ABSTRACT
Transfer RNAs (tRNAs) play critical roles in human cancer. Currently, no database provides the expression landscape and clinical relevance of tRNAs across a variety of human cancers. Utilizing miRNA-seq data from The Cancer Genome Atlas, we quantified the relative expression of tRNA genes and merged them into the codon level and amino level across 31 cancer types. The expression of tRNAs is associated with clinical features of patient smoking history and overall survival, and disease stage, subtype, and grade. We further analysed codon frequency and amino acid frequency for each protein coding gene and linked alterations of tRNA expression with protein translational efficiency. We include these data resources in a user-friendly data portal, tRic (tRNA in cancer, https://hanlab.uth.edu/tRic/ or http://bioinfo.life.hust.edu.cn/tRic/), which can be of significant interest to the research community.
KEYWORDS:
Introduction
Transfer RNAs (tRNAs) play critical roles in protein translation by delivering amino acids to initiate and elongate peptide chains [Citation1]. Transcription of tRNAs is mediated by RNA polymerase III, and aberrant tRNA expression contributes to disease [Citation2,Citation3]. For example, overexpression of tRNAiMetCAT (initiator tRNA that identifies a methionyl translation start codon) can enhance global protein synthesis and increase endoplasmic reticulum stress to promote the development of diabetes [Citation4]. Decreased expression of tRNAGlnCTG promotes progression of Huntington’s disease in the early stage by increasing the frequency of translational frame-shifting [Citation5]. In human cancers, enhanced tRNA expression drives mRNA translation and cell growth [Citation6]. For example, expression of tRNAArg in breast cancer is positively correlated with codon frequency in oncogenic signatures, suggesting that tRNAArg overexpression may accelerate the translational efficiency of these oncogenic genes [Citation7–Citation9]. Up-regulation of tRNAGluTTC optimizes EXOSC2 expression to promote metastatic progression of tumours[Citation10].
The Cancer Genome Atlas (TCGA) project generated multi-omic data for more than 10,000 patient samples, including exome-seq, RNA-seq, miRNA-seq, and DNA methylation[Citation11]. It also collected clinical features, including disease stage and patient age and overall survival. These rich data provide valuable opportunities to understand transcriptomic events and oncogenic pathways [Citation12–Citation16]. Several databases have been developed to benefit the biomedical research community in utilizing this large-scale dataset. For example, cBioPortal provides a web resource for exploring, visualizing, and analysing cancer genomic data, especially for protein-coding genes [Citation17,Citation18]. The Cancer Proteome Atlas includes protein expressions of ~200 proteins for > 8,000 tumour samples[Citation19]. PancanQTL was developed to explore both trans-quantitative trait loci (QTL) and cis-eQTL across 33 cancer types[Citation20]. Several other databases focus on non-coding RNAs. For example, The Atlas of Non-coding RNA In Cancer focuses on the functions and clinical relevance of long non-coding RNAs[Citation21], while SnoRNA In Cancer focuses on the expression landscape and clinical relevance of small nucleolar RNAs[Citation22]. However, there is still no tRNA database in cancer, likely due to the technical difficulty of estimating tRNA expression levels accurately from high-throughput sequencing data[Citation23]. Recent studies used miRNA-seq to quantify the relative expression level of tRNAs in multiple organisms, including E.coli, yeast, and humans [Citation24–Citation32]. In particular, we used a similar computational pipeline to quantify the relative expression levels of tRNAs from TCGA[Citation33]. We further built a user-friendly database, tRNA In Cancer (tRic), the first comprehensive database for tRNAs in cancer, which can significantly benefit cancer research.
Results and discussion
Data preparation
We collected clinical information, including stage, grade, subtype, patient survival, and smoking history, from ~10,000 patients across 31 human cancers (). We obtained miRNA seq files for these samples and quantified their expression profile at tRNA, codon and amino acid level as described in our previous study (method and )[Citation33]. We also calculated frequency of codon and amino acid for each coding gene throughout human genomes (). These datasets were deposited in our database.
Figure 1. Data processing and web design of tRic. a. Summary of clinical information across 31 human cancer types in tRic. Full names of cancer type are listed in . b. Data collection and processing of tRic dataset, including miRNAseq, tRNA annotation and human coding sequences (CDS). QC denotes quantify control. c. Interface and infrastructure of tRic.
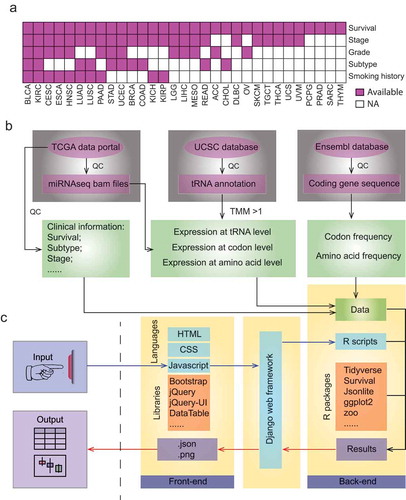
Database infrastructure
The web interface is based on traditional HTML, CSS, and JavaScript with modern libraries, such as Bootstrap and JQuery. The backend of the data portal is based on R and data manipulation libraries, such as Tidyverse. The Django web framework is adopted to connect the backend and frontend of the database (). Users can browse or query items of interest on the user-friendly web pages. We established two mirrored links for tRic at https://hanlab.uth.edu/tRic/ or http://bioinfo.life.hust.edu.cn/tRic/). We will continue to support the database for possible updates.
Functional modules and examples
tRic has four functional modules: tRNA level, codon level, amino acid level, and codon usage (). In the ‘tRNA level’ module, users can query expression level of tRNAs in a specific cancer type and/or subgroup. tRic will return the expression level of tRNAs and differentially expressed tRNAs between tumour and normal samples if there were more than 5 paired samples. For example, tRNA-His-GTG-1–9 is differentially expressed between tumour and normal samples in LUAD (). Users can also choose to perform comprehensive analysis for tRNAs associated with clinical features. For example, tRNA-Arg-TCG-5–1 is associated with patient survival in KIRC (). Expression at tRNA level was merged into codon level and amino acid level. tRic also provides similar query functions in module ‘codon level’ and module ‘amino acid level’ to ‘tRNA level’. For example, tRNAArg(CGT) is differentially expressed among KIRC stages (), while tRNAArg(AGA) is differentially expressed among BRCA subtypes (), tRNAGlu is differentially expressed among patients with different smoking histories in LUSC (), and tRNALeu is differentially expressed among LIHC grades ().
Figure 2. Overview of tRic database. a. Four modules in tRic: expression of tRNs at tRNA level, codon level, and amino acid level, respectively, as well as codon usage. b. Differentially expressed tRNAs between tumour and normal samples. c. Expression of tRNA associated with patient survival. d. Differentially expressed codons among different stages. e. Differentially expressed codons among different subtypes. f. Differentially expressed amino acids among patients with different smoking histories. g. Differentially expressed amino acids among different tumour grades. h. Amino acid frequency of human SRSF2 gene.
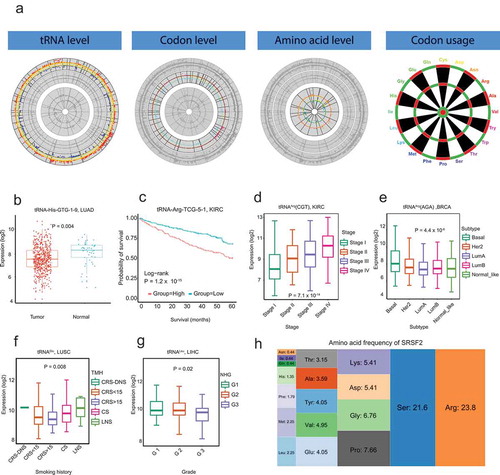
tRNAs play important translation roles in initiating and elongating peptides[Citation1]. Therefore, the expression alterations of tRNA may impact translational efficiency. The module ‘codon usage’ aims to pinpoint potential effects of tRNA expression on protein translation. Users can search a protein-coding gene for its codon frequency and amino acid frequency. For example, Arg frequency in SRSF2 (23.8%) is significantly higher than the average genomic level (5.5%), suggesting that tRNAArg overexpression may increase the translational product of SRSF2 (). Users can also search the gene list with high frequency for specific codons or amino acids.
Data download
Expressions at tRNA, codon, and amino acid levels, as well as the codon and amino acid frequency for all protein-coding genes are available on tRic download pages (https://hanlab.uth.edu/tRic/download/ or http://bioinfo.life.hust.edu.cn/tRic/download/).
Conclusion
We have developed the first comprehensive database for tRNA expression in more than 10,000 tumour samples across 31 cancer types. We provide the tRNA expression profile, differential expression between tumour and normal samples and among different groups of samples (e.g., subtypes, stages) at tRNA, codon and amino acid levels. We also provide the codon frequency and amino acid frequency for all protein-coding genes in the human genome, which may unveil potential connections between tRNA expression and the usage bias of gene translation. Our database will provide the biomedical research community with insights in functional discoveries of tRNAs in cancer.
Materials and methods
Clinical information for TCGA samples
The clinical information of TCGA samples was obtained from TCGA data portal (https://portal.gdc.cancer.gov/). Clinical information for each cancer type, including stage, grade, subtype, and patient survival and smoking history, is summarized in .
Quantification of tRNAs
We downloaded and processed 16,591 miRNA-seq data from TCGA data portal (https://portal.gdc.cancer.gov/) as we previously described[Citation22]. In brief, we filtered out duplicated samples and low-quality samples with quality control-passed reads < 50% or reads mapped rate < 80%. After quality control, 10,594 samples, comprising 9931 tumour samples and 663 normal samples, were included in our study (, , left panel).
Table 1. Summary of tRic data for each cancer type.
We quantified tRNA expression levels as previously described[Citation33]. In brief, we downloaded tRNA annotations from UCSC Genome Browser (http://hgdownload.soe.ucsc.edu/) and filtered out those without clear anticodon and amino acid information. In total, we collected 604 tRNAs decoding 52 anticodons (codons) and 21 amino acids. We then mapped TCGA miRNA-seq reads to tRNA annotations and normalized tRNA expression using the trimmed mean of M values (TMM) method [Citation34,Citation35]. We defined tRNAs that have relatively high expression value (average TMM > 1) as detectable tRNAs. These tRNAs were categorized into 52 codon groups and 21 amino acid groups according to the codon and amino acid information (, middle panel).
Estimation of codon frequency and amino acid frequency
The human coding sequences with complete open reading frames were downloaded from Ensembl database (www.ensembl.org/). For each coding gene, we estimated the frequency for each codon and each amino acid based on the sequence information. At the codon level, we calculated the total number of codons (N) and then calculated the total number of each specific codon (n). The codon frequency is calculated as N divided by n. We used a similar approach to calculate the amino acid frequency (, right panel).
Statistical analyses
All statistical tests were performed using R. We used the Student’s t-test to examine the differential expression between tumour and normal samples. The analysis of variance test was used to test differentially expressed tRNAs among different stages, subtypes, grades, and smoking history groups. The univariate Cox model was used to test if tRNA expression correlated with patient survival.
Authors’ contributions
L.H. conceived and supervised the project. Z.Z., Y.Y., C-J.L., H.R., J.G., L.D., A-Y.G., and L.H. performed the analyses. Z.Z, H.R., C-J.L., and A-Y.G. developed the database. Z.Z., H.R., L.D., and L.H. wrote the manuscript with input from all other authors.
Supplemental Material
Download Zip (15.8 KB)Acknowledgments
This work was supported by the Cancer Prevention & Research Institute of Texas (RR150085) to CPRIT Scholar in Cancer Research (L.H.); UTHealth Innovation for Cancer Prevention Research Training Program Post-doctoral Fellowship (Cancer Prevention and Research Institute of Texas, RP160015); China Postdoctoral Science Foundation (2019M652623 to C-J. Liu); National Natural Science Foundation of China (31822030 and 31771458 to A-Y. Guo). We gratefully acknowledge contributions from TCGA Research Network. We thank LeeAnn Chastain for editorial assistance.
Disclosure statement
No potential conflict of interest was reported by the authors.
Supplementary material
Supplemental data for this article can be accessed here
Additional information
Funding
References
- Dever TE, Green R. The elongation, termination, and recycling phases of translation in eukaryotes. Cold Spring Harb Perspect Biol. 2012;4:1–16.
- Geiduschek EP, Kassavetis GA. The RNA polymerase III transcription apparatus. J Mol Biol. 2001;310:1–26.
- Paule MR, White RJ. Survey and summary: transcription by RNA polymerases I and III. Nucleic Acids Res. Internet] 2000; 28:1283–1298. Available from: http://www.ncbi.nlm.nih.gov/pubmed/10684922%5Cnhttp://www.pubmedcentral.nih.gov/articlerender.fcgi?artid=PMC111039.
- Krokowski D, Han J, Saikia M, et al. A self-defeating anabolic program leads to ??-cell apoptosis in endoplasmic reticulum stress-induced diabetes via regulation of amino acid flux. J Biol Chem. 2013;288:17202–17213.
- Girstmair H, Saffert P, Rode S, et al. Depletion of cognate charged transfer RNA causes translational frameshifting within the expanded CAG stretch in huntingtin. Cell Rep Internet] 2013; 3:148–159. DOI: 10.1016/j.celrep.2012.12.019
- Grewal SS. Why should cancer biologists care about tRNAs? TRNA synthesis, mRNA translation and the control of growth. Biochim Biophys Acta - Gene Regul Mech Internet] 2014; 1849:898–907.
- Pavon-Eternod M, Gomes S, Geslain R, et al. tRNA over-expression in breast cancer and functional consequences. Nucleic Acids Res. 2009;37:7268–7280.
- Truitt ML, Ruggero D. New frontiers in translational control of the cancer genome. Nat Rev Cancer. Internet] 2016; 16:288–304. Available from: http://www.ncbi.nlm.nih.gov/pubmed/27112207.
- Zhou Y, Goodenbour JM, Godley LA, et al. High levels of tRNA abundance and alteration of tRNA charging by bortezomib in multiple myeloma. Biochem Biophys Res Commun Internet] 2009; 385:160–164.
- Goodarzi H, Nguyen HCB, Zhang S, et al. Modulated expression of specific tRNAs drives gene expression and cancer progression. Cell Internet] 2016; 165:1416–1427. Available from. ;:.
- Weinstein JN, Collisson EA, Mills GB, et al., The Cancer Genome Atlas Research Network. The cancer genome atlas pan-cancer analysis project. Nat Genet. Internet] 2013; 45:1113–1120. Available from: http://www.ncbi.nlm.nih.gov/pubmed/24071849
- Hu Q, Ye Y, Chan L-C, et al. Oncogenic lncRNA downregulates cancer cell antigen presentation and intrinsic tumor suppression. Nat Immunol. 2019;20:835–851.
- Xiang Y, Ye Y, Lou Y, et al. Comprehensive characterization of alternative polyadenylation in human cancer. J Natl Cancer Inst. 2018;110:379–389.
- Ye Y, Xiang Y, Ozguc FM, et al. The genomic landscape and pharmacogenomic interactions of clock genes in cancer chronotherapy. Cell Syst. 2018;6:314–328.e2.
- Xiang Y, Ye Y, Zhang Z, et al. Maximizing the utility of cancer transcriptomic data. Trends Cancer. 2018;4:823–837.
- Ye Y, Hu Q, Chen H, et al. Characterization of hypoxia-associated molecular features to aid hypoxia-targeted therapy. Nat Metab. 2019;1:431–444.
- Gao J, Aksoy BA, Dogrusoz U, et al. Integrative analysis of complex cancer genomics and clinical profiles using the cBioPortal. Sci Signal. 2013;6(269).
- Cerami E, Gao J, Dogrusoz U, et al. The cBio cancer genomics portal: an open platform for exploring multidimensional cancer genomics data: figure 1. Cancer Discov. 2012;2:401–404.
- Li J, Lu Y, Akbani R, et al. TCPA: A resource for cancer functional proteomics data. Nat Methods. 2013;10:1046–1047.
- Gong J, Mei S, Liu C, et al. PancanQTL: systematic identification of cis-eQTLs and trans-eQTLs in 33 cancer types. Nucleic Acids Res Internet] 2017; Available from: https://academic.oup.com/nar/article/46/D1/D971/4210944
- Ewing B, Green P. Analysis of expressed sequence tags indicates 35,000 human genes. Nat Genet. Internet] 2000; 25:232–234. Available from: http://www.ncbi.nlm.nih.gov/pubmed/10835644.
- Gong J, Li Y, Liu C-J, et al. A pan-cancer analysis of the expression and clinical relevance of small nucleolar RNAs in human cancer. CellReports. Internet] 2017; 21:1968–1981. Available from: https://www.cell.com/cell-reports/fulltext/S2211-1247(17)31533-4.
- Cozen AE, Quartley E, Holmes AD, et al. ARM-seq: AlkB-facilitated RNA methylation sequencing reveals a complex landscape of modified tRNA fragments. Nat Methods. 2015;12:879–884.
- Guo Y, Xiong Y, Sheng Q, et al. A micro-RNA expression signature for human NAFLD progression. J Gastroenterol. 2016;51:1022–1030.
- Pundhir S, Gorodkin J. Differential and coherent processing patterns from small RNAs. Sci Rep. Internet] 2015; 5:12062. Available from: https://www.nature.com/articles/srep12062.
- Pang YLJ, Abo R, Levine SS, et al. Diverse cell stresses induce unique patterns of tRNA up- and down-regulation: tRNA-seq for quantifying changes in tRNA copy number. Nucleic Acids Res. 2014;42(22).
- Krishnan P, Ghosh S, Wang B, et al. Genome-wide profiling of transfer RNAs and their role as novel prognostic markers for breast cancer. Nat Publ Gr Internet] 2016;:1–12. Available from. ;. DOI:10.1038/srep32843.
- Danielson KM, Rubio R, Abderazzaq F, et al. High throughput sequencing of extracellular RNA from human plasma. PLoS One. 2017;12:1–18.
- Beck D, Ayers S, Wen J, et al. Integrative analysis of next generation sequencing for small non-coding RNAs and transcriptional regulation in Myelodysplastic Syndromes. BMC Med Genomics. Internet] 2011; 4:19.Available from: https://bmcmedgenomics.biomedcentral.com/articles/10.1186/1755-8794-4-19.
- Sheng Q, Vickers K, Zhao S, et al. Multi-perspective quality control of Illumina RNA sequencing data analysis. Brief Funct Genomics. Internet] 2016; 16:elw035. Available from: https://academic.oup.com/bfg/article-lookup/doi/10.1093/bfgp/elw035.
- Zhong J, Xiao C, Gu W, et al. Transfer RNAs mediate the rapid adaptation of escherichia coli to oxidative stress. PLoS Genet. 2015;11:1–24.
- Guo Y, Bosompem A, Mohan S, et al. Transfer RNA detection by small RNA deep sequencing and disease association with myelodysplastic syndromes. BMC Genomics. Internet] 2015; 16:727. Available from: https://bmcgenomics.biomedcentral.com/articles/10.1186/s12864-015-1929-y.
- Zhang Z, Ye Y, Gong J, et al. Global analysis of tRNA and translation factor expression reveals a dynamic landscape of translational regulation in human cancers. Commun Biol. 2018;1(234).
- Robinson M, Oshlack A, Halsall JA, et al. A scaling normalization method for differential expression analysis of RNA-seq data. Genome Biol. Internet] 2010; 11:R25.Available from: https://genomebiology.biomedcentral.com/articles/10.1186/gb-2010-11-3-r25.
- Robinson MD, McCarthy DJ, Smyth GK. edgeR: a bioconductor package for differential expression analysis of digital gene expression data. Bioinformatics. 2009;26:139–140.