in vitro diagnostics using immunoassay analyzers. Clin Chem. 2015;61:600–7.
- Mestdagh P, Hartmann N, Baeriswyl L, et al. Evaluation of quantitative miRNA expression platforms in the microRNA quality control (miRQC) study. Nat Methods. 2014;11:809–15.
- Kozomara A, Griffiths-Jones S. miRBase: annotating high confidence microRNAs using deep sequencing data. Nucleic Acids Res. 2014;42:D68–73.
- Yu SL, Chen HY, Chang GC, et al. MicroRNA signature predicts survival and relapse in lung cancer. Cancer Cell. 2008;13:48–57.
- Ma L, Teruya-Feldstein J, Weinberg RA. Tumour invasion and metastasis initiated by microRNA-10b in breast cancer. Nature. 2007;449:682–8.
- Chan JA, Krichevsky AM, Kosik KS. MicroRNA-21 is an antiapoptotic factor in human glioblastoma cells. Cancer Res. 2005;65:6029–33.
- Meder B, Keller A, Vogel B, et al. MicroRNA signatures in total peripheral blood as novel biomarkers for acute myocardial infarction. Basic Res Cardiol. 2011;106:13–23.
- Keller A, Leidinger P, Borries A, et al. miRNAs in lung cancer – studying complex fingerprints in patient's blood cells by microarray experiments. BMC Cancer. 2009;9:353.
- Keller A, Leidinger P, Lange J, et al. Multiple sclerosis: microRNA expression profiles accurately differentiate patients with relapsing-remitting disease from healthy controls. PLoS One. 2009;4:e7440.
- Keller A, Leidinger P, Steinmeyer F, et al. Comprehensive analysis of microRNA profiles in multiple sclerosis including next-generation sequencing. Mult Scler. 2014;20:295–303.
- Leidinger P, Keller A, Borries A, et al. High-throughput miRNA profiling of human melanoma blood samples. BMC Cancer. 2010;10:262.
- Hausler SF, Keller A, Chandran PA, et al. Whole blood-derived miRNA profiles as potential new tools for ovarian cancer screening. Br J Cancer. 2010;103:693–700.
- Leidinger P, Keller A, Borries A, et al. Specific peripheral miRNA profiles for distinguishing lung cancer from COPD. Lung Cancer. 2011;74:41–7.
- Roth P, Wischhusen J, Happold C, et al. A specific miRNA signature in the peripheral blood of glioblastoma patients. J Neurochem. 2011;118:449–57.
- Leidinger P, Backes C, Deutscher S, et al. A blood based 12-miRNA signature of Alzheimer disease patients. Genome Biol. 2013;14:R78.
- Keller A, Leidinger P, Vogel B, et al. miRNAs can be generally associated with human pathologies as exemplified for miR-144. BMC Med. 2014;12:224.
- Lee I, Ajay SS, Yook JI, et al. New class of microRNA targets containing simultaneous 5'-UTR and 3'-UTR interaction sites. Genome Res. 2009;19:1175–83.
- Bartel DP. MicroRNAs.: genomics, biogenesis, mechanism, and function. Cell. 2004;116:281–97.
- Almeida MI, Reis RM, Calin GA. MicroRNA history: discovery, recent applications, and next frontiers. Mutat Res. 2011;717:1–8.
- Reyes-Herrera PH, Ficarra E. One decade of development and evolution of microRNA target prediction algorithms. Genomics Proteomics Bioinformatics. 2012;10:254–63.
- Srivastava PK, Moturu TR, Pandey P, et al. A comparison of performance of plant miRNA target prediction tools and the characterization of features for genome-wide target prediction. BMC Genomics. 2014;15:348.
- Peterson SM, Thompson JA, Ufkin ML, et al. Common features of microRNA target prediction tools. Front Genet. 2014;5:23.
- Agarwal V, Bell GW, Nam JW, et al. Predicting effective microRNA target sites in mammalian mRNAs. Elife. 2015;4:
- Reczko M, Maragkakis M, Alexiou P, et al. Functional microRNA targets in protein coding sequences. Bioinformatics. 2012;28:771–6.
- Hamberg M, Backes C, Fehlmann T, et al. MiRTargetLink–miRNAs, Genes and Interaction Networks. Int J Mol Sci. 2016;17:564.
- Bartel DP. MicroRNAs.: target recognition and regulatory functions. Cell. 2009;136:215–33.
- Brennecke J, Stark A, Russell RB, et al. Principles of microRNA-target recognition. PLoS Biol. 2005;3:e85.
- Hart M, Rheinheimer S, Leidinger P, et al. Identification of miR-34a-target interactions by a combined network based and experimental approach. Oncotarget. 2016;7:34288–99.
- Ludwig N, Kim YJ, Mueller SC, et al. Posttranscriptional deregulation of signaling pathways in meningioma subtypes by differential expression of miRNAs. Neuro Oncol. 2015;17:1250–60.
- Landi MT, Zhao Y, Rotunno M, et al. MicroRNA expression differentiates histology and predicts survival of lung cancer. Clin Cancer Res. 2010;16:430–41.
- Vogt M, Munding J, Gruner M, et al. Frequent concomitant inactivation of miR-34a and miR-34b/c by CpG methylation in colorectal, pancreatic, mammary, ovarian, urothelial, and renal cell carcinomas and soft tissue sarcomas. Virchows Arch. 2011;458:313–22.
- Lodygin D, Tarasov V, Epanchintsev A, et al. Inactivation of miR-34a by aberrant CpG methylation in multiple types of cancer. Cell Cycle. 2008;7:2591–600.
- Siemens H, Neumann J, Jackstadt R, et al. Detection of miR-34a promoter methylation in combination with elevated expression of c-Met and beta-catenin predicts distant metastasis of colon cancer. Clin Cancer Res. 2013;19:710–20.
- Cui X, Zhao Z, Liu D, et al. Inactivation of miR-34a by aberrant CpG methylation in Kazakh patients with esophageal carcinoma. J Exp Clin Cancer Res. 2014;33:20.
- Xie K, Liu J, Chen J, et al. Methylation-associated silencing of microRNA-34b in hepatocellular carcinoma cancer. Gene. 2014;543:101–7.
- Li Y, Guessous F, Zhang Y, et al. MicroRNA-34a inhibits glioblastoma growth by targeting multiple oncogenes. Cancer Res. 2009;69:7569–76.
- He L, He X, Lim LP, et al. A microRNA component of the p53 tumour suppressor network. Nature. 2007;447:1130–4.
- Liu C, Kelnar K, Liu B, et al. The microRNA miR-34a inhibits prostate cancer stem cells and metastasis by directly repressing CD44. Nat Med. 2011;17:211–5.
- Bu P, Chen KY, Chen JH, et al. A microRNA miR-34a-regulated bimodal switch targets Notch in colon cancer stem cells. Cell Stem Cell. 2013;12:602–15.
- Yamakuchi M, Ferlito M, Lowenstein CJ. miR-34a repression of SIRT1 regulates apoptosis. Proc Natl Acad Sci U S A. 2008;105:13421–6.
- Eichelser C, Flesch-Janys D, Chang-Claude J, et al. Deregulated serum concentrations of circulating cell-free microRNAs miR-17, miR-34a, miR-155, and miR-373 in human breast cancer development and progression. Clin Chem. 2013;59:1489–96.
- Aherne ST, Madden SF, Hughes DJ, et al. Circulating miRNAs miR-34a and miR-150 associated with colorectal cancer progression. BMC Cancer. 2015;15:329.
- Franchina T, Amodeo V, Bronte G, et al. Circulating miR-22, miR-24 and miR-34a as novel predictive biomarkers to pemetrexed-based chemotherapy in advanced non-small cell lung cancer. J Cell Physiol. 2014;229:97–9.
- Baier G, Wagner J. PKC inhibitors: potential in T cell-dependent immune diseases. Curr Opin Cell Biol. 2009;21:262–7.
- Huse M. Microtubule-organizing center polarity and the immunological synapse: protein kinase C and beyond. Front Immunol. 2012;3:235.
- Quann EJ, Liu X, Altan-Bonnet G, et al. A cascade of protein kinase C isozymes promotes cytoskeletal polarization in T cells. Nat Immunol. 2011;12:647–54.
- Grybko MJ, Pores-Fernando AT, Wurth GA, et al. Protein kinase C activity is required for cytotoxic T cell lytic granule exocytosis, but the theta isoform does not play a preferential role. J Leukoc Biol. 2007;81:509–19.
- Wei SY, Lin TE, Wang WL, et al. Protein kinase C-delta and -beta coordinate flow-induced directionality and deformation of migratory human blood T-lymphocytes. J Mol Cell Biol. 2014;6:458–72.
- Yates A, Akanni W, Amode MR, et al. Ensembl 2016. Nucleic Acids Res. 2016;44:D710–6.
- Chou CH, Shrestha S, Yang CD, et al. miRTarBase update 2018: a resource for experimentally validated microRNA-target interactions. Nucleic Acids Res. 2018;46:D296–D302.
- Vergoulis T, Vlachos IS, Alexiou P, et al. TarBase 6.0: capturing the exponential growth of miRNA targets with experimental support. Nucleic Acids Res. 2012;40:D222–9.
- Xiao F, Zuo Z, Cai G, et al. miRecords: an integrated resource for microRNA-target interactions. Nucleic Acids Res. 2009;37:D105–10.
- Helwak A, Kudla G, Dudnakova T, et al. Mapping the human miRNA interactome by CLASH reveals frequent noncanonical binding. Cell. 2013;153:654–65.
- Acuto O, Hussey RE, Fitzgerald KA, et al. The human T cell receptor: appearance in ontogeny and biochemical relationship of alpha and beta subunits on IL-2 dependent clones and T cell tumors. Cell. 1983;34:717–26.
- Moran AE, Hogquist KA. T-cell receptor affinity in thymic development. Immunology. 2012;135:261–7.
- Merkle PS, Irving M, Hongjian S, et al. The T-Cell Receptor Can Bind to the Peptide-Bound Major Histocompatibility Complex and Uncomplexed beta2-Microglobulin through Distinct Binding Sites. Biochemistry. 2017;56:3945–61.
- Yaciuk JC, Pan Y, Schwarz K, et al. Defective selection of thymic regulatory T cells accompanies autoimmunity and pulmonary infiltrates in Tcra-deficient mice double transgenic for human La/Sjogren's syndrome-B and human La-specific TCR. J Immunol. 2015;194:1514–22.
- Beitzinger M, Peters L, Zhu JY, et al. Identification of human microRNA targets from isolated argonaute protein complexes. RNA Biol. 2007;4:76–84.
- Quinlan AR, Hall IM. BEDTools: a flexible suite of utilities for comparing genomic features. Bioinformatics. 2010;26:841–2.
- Langmead B, Trapnell C, Pop M, et al. Ultrafast and memory-efficient alignment of short DNA sequences to the human genome. Genome Biol. 2009;10:R25.
- Li H, Handsaker B, Wysoker A, et al. The Sequence Alignment/Map format and SAMtools. Bioinformatics. 2009;25:2078–9.