ABSTRACT
The study of cancer prognosis serves as an important part of cancer research. Large-scale cancer studies have identified numerous genes and microRNAs (miRNAs) associated with prognosis. These informative genes and miRNAs represent potential biomarkers to predict survival and to elucidate the molecular mechanism of tumour progression. MiRNAs and transcription factors (TFs) can work cooperatively as essential mediators of gene expression, and their dysregulation affects cancer prognosis. A panoramic view of cancer prognosis at the system level, considering the co-regulation roles of miRNA and TF, remains elusive. Here, we establish 12 prognosis-related miRNA-TF co-regulatory networks. The characteristics of prognostic target genes and their regulators in the network are depicted. Although the target genes and co-regulatory patterns exhibit cancer-specific properties, some miRNAs and TFs are highly conserved across cancers. We illustrate and interpret the roles of these conserved regulators by building a model associated with cancer hallmarks, functional enrichment analysis, network community detection, and exhaustive literature research. The elaborated system-level prognostic miRNA-TF co-regulation landscape, including the highlighted roles of conserved regulators, provides a novel and powerful insights into further biological and medical discoveries.
Introduction
Prognostic information is important for clinicians treating patients with cancer; it may inform decisions about reasonable medical interventions and strategies for precision medicine [Citation1,Citation2]. Owing to recent advances in next-generation sequencing technology and its emerging application in various clinical settings, a number of signatures associated with survival outcomes have been extensively investigated. These markers, either genetic or epigenetic, carry various indicative features and clues for further biological and clinical discoveries [Citation3,Citation4].
The regulation of gene expression controls developmental, physiological, and pathophysiological processes in eukaryotic organisms. Associated dysfunction is tightly related to tumorigenesis and progression [Citation5–Citation7]. In the fine-tuned modulation at multiple levels, transcription factors (TFs) and microRNAs (miRNAs) have been recognized to play important roles at the transcriptional and post-transcriptional levels, respectively. The transcriptional program determines cancer phenotype and prognosis by shaping the gene signature in cancer cells [Citation8]. Detectable dysregulated miRNAs in tumour biopsies have readily emerged as promising diagnostic, prognostic and therapeutic indicators [Citation9–Citation11]. In particular, increasing evidence suggests the existence of cooperation and crosstalk between miRNAs and TFs, mainly to buffer gene expression and/or adjust signalling [Citation12]. Specifically, miRNAs and TFs can coordinatively regulate shared target genes in feed-forward loops (FFLs) [Citation13]. Indeed, as recent studies have shown, perturbations of the interwoven regulatory system involving miRNAs and TFs may trigger global alterations in gene expression and affect cancer prognosis (Fig. S1). For example, in colorectal cancer, Mullany et al. found the expression of TFs and their related miRNAs together influence survival [Citation14] and Wang et al. pointed out abnormal expression of two miRNAs (hsa-mir-25 and hsa-mir-31), one TF (BRCA1), and two other genes (ADAMTSL3 and AXIN1) affected patient survival [Citation15]. Fulciniti et al. exhibited the existence of a novel miRNA-TF FFL with a critical role in growth and survival in multiple myeloma [Citation16]. Kong et al. identified an interwoven network of miRNAs and TFs that regulates CD147, a known risk factor for breast cancer associated with poor prognosis in breast cancer patients [Citation17].
The biological network is an integrated and system-level lens through which researchers may uncover the mechanism underlying disease [Citation18]. At the network level, miRNA-TF FFLs are major network motifs (i.e., interconnection patterns that occur more often by chance in biological networks), forming the basic building blocks of the miRNA-TF co-regulatory network [Citation19–Citation21]. Despite substantial efforts to identify the prognostic signatures and potential roles of FFLs in prognosis, an integrative and system-level analysis remains lacking. Hence, we seek to investigate prognostic signatures and the regulatory mechanism behind them in the context of the miRNA-TF co-regulatory network.
In this study, we discerned prognostic FFLs and constructed 12 prognosis-related miRNA-TF co-regulatory networks by integrating cancer genomics data, prognostic signature findings, and interactome data. The characteristics and features of the co-regulatory network are summarized. Through a detailed analysis, we found some miRNAs and TFs are common and conserved during co-regulation of the prognostic network. After considering the conserved regulators, we designed a hierarchical model associated with cancer hallmarks to elucidate the regulatory mechanism affecting cancer prognosis. Detailed enrichment analysis revealed a common theme among prognostic signatures in the co-regulatory network. Several potential prognostic modules were identified inside the miRNA-TF co-regulatory network. A comprehensive survey of the conserved regulators, including literature consultation, was performed to validate and highlight their pan-cancer prognostic functionality. The investigation of prognostic miRNA-TF co-regulatory networks provides novel insight into cancer outcomes, elucidates the commonality among regulatory mechanisms, and offers implications for clinical biomarkers and the study of therapeutics.
Results
Construction of prognostic miRNA-TF co-regulatory networks for human cancers
We developed a five-step pipeline based on the framework in our previous studies [Citation22] in order to build prognosis-related miRNA-TF co-regulatory networks across cancers types.
First, we obtained prognostic genes and miRNAs from the Human Pathology Atlas (HPA) and OncomiR, respectively (). We then used the information provided by 10 established regulatory databases (). In total, 72,801 TF-gene, 178,689 TF-miRNA, 305,858 miRNA-gene interactions were collected. We identified prognostic regulatory interactions whose target nodes or regulator nodes are known to be relevant to prognosis and formed a combinatorial network by merging all interaction types (). Using the network motif detection algorithm, we identified three types of FFLs (TF-FFLs, miRNA-FFLs, and composite-FFLs) in the combinatorial network (). We then constructed the co-regulatory network, which comprises three types of FFLs (Dataset S1) and incorporated expression data from The Cancer Genome Atlas (TCGA) to discern more precise FFL patterns in each network () (Dataset S2). The final information for each prognosis-related network is shown in and Figure S2.
Table 1. Summary of FFLs in 12 prognosis-related co-regulatory networks.
Figure 1. An overview of the computational approach to build prognostic miRNA-TF co-regulatory networks in human cancers. (a) We collected prognosis-related genes and miRNAs for 12 cancers by referring to established databases. (b) Regulatory relationships were obtained from 10 public interactome resources. (c) We screened out prognosis-related regulatory interactions whose target nodes or regulator nodes are known to be relevant to prognosis, forming an entirely synthetic network by merging all interaction types. (d) We then identified three types of FFLs from the combinatorial network using a network motif detection algorithm. (e) We constructed the co-regulatory network which comprises three types of FFLs and incorporated expression data from TCGA to filter out more precise FFL patterns in each network.
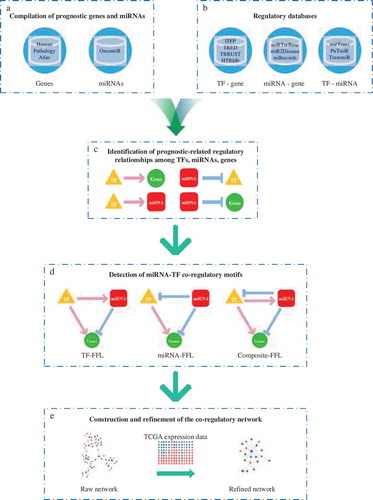
The landscape of prognostic miRNA-TF co-regulatory networks
To assess the topological structure of all 12 co-regulatory networks, we examined the degree distribution of each network (Table S1). The results showed that each prognosis-related co-regulatory network followed the power-law distribution, indicating that these co-regulatory networks had scale-free characteristics, a common feature of most types of biological networks [Citation23].
We investigated the components of co-regulatory networks by examining similarities in FFLs, genes, TFs, and miRNAs across cancer types. In order to measure the pairwise overlap of FFLs, genes, TFs and miRNAs across cancer types, we used two metrics: Fisher’s p-value and the Jaccard index ( and Fig. S3). Little similarity of prognostic FFLs with genes was observed across 12 cancers, suggesting a relatively limited number of common FFLs and genes. In contrast, significant overlap was observed among regulators (i.e., miRNAs and TFs) in the networks. The low conservation of target genes in the prognostic co-regulatory network was concordant with previous studies finding that prognostic genes themselves lack cross-cancer conservation [Citation24,Citation25], which also led to the lack of cross-cancer conservation of FFLs that comprise prognostic genes. However, when we focused on miRNAs and TFs that regulate target genes, some miRNAs and TFs played roles in multiple prognostic co-regulatory networks. For example, ETS1 had a regulatory role in 12 co-regulatory networks; ESR1, MYC, and GATA2 appearing in 11 networks, respectively. These results indicate that conserved regulators impact non-conserved prognostic targets. Based on this FFL pattern, some conserved miRNAs and TFs may influence the cancerous and clinical outcomes for multiple cancers.
Regulators common to multiple prognostic co-regulatory networks
To systematically investigate regulators that acted across networks, miRNAs and TFs were grouped into two categories based on the extent to which a regulator was common across prognostic-related co-regulatory networks. We analysed the distribution of target genes, miRNAs, and TFs in different cancers (). Only 0.19% of target genes were present in >6 cancers; 3.37% miRNAs and 6.09% TFs occurred in ≥7 cancers. We defined ‘common’ regulators (including common miRNAs and common TFs) as miRNAs or TFs that occurred in ≥7 cancer networks (18 TFs and 11 miRNAs). All others were considered to be ‘specific’ regulators. The 18 common TFs and 11 common miRNAs were shown in , and the lists of prognostic target genes and ‘specific regulators’ were recorded in Supplementary datasets S3-S5.
Figure 3. The conserved regulators in prognostic miRNA-TF co-regulatory networks. (a-c) Occurrence of prognostic (a) genes, (b) miRNAs and (c) TFs in 12 co-regulatory networks. (d-e) The identification of common (d) miRNAs and (e) TFs occurred in ≥7 co-regulatory networks.
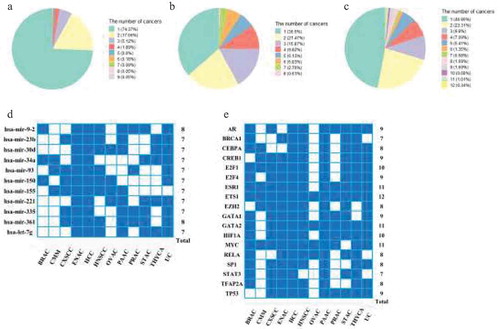
Using this classification, we divided the regulatory elements in each prognostic co-regulator network into two categories. Nodes with high degree (regarded as hub nodes) are known to play important roles in networks. We compared the degree of common vs. specific regulators in each network (). In nine cancers, common TFs were hub nodes with significant high degree, rather than non-common TFs. Common miRNAs had a significant high degree in five cancers. These results highlight the pivotal function of common regulators conserved across prognostic co-regulatory networks. Compared to common TFs, such tendency of common miRNAs was weaker, which may be explained by the relatively weaker regulatory function of miRNAs.
Figure 4. Common regulators may govern and maintain prognostic co-regulatory networks’ architecture across cancers. (a) Common regulators tend to have a higher degree in each network. The left plot shows the node degree comparison of common TFs vs. specific TFs, and the right plot makes a comparison of common miRNAs. (b) Common regulators controlled broad FFLs (left panel) and target genes (right panel) in each network.
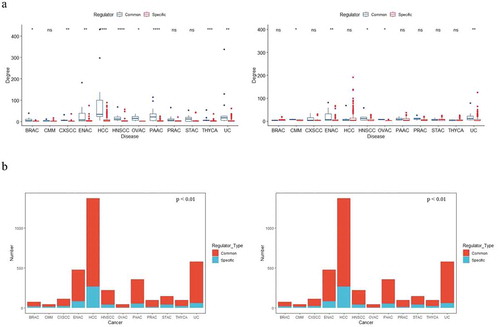
For each co-regulatory network, we further investigated the number of FFLs and target genes containing common regulators (). It is noteworthy that common regulators controlled broad FFLs (p-value <0.01, Wilcoxon test, paired) and target genes (p-value <0.01, Wilcoxon test, paired) in each network. This suggests that common regulators not only affect multiple cancers but also govern broad targets through FFLs in each co-regulatory network. Both of these findings indicate that common regulators may govern and maintain prognostic co-regulatory networks’ architecture across cancers.
A hierarchical model to illustrate the roles of common regulators in cancer prognosis
Despite the daunting complexity and remarkable diversity of neoplastic diseases, several cancer hallmarks contribute to the development of human tumours [Citation26,Citation27]. Each cancer hallmark represents the biological capability for oncogenic progress that underlies [Citation28] the tumour phenotype. Cancer hallmark genes also have a tight and subtle relationship with cancer prognosis [Citation29]. After considering the important role of common regulators in the co-regulatory network, we designed a hierarchical model related to prognosis in order to elucidate the contribution of common regulators to the development of cancer and the effect of prognostic genes on cancer biology ().
Figure 5. A hierarchical model associated with cancer hallmarks. (a) A hierarchical model considering cancer hallmarks to comprehend the functions of common regulators in cancer prognosis. (b) Heatmaps containing the number of target genes (under the control of common regulators), common TFs and common miRNAs that link to cancer hallmarks across cancer types.
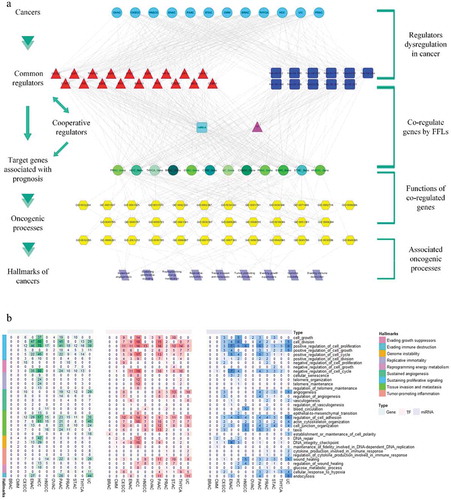
This model lays out a hierarchy for 12 major cancers, common regulators, the cooperative regulators of common regulators, target prognostic genes, annotated GO terms related to biological progress, and nine cancer hallmarks. Common regulators (top layer), govern prognostic target genes directly or with the help of their cooperative miRNAs/TFs, in an FFL pattern. These prognostic genes are enriched in specific GO (p-value <0.05, Dataset S6) and GO terms are correlated with various cancer hallmarks, demonstrating the vivid interaction between cancer hallmarks and clinical outcomes.
We next enumerated the common regulators and target genes linked to each GO term related to cancer hallmarks, across diverse cancer types (). We found that common regulators and target prognostic genes tended to appear in cancer hallmarks for ‘sustaining proliferative signaling’ and for ‘tissue invasion and metastasis’. Sustaining proliferative signalling plays a fundamental role in cancer. Cancer cells display autonomous, chaotic growth because of dysregulated growth signals. Tissue invasion and metastasis are known to be associated with the progression of carcinoma [Citation30,Citation31]. Our model suggests that the production and release of growth-promoting signals and the invasion-metastasis cascade may play key driver roles, reflecting the organizing principle of common regulators with respect to cancer prognosis.
We sought to further elucidate the biological role of common regulators and target genes in the model. Pathway enrichment analysis was performed for common TFs, common miRNAs, and prognostic genes under the control of common regulators (p-value <0.05, Fig S4 and S). In addition to pathways related to specific cancers, the cell cycle is the most shared enriched pathway for common regulators and their targets. Disturbance of the cell cycle has already been proven significant in the prognosis of several cancers [Citation32,Citation33]. Furthermore, pathways that target genes enriched, such as focal adhesion, TGF-ß and p53 may be significant for prognosis [Citation34–Citation37]. While pathways such as ERBB signalling and regulation of the actin cytoskeleton are previously less characterized pathways in prognosis. The results of pathway enrichment analysis may be used to identify additional regulators and genes related to cancer prognosis.
miRNA-TF cooperative modules as prognostic biomarkers in multiple cancer types
Networks present modular structure, and decomposition of the network is beneficial for the elucidation of complex systems [Citation38]. Compared with individual genes, module biomarkers are more powerful predictors of prognosis [Citation39]. Given the characteristics of pan-cancer and core roles inside each network, we are interested in finding modules that comprise common regulators associated with the survival of cancer patients.
We used the GLay community detection algorithms to decompose each prognostic co-regulatory network. In total, 115 modules were identified (Dataset S7). The results of survival analysis for each module identified 32 modules that could predict overall survival (log-rank test, p < 0.05); 23 out of 32 of these modules comprised common regulators (Dataset S8).
We focused on two significant modules of cervical cancer (CXSCC), a common gynaecological cancer. The two modules comprised the common regulators MYC and GATA2 (Fig S6), and the elements contained in the two modules are closely related to cancer progression. Amplification and overexpression of MYC are related to CXSCC progression and GATA2 mutations cause a multifaceted disorder [Citation40,Citation41].In the first module, GATA2 regulates both hsa-mir-30e and the target genes IL1A and ITGA5, with the hsa-mir-30e repressing the target genes. The target IL1A can promote tumour growth, invasion and migration [Citation42] and the ITGA5 expression is induced in transformed epithelial cells during epithelial to mesenchymal transition (EMT) process which fuels metastasis by endowing cells with enhanced migratory and invasive potential [Citation43–Citation45]. In the second module, MYC and hsa-mir-342 control a joint target TFRC. Hsa-mir-342 has the potential to suppress cell proliferation, migration and invasion of human cervical cells [Citation46]. Clinical data have shown that high TFRC expression in cervical cancers is related to advanced clinical pathologic characteristics, and the TFRC is also an independent predictor for survival in cervical cancer [Citation47].
The above results showed that larger clusters of FFLs may play a role in prognosis stratification. Furthermore, common regulators may affect prognosis in larger modules.
A comprehensive survey of common regulators
After establishing the significant roles of common regulators, we investigated the association between common regulators and pan-cancer signatures (). We collected four consensus lists of pan-cancer gene data [Citation48–Citation51]. Notably, the overlap result showed that 14 out of 18 common TFs have been identified as pan-cancer genes. The most notable TF is EZH2, which occurred in all four data sets. Although current studies do not provide enough data on pan-cancer miRNAs, the overlap between common miRNA and two pan-cancer-related miRNA datasets [Citation52,Citation53], namely ‘Pan-cancer miRNA superfamily’ and ‘SDEmiRNA’, showed that common miRNA hsa-mir-93 is oncogenic in multiple cancers. These results suggest the dual function of several TFs and miRNAs in oncogenesis and prognosis.
Figure 6. Overlap between common regulators and pan-cancer signatures. (a) A Venn diagram showing the overlap between common miRNAs and two pan-cancer miRNAs datasets, namely pan-cancer miRNA superfamily and SDEmiRNA. (b) A Venn diagram showing the overlap between common TFs and four pan-cancer gene datasets, namely Cosmic, Cancer5000, Netsig5000 and IntOGen.
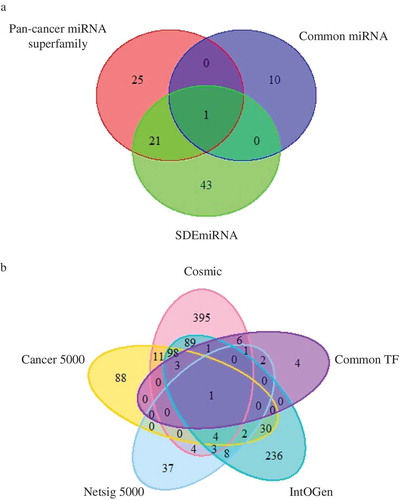
We then carried out a detailed literature survey of common regulators. We searched the PubMed database with keywords including ‘prognosis’, ‘prognostic’, ‘survival’, and ‘clinical outcome’ for each common regulator that we found. We manually extracted analyses related to cancer. As a result, we consulted about 130 published studies describing the associations between common regulators and cancer prognosis (, ). Common TFs are reportedly associated with the prognosis of 3–7 cancers. Remarkably, the most common TFs reported are ETS1 and EZH2; the former is a common TF that plays roles in 12 co-regulatory networks, while the latter is the significant one who has a dual function in both oncogenesis and prognosis as noted before. The most heavily studied prognostic common miRNA is hsa-mir-34a, which is related to 18 cancers. Common miRNAs such as hsa-mir-9–2, hsa-mir-23b, and hsa-mir-361 have not previously been investigated, and further study will be necessary to verify their pan-cancer prognostic potential. These findings validate and support the pan-cancer prognostic functionality of conserved regulators.
Table 2. Published studies describing the associations between common TFs and cancer prognosis.
Table 3. Published studies describing the associations between common miRNAs and cancer prognosis.
Discussion
In the present study, integrated data and network-based methods were used to identify miRNA-TF cooperative events for cancer prognosis. Twelve prognosis-related co-regulatory networks were identified by our multi-step pipeline. Since the incorporation of multi-omics data, prognostic signatures, mechanistic regulatory information, and careful refinement in the pipeline of network construction, the prognostic miRNA-TF co-regulatory network is powerful and reliable. MiRNAs and TFs may jointly regulate gene expression in the form of FFLs, which impact many aspects of cellular processes and disease progression. The miRNA-TF co-regulatory network brings a system-level heuristic view of gene expression regulation to cancer prognosis. A panoramic view of the functional networks may help to characterize prognostic targets and conserved regulators. We observed that common regulators maintain the structure of the co-regulatory network. This motivates us to move the focus from heterogeneous prognostic genes to their regulators – especially conservative regulators. MiRNAs and TFs have been treated as diagnostic, prognostic, and therapeutic objects. Evidence shows that therapies targeting TFs constitute an important part of the most commercially successful drugs approved by the US Food & Drug Administration. A broader effect was found when therapies targeting miRNAs and TFs were compared with those targeting a single gene [Citation54].
Although the prognosis of cancer is as complex as cancer itself, the results presented above pertaining to common regulators demonstrate the cascaded regulatory principle among regulators, prognostic targets, and cancer hallmarks. We note that ‘sustaining proliferative signaling’ and ‘tissue invasion and metastasis’ are vital to cancer prognosis as cancer hallmarks. Pathways enrichment analysis and network community detection were used to elucidate the biological and topological roles of conserved regulators. Finally, we conducted a comprehensive survey of common regulators, emphasizing evaluation of the pan-cancer prognostic function of conserved regulators.
Conclusion
In this study, we investigated 12 miRNA-TF co-regulatory networks in the context of cancer prognosis, in order to elucidate prognostic signatures and the regulatory mechanism behind them. This network-based study highlights conservative regulators (beyond the prognostic genes that vary across cancers), highlighting the clinical importance of regulatory mechanisms in prognosis. We hope our work opens new avenues for the study of cancer prognosis and accelerates the development of precision medicine.
Materials and methods
Collection of prognostic miRNAs and genes
In this study, we focused our analysis on 12 tumour types. We used open resources to obtain the genes and miRNAs for 12 major clinical cancer outcomes. Prognostic genes were identified using the Human Pathology Atlas [Citation29]. MiRNAs associated with clinical outcomes were identified using OncomiR [Citation55] (significance threshold:0.05) (Table S2).
Regulatory relationships among miRNAs, TFs, and target genes
Regulatory relationships among miRNAs, TFs, and target genes were determined from public databases, as follows: (i) TF-gene: ITFP, TRED, TRRUST, HTRIdb [Citation56–Citation59]; (ii) miRNA-gene: miRTarBase, miR2Disease, miRecords [Citation60–Citation62]; (iii) TF-miRNA: mirTrans, PuTmiR, TransmiR [Citation63–Citation65] (Table S3). In this paper, the term ‘TF’ refers specifically to TF genes; the term ‘gene’ includes both TF and non-TF genes; ‘target gene’ refers to only non-TF genes. We unified TF/miRNA/target gene symbols in the regulatory relationship by referring the Hugo Gene Nomenclature Committee (HGNC) [Citation66], the approval TF list [Citation67,Citation68], and miRBase [Citation69].
Omics data across multiple cancers
We capitalized on expression data from TCGA [Citation70] to filter more precise co-regulatory interactions. The TCGA clinical data were used for survival analysis of network clusters (Table S4). The R package RTCGAToolbox [Citation71] was used to assess TCGA data (run date Jan. 2016) provided in the Firehose data repository and to perform survival analysis. The RNA-Seq expression values were transformed by the log2(x + 1) transformation, where ‘x’ is the original expression value. These values were used for subsequent analyses.
Network motif detection
Based on the collected prognostic signatures and regulatory relationships, we filtered out prognostic regulatory interactions whose target nodes or regulator nodes are known to be relevant to prognosis. We then pooled the prognostic regulatory relationships including TF-gene, TF-miRNA and miRNA-gene, generating a combinational network. Using a high-efficiency FANMOD algorithm for network motif detection [Citation72], we detected three types of three-node FFLs: TF-FFLs, miRNA-FFLs, and composite-FFLs and formed the raw co-regulatory network.
Prognostic co-regulatory network construction and refinement
The preliminary co-regulatory network comprises three types of FFLs. A single TF-FFL has a master TF that regulates a partner miRNA and their joint target. A miRNA-FFL contains a master miRNA regulator, which represses its partner TF and their joint target gene. In a composite-FFL, the miRNA and TF regulate each other, thereby controlling their joint target.
We used TCGA expression data to discern more precise co-regulatory interactions in the raw co-regulatory network. We calculated pairwise Spearman correlation values among TFs, miRNAs, and genes for each FFL in the raw co-regulatory network. For TF-genes and TF-miRNA pairs, we retained p < 0.05 as the level of statistical significance. For miRNA-gene, we retained p < 0.05 and correlation coefficient <0, because most miRNAs are assumed to inhibit the expression of their targets. We removed less-significant FFLs from the raw co-regulatory networks in order to yield the final prognosis-related co-regulatory networks.
Classification of network regulators
For systematic analysis of the regulators in co-regulatory networks, we split the intra-network miRNAs and TFs into two groups: (i) common regulators: miRNAs or TFs that occurred in ≥7 co-regulatory networks; (ii) specific regulators: regulators with frequency <7 across 12 networks.
Network visualization, topological measurements, and identification of network modules
The miRNA-TF co-regulatory networks and the cancer hallmark-associated model were visualized with Cytoscape 3.7.0 [Citation73]. Topological measurements of the networks were obtained using the NetworkAnalyzer plugin for Cytoscape. We used the GLay community clustering algorithm to generate clusters from large complex networks [Citation74].
Functional annotation and enrichment analysis
The R/Biocondutor software ClusterProfiler [Citation75] package was used for enrichment analysis. Data from MSigDB(v6.2) [Citation76], miEAA (release date Apr.2016) [Citation77] were utilized in enrichment analysis. Specifically, we selected MSigDB GO gene sets for GO enrichment analysis, and chose MSigDB KEGG gene sets for pathway enrichment analysis. We utilized miEAA pathway annotation to perform miRNA pathway enrichment analysis. To build a linkage between target genes and cancer hallmarks, we referred to a previous study [Citation78] to determine a list of GO terms related to the hallmarks (Table S5).
Disclosure of potential conflicts of interest
No potential conflicts of interest were disclosed.
Supplemental Material
Download Zip (10.2 MB)Supplementary material
Supplemental data for this article can be accessed here.
Additional information
Funding
References
- Gwilliam B, Keeley V, Todd C, et al. Development of prognosis in palliative care study (PiPS) predictor models to improve prognostication in advanced cancer: prospective cohort study. BMJ. 2011 Aug 25;343:d4920. PubMed PMID: 21868477; PubMed Central PMCID: PMCPMC3162041.
- Li J, Lenferink AE, Deng Y, et al. Identification of high-quality cancer prognostic markers and metastasis network modules. Nat Commun. 2010 Jul 13;1:34. PubMed PMID: 20975711; PubMed Central PMCID: PMCPMC2972666. eng.
- Gentles AJ, Newman AM, Liu CL, et al. The prognostic landscape of genes and infiltrating immune cells across human cancers. Nat Med. 2015;21(8):938–945.
- Subramanian J, Simon R. What should physicians look for in evaluating prognostic gene-expression signatures? Nat Rev Clin Oncol. 2010 Jun;7(6):327–334. PubMed PMID: 20421890; eng.
- Andrews E, Wang Y, Xia T, et al. Contextual Refinement of Regulatory Targets Reveals Effects on Breast Cancer Prognosis of the Regulome. PLoS Comput Biol. 2017 Jan;13(1):e1005340. PubMed PMID: 28103241; PubMed Central PMCID: PMCPMC5289608. eng.
- Defoort J, Van de Peer Y, Vermeirssen V. Function, dynamics and evolution of network motif modules in integrated gene regulatory networks of worm and plant. Nucleic Acids Res. 2018 Jul 27;46(13):6480–6503. PubMed PMID: 29873777; PubMed Central PMCID: PMCPMC6061849. eng.
- Mei S, Meyer CA, Zheng R, et al. Cistrome Cancer: A Web Resource for Integrative Gene Regulation Modeling in Cancer. Cancer Res. 2017 Nov 1;77(21):e19–e22. PubMed PMID: 29092931; PubMed Central PMCID: PMCPMC5826647. eng.
- Shi L, Wang Y, Lu Z, et al. miR-127 promotes EMT and stem-like traits in lung cancer through a feed-forward regulatory loop. Oncogene. 2017 Mar 23;36(12):1631–1643. PubMed PMID: 27869168; eng.
- Beermann J, Piccoli MT, Viereck J, et al. Non-coding RNAs in Development and Disease: background, Mechanisms, and Therapeutic Approaches. Physiol Rev. 2016 Oct;96(4):1297–1325. PubMed PMID: 27535639; eng.
- Nassar FJ, Nasr R, Talhouk R. MicroRNAs as biomarkers for early breast cancer diagnosis, prognosis and therapy prediction. Pharmacol Ther. 2017 Apr;172:34–49. PubMed PMID: 27916656; eng.
- Shigeyasu K, Toden S, Zumwalt TJ, et al. Emerging Role of MicroRNAs as Liquid Biopsy Biomarkers in Gastrointestinal Cancers. Clin Cancer Res. 2017 May 15;23(10):2391–2399. 10.1158/1078-0432.ccr-16-1676. PubMed PMID: 28143873; PubMed Central PMCID: PMCPMC5433899. eng.
- Bracken CP, Scott HS, Goodall GJ. A network-biology perspective of microRNA function and dysfunction in cancer. Nat Rev Genet. 2016 Dec;17(12):719–732. PubMed PMID: 27795564; eng.
- Jiang W, Mitra R, Lin CC, et al. Systematic dissection of dysregulated transcription factor-miRNA feed-forward loops across tumor types. Brief Bioinform. 2016 Nov;17(6):996–1008. PubMed PMID: 26655252; PubMed Central PMCID: PMCPMC5142013. eng.
- Mullany LE, Herrick JS, Wolff RK, et al. Transcription factor-microRNA associations and their impact on colorectal cancer survival. Mol Carcinog. 2017 Nov;56(11):2512–2526. PubMed PMID: 28667784; PubMed Central PMCID: PMCPMC5633497. eng.
- Wang H, Luo J, Liu C, et al. Investigating MicroRNA and transcription factor co-regulatory networks in colorectal cancer. BMC Bioinformatics. 2017 Sep 2;18(1):388. PubMed PMID: 28865443; PubMed Central PMCID: PMCPMC5581471. eng.
- Fulciniti M, Amodio N, Bandi RL, et al. miR-23b/SP1/c-myc forms a feed-forward loop supporting multiple myeloma cell growth. Blood Cancer J. 2016 Jan 15;6:e380. PubMed PMID: 26771806; PubMed Central PMCID: PMCPMC4742623. eng.
- Kong LM, Liao CG, Zhang Y, et al. A regulatory loop involving miR-22, Sp1, and c-Myc modulates CD147 expression in breast cancer invasion and metastasis. Cancer Res. 2014 Jul 15;74(14):3764–3778. PubMed PMID: 24906624; eng.
- Furlong LI. Human diseases through the lens of network biology. Trends Genet. 2013 Mar;29(3):150–159. PubMed PMID: 23219555; eng.
- Mangan S, Alon U. Structure and function of the feed-forward loop network motif. Proc Natl Acad Sci U S A. 2003 Oct 14;100(21):11980–11985. PubMed PMID: 14530388; PubMed Central PMCID: PMCPMC218699. eng.
- Zhang HM, Kuang S, Xiong X, et al. Transcription factor and microRNA co-regulatory loops: important regulatory motifs in biological processes and diseases. Brief Bioinform. 2015 Jan;16(1):45–58. PubMed PMID: 24307685; eng.
- Farahani M, Rezaei-Tavirani M, Zali H, et al. Deciphering the transcription factor-microRNA-target gene regulatory network associated with graphene oxide cytotoxicity. Nanotoxicology. 2018 Oct;16:1–13. PubMed PMID: 30325693; eng.
- Li R, Chen H, Jiang S, et al. CMTCN: a web tool for investigating cancer-specific microRNA and transcription factor co-regulatory networks. PeerJ. 2018;6:e5951. PubMed PMID: 30473937; PubMed Central PMCID: PMCPMC6237116. eng.
- Barabasi AL, Oltvai ZN. Network biology: understanding the cell‘s functional organization. Nat Rev Genet. 2004 Feb;5(2):101–113. PubMed PMID: 14735121; eng.
- Wang Y, Goodison S, Li X, et al. Prognostic cancer gene signatures share common regulatory motifs. Sci Rep. 2017 Jul 6;7(1):4750. PubMed PMID: 28684851; PubMed Central PMCID: PMCPMC5500535. eng.
- Anaya J, Reon B, Chen WM, et al. A pan-cancer analysis of prognostic genes. PeerJ. 2015;3:e1499. PubMed PMID: 27047702; PubMed Central PMCID: PMCPMC4815555. eng.
- Hanahan D, Weinberg RA. Hallmarks of cancer: the next generation. Cell. 2011 Mar 4;144(5):646–674. PubMed PMID: 21376230; eng.
- Fouad YA, Aanei C. Revisiting the hallmarks of cancer. Am J Cancer Res. 2017;7(5): 1016–1036. PubMed PMID: 28560055; PubMed Central PMCID: PMCPMC5446472. eng.
- Mesri EA, Feitelson MA, Munger K. Human viral oncogenesis: a cancer hallmarks analysis. Cell Host Microbe. 2014 Mar 12;15(3):266–282. PubMed PMID: 24629334; PubMed Central PMCID: PMCPMC3992243. eng.
- Uhlen M, Zhang C, Lee S, et al. A pathology atlas of the human cancer transcriptome. Science (New York, NY). 2017 Aug 18;357(6352). PubMed PMID: 28818916; eng.
- Hanahan D, Weinberg RA. The hallmarks of cancer. Cell. 2000 Jan 7;100(1):57–70. PubMed PMID: 10647931; eng.
- Sasahira T, Kirita T. Hallmarks of cancer-related newly prognostic factors of oral squamous cell carcinoma. Int J Mol Sci. 2018 Aug 16;19(8). PubMed PMID: 30115834; PubMed Central PMCID: PMCPMC6121568. eng.
- Williams GH, Stoeber K. The cell cycle and cancer. J Pathol. 2012 Jan;226(2):352–364. PubMed PMID: 21990031; eng.
- Dominguez D, Tsai YH, Gomez N, et al. A high-resolution transcriptome map of cell cycle reveals novel connections between periodic genes and cancer. Cell Res. 2016 Aug;26(8):946–962. PubMed PMID: 27364684; PubMed Central PMCID: PMCPMC4973334. eng.
- Tsai LH, Chen PM, Cheng YW, et al. LKB1 loss by alteration of the NKX2-1/p53 pathway promotes tumor malignancy and predicts poor survival and relapse in lung adenocarcinomas. Oncogene. 2014 Jul 17;33(29):3851–3860. PubMed PMID: 23995788; eng.
- Kleinschmidt EG, Schlaepfer DD. Focal adhesion kinase signaling in unexpected places. Curr Opin Cell Biol. 2017 Apr;45:24–30. PubMed PMID: 28213315; PubMed Central PMCID: PMCPMC5482783. eng.
- Korkut A, Zaidi S, Kanchi RS, et al. A pan-cancer analysis reveals high-frequency genetic alterations in mediators of signaling by the TGF-beta superfamily. Cell Syst. 2018 Oct 24;7(4):422–437.e7. PubMed PMID: 30268436; eng.
- Sanchez-Vega F, Mina M, Armenia J, et al. Oncogenic signaling pathways in the cancer genome atlas. Cell. 2018 Apr 5;173(2):321–337.e10. PubMed PMID: 29625050; PubMed Central PMCID: PMCPMC6070353. eng.
- Zitnik M, Sosic R, Leskovec J. Prioritizing network communities. Nat Commun. 2018 Jun 29;9(1):2544. PubMed PMID: 29959323; PubMed Central PMCID: PMCPMC6026212. eng.
- Wu G, Stein L. A network module-based method for identifying cancer prognostic signatures. Genome Biol. 2012 Dec 10;13(12):R112. PubMed PMID: 23228031; PubMed Central PMCID: PMCPMC3580410. eng.
- Collin M, Dickinson R, Bigley V. Haematopoietic and immune defects associated with GATA2 mutation. Br J Haematol. 2015 Apr;169(2):173–187. PubMed PMID: 25707267; PubMed Central PMCID: PMCPMC4409096. eng.
- Zhu D, Jiang XH, Jiang YH, et al. Amplification and overexpression of TP63 and MYC as biomarkers for transition of cervical intraepithelial neoplasia to cervical cancer. Int J Gynecological Cancer. 2014 May;24(4):643–648. PubMed PMID: 24662128; eng.
- Huang J, Ni S, Li D, et al. An insertion/deletion polymorphism at miRNA-122 binding site in the IL1A is associated with a reduced risk of cervical squamous cell carcinoma. Genet Test Mol Biomarkers. 2015 Jun;19(6):331–334. PubMed PMID: 25955681; eng.
- Suarez-Carmona M, Lesage J, Cataldo D, et al. EMT and inflammation: inseparable actors of cancer progression. Mol Oncol. 2017 Jul;11(7):805–823. PubMed PMID: 28599100; PubMed Central PMCID: PMCPMC5496491. eng.
- Yun J, Song SH, Kim HP, et al. Dynamic cohesin-mediated chromatin architecture controls epithelial-mesenchymal plasticity in cancer. EMBO Rep. 2016 Sep;17(9):1343–1359. PubMed PMID: 27466323; PubMed Central PMCID: PMCPMC5007572. eng.
- George JT, Jolly MK, Xu S, et al. Survival outcomes in cancer patients predicted by a partial EMT gene expression scoring metric. Cancer Res. 2017 Nov 15;77(22):6415–6428. PubMed PMID: 28947416; PubMed Central PMCID: PMCPMC5690883. eng.
- Li XR, Chu HJ, Lv T, et al. miR-342-3p suppresses proliferation, migration and invasion by targeting FOXM1 in human cervical cancer. FEBS Lett. 2014 Aug 25;588(17):3298–3307. PubMed PMID: 25066298; eng.
- Xu X, Liu T, Wu J, et al. Transferrin receptor-involved HIF-1 signaling pathway in cervical cancer. Cancer Gene Ther. 2019 Jan 17. PubMed PMID: 30651591; eng.
- Forbes SA, Beare D, Boutselakis H, et al. COSMIC: somatic cancer genetics at high-resolution. Nucleic Acids Res. 2017 Jan 4;45(D1):D777–d783. PubMed PMID: 27899578; PubMed Central PMCID: PMCPMC5210583. eng.
- Lawrence MS, Stojanov P, Mermel CH, et al. Discovery and saturation analysis of cancer genes across 21 tumour types. Nature. 2014 Jan 23;505(7484):495–501. PubMed PMID: 24390350; PubMed Central PMCID: PMCPMC4048962. eng.
- Gundem G, Perez-Llamas C, Jene-Sanz A, et al. IntOGen: integration and data mining of multidimensional oncogenomic data. Nat Methods. 2010 Feb;7(2):92–93. PubMed PMID: 20111033; eng.
- Horn H, Lawrence MS, Chouinard CR, et al. NetSig: network-based discovery from cancer genomes. Nat Methods. 2018 Jan;15(1):61–66. PubMed PMID: 29200198; PubMed Central PMCID: PMCPMC5985961. eng.
- Hu Y, Dingerdissen H, Gupta S, et al. Identification of key differentially expressed MicroRNAs in cancer patients through pan-cancer analysis. Comput Biol Med. 2018 Dec 1;103:183–197. PubMed PMID: 30384176; PubMed Central PMCID: PMCPMC6279243. eng.
- Hamilton MP, Rajapakshe K, Hartig SM, et al. Identification of a pan-cancer oncogenic microRNA superfamily anchored by a central core seed motif. Nat Commun. 2013;4:2730. PubMed PMID: 24220575; PubMed Central PMCID: PMCPMC3868236. eng.
- Plaisier CL, O‘Brien S, Bernard B, et al. Causal mechanistic regulatory network for glioblastoma deciphered using systems genetics network analysis. Cell Syst. 2016 Aug;3(2):172–186. PubMed PMID: 27426982; PubMed Central PMCID: PMCPMC5001912. eng.
- Wong NW, Chen Y, Chen S, et al. OncomiR: an online resource for exploring pan-cancer microRNA dysregulation. Bioinformatics. 2018 Feb 15;34(4):713–715. PubMed PMID: 29028907; PubMed Central PMCID: PMCPMC5860608. eng.
- Jiang C, Xuan Z, Zhao F, et al. TRED: a transcriptional regulatory element database, new entries and other development. Nucleic Acids Res. 2007;35(Database issue):D137–D140.
- Zheng G, Tu K, Yang Q, et al. ITFP: an integrated platform of mammalian transcription factors. Bioinformatics. 2008;24(20):2416–2417.
- Bovolenta LA, Acencio ML, Lemke N. HTRIdb: an open-access database for experimentally verified human transcriptional regulation interactions. Bmc Genomics. 2012;13(1):405.
- Han H, Cho JW, Lee S, et al. TRRUST v2: an expanded reference database of human and mouse transcriptional regulatory interactions. Nucleic Acids Res. 2017;46(Databaseissue):D380–D386.
- Jiang Q, Wang Y, Hao Y, et al. miR2Disease: a manually curated database for microRNA deregulation in human disease. Nucleic Acids Res. 2009;37(1):D98–104.
- Xiao F, Zuo Z, Cai G, et al. miRecords: an integrated resource for microRNA–target interactions. Nucleic Acids Res. 2009;37(Databaseissue):D105.
- Chou CH, Shrestha S, Yang CD, et al. miRTarBase update 2018: a resource for experimentally validated microRNA-target interactions. Nucleic Acids Res 2017;46(Databaseissue):D296–D302.
- Hua X, Tang R, Xu X, et al. mirTrans: a resource of transcriptional regulation on microRNAs for human cell lines. Nucleic Acids Res. 2018 Jan 4;46(D1):D168–d174. PubMed PMID: 29077896; PubMed Central PMCID: PMCPMC5753250. eng.
- Bandyopadhyay S, Bhattacharyya M. PuTmiR: a database for extracting neighboring transcription factors of human microRNAs. BMC Bioinformatics. 2010 Apr 15;11:190.
- Tong Z, Cui Q, Wang J, et al. TransmiR v2.0: an updated transcription factor-microRNA regulation database. Nucleic Acids Res. 2019 Jan 8;47(D1):D253–d258. PubMed PMID: 30371815; PubMed Central PMCID: PMCPMC6323981. eng.
- Yates B, Braschi B, Gray KA, et al. Genenames.org: the HGNC and VGNC resources in 2017. Nucleic Acids Res. 2017 Jan 4;45(D1):D619–d625. PubMed PMID: 27799471; PubMed Central PMCID: PMCPMC5210531. eng.
- Ravasi T, Suzuki H, Cannistraci CV, et al. An atlas of combinatorial transcriptional regulation in mouse and man. Cell. 2010 Mar 5;140(5):744–752. PubMed PMID: 20211142; PubMed Central PMCID: PMCPMC2836267. eng.
- Nicolle R, Radvanyi F, Elati M. CoRegNet: reconstruction and integrated analysis of co-regulatory networks. Bioinformatics. 2015 Sep 15;31(18):3066–3068. PubMed PMID: 25979476; PubMed Central PMCID: PMCPMC4565029. eng.
- Kozomara A, Birgaoanu M, Griffiths-Jones S. miRBase: from microRNA sequences to function. Nucleic Acids Res. 2019 Jan 8;47(D1):D155–d162. PubMed PMID: 30423142; PubMed Central PMCID: PMCPMC6323917. eng.
- Katarzyna T, Patrycja C, Maciej W. The Cancer Genome Atlas (TCGA): an immeasurable source of knowledge. Contemp Oncol. 2015;19(1A):68–77.
- Samur MK. RTCGAToolbox: a new tool for exporting tcga firehose data. Plos One. 2014;9(9):e106397.
- Wernicke S, Rasche F. FANMOD: a tool for fast network motif detection. Bioinformatics. 2006;22(9):1152–1153.
- Shannon P, Markiel A, Ozier O, et al. Cytoscape: a software environment for integrated models of biomolecular interaction networks. Genome Res. 2003 Nov;13(11):2498–2504. PubMed PMID: 14597658; PubMed Central PMCID: PMCPMC403769. eng.
- Su G, Kuchinsky A, Morris JH, et al. GLay: community structure analysis of biological networks. Bioinformatics. 2010 Dec 15;26(24):3135–3137. PubMed PMID: 21123224; PubMed Central PMCID: PMCPMC2995124. eng.
- Yu G, Wang LG, Han Y, et al. clusterProfiler: an R package for comparing biological themes among gene clusters. Omics. 2012 May;16(5):284–287. PubMed PMID: 22455463; PubMed Central PMCID: PMCPMC3339379. eng.
- Liberzon A, Subramanian A, Pinchback R, et al. Molecular signatures database (MSigDB) 3.0. Bioinformatics. 2011 Jun 15;27(12):1739–1740. PubMed PMID: 21546393; PubMed Central PMCID: PMCPMC3106198. eng.
- Backes C, Khaleeq QT, Meese E, et al. miEAA: microRNA enrichment analysis and annotation. Nucleic Acids Res. 2016;44(WebServer issue):W110–W116.
- Knijnenburg TA, Bismeijer T, Wessels LF, et al. A multilevel pan-cancer map links gene mutations to cancer hallmarks. Chin J Cancer. 2015 Sep 14;34(10):439–449. PubMed PMID: 26369414; PubMed Central PMCID: PMCPMC4593384. eng.