ABSTRACT
Long non-coding RNAs have emerged as an important regulatory layer in biological systems. Of the various types of lncRNAs, one class (designated as divergent RNAs/XH), which is in head-to-head overlap with the coding genes, has emerged as a critical biotype that regulates development and cellular differentiation. This work aimed to analyze previously published data on differential expression, epigenetic and network analysis in order to demonstrate the association of divergent lncRNAs, a specific biotype with the differentiation of human neural progenitor cells (hNPCs). We have analyzed various available RNAseq databases that address the neuronal and astrocytic differentiation of hNPCs and identified differentially expressed lncRNAs (DELs) during cell-fate determination. Key DELs identified from the databases were experimentally verified by us in our in-vitro hNPC differentiation system. We also analyzed the change in promoter activity using ChIP-seq datasets of the histone markers H3K4me3 (activation) and H3K27me3 (inactivation) of these DELs. Additionally, we explored the change in the euchromatinization state of DELs (by analyzing DNase-seq data) during lineage-specific differentiation of hNPCs and performed their network analysis. We were able to identify differences between neuronal and astrocytic differentiation of hNPCs at the level of divergent DELs epigenetic markers, DNAase hypersensitive sites and gene expression network. Divergent lncRNAs are more involved in neuronal rather than astrocytic differentiation, while the sense downstream lncRNA biotype appears to be more involved in astrocytic differentiation. By studying the lncRNA involvement of distinct biotypes, we have been able to indicate the preferential role of a particular biotype during lineage-specific differentiation.
Introduction
Long non-coding RNAs (lncRNAs) have a characteristic length of more than 200 nucleotides and low protein-coding potential. The number of annotated lncRNA genes in human genomes surpasses the number of coding genes, and they represent a functionally diverse class of transcribed RNA [Citation1]. lncRNAs are believed to encompass nearly 30,000 different transcripts in humans and account for the majority of the non-coding transcriptome [Citation2]. lncRNAs can be classified by their genomic distribution pattern relative to protein-coding loci [Citation3]. According to this classification, lncRNAs can be classified into two classes, genic lncRNA (<5 kb from a coding gene) and intergenic lncRNA (lincRNA, >5 kb). The genic lncRNA class is further divided into six biotypes; Antisense Head-to-head (XH) or divergent, Antisense Tail-to-tail (XT) or convergent, Antisense Inside (XI), Antisense Outside (XO), Sense Downstream (SD), Sense Upstream (SU). lncRNAs are called divergent when they are transcribed in the opposite direction to nearby protein-coding gene [Citation4]. Divergent lncRNAs constitute a major population of total lncRNA in the mammalian genome [Citation5]. Divergent XH and antisense-inside XI lncRNAs comprise of around 19%–27% and 20%-21% of total lncRNAs respectively, representing the two largest genic lncRNA biotypes in human and mouse genome. Divergent XH lncRNAs are a crucial class of RNA that regulate developmental processes and have different regulatory functions [Citation3]. Studies have also shown that some antisense transcripts affect chromatin remodeling and act as epigenetic regulators of gene expression [Citation6,Citation7]. However, the role of divergent lncRNAs in the maintenance of stemness and lineage-specific differentiation of human pluripotent stem cells is poorly understood. Various individual lncRNAs have been described to play an important role in brain development, but the critical role of divergent lncRNAs as a class is yet to be explored.
Neural progenitor stem cells are multipotent and have the ability to differentiate into committed brain cells (astrocytes and neurons) [Citation8,Citation9]. Since lncRNAs are tissue-specific and developmentally regulated, we studied their association with fate determination of NPCs. LncRNAs have been shown to play a significant role in neuronal development [Citation10]. The molecular function and unique developmental functions of a neural-specific lncRNA named as Pnky has been studied, Pnky has been demonstrated to regulate development of the embryonic mouse cortex [Citation11]. As regards astroglial differentiation, there are some reports of specific lncRNAs in the differentiation oligodendrocytes and astrocyte enriched lncRNAs [Citation12–Citation15]. However, there are no reports on the lineage-specific global identification and characterization of classes of lncRNAs in NPCs especially human NPCs.
In this study, we have explored publically available next-generation sequencing datasets to identify novel lncRNAs, which might play a role in human neuronal and glia differentiation from a neural precursor or stem cells. We analyzed RNAseq datasets of neuronal and astrocytic differentiation of human NPCs. These datasets indicated the presence of many lineage-specific lncRNAs that were associated with the distinct cellular identity. We then analyzed the promoter activity of these differentially expressed lncRNAs using ChIPseq data. This showed that the epigenetic markers of transcriptional activation (H3K4me3) and inhibition (H3K27me3) had the expected association with divergent lncRNA expression in hNPCs and differentiated cells. This was further validated by network analysis. We also did Gene Ontology (GO) analysis of all the identified lncRNAs to understand their possible functional roles. Further, we experimentally validated some of these differentially expressed lncRNAs in our well-characterized in-vitro system of hNPC differentiation. Combining RNA-seq, epigenetics, network analysis, GO analysis and experimental validation, we have been able to show the association of divergent lncRNAs with neuronal but not astrocytic differentiation. The sense downstream lncRNA biotype appears to be more associated with astrocytic differentiation. Our study thus indicates that specific lncRNA biotypes may be associated with the different process of cellular differentiation especially in the case of hNPCs.
Results
Identification and epigenetic analysis of divergent lncRNAs during neuronal differentiation of hNPCs
To identify lncRNAs which could play a role in neuronal differentiation, we have analyzed already available RNA-seq dataset (GSE75719) from NCBI [Citation16] (Supplementary Figure S1). This dataset analyzes the change and the role of metabolic related genes during reprogramming of human neural progenitors to neurons. According to this report, these NPCs were derived from iPSCs reprogrammed from the human BJ male fibroblast. The authors had performed RNA-seq experiments with two different NPC lines reprogrammed from independent BJ iPSCs clones. Procedure and protocol for characterization, reprogramming, and differentiation of hNPCs can be obtained from the same article [Citation16]. We used this dataset to identify differentially expressed lncRNAs associated genes during neuronal differentiation. First, we combined NPCs, neurons and annotated lncRNAs datasets [Citation3] and then extracted divergent (XH) lncRNAs associated genes expression. We identified differentially expressed lncRNAs between NPCs and neurons by taking neurons versus NPCs gene expression ratio. We shortlisted only those genes that showed statistically significant differential expression as determined by using two-tail student t-test with corrected p values of p ≤ 0.05. We further restricted our analysis to those genes, which were showing at least two-fold upregulation or downregulation.
After this analysis, we have found that 62 divergent lncRNAs-associated genes were upregulated and 103 were downregulated (), Supplementary S1). Several of these divergent lncRNA associated genes have already been shown to be important for neuronal differentiation (SOX2, NEUROD1, LHX5, DUSP26, and SYNGR3), while the function of many are not yet known. When these genes were subjected to Gene Ontology (GO) analysis, they showed significant enrichment of processes for nervous system development and neurogenesis (). To further validate their differential expression pattern between hNPCs and neurons, we have assessed their chromatin state using ChIP-seq data of H3K4me3 (histone 3 lysine 4-trimethylation) and H3K27me3 (histone 3 lysine 27-trimethylation) in hNPCs (GSE96300, GSM896165) and neurons (GSM772776, GSM772787). H3K4me3 and H3K27me3 are epigenetic histone markers of activation and repression of chromatin, which indicate the state of transcription of their respective the genes. We observed a highly significant increase in the activation marker (H3K4me3) scores in both upregulated (p = 2.3*10−7) and downregulated (p = 1.5*10−19) genes in neurons compared to hNPCs (), Chip-seq UCSC tracks of examples of some genes shown in Supplementary Figure S2). However, while there was a difference in the H3K27me3 markers scores in neuronal upregulated (p ≤ 0.06) DELs, it only approached statistical significance. For downregulated genes, it did not even approach significance (p = 0.711) genes (). Hence the downregulated genes during neuronal differentiation unexpectedly showed a pattern closer to that seen in upregulated genes as regards the chromatin activation markers.
Figure 1. Identification of DELs in neuronal differentiation of hNPCs. (a–b) represents the heatmap of upregulated and downregulated DELs respectively. (c–d) shows GO analysis of upregulated and downregulated DELs respectively.
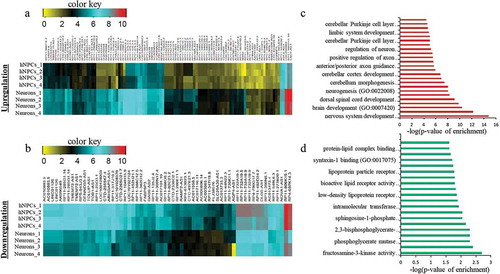
Figure 2. ChIP-seq analysis of H3K4me3 and H3K27me3 of DELs during neuronal differentiation. (a–b) shows the ChIP-seq scores of H3K4me3 of upregulated (p ≤ 2.3 × 10−7) and downregulated (p ≤ 1.5 × 10−19) DELs. (c–d) shows the ChIP-seq scores of H3K27me3 of upregulated (p ≤ 0.06) and downregulated (p ≤ 0.7) DELs.
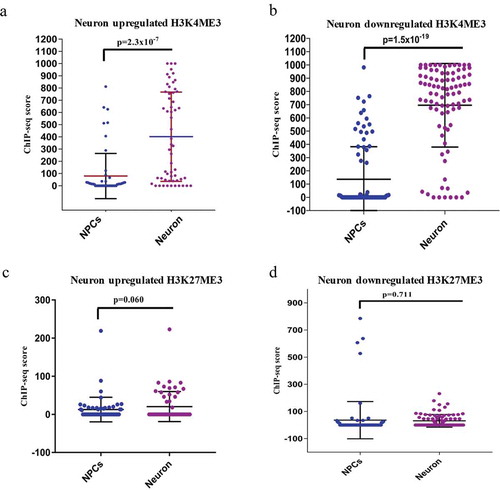
The markedly stronger statistical association of the activated state of H3K4me3 with both upregulation and downregulation points to the role of the divergent lncRNAs, which share or are close to the promoter of the coding genes. Activated divergent lncRNAs are known to both downregulate and upregulate their paired coding genes [Citation3]. The increased lncRNA expression during both the upregulation and downregulation of the coding gene may indicate that the direction of the regulation may depend more on the effects of lncRNA rather that of the localized chromatin state, which tends to be in activated form during both upregulation and downregulation.
Lower involvement of divergent lncRNAs during astrocytic differentiation
Similar to the process of identifying the divergent lncRNA biotypes involved in neuronal differentiation, for astrocytic differentiation we have analyzed the available RNA-seq dataset (GSE76122) from NCBI (27,564,458) (Supplementary Figure S3). The authors had analyzed the data for coding genes but not for lncRNAs. The analysis of astrocyte-specific lncRNAs was done as already mentioned for neurons. Here we combined NPCs, Astrocytes and annotated lncRNAs RNAseq datasets [Citation17]. We then extracted XH lncRNA expression, as already explained for neuronal differentiation. We then proceeded to identify differentially expressed genes between NPCs and Astrocytes by taking Astrocytes versus NPCs gene expression ratio. We estimated the significant differential expression using two-tail student t-test with corrected p-value p ≤ 0.05 and then restricted the analysis to those genes showing more than two-fold change in expression. From this analysis, we have found 21 upregulated genes and 18 downregulated genes respectively (), Supplementary S2), which is markedly less than seen for neuronal differentiation (there were 62 upregulated and 103 downregulated genes). GO analysis showed that the coding genes are linked to astrocytes function like ion transport, amino acid metabolism, helps in action potential, etc ().
Figure 3. Identification of DELs in astroglial differentiation of hNPCs. a-b represent the heatmap of upregulated and downregulated DELs respectively. c-d shows GO analysis of upregulated and downregulated DELs respectively.
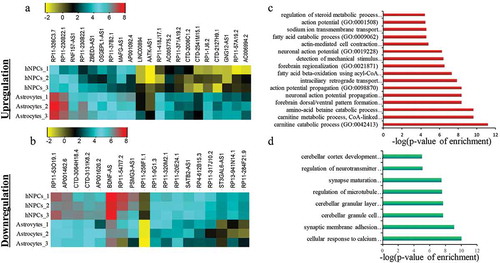
We further analyzed ChIP-seq data of H3K4me3 (histone 3 lysine 4-trimethylation) and H3K27me3 (histone 3 lysine 27-trimethylation) in hNPCs and astrocytes. Here the results differed from that observed in neuronal differentiation. We have observed a markedly significant decrease in activation markers (H3K4me3) scores in both upregulated (p ≤ 6.1*10−4) and downregulated genes (p ≤ 9.8*10−5) in astrocytes compared to hNPCs (), supplementary Figure S4). However, the difference in H3K27me3 marker scores in upregulated genes had a much lower level of significance (p ≤ 0.027) as compared p ≤ 2.3x10−7 for neurons and no significant difference in downregulated (p ≤ 0.132) as compared to p ≤ 1.5 × 10−19 for neurons ().
Figure 4. ChIP-seq analysis of H3K4me3 and H3K27me3 of DELs during astroglial differentiation. (a–b) shows the ChIP-seq scores of H3K4me3 of upregulated (p ≤ 6.1 × 10−4) and downregulated (p ≤ 9.8 × 10−8) DELs. (c–d) shows the ChIP-seq scores of H3K27me3 of upregulated (p ≤ 0.027) and downregulated (p ≤ 0.132) DELs.
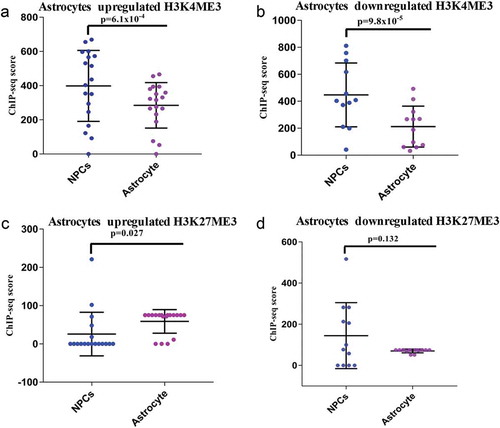
Further, to assess the nature of epigenetic markers during the neuronal and astrocytic differentiation of hNPCs, we calculated the percentage of DELs having H3Kme3, the epigenetic marker of activation. We have found that around 81% of neuronal differentiated DELs genes posses’ activation histone markers as compared to this only around 36–39% of astrocytic differentiated DELs genes have H3Kme3 markers. This interesting finding shows the higher impact of epigenetic regulation on neuronal differentiation as compared to astrocytic differentiation ().
Figure 5. Verification of DELs in hNPCs and differentiated neurons and astrocytes. (a) Phase-contrast image of hNPCs derived neurosphere, (b) ICC images of hNPCs showing positive for NESTIN and SOX2 markers, (c) hNPCs derive neurons were positive for neuronal markers MAP2 and TUJ1, (d) hNPCs derive astrocytes were positive for GFAP and S100β, (e) relative expression of significantly upregulated lncRNAs, AQP4-AS1, CYP-AS1 and LHX-AS1 during neuronal differentiation in our hNPCs culture system, (f) relative expression of significantly downregulated lncRNAs, SPAG5-AS1 and SBTB-AS1 during neuronal differentiation, (g) relative expression of significantly upregulated lncRNAs, RNF-AS1 and CCNT2-AS1 during astrocytic differentiation, (h) relative expression of significantly downregulated lncRNAs, PRKAG-AS1, KRTAP-AS1 and PSMG-AS1 during astrocytic differentiation. *p ≤ 0.05, **p ≤ 0.005, ***p ≤ 0.0005, student t-test.
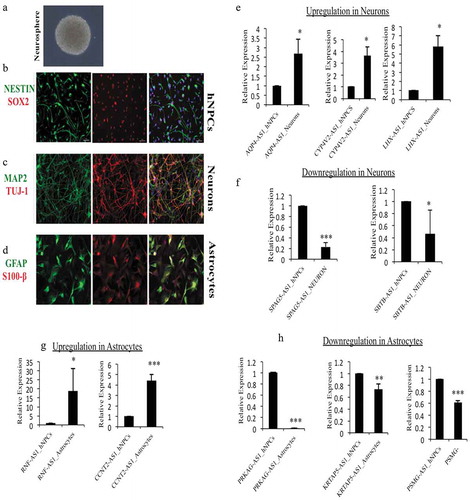
Differentiated neurons possess more DNase-I hypersensitive sites compared to astrocytes on differentially expressed divergent lncRNA loci
Euchromatin or the open genomic region of the genome is the accessible genomic region available for transcription. These regions are tissue and cell type specific [Citation18]. Selectively accessible regions in specific cell types are important for maintaining their identity. These regions are DNase hypersensitive and have a good concordance with euchromatin. Brain cells are very diverse in their functions, morphology and molecular niches. Various types of brain cells have specific euchromatin regions, which is necessary for their functionality. The euchromatin status of lncRNA genes might also vary among different types of brain cells as lncRNAs are also developmentally regulated and are cell/tissue specific. To check this hypothesis, we employed ENCODE DNase-seq datasets of hNPCs, neurons, astrocytes and embryonic stem cells (ESCs). First, we analyzed the overall DNase-seq scores from all the mentioned cell types (). According to their state of differentiation, we thought that ESCs should have the maximum accessible genome or DNase-seq scores as compared to other cell types. However, surprisingly NPCs showed more DNase scores as compared to ESCs and other cell types ()). This data reflects that NPCs need more genes accessible for transcription for maintaining their state as compared to ESCs. A possible reason for this could be their ability to differentiate into diverse cell types (various subtypes of neurons, astrocytes, and oligodendrocytes).
Figure 6. Divergent DELs promoters have higher number of H3K4me3 imprints during neural differentiation.(a) represent the percentage of upregulated and downregulated DELs having H3K4me3 histone marks during neural differentiation of hNPCs. (b) represent the percentage of upregulated and downregulated DELs having H3K4me3 markers during astroglial differentiation of hNPCs. Around 81% of DELs were positive for H3K4me3 histone marks during neural differentiation, while only there were around 36–39% present on DELs promoter during astroglial differentiation, which shows the impact of epigenetic effect of XH lncRNAs on neural differentiation. (c) represent the percentage of upregulated and downregulated DELs having H3K27me3 histone marks during neural differentiation of hNPCs. (b) represent the percentage of upregulated and downregulated DELs having H3K27me3 markers during astroglial differentiation of hNPCs.
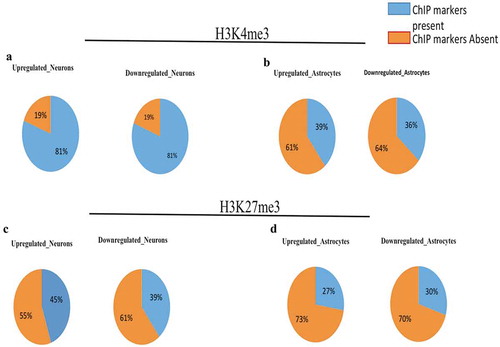
We also compared the change in state of euchromatin around differentially expressed lncRNA genes during neurogenesis and astrogliogenesis in the data obtained from the databases. For these differentially expressed genes, one significant observation was that divergent lncRNAs in ESCs had much lower scores as compared to hNPCs (p = 2.9 × 10−10). In differentiated neurons DNase-seq scores were slightly higher than hNPCs in a moderate significant manner (p = 0.03) ()). However, in differentiated astrocytes, the average DNase-seq scores of astrocytes specific DELs of the divergent lncRNA biotypes were significantly lower than in hNPCs (p = 2.08 × 10−6) ()). These were also lower than for ESCs. The differential nature of the regulation of cell-specific DELs (divergent lncRNA biotypes) is very remarkable. For neurons, neuronal specific DELs are associated with a high degree of accessible genome conformation (higher than hNPCs and much higher than ESCs). However, in differentiated astrocytes, the astrocyte-specific DEL coding regions are less accessible than in ESCs and much less than accessible as compared to NPCs. This data further emphasis on the distinct role divergent lncRNAs loci during neuronal differentiation.
Experimental verifications of DELs in the hNPCs culture system
The expression of selected DELs from the database used was experimentally verified in our laboratory using in vitro hNPC differentiation system. hNPCs were isolated from the telencephalon of 10–15 week old fetuses as described earlier [Citation19,Citation20]. Cultured hNPCs shows characteristic neurosphere formation in pluripotency maintenance medium ()). These hNPCs also tested positive for stemness marker genes such as nestin and SOX2 ()). These hNPCs were differentiated into neurons and astrocytes in the medium mentioned above. hNPCs differentiated neurons expresses neuronal markers MAP2 and Tuj-1, while hNPCs differentiated astrocytes were positive for GFAP and S100β markers (). We have validated significant differentially expressed lncRNAs using Real-Time PCR in our characterized hNPCs culture system. For experimental validation of neuronal differentiation, a cut-off of 3 fold change in gene expression was taken. This resulted in identifying 11 significantly differentially expressed genes (6 upregulated and 5 downregulated). Of these, AQP4-AS1 (n = 3, p ≤ 0.05), CYP4V2-AS1 (n = 3, p ≤ 0.02), and LHX5-AS1 (n = 3, p ≤ 0.02) were found significantly upregulated, while SPAG5-AS1 (n = 3, p ≤ 4.0 × 10−5) and SATB-AS1 (n = 3, p ≤ 0.04) were significantly downregulated (). The roles of AQP4 and LHX5 were found to be important for adult neurogenesis [Citation21–Citation23]. Knockdown of AQP4 has been shown to decrease the neuronal differentiation of cultured adult NSCs [Citation24].
Figure 7. Comparision of Euchromatinization using DNase-seq data in hESCs, hNPCs, neuron and astrocytes. (a–d) Heatmaps of DNase-seq Reads Per Kilobase Million (RPKM) in hESCs, hNPCs, neurons and astrocytes of ENCODE datasets. (e) showing enrichment scores using plotprofile of each cell types, (f) Comparision of DNase-seq scores of dELs in hNPCs, Neurons and ESCs, (g) Comparision of DNase-seq scores of dELs in hNPCs, Astrocytes and ESCs.
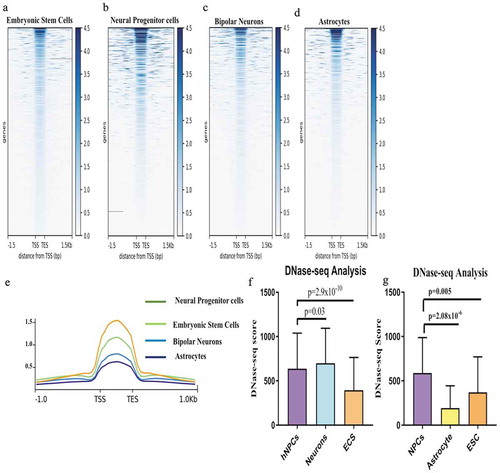
During astroglial differentiation, we chose 10 significantly differentially expressed genes; DELs with a fold change of more than 3 folds were taken for validation. Of these, RNF-AS1 (n = 3, p ≤ 0.03) and CCNT2-AS1 (n = 3, p ≤ 0.0003) were found significantly upregulated, while PRKAG-AS1 (n = 3, p ≤ 4.7 × 10−25), KRTAP5-AS1 (n = 3, p ≤ 0.003) and PSMG-AS1 (n = 3, p ≤ 2.3 × 10−5) were significantly downregulated (–h)).
Hence it appears that approximately 50% of the DELs identified in the databases behave in concordance with the results obtained from our experiment during lineage-specific differentiation. Considering the heterogeneity of the databases used, the experimental verification of the DELs further supports the use of these databases for the analysis.
Gene co-expression network analysis reveals the importance of XH lncRNAs in neuronal differentiation
Differential expression analysis of lncRNAs in hNPCs, neurons and astrocytes reveals many lineage-specific lncRNAs. To understand further their collective impact on the differentiation of hNPCs we have done co-expression network analysis. Co-expression network analysis is a measure of a set of genes being modulated in a similar manner (either upregulated or downregulated) during a particular process, in this case, hNPCs differentiation to either neurons or astrocytes. We have employed differentially expressed lncRNAs gene expression of neuronal and astrocytic differentiation, as indicated by databases studies, as inputs for network analysis. We did matrix correlation analysis followed by network formation using Cytoscape to quantify changes in network connectivity between hNPCs and their differentiated state viz., neurons and astrocytes. We have calculated network strength by a change in clustering coefficient between hNPCs and differentiated state. We have found that there was an increase in clustering coefficient in only of upregulated DELs (~7%) in neuronal differentiation but not in downregulated DELs (~-0.5%) or in astroglial differentiation (upregulated ~ −0.4% and downregulated ~ −0.31%) (), Supplementary Figure S5-S8). Our analysis shows that different aspects of XH lncRNA expression and epigenetic signatures are linked only during neuronal but not astrocytic differentiation.
Figure 8. Network analysis of XH lncRNAs and other biotypes during hNPCs differentiation. (A) showing change in clustering coefficient during neuronal differentiation and astroglial differentiation in upregulated and downregulated DELs, XH lncRNAs shows increase in network strength only during neuronal differentiation of hNPCs, (B) showing change in clustering coefficient of other lncRNAs biotypes during astroglial differentiation of hNPCs, SD biotypes shows the maximum changes in network strength during astroglial differentiation.
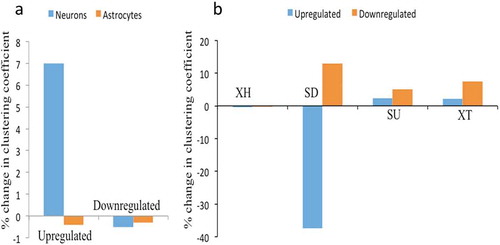
Next, we asked whether any other biotype of lncRNAs has a collective impact on astrocytic differentiation. For this, we first identified DELs of other biotypes of lncRNAs, followed by matrix correlation analysis and network analysis. Interestingly, out of all biotypes of lncRNAs Sense Downstream (SD) biotypes have shown a maximum change in cluster coefficient. Astrocyte differentiation resulted in the disintegration of the SD lncRNA cluster of hNPCs accompanied by a decrease in cluster coefhficient of upregulated genes (~ −37.4%) and increase in downregulated genes (~12.9%) during astrocytic differentiation ()). Other XH, SU and XT biotypes remained nearly unchanged. We did not find any differentially expressed lncRNA in remaining biotypes. The higher number of epigenetic markers on neuronal differentiation associated DELs thus emphasizes the differential nature of the involvement of lncRNA biotypes during lineage-specific hNPCs differentiation.
Material and methods
Accession numbers
The RNA-seq, ChIP-seq and DNase-seq data used in this paper have previously been deposited in the NCBI Gene Expression Omnibus (GEO) database and ENCODE database. GEO database for neuronal differentiation of hNPCs was deposited as GEO number GSE75719. GEO database for astrocytic differentiation of hNPCs was deposited as GEO number GSE76122
ChIP-seq data from ENCODE (https://www.encodeproject.org/)
DNase-seq data from ENCODE
RNA-seq data analysis
We have retrieved raw RNA-seq data in the form of fastq formats from European Nucleotide Archive (ENA, https://www.ebi.ac.uk/ena) with respective accession numbers (given earlier). Transcript level expression analysis of RNA-seq experiment was done with HISAT, StringTie and R-based visualization tool Ballgown [Citation25](27,560,171). Human genome (hg19) indexed file were generated using a hisat2-build script of HISAT2 [Citation26](25,751,142). Quality checked fastq files were aligned using HISAT2 with default parameter of the hisat2 script. Output sam files were then converted and sorted into bam files using samtools. StringTie was used to assemble and quantify expressed genes and transcripts. Differential expression analysis was done using R-based packages that include Ballgown for FPKM abundance estimation and statistical analysis for significant differences between the groups, RSkittleBrewer for setting up color, genefilter for fast calculation for means and variance, dplyr for sorting and arranging results and devtools for reproducibility and installing packages. Fold change of gene expression between the groups were again checked by two-tailed test and Bonferroni p-correction. File containing corrected p-values fold changes of XH lncRNA is submitted as Supplementary S1. Heatmap were generated using R-based (https://cran.r-project.org/) tool gplots (https://CRAN.R-project.org/package=gplots) and RColorBrewer (Brewer, Cynthia A., 200x. http://www.ColorBrewer.org).
DNase-seq data analysis
For Euchromatinization analysis in different cell types, we have used peak files from ENCODE (https://www.encodeproject.org/) DNase-seq data. From these peak files, we generated matrix using ComputeMatrix (a galaxy based web server) [Citation27]. The matrix files generated from ComputeMatrix were used to generate heatmap and peak profile using plotHeatmap and plotProfile tools.
Network analysis
Gene expressions of differentially expressed lncRNAs were taken to generate matrix correlation using R-based program ‘corrplot’ (https://github.com/taiyun/corrplot). Network analysis was done using ‘Cytoscape’ [Citation28]. The input files for Cytoscape were generated from matrix correlation data using in-house code.
Human fetal neural stem cell (hNSC) isolation and differentiation
Handling of human fetal brain tissues was done after obtaining mother’s informed consent and in accordance with the protocol approved by Institutional Human Ethics and Stem Cell and Research Committee of National Brain Research Centre, India in compliance with recommendations of the Indian Council of Medical Research (ICMR), India. Only 10–15 weeks old human aborted fetuses were utilized for isolation of hNPCs. hNPCs were cultured on Poly-D-lysine (Sigma-Aldrich, St. Louis, MO, USA) coated culture – wares as a monolayer. Growth media used was neurobasal (Invitrogen, San Diego, CA, USA) supplemented with Bovine Serum Albumin (Sigma-Aldrich, St. Louis, MO, USA), N2 supplement (Invitrogen, San Diego, CA, USA), Neural Survival Factor-1 (Lonza, Charles City, IA, USA), glutamine (Sigma, St. Louis, MO, USA), penicillin and streptomycin solution (Invitrogen, San Diego, CA, USA), gentamycin (Sigma, St. Louis, MO, USA), 25 ng/ml basic fibroblast growth factor (bFGF) (Peprotech, Rocky Hill, NJ) and 20 ng/ml epidermal growth factor (EGF) (Peprotech, Rocky Hill, NJ). hNPCs were passaged at least 9 times and characterized before performing experiments. The characterized of hNPCs was done by assessing the expression of neural stem cell markers nestin and SOX2, and lineage-specific makers MAP2 and GFAP. hNPCs were differentiated with neuronal lineage by the withdrawal of mitotic factor bFGF and EGF, and supplementing with 10 ng/ml brain-derived neurotrophic factor (BDNF) (Peprotech, Rocky Hill, NJ) and 10 ng/ml platelet-derived growth factor (PDGF-AB) (Peprotech, Rocky Hill, NJ). Cells were differentiated with neurons for 21 days in the above-mentioned medium before performing experiments. 99.9% of the cells were positive for neuronal marker MAP2 and Tuj-1. For astrocytic differentiation stem cell medium was replaced with Minimum Essential Medium (MEM) (Sigma–Aldrich, USA) containing 10% fetal bovine serum (Sigma–Aldrich, USA). Differentiated astrocytes were positive for astrocytic markers GFAP and S100b.
Discussion
Over the last decade, the regulatory role of non-coding RNAs has gained much attention. The recent classification of lncRNAs shows their non-random distribution in the genome and their locus based classification has given an insight into their role in the regulation of protein-coding genes. Out of many subtypes or classes of lncRNAs, we have focused on divergent lncRNAs because of their higher proportion as compared to the other lncRNAs subtypes in the mammalian genome. They show a comparatively higher enrichment with the development and transcription regulator genes [Citation5]. Some of the well-characterized divergent lncRNAs have been shown to have a role in neural precursor cell pluripotency and cell fate determination. However, the study of large databases also enabled us to study the lncRNAs in the context of their biotype/class. In this work, we have tried to understand the role of divergent lncRNAs as a class during hNPC differentiation into neurons and astrocytes.
There have been several prior publications related to the transcriptional profiling of hNPCs, neurons and astrocytes [Citation29–Citation31]. Several lncRNAs that are specific to these differentiation states have been identified, and their function elucidated [Citation11]. This is true for hNPCs and all the above cell types. The authors have also kindly deposited their data including microarray and RNA-seq data in publically accessible databases. We have built upon their work by utilizing the contents of these databases for identifying specific signatures, especially for divergent lncRNAs. We focused mainly on the divergent biotype (XH) lncRNAs out of various biotypes due to their reported involvement in developmental and regulatory processes. Hence, in this study, we have identified many lineage-specific DELs from the databases. The experimental verification of some of these DELs was done by employing primary cultures of hNPCs and differentiated hNPCs.
When lncRNAs expressing in human and murine embryonic stem cells were mapped to their genomic origin, a large fraction of these transcripts (>60%) has been previously found to be divergent lncRNAs [Citation5]. This fraction was not only expressed in ESCs, but a large fraction of these divergent lncRNAs and associated mRNA were in coordinated transcription during ESCs differentiation. In our study, we observe that many divergent lncRNAs have overlapped with the genes that have important roles during hNPCs pluripotency and its differentiation. These divergent lncRNA/coding genes include such as SOX2, NEUROD1, LHX5, DUSP26, and SYNGR3. The experimental validation of a few such has been reported. Knockdown of sense-antisense pair encoded by locus 6p22.3 (CAPN10 associated lncRNA locus959) had led to perturbation of retinoic-acid induced neuronal differentiation of SHSY-5Y cells [Citation32]. In another example of the divergent lncRNA, Evx1as was shown to regulate mesendodermal differentiation by promoting the transcription of its neighboring gene, EVX1 [Citation5]. Divergent lncRNAs have also been shown to be involved in neurodevelopmental disorder [Citation10].
Activation or repression of genes during neurodevelopment is governed by the epigenetic regulation of chromatin structures. Particularly, DNA methylation has an important role in the neurodevelopment and also is essential for the fate determination of NSCs [Citation33,Citation34]. However, the nature of epigenetic modification near divergent or any other biotypes of lncRNAs as a group in pluripotency and differentiation of NPCs has yet to be identified. In this study, we have investigated the epigenetic changes such as H3K4me3 (activation mark) and H3K27me3 (repression marks) during hNPCs neuronal and astrocytic differentiation. Interestingly, after epigenetic analysis of DELs, we found significant difference only in ChIP scores of H3K4me3 (activation histone mark) but not in H3K27me3 (suppression histone mark) both for upregulated and downregulated DELs. This suggests a specific role of activation of promoter activity of lncRNAs in hNPCs differentiation. Divergent lncRNAs have earlier been shown to have a role in both activations as well as suppression of coding gene transcription [Citation35]. Further, to understand the impact of epigenetics on DELs, we studied the number of DELs showing the presence of epigenetic markers on their promoters. Surprisingly we found that about ~81% of neuronal differentiation DELs have H3K4me3 markers as compared to astrocytic differentiation which has only around 36–39%. The significantly higher number of epigenetic H3Kme3 mark on DELs of differentiated neurons shows its greater involvement of divergent lncRNAs on neuronal differentiation as compared to astrocytic differentiation.
DNase I hypersensitive sites (DHSs) represent regions of relatively open chromatin (euchromatin) that are associated with the binding of cell-specific transcriptional factors. Euchromatinization is important to maintain cell identity and is specific to the cell type. The DNAase-seq signature of DELs appears to have a different pattern from the overall DNAase-seq following differentiation. The overall extent of euchromatization is highest in hNPCs, followed by ESCs, neurons and then astrocytes. However, when it comes to euchromatization associated with DELs, it is highest in neurons. These are somewhat higher than hNPCs, followed by ESCs (markedly lower) and the lowest is again in astrocytes. This further supports the preferential role of DELs during neuronal, as opposed to astrocytic, differentiation.
Co-expression network analysis is a measure of the extent the expression (either upregulation or downregulation) of genes in a large dataset is associated with each other. The basic assumption is that genes that have coordinately associated expression are also functionally linked. Co-expression gene network analysis has been proved very important in identifying signature transcriptional module in brain disorders and neurodevelopment [Citation36]. Each divergent lncRNAs is linked to coding genes, which then affects cellular functionality. Hence, network analysis of a set of coding genes (that are linked to differentially expressed divergent lncRNAs), would indicate whether divergent lncRNAs are important for the regulation of the cellular process. While this is a study of association, it provides important clues to the study of functionality. Hence, we did network co-expression analysis of DELs during neuronal and astrocytic differentiation of hNPCs. We observed an increase in the clustering coefficient and network density of differentially expressed divergent lncRNAs associated genes during neuronal differentiation, which substantiates our other results showing the importance of this biotype of lncRNAs in the process. However, there was no change in cluster coefficient or network density in divergent DELs during astrocytic differentiation. This led us to analyze the change in a network of other biotypes of DELs during astrocytic differentiation. Interestingly, we observed a sharp decrease in clustering coefficient of sense downstream (SD) biotypes (with no change in other biotypes) during astrogliogenesis. This can be interpreted that a network of SD biotypes broken down during astrocytic differentiation. This is only a preliminary analysis and needs to be further substantiated. However, the results of the network analysis of both neuronal and astrocytic differentiation point out the possibility of biotype specific regulation during cellular differentiation. This further support the other analyses performed during the study.
In conclusion, we were able to identify many neuronal or astrocytic specific divergent lncRNAs associated with differentiation along the neuronal/astroglial lineage by using an integrated approach, combining differential gene expression, ChIP-seq of regulatory methylation marks, DNase-seq and network analysis. These different approaches all indicate that the role of divergent lncRNAs is more specific and evident during neuronal differentiation of hNPCs. This is clearly indicated by the convergence of the results of different forms of analysis of publically available datasets can be useful for the study of specific roles of lncRNA biotypes. Our work also points out to the role of another biotype, the SD biotype, during the differentiation of hNPCs to astrocytes. This, however, has to be confirmed by further in-depth analysis. Our work also indicated that the study of the coordinated regulation of lncRNA biotypes could reveal further insights into their role in cellular differentiation. It also highlights that the extensive resources available in publically accessible databases and their analysis could increase in our understanding of different biological processes.
Author Contribution
SS and PS conceived and directed this study. PS coordinated the hNPC work and BP, MKS, SS and PS designed, performed and analysis of cell culture work. BP, Mena F, MKS, TD, PM performed bioinformatics work. BP, SS, Mena F wrote the manuscript with the collaboration of coauthors. Mahar F, RB performed critical editing in manuscript. All authors discussed the results and commented on the manuscript.
Supplemental Material
Download Zip (16 MB)Acknowledgments
We thank Mr. P Manish of NBRC for his technical assistance. The project was supported by core funds from NBRC, and a research grant of the Cognitive Science Research Initiative, Department of Science and Technology (DST), India to PS and SS. BP has received a fellowship from CSIR-UGC, India and NBRC core. SS is a recipient of J C Bose National Fellowship of the DST. We gratefully acknowledge the NGS facility at CSIR-IGIB.
Dr. Mahar Fatima is presently employed as a post-doctoral fellow at the Department of Molecular, Cellular and Developmental Biology, University of Michigan, USA. Mena Fatma is currently pursuing her doctoral research at the Department of Psychiatry and Neurosciences, Laval University, Quebec, CANADA.
Disclosure statement
The authors report no potential conflicts of interest.
Supplementary material
Supplemental data can be accessed here.
Additional information
Funding
References
- Cao J. The functional role of long non-coding RNAs and epigenetics. Biol Proced Online. 2014;16:11. PubMed PMID: 25276098; PubMed Central PMCID: PMC4177375.
- Jia H, Osak M, Bogu GK, et al. Genome-wide computational identification and manual annotation of human long noncoding RNA genes. Rna. 2010 Aug;16(8):1478–1487. PubMed PMID: 20587619; PubMed Central PMCID: PMC2905748.
- Luo S, Lu JY, Liu L, et al. Divergent lncRNAs regulate gene expression and lineage differentiation in pluripotent cells. Cell Stem Cell. 2016 May 5;18(5):637–652. PubMed PMID: 26996597.
- Katayama S, Tomaru Y, Kasukawa T, et al. Antisense transcription in the mammalian transcriptome. Science. 2005 Sep 2;309(5740):1564–1566. PubMed PMID: 16141073.
- Sigova AA, Mullen AC, Molinie B, et al. Divergent transcription of long noncoding RNA/mRNA gene pairs in embryonic stem cells. Proc Natl Acad Sci U S A. 2013 Feb 19;110(8):2876–2881. PubMed PMID: 23382218; PubMed Central PMCID: PMC3581948.
- Magistri M, Faghihi MA, St Laurent G 3rd, et al. Regulation of chromatin structure by long noncoding RNAs: focus on natural antisense transcripts. Trends Genet. 2012 Aug;28(8):389–396. PubMed PMID: 22541732; PubMed Central PMCID: PMC3768148.
- Cabili MN, Trapnell C, Goff L, et al. Integrative annotation of human large intergenic noncoding RNAs reveals global properties and specific subclasses. Genes Dev. 2011 Sep 15;25(18):1915–1927. PubMed PMID: 21890647; PubMed Central PMCID: PMC3185964.
- Bonaguidi MA, Wheeler MA, Shapiro JS, et al. In vivo clonal analysis reveals self-renewing and multipotent adult neural stem cell characteristics. Cell. 2011 Jun 24;145(7):1142–1155. PubMed PMID: 21664664; PubMed Central PMCID: PMC3124562.
- Dinger ME, Amaral PP, Mercer TR, et al. Long noncoding RNAs in mouse embryonic stem cell pluripotency and differentiation. Genome Res. 2008 Sep;18(9):1433–1445. PubMed PMID: 18562676; PubMed Central PMCID: PMC2527704.
- D’Haene E, Jacobs EZ, Volders PJ, et al. Identification of long non-coding RNAs involved in neuronal development and intellectual disability. Sci Rep. 2016 Jun20;6:28396. PubMed PMID: 27319317; PubMed Central PMCID: PMC4913242.
- Ramos AD, Andersen RE, Liu SJ, et al. The long noncoding RNA Pnky regulates neuronal differentiation of embryonic and postnatal neural stem cells. Cell Stem Cell. 2015 Apr 2;16(4):439–447. PubMed PMID: 25800779; PubMed Central PMCID: PMC4388801.
- He D, Wang J, Lu Y, et al. lncRNA functional networks in oligodendrocytes reveal stage-specific myelination control by an lncOL1/Suz12 complex in the CNS. Neuron. 2017 Jan 18;93(2):362–378. PubMed PMID: 28041882; PubMed Central PMCID: PMC5600615.
- Dong X, Chen K, Cuevas-Diaz Duran R, et al. Comprehensive identification of long non-coding RNAs in purified cell types from the brain reveals functional LncRNA in OPC fate determination. PLoS Genet. 2015 Dec;11(12):e1005669. PubMed PMID: 26683846; PubMed Central PMCID: PMC4980008.
- Mercer TR, Qureshi IA, Gokhan S, et al. Long noncoding RNAs in neuronal-glial fate specification and oligodendrocyte lineage maturation. BMC Neurosci. 2010 Feb5;11:14.. PubMed PMID: 20137068; PubMed Central PMCID: PMC2829031.
- Zhang Y, Sloan SA, Clarke LE, et al. Purification and characterization of progenitor and mature human astrocytes reveals transcriptional and functional differences with mouse. Neuron. 2016 Jan 6;89(1):37–53. PubMed PMID: 26687838; PubMed Central PMCID: PMC4707064.
- Agostini M, Romeo F, Inoue S, et al. Metabolic reprogramming during neuronal differentiation. Cell Death Differ. 2016 Sep 1;23(9):1502–1514. PubMed PMID: 27058317; PubMed Central PMCID: PMC5072427.
- Magistri M, Khoury N, Mazza EM, et al. A comparative transcriptomic analysis of astrocytes differentiation from human neural progenitor cells. Eur J Neurosci. 2016 Nov;44(10):2858–2870. PubMed PMID: 27564458; PubMed Central PMCID: PMC5118072.
- Dritsoula A, Papaioannou I, Guerra SG, et al. The molecular basis for dysregulated activation of NKX2-5 in vascular remodelling of systemic sclerosis. Arthritis Rheumatol. 2018 Jan 17. DOI:10.1002/art.40419 PubMed PMID: 29342503.
- Fatima M, Kumari R, Schwamborn JC, et al. Tripartite containing motif 32 modulates proliferation of human neural precursor cells in HIV-1 neurodegeneration. Cell Death Differ. 2016 May;23(5):776–786. PubMed PMID: 26586575; PubMed Central PMCID: PMC4832097.
- Fatima M, Prajapati B, Saleem K, et al. Novel insights into role of miR-320a-VDAC1 axis in astrocyte-mediated neuronal damage in neuroAIDS. Glia. 2017 Feb;65(2):250–263. PubMed PMID: 27761954.
- Zheng GQ, Li Y, Gu Y, et al. Beyond water channel: aquaporin-4 in adult neurogenesis. Neurochem Int. 2010 Apr;56(5):651–654. PubMed PMID: 20138100.
- Kong H, Fan Y, Xie J, et al. AQP4 knockout impairs proliferation, migration and neuronal differentiation of adult neural stem cells. J Cell Sci. 2008 Dec 15;121(Pt 24):4029–4036. PubMed PMID: 19033383.
- Zhao Y, Sheng HZ, Amini R, et al. Control of hippocampal morphogenesis and neuronal differentiation by the LIM homeobox gene Lhx5. Science. 1999 May 14;284(5417):1155–1158. PubMed PMID: 10325223.
- Kong H, Sha LL, Fan Y, et al. Requirement of AQP4 for antidepressive efficiency of fluoxetine: implication in adult hippocampal neurogenesis. Neuropsychopharmacol. 2009 Apr;34(5):1263–1276. PubMed PMID: 18923397.
- Pertea M, Kim D, Pertea GM, et al. Transcript-level expression analysis of RNA-seq experiments with HISAT, StringTie and Ballgown. Nat Protoc. 2016 Sep;11(9):1650–1667. PubMed PMID: 27560171; PubMed Central PMCID: PMC5032908.
- Kim D, Langmead B, Salzberg SL. HISAT: a fast spliced aligner with low memory requirements. Nat Methods. 2015 Apr;12(4):357–360. PubMed PMID: 25751142; PubMed Central PMCID: PMC4655817.
- Ramirez F, Ryan DP, Gruning B, et al. deepTools2: a next generation web server for deep-sequencing data analysis. Nucleic Acids Res. 2016 Jul 8;44(W1):W160–5. PubMed PMID: 27079975; PubMed Central PMCID: PMC4987876.
- Shannon P, Markiel A, Ozier O, et al. Cytoscape: a software environment for integrated models of biomolecular interaction networks. Genome Res. 2003 Nov;13(11):2498–2504. PubMed PMID: 14597658; PubMed Central PMCID: PMC403769.
- Wu JQ, Habegger L, Noisa P, et al. Dynamic transcriptomes during neural differentiation of human embryonic stem cells revealed by short, long, and paired-end sequencing. Proc Natl Acad Sci U S A. 2010 Mar 16;107(11):5254–5259. PubMed PMID: 20194744; PubMed Central PMCID: PMC2841935.
- Lin M, Pedrosa E, Shah A, et al. RNA-Seq of human neurons derived from iPS cells reveals candidate long non-coding RNAs involved in neurogenesis and neuropsychiatric disorders. PloS one. 2011;6(9):e23356. PubMed PMID: 21915259; PubMed Central PMCID: PMC3168439.
- Zhang Y, Chen K, Sloan SA, et al. An RNA-sequencing transcriptome and splicing database of glia, neurons, and vascular cells of the cerebral cortex. J Neurosci. 2014 Sep 3;34(36):11929–11947. PubMed PMID: 25186741; PubMed Central PMCID: PMC4152602.
- Mondal T, Juvvuna PK, Kirkeby A, et al. Sense-antisense lncRNA pair encoded by locus 6p22.3 determines neuroblastoma susceptibility via the USP36-CHD7-SOX9 regulatory axis. Cancer Cell. 2018 Mar 12;33(3):417–434 e7. PubMed PMID: 29533783.
- Chen Y, Ozturk NC, Zhou FC. DNA methylation program in developing hippocampus and its alteration by alcohol. PloS one. 2013;8(3):e60503. PubMed PMID: 23544149; PubMed Central PMCID: PMC3609790.
- Chen Y, Damayanti NP, Irudayaraj J, et al. Diversity of two forms of DNA methylation in the brain. Front Genet. 2014;5:46. PubMed PMID: 24653733; PubMed Central PMCID: PMC3948076.
- Neumann P, Jae N, Knau A, et al. The lncRNA GATA6-AS epigenetically regulates endothelial gene expression via interaction with LOXL2. Nat Commun. 2018 Jan 16;9(1):237. PubMed PMID: 29339785; PubMed Central PMCID: PMC5770451.
- Labonte B, Engmann O, Purushothaman I, et al. Sex-specific transcriptional signatures in human depression. Nat Med. 2017 Sep;23(9):1102–1111. PubMed PMID: 28825715; PubMed Central PMCID: PMC5734943.