ABSTRACT
Recent studies suggest RNAs act as promising drug targets. However, limited development has been achieved in detecting RNA–ligand interactions. To guide the discovery of RNA-binding ligands, it is necessary to characterize them comprehensively, especially in the binding specificity, binding affinity and drug-like properties. We established a database, RNALID (http://biomed.nscc-gz.cn/RNALID/html/index.html#/database), which collects RNA–ligand interactions validated by low-throughput experiment. RNALID contains 358 RNA–ligand interactions. Comparing to the fellow database, 94.5% of ligands in RNALID are completely or partially novel collections, and 51.78% have novel two-dimensional (2D) structures. Through the analysis of ligand structure, binding affinity and cheminformatic parameters we found that multivalent (MV) ligands mainly binding to RNA repeats are more structurally conserved in both 2D and 3D structures than other ligand types, exhibit higher binding specificity and binding affinity than ligands binding to non-repeat RNAs, but deviate far from the Lipinski’s rule of five. In contrary, small molecule (SM) ligands binding to virus RNA exhibit higher affinity and more resemble protein-ligands, but potentially possess low binding specificity. Further analysis on 28 detailed drug-likeness properties indicated that RNA-ligands’ development need to balance between the binding affinity and the drug-likeness because of the significant linear co-relationship between the two. Comparing RNALID ligands to FDA-approved drugs and ligands without bioactivity indicated that RNA-binding ligands are different from them in chemical properties, structural properties and drug-likeness. Thus, characterizing the RNA–ligand interactions in RNALID in multiple respects provides new insights into discovering and designing druggable ligands binding with RNA.
Introduction
Protein–ligands interactions discovered in traditional paradigm potentially fail to meet the urgent disease-therapy needs because protein-coding genes take up only ~1.5% of human genome and only 10–15% of them are druggable [Citation1]. Comparably, human genome produces a huge number of non-coding RNAs, for example, between 30,000 and 60,000 human long noncoding RNAs (lncRNAs) have been reported in recent lncRNA annotations [Citation2,Citation3] Ligands targeting on RNAs may involve in cellular regulation no less than ligands targeting on proteins, so detecting RNA ligands provides a promising approach for drug discovery [Citation4]. Recent studies presented small molecules and oligonucleotides for cancer treatment in clinical trials by targeting the mRNA processing, for instance, pladienolides, spliceostatins and sudemycins target the splicing factor 3B (SF3B) to interfere with the interaction of U2 snRNP with pre-mRNA and prevent the assembly of a catalytic spliceosome, thus inhibiting splicing [Citation5,Citation6]. Other studies have reported the roles of microRNA and long non-coding RNA (lncRNA) in human diseases, for instance, the circRNA circ_0124554 (or circ-LNLM) promote the early metastasis for the lymph node-negative colorectal cancer by blocking the ubiquitination of AKT [Citation7], targeting lncRNA (AC104041.1) by antisense oligonucleotides induces antitumor activity in head and neck squamous cell carcinomas, through Wnt2B/β-catenin pathway [Citation8], and microRNA can co-operate with lncRNA to involve in premature rupture of membranes through the circRNA/miRNA/NF-κB pathway [Citation9].
By interacting with these non-coding RNAs, small molecules have been discovered as playing functions in disease therapy [Citation10,Citation11]. Until now, only six ligands (Azacitidine, Carmustine, Fluorouracil, Capecitabine, Tegafur-uracil and Cocarboxylase) interacting with RNAs have been approved as drugs by FDA (https://www.drugbank.ca/). Thus, it is necessary to identify more RNA-targeting drugs.
A plenty of studies have been performed to identify RNA–ligand interactions experimentally. For instance, Serganov et al. presented the crystal structures of the Fusobacterium nucleatum riboswitch bound to riboflavin and antibiotic roseoflavin [Citation12]. However, there are few databases integrating these published RNA–ligand interactions. NALDB [Citation13] is a database including experimentally validated interactions between small molecules and various types of nucleic acids. In NALDB, 104 entries of RNA–ligand interactions were included. However, only information of ligands can be accessed but not RNAs. R-BIND [Citation14–16] is a more recently developed database containing information on reported chemical probes that target on non-ribosomal RNAs. Totally, 116 bioactive ligands were included in R-BIND. Nevertheless, R-BIND lacks several aspects of information on ligands. First, in R-BIND, only chemical information but not ligand structural information was available, whereas studies have revealed that ligands binding with the same RNA are more likely to share similar two-dimensional structures [Citation17]. Secondly, R-BIND divided ligands simply into small molecules (SM) and multivalent ligands (MV), and ignored the difference of ligands in other respects [Citation15]. Moreover, sequences of RNA binding with ligands as well as the therapeutical destinations of the ligands were not included in R-BIND database. Using the data of R-BIND, Warner et al. analysed RNA–ligand interactions on relationships between drug-like scores and binding affinities [Citation4]. This analysis is based on a small sample of totally 30 ligands because of the prerequisite that each sample ligand should bind to RNAs with known tertiary structures. Currently, no studies have characterized the binding affinity, binding specificity and drug-likeness of RNA-binding ligands in the perspective of 2-dimensional (2D) and 3-dimensional (3D) structures, chemical properties and spatial properties inside and across detail ligand types. Furthermore, no studies have been performed to reveal the relationship between RNA-ligand-binding affinities and drug-likeness of ligands.
In this study, we manually curated a database, RNALID, including experimentally validated RNA–ligand interactions published before Feb, 2020. The database contains totally 358 experimentally validated RNA–ligand interactions, in which 340 entries are partially new (added new information for previously collected RNA–ligand interactions) or completely new (newly collected RNA–ligand interactions) comparing to existing databases. A webservice (http://biomed.nscc-gz.cn/RNALID/html/index.html#/database) was provided to predict ligand–RNA interactions through comparing the query on ligand structures, chemical properties, RNA sequence and RNA secondary structure to the ligands or RNAs in RNALID. Using the RNALID data, comprehensive analysis was performed on 2D and 3D structural characteristics, spatial properties and chemical properties of RNA-binding ligands to access their binding affinity, binding specificity and drug-likeness. Moreover, this study revealed the relationships between RNA-ligand-binding affinities and 28 comprehensive drug-like properties. To identify the differences between RNA-binding and non-RNA-binding ligands, we collected 42,234 ligands without bioactivates and 7,753 FDA approved non-RNA-binding drugs, and compared them with the RNA-binding ligands in RNALID on chemical properties, spatial properties, structures and drug-likeness.
Material and methods
Collection of RNA–ligand interactions
RNA–ligand interactions were collected in RNALID if the interactions were validated by low-throughput experiments according to published studies in PubMed. The low-throughput experiments include Isothermal Titration Calorimetry (ITC), spectroscopic deciphering, circular dichroism (CD) spectroscopy, Fluorescence-based binding assay, NMR, gel mobility shift assays, CD spectroscopy, and UV spectroscopy. Interactions involving only rRNAs were ignored [Citation14,Citation16]. We searched PubMed with keywords ‘RNA’, ‘drug’, ‘ligand’ and ‘interaction’ along with their plural forms. Totally, 103 literature were involved in this study. From these literature, we manually extracted information on ligand structures, sequences of RNA binding with ligands, HGNC names of binding RNAs, and targeted diseases of ligands. If a series of analogs was studied in single report, we would kept only the one representative for each compound series, which is highlighted by the author in the abstract or discussion section would be adopted. The graphical illustrations of ligand structure were converted to SMILES format by BKChem (http://bkchem.zirael.org/). If a PDB ID (http://www.rcsb.org/) of the ligand was offered by the author, the SMILES and InChIKey on the PDB webpage were both kept in RNALID. If the ligand has been included in the PubChem database (https://pubchem.ncbi.nlm.nih.gov/), the SMILES and InChIKey of it in the PubChem were kept.
To integrate RNA–ligand interactions in RNALID with other databases, we manually curated RNA sequence, HGNC names of corresponding RNA and targeted diseases for RNA–ligand interactions in R-Bind, the only existing database containing downloadable RNA–ligand interactions. All ligands in R-BIND were transformed from SMILES format to InChIKeys using OpenBabel software [Citation18].
Ligand classification
Ligands in RNALID were grouped under a variety of classification principles as shown in Table S1. In detail, using the principle of structural characters, ligands were defined as ‘MV’ (multivalent ligands) if their structures were in the presence of an alkyl, aryl, or peptide-like linker between two or more binding moieties. Otherwise, they were defined as ‘SM’ (small molecules). Using the principle of target preference, ligands in format of InChIKeys in the BindingDB database [Citation19,Citation20] (https://www.bindingdb.org/bind/index.jsp) were considered as binding with both RNAs and proteins (‘RNA&protein’ type) while the remained ligands were considered as only binding with RNAs (‘RNA’ type). Ligands were classified as ‘rep RNA’ if their RNA targets contains nucleotide repeat expansions, such as r(CUG)exp, otherwise they were defined as ‘non-rep RNA’ type. Finally, according to RNA types binding with them, ligands were classified into six groups including mRNA, vRNA, miRNA, tRNA, ncRNA, siRNA, and others. Here, vRNA represents mRNA from virus, and mRNA represents mRNA from cellular organisms. To obtain statistically meaningful results, the analysis focused on ligands binding with mRNA and vRNA because their sample sizes are more than 20 (Table S1.).
Ligand similarity
In this study, ligand similarities include chemical similarity and structural similarity. To evaluate the chemical similarity between ligands, 12 cheminformatic parameters for each ligand were generated by RDKit (http://www.rdkit.org/) (Table S2). Chemical similarities between ligands based on these cheminformatic parameters were calculated by procedures shown in Supplementary material.
The ligand structure similarities were evaluated by 2D structure similarly and 3D structure similarity, respectively. The calculation of 2D structure similarity was performed by the procedure described in a previous study [Citation21], where ligand structures were first transformed to fingerprint format of FP2 by OpenBabel [Citation18]. The 3D structure similarity of ligands was evaluated by LSalign [Citation22] under the mode of flexible alignment as described in a previous study [Citation23].
Spatial property of ligand
The spatial property of ligand was evaluated by the principal moment of inertia (PMI). The PMI was performed using a functional module in RDKit. The detailed steps are included in the Supplementary material.
Drug-like properties
Totally, 28 drug-like properties were considered in this study (Table S3). They were calculated by CMPNN algorithm [Citation24] with training data from databases of BBBP (http://moleculenet.ai/datasets-1), Tox21 (https://tox21.gov/), Sider (http://sideeffects.embl.de/), ClinTox (https://www.clintox.org/), ESOL [Citation25] and FreeSolv [Citation26]. The differences of drug-like index scores between ligands types were evaluated by Mann-Whitney U test.
Collection of FDA drugs and ligands without bioactivities
FDA drugs were collected from the DrugBank database [Citation27–31], which contains drugs approved by Food and Drug Administration (FDA). They were filtered by Lipinski’s rule of five (RO5) [Citation32] to obtain the protein-binding drugs [Citation4]. Ligands without bioactivates were collected from the Bioassay&Bioactivities section of PubChem project (https://pubchem.ncbi.nlm.nih.gov/). These ligands were named as ‘control ligand’ in this study.
Results
Establishing an RNA–ligand interaction database (RNALID)
RNALID database (http://121.46.19.89/RNALID/html/index.html#/database, Figure S1) contains 358 experimentally validated RNA–ligand interactions, in which 74.86% of RNAs have sequence information, 11.73% of ligands have drug therapy information obtained from DRUGBANK [Citation27–31] (Table S4), 30.17% of RNAs have HGNC symbols, 41.9% of RNAs or ligands bind with other types of targets except ligands or RNAs, and 93.58% of RNA–ligand interactions have information on associated diseases (Table S5 and Figure S2). All this information is not available yet in existing databases. Additionally, RNALID includes binding affinity for 58.66% of RNA–ligand interactions (in at least one record of Ki, Kd, IC50, EC50 and CC50).
To access if structural and chemical space covered by RNALID ligands is enough to draw reliable conclusion, the chemical and structural properties of ligands in RNALID were compared with RNALigands, the newest RNA–ligand interactions databases, and R-BIND, the public RNA–ligand interaction database with currently most comprehensive information. As shown in supplementary Figure S3, PCA analysis on cheminformatic parameters indicates that RNALID ligands exhibit roughly equal cheminformatic novelty as the RNALigands ligands. As to the structural information, ligands in neither RNALID nor RNALigands exhibit inter- 2D similarity less than 0.4, indicates ligands in these two databases possess equal novelty in the perspective of 2D structure. However, the distribution of intra-2D similarities of RNALID ligands are relatively more dense approaching 0.4, indicating a higher 2D structural variety. Detail interpretation of the 2D similarity plot can be found in the ‘Comparing RNALID to other databases on chemical and structure properties’ section of the supplementary material. Under the same paradigm, similar conclusion could be drawn in 3D similarity comparison between RNALID and RNALigands, that RNALID ligands possess equal 3D structural novelty as RNALigands but higher 3D structural variety. This result make confidence of our further conclusions analysed based on RNALID ligands’ structure.
Actually, RNALID and RNALigands are built for rather different purpose. RNALigands database aimed to predict RNA–ligand interaction based on the RNA secondary structure characters, while RNALID is more focusing on further data mining work, so comprehensive information of each term of RNA–ligand interaction is needed, including the disease for whose therapy the RNA is target by the ligand. More important, the RNALID database pays more attention on the probability of being oral drug of the ligands than the RNALigands database. For example, Co iron was collected by RNALigands database as a RNA ligand (PDB ID_chain ID: 1Q29_A and 4RUM_A, as shown in the supplementary Table S1 of the RNALigands reference), but it is unlikely to be oral drug for disease therapy.
Sharing more similarity with our work, the R-BIND database collect comprehensive information for RNA–ligand interactions, so we made a parallel comparison with the R-BIND database. As shown in supplementary Figure S4-S5, 51.78% of ligands have novel 2D structures comparing to other databases when using 2D structure similarity score > 0.4 to define ligands with similar structures.
Cross-enrichment analysis of ligand types
We consider it would be easier to disclose the regular pattern of binding affinity, binding specificity and drug-likeness for RNA ligands, so ligands in RNALID were divided into 14 types as shown in Table S1.The binding Cross-enrichment analysis was employed to detect if one type of ligands is enriched with ligands belonging to another type. The enrichment was evaluated by single-tailed Fisher’s exact test. The results were shown in supplementary Figure S6. We found that SM ligands prefer to bind virus RNA (OR = 8.96, P-value = 1.20 × 10−2), while MV ligands were significantly enriched with ligands binding with RNA repeats (OR = 6.63, P-value = 8.65 × 10−3). These two results are supported by current reports [Citation4,Citation15]. Moreover, we found that ligands binding with both RNA and protein were all SM ligands, indicating that SM ligands are more resemble with protein ligands, like the riboflavin ligand [Citation4]. However, ligands binding with sole RNA targets were not enriched by any other types.
Chemical properties of different types of ligands in RNALID
The varieties in cheminformatic parameters (detail definition in the supplementary Table S2) of different types of ligands were evaluated by MW-U test. The cheminformatic parameters of SM and MV ligands were compared in . Totally, 9 out of 12 cheminformatic parameters are significantly different in SM and MV ligands. MV ligands have higher molecular weight, topological polar surface area (tPSA), Rings, aromatic rings (ArRings), heteroatom-containing rings (HetRings), rotatable bonds (RotB), accessible surface area(ASA), as well as hydrogen-bond acceptors (HBA) and donors (HBD). According to Lipinski’s rule of five (RO5) [Citation32], MV ligands may have lower probability to be oral bioavailable ligands than SM ligands.
Figure 1. Comparing cheminformatic parameters of ligands in RNALID. (A) Comparing SM ligands and MV ligands. (B) Comparing ligands only binding with RNA, and ligands binding with both RNA and protein. (C) Comparing ligands binding with mRNA from cellular organisms and ligands binding with mRNA from virus (vRNA). (D) Comparing ligands binding with RNA including repeat sequence (rep RNA) and ligands binding with RNA without repeat sequence (non-rep RNA). The detail definition of each cheminfomatic parameter is in the supplementary Table S2. The differences of chemical characters of ligands were evaluated by MW-U test. ‘*’ denotes P-value <0.05, ‘**’ denotes P-value <0.01, ‘***’ denotes P-value <0.001.
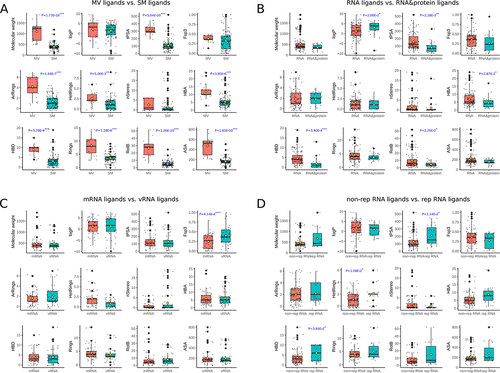
Comparing ligands only binding with RNA (RNA type) and ligands binding with both RNA and protein (RNA&protein type) on cheminformatic parameters, we found the RNA&protein type of ligands exhibited a significant increasing in logP while a reduction in polar surface area (tPSA), hydrogen bond donors (HBD), rotatable bonds (RotB), and hydrogen-bond acceptors (HBA) than ligands only binding with RNA (). This is in accordance with the truth that RNAs are charged due to the phosphate group in each of the four types of ribonucleotide, while not the case for the 20 types of protein residues. This result also indicated that ligands binding with both RNA and protein have a higher possibility to be oral bioavailable ligands according to Lipinski’s rule of five [Citation32] than ligands only binding with RNA.
Ligands interacting with mRNA have significantly lower fraction of hybridized SP [Citation3] carbons (Fsp [Citation3] values than ligands binding with vRNA while they show similar values in the other 11 cheminformatic parameters (). This may be explained referring to the truth that virus RNAs were mostly bound by SM ligands, and the latter possess lower Fsp [Citation3], which indicates better physicochemical properties to be oral drug.
Comparing ligands binding with rep RNA to ligands binding with non-rep RNA indicated that ligands binding with rep RNAs have significantly (P-value = 1.14 × 10−2) higher polar surface area (tPSA), a greater number of HetRings (P-value = 1.08 × 10−2) and HBD (P-value = 3.93 × 10−2) than ligands binding with non-rep RNAs (). This is also probably driven by the truth that RNA repeats were mostly bound by MV ligands, which have lower probability to be oral bioavailable. However, considering that RNA repeats serve as therapy targets for disease like myotonic dystrophy type 1(DM1), a solution is still needed such as structural optimization or good drug-delivery system for MV ligands.
Structure diversity of different types of ligands
For a fixed certain target, higher ligand structural diversity indicates larger space for lead compound discovery to gain better drug physicochemical properties or higher binding affinity. Here we calculated pair-wised 2D structure and 3D structure similarities of all ligands in RNALID. The pair-wised structure similarities for the same type of ligands were defined as intra-similarity. We calculated the average scores of the intra-similarity. The results were shown in . MV ligands have the highest intra-similarity in 2D structures than other types of ligands. The average 2D structure similarity score of MV ligands was significantly (P-value = 7.61 × 10−24) higher than that of SM ligands. Thus, ligands in MV group may be less diverse in 2D structures than ligands in other types. The average 3D structure similarity score of ligands binding both RNA and protein was significantly (P-value = 1.63 × 10−4) higher than that of ligands binding only with RNAs, indicating the ligands binding with both RNA and protein are less structurally diverse than ligands only binding with RNAs. Moreover, ligands binding with non-rep RNA have significantly (P-value = 4.69 × 10−11 and 6.43 × 10−4) lower 2D and 3D structure similarity than the ligands binding with rep RNA, suggesting a higher structural conservation of ligands binding with repeated RNA sequence.
Figure 2. Comparing 2D and 3D structure similarity of ligands in RNALID. (A) Comparing 2D structures of the same type of ligands. (B) Comparing 3D structures of the same type of ligands.
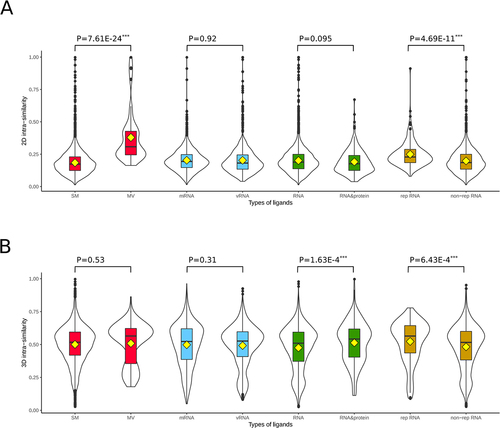
We further calculated the pair-wised structure similarities of ligands across different types. These structure similarity scores were defined as inter-similarity. The results were shown in Figure S7. We found that ligands in types of MV, mRNA, vRNA and rep RNA exhibited higher intra-similarity scores than inter-similarity scores in both 2D and 3D structures, suggesting they are more structurally conserved, and may possess high binding specificity to the RNA target, suggesting less side-effect caused by off-target effects.
PMI analysis on ligands in different groups
Spatial properties of ligands were evaluated by PMI analysis. As shown in , ligands binding with mRNA from cellular organisms are mainly near rod vertex while ligands binding with vRNA are close to disc vertex (). However, the cumulative fraction graphs (Figure S8A) did not confirm the statistical significance between these two types of ligands. When ligands were grouped into ligands solely binding with RNA and binding with both RNA and protein (RNA&protein), they were found sharing the same spatial properties and mainly located near rod and disc vertex (). These conclusions were verified by cumulative fraction graphs. Meanwhile, neither of them preferred sphere-like shape (Figure S8B). The analysis was finally performed for the ligands belonging to ‘rep RNA’ and ‘non-rep RNA’ groups, respectively. Ligands binding with rep RNA were largely populated in the region near rod vertex while the ligands binding with non-rep RNA group were mainly located near disc vertex (). Meanwhile, the cumulative fraction graphs indicated that ligands binding with rep RNA were more rod-like ligands while ligands binding with non-rep RNA were more disc-like ligands (Figure S8C).
Figure 3. Comparing spatial properties of different types of ligands in RNALID. (A) Comparing ligands binding with mRNA and ligands binding with vRNA. (B) Comparing the spatial properties of ligands only binding with RNA to the ligands binding with both RNA and protein. (C) Comparing ligands binding with RNA with repeat sequence and ligands binding with RNA without repeat sequence. Each point represents one ligand. A triangle (average) represents the mean values of coordinates in the 2D-space formed by I1/I3 and I2/I3for each type of individual ligands.
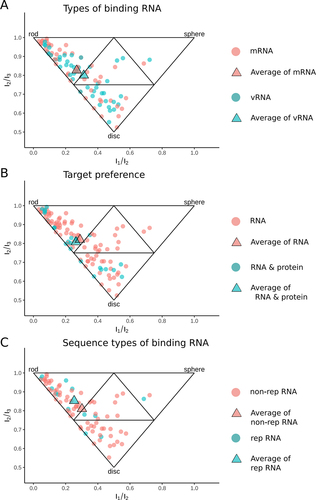
Binding affinity of RNA–ligand interactions for ligands belonging to different groups
In this study, we collected binding affinities for 204 RNA–ligand interactions, which are in IC50, EC50, Ki, Kd or CC50 format. As shown in Figure S9A, significant difference (two-tailed MW-U test P-value = 0.012) in binding affinity is observed between ligands binding with rep RNAs and ligands binding with non-rep RNAs. The interactions between ligands binding with rep RNAs were significantly stronger than the interactions between ligands binding with non-rep RNAs. Meanwhile, the interactions between ligands and vRNAs were significantly (two-tailed MW-U test P-value = 0.012) stronger than those between ligands and mRNA (Figure S9B). No significant differences were observed between MV ligands and SM ligands (Figure S9C), and between ligands binding with only RNA and ligands binding with RNA&proteins (Figure S9D). So the MV ligands binding to RNA repeats and SM ligands binding to virus RNA exhibit high binding affinities.
Relationships between drug-like properties and RNA-ligand-binding affinities
Though Lipinski’s rule of five is canonical in oral-drug bio-availability estimation, detailed drug-like properties of RNA ligands are still necessary to explore. Here, we predicted 28 detail drug-like properties for RNALID ligands, with detail definition in supplementary Table S3. As shown in and Figure S10, ligands binding with mRNA from cellular organisms have significantly higher QED scores for drug-like properties of SR.p53, SR.MMP, SR.HSE, SR.ATAD5, SR.ARE, NR.PRAR.gamma, NR.ER.LBD, NR.ER, NR.Aromatase, NR.AR.LBD, NR.AhR and LIVER than ligands binding with coding RNA from virus (vRNA). This result indicates that vRNA ligands process higher drug-likeness than mRNA ligands because druglike index of LIVER (drug metabolism in liver) is positively related to unstable and unpredictable bio-effects of drugs while the other druglike indexes are positively related to toxicity. Ligands binding with rep RNA show significantly higher QED scores for drug-like properties of SR.ATAD5, NR.ER, NR.AhR, and LIVER than ligands binding with non-rep RNA. Because higher QED scores of these druglike indexes were positively related with toxicity or unstable drug effects, non-rep RNA ligands exhibit higher drug-likeness. Ligands binding with RNA & proteins have significantly higher QED scores for drug-like properties of LOGP, CYP2C9_S, CLINTOX and CACO2 than ligands solely binding with RNA. Finally, ligands in MV are indicated with higher QED scores for drug-like properties of SR.p53, SOLUBILITY, NR.PRAR.gamma, NR.AR.LBD, CYP3A4_S and CYP2D6_I than ligands in SM. Four out of the five indexes were positively related with toxicity. Thus, SM ligands may have higher probability to be drugs than MV ligands. This is in consistent with the findings in comparing cheminformatic parameters of these two types of ligands.
Figure 4. Relationships between RNA-ligand-binding affinity and drug-like properties. (A) Comparison of QED scores among different types of ligands (two-tailed MW-U test was used to evaluate the significance). (B)–(E) P-values and effect sizes of Pearson correlation coefficient of RNA-ligand-binding affinity and QED scores for different types of ligands. Statistically significant Pearson co-relationship (P-value <0.05 and |PCC|>0.4) was marked as black-circled points.
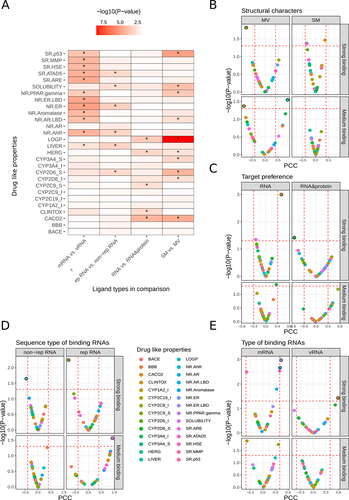
For each type of ligands, we examined the Pearson correlation between the binding affinity and QED scores of drug-like properties ( and Figure S11). It can be observed that the P-values of Pearson Correlation Coefficient (PCC) between QED score and binding affinity of ligand and RNA, and indicated that the binding affinities of strong RNA–ligand interactions [binding affinity lower than 1 μM (1E–6 mol/L) and higher than 0 nM] were more likely to be significantly correlated with QED scores than the medium interactions (binding affinities were higher than 1 μM and lower than 1 mM). The detailed definitions of strong and medium RNA–ligand interactions were shown in Supplementary Material. The QED scores of 10 drug-like properties, CACO2, NR.ER, NR.AR, SR.ATAD5, SR.HSE, CYP2C9_S, NR.AR.LBD, NR.AhR, CYP2D6_S and SR.ARE were found significantly correlated with binding affinities of strong interactions while the QED scores of two drug-like properties, CYP3A4_S and LIVER were found significantly correlated with binding affinities of medium interactions.
When the binding affinities were strong interactions, the binding affinities of SM ligands were found significantly correlated with the QED scores of NR.AhR, CACO2, and SR.HSE. The binding affinities of MV ligands with strong interactions were found correlated with the QED score of CYP2C9_S only. In comparison, the binding affinity of MV ligands with medium interactions were found significantly correlated with the QED scores of LIVER while the binding affinity of SM ligands with medium interactions were not found significantly correlated with any QED scores. Thus, the binding affinities of MV and SM ligands were possibly influenced by different drug-like index. Figure S11 shows the scatter plots describing the correlations between binding affinity and 16 drug-like properties. These results indicates that it is unwise to solely consider binding affinity in drug development, instead, the balance between binding affinity and drug-like properties are more important, it is essentially the balance between expected bio-effects and cellular toxicity.
Comparing ligands in RNALID with FDA-approved drugs and ligands without bioactivities
We collected 7,753 FDA-approved drugs from DrugBank database [Citation27–31], and 42,234 ligands without bioactivities from PubChem database (https://pubchem.ncbi.nlm.nih.gov/). The ligands without bioactivities were referred to as control ligands. For these ligands, we calculated their chemical properties, 2D and 3D structures and drug-like properties.
To compare the chemical properties of ligands in RNALID with the FDA drugs and the control ligands, we performed PCA analysis on the cheminformatic parameters. As shown in , many MV ligands and SM ligands in RNALID are separated from FDA drugs and control ligands. The PC1 differences were evaluated by two-tailed MW-U tests (). When the RNALID ligands were grouped into solely binding with RNA and binding with RNA&protein, we found that ligands solely binding with RNA are significantly different from the FDA drugs and the control ligands in PC1. When the ligands from RNALID were divided into types of binding with vRNA and mRNA, they both were significantly different from the FDA drugs and the control ligands in PC1. When the ligands were grouped into types of rep RNA and non-rep RNA, they both were significantly distinctive from the FDA drugs and the control ligands in PC1.
Figure 5. Comparing chemical characters of ligands in RNALID, FDA approved drugs and ligands without bioactivities. (A) Principal component analysis (PCA) results of chemical characters of ligands from three sources. Two-tailed MW-U tests were performed to evaluate the difference of them on PC1. (B) Comparing ligand structure similarity. Single-tail Student’s t-test was used to evaluate the differences of ligands from three sources.
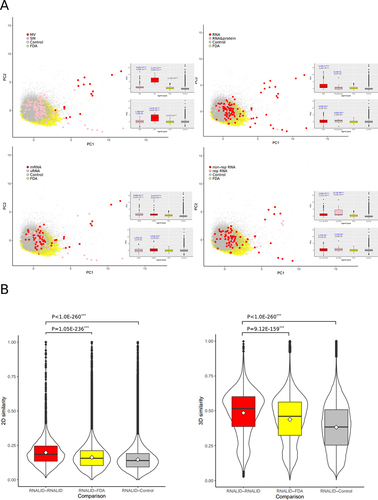
Further analysis is to compare cheminformatic parameters for ligands in RNALID, the FDA drugs, and the control ligands by two-tailed MW-U tests. As shown in Table S6, only two cheminformatic parameters, Fsp3 and nStereo are not significantly different between the RNALID ligands and the control ligands. Only one cheminformatic parameter, LogP, is not significantly different between the RNALID ligands and the FDA drugs.
We further compared the 2D and 3D structures of RNALID ligands, FDA drugs, and control ligands. The intra-similarity of RNALID ligands was compared with inter-similarity with FDA drugs and control ligands. As shown in , the intra-similarities of RNALID ligands are significantly higher than the inter-similarity with the FDA drugs or the control ligands, indicating that the RNALID ligands are different from both the FDA drugs and the control ligands in structures.
The drug-likeness of the RNALID ligands was compared with the FDA drugs and the control ligands. We found that QED scores of CYP2D6_I, CYP3A4_I, HERG, and LIVER are not significantly different between the RNALID ligands and the control ligands (Table S7). Further comparison on the RNALID ligands and the FDA drugs indicated that CYP1A2_I, CYP2D6_I, and LIVER are not significantly different between these two groups of ligands (Table S7).
Above results remind us to pay more attention in the development of RNA-target small molecular drugs, that RNA-ligands are probable to possess significantly different physicochemical characteristics from protein-drugs, so the protein-target oral drug standard like Lipinski’s rule of five may not be suitable for RNA-target drugs. This problem had been put forward by the current review [Citation33].
Discussion
RNAs have been considered as potential drug targets [Citation34,Citation35]. However, the properties of RNA–ligand interactions are still poorly understood which has hindered the design of RNA-targeting drugs. Recently, several databases collecting RNA–ligand interactions have been established, whereas most of them have limited information to comprehensively reflect the characteristics of RNA–ligand interactions. To date, there are no studies carrying comprehensive analysis on the characteristics of RNA–ligand interactions by dividing ligands into different types under varieties of classification principles, taken both ligand and target characters into consideration.
This study established a database, RNALID, containing the largest number of experimentally validated RNA–ligand interactions and the most comprehensive information attached with them. To insure the RNALID ligands cover enough chemical and structural space to draw confident results, we compared the cheminformatic parameters and 2D/3D structural similarities of RNALID ligands with the RNALigands database (the newest) and the R-BIND database (currently the most comprehensive). As shown in supplementary Figure S3, RNALID database exhibited equal chemical and structural novelty, but higher structural variety and more attention to ligand oral-drug-likeness comparing with the RNALigands database. RNALID database exhibited higher chemical and structural novelty than the R-BIND database. This result makes confidence for the conclusions drawn by further analysis, even though the first version of RNALID collection ended up in Feb. 2020. Actually, we have queried the PubMed database to obtain the RNA–ligand interactions discovered after Feb. 2020, and we find only five publications [Citation36–40], The small scale of new data won’t considerably change the conclusion of this work, whereas we will add it to the updated version of RNALID website. What needs to mention is about the structure availability of RNAs in RNALID database. For secondary structures, 74.86% of RNAs with sequence information were attached with reported or reliably predicted secondary structure, because the technology for predicting the secondary structure from the RNA sequence has been mature. As to the tertiary structure, only three of the RNAs have PDB IDs (5C45, 3F4H and 218C) attached in the reference, which indicate a tertiary structure. We did not try to predict the tertiary structures for the remained RNAs, because we consider no model could make robust and reliable results currently.
When the ligands in RNALID are divided into many types using variety of classification principles, the ligands in different groups show various characteristics. MV ligands exhibited significantly higher values of nine cheminformatic parameters comparing to SM ligands, indicating that SM ligands are more approaching the drug-like rules as illustrated in the study of protein-binding ligands [Citation4]. Ligands binding to short-repeat RNAs (rep RNA) were the only type of ligands that exhibited significantly higher structural conservative comparing with its opposite ligand type, non-rep RNA ligands both in 2D and 3D structure comparisons. To discover if ligands belonging to one group preferred to have characteristics of other groups, we additionally analysed the enrichment of ligands in multiple groups. As shown in Figure S6, MV ligands are enriched with ligands binding with rep RNA and ligands binding with tRNA while SV ligands are enriched with ligands binding with vRNA.
Drug-likeness is a key factor in drug development [Citation41]. It has been proved that more than 40% of compounds failed to be developed into drugs due to drug-like properties [Citation42]. Thus, it is important to discover the relationship among drug-like properties, RNA-ligand-binding affinity and ligand properties. In this study, 2D and 3D structural characteristics, chemical properties of ligands, and the relationship between ligand-binding affinity and 28 drug-like properties were analysed for ligands grouped under various classification principles. We found that the drug-like properties CACO2, NR.AhR, SOLUBILITY and SR.HSE of ligands were the most frequently related with binding affinity of ligands from different types. In most of the cases, CACO2 cell model was used to validate the capability of drugs to go through human Intestinal epithelium [Citation43]. Besides, previous studies have indicated the close relationship between CACO2 cell activities and protein targets [Citation44–50], for example, antioxidant agent can lead to the morphological changes and cell viability for Vero and Caco-2 cell line [Citation44], exhibiting close relationship between caco-2 cell activity and oxidation circumstances [Citation45,Citation46].
Studies have revealed that many virus genomes contain structures or sequence motifs for binding ligands [Citation51]. The analysis in this study indicated that ligands binding to mRNAs and vRNAs exhibited significant difference in binding affinities, chemical characters and co-relationships with drug-like properties. Additionally, we found that vRNA-binding ligands were enriched with ligands binding with non-rep RNA. In comparison, ligands binding with mRNAs from cellular organisms were enriched with ligands binding to rep RNAs. A potential explanation on the differences is the difference of ligand structures. vRNAs prefer to bind with SM ligands while mRNAs from cellular organisms prefer to binding with MV ligands. More efforts are required to reveal the biological mechanisms underlying the ligand-binding preferences of vRNA and mRNAs in cellular organisms.
To distinguish the properties of ligands in RNALID with ligands not binding with RNA, we compared RNALID with FDA approved protein-binding drugs and control ligands without bioactivity in several respects. Two drug-like properties, LIVER and CYP2D6_I, are not significantly different between RNALID ligands and FDA drugs, and between RNALID ligands and the control ligands. Further study is required to detect the underlying reasons.
In conclusion, this study established the RNALID, a comprehensive database collecting RNA–ligand interactions. By analysing RNALID database, the signatures of different types of ligands in chemical characters, spatial properties, binding affinities, structural conservation were described. Further drug-likeness evaluation provided clues to discover ligands with high potential to be drugs.
Key Points
We established a database, RNALID, by collecting RNA–ligand interactions validated by low-throughput experiments, which contains 358 RNA–ligand interactions, and 94.5% of them are completely or partially novel collections.
Ligands in RNALID were divided into 14 types, and were analysed on chemical properties, spatial properties, binding affinity and drug-likeness. Small molecule (SM) ligands binding with non-repeat RNA or virus RNA were indicated having higher probability to be drugs than other types of ligands.
Ligands in RNALID were different from protein-binding ligands and ligands without bioactivity in chemical properties, structural properties and drug-likeness.
Contributors
Prof. Huiying Zhao designed this study. Miss. Xin Wang and Mr. Tianze Ling collected data and performed analysis. Dr. Cong Fan performed analysis and prepared the manuscript. Prof. Yuedong Yang performed analysis and prepared the manuscript.
Patient and public involvement
Patients and/or the public were neither involved in the design/conduct, nor reporting/disseminating plans of this research. Patient consent for publication are not required.
Supplemental Material
Download MS Word (1.5 MB)Disclosure statement
No potential conflict of interest was reported by the author(s).
Data availability statement
RNALID web service is available at http://121.46.19.89/RNALID/html/index.html#/database
All data was available in the manuscript or the supplementary material.
Supplementary material
Supplemental data for this article can be accessed online at https://doi.org/10.1080/15476286.2023.2231708.
Additional information
Funding
References
- Cheng AC, Coleman RG, Smyth KT, et al. Structure-based maximal affinity model predicts small-molecule druggability. Nature Biotechnol. 2007;25(1):71–75. doi: 10.1038/nbt1273
- Hon CC, Ramilowski JA, Harshbarger J, et al. An atlas of human long non-coding RNAs with accurate 5′ ends. Nature. 2017;543(7644):199–204. doi: 10.1038/nature21374
- Iyer MK, Niknafs YS, Malik R, et al. The landscape of long noncoding RNAs in the human transcriptome. Nat Genet. 2015;47(3):199–208. doi: 10.1038/ng.3192
- Warner KD, Hajdin CE, Weeks KM, et al. Principles for targeting RNA with drug-like small molecules. Nat Rev Drug Discov. 2018;17(8):547–558. doi: 10.1038/nrd.2018.93
- Effenberger KA, Urabe VK, Jurica MS, et al. Modulating splicing with small molecular inhibitors of the spliceosome. Wiley Interdiscip Rev RNA. 2017;8(2):e1381. doi: 10.1002/wrna.1381
- Lee SC, Dvinge H, Kim E, et al. Modulation of splicing catalysis for therapeutic targeting of leukemia with mutations in genes encoding spliceosomal proteins. Nat Med. 2016;22(6):672–678. doi: 10.1038/nm.4097
- Tang J, Zhang C, Huang Y, et al. CircRNA circ_0124554 blocked the ubiquitination of AKT promoting the skip lymphovascular invasion on hepatic metastasis in colorectal cancer. Cell Death Dis. 2021;12(3):270. doi: 10.1038/s41419-021-03565-3
- Li M, Ding X, Zhang Y, et al. Antisense oligonucleotides targeting lncRNA AC104041.1 induces antitumor activity through Wnt2B/β-catenin pathway in head and neck squamous cell carcinomas. Cell Death Dis. 2020;11(8):672. doi: 10.1038/s41419-020-02820-3
- Gao Y, Mi N, Zhang Y, et al. Uterine macrophages as treatment targets for therapy of premature rupture of membranes by modified ADSC-EVs through a circRna/mirna/nf-κB pathway. J Nanobiotechnology. 2022;20(1):487. doi: 10.1186/s12951-022-01696-z
- Costales MG, Hoch DG, Abegg D, et al. A designed small molecule inhibitor of a non-coding RNA sensitizes HER2 negative cancers to herceptin. J Am Chem Soc. 2019;141(7):2960–2974. doi: 10.1021/jacs.8b10558
- Petrescu GED, Sabo AA, Torsin LI, et al. MicroRNA based theranostics for brain cancer: basic principles. J Exp Clin Cancer Res. 2019;38(1):231. doi: 10.1186/s13046-019-1180-5
- Serganov A, Huang L, Patel DJ, et al. Coenzyme recognition and gene regulation by a flavin mononucleotide riboswitch. Nature. 2009;458(7235):233–237. doi: 10.1038/nature07642
- Kumar Mishra S, Kumar A. NALDB: nucleic acid ligand database for small molecules targeting nucleic acid. Database (Oxford). 2016;2016:2016. doi: 10.1093/database/baw002
- Morgan BS, Forte JE, Culver RN, et al. Discovery of key physicochemical, structural, and spatial properties of RNA-Targeted bioactive ligands. Angew Chem Int Ed Engl. 2017;56(43):13498–13502. doi: 10.1002/anie.201707641
- Morgan BS, Forte JE, Hargrove AE, et al. Insights into the development of chemical probes for RNA. Nucleic Acids Res. 2018;46(16):8025–8037. doi: 10.1093/nar/gky718
- Morgan BS, Sanaba BG, Donlic A, et al. R-BIND: an interactive database for exploring and developing RNA-Targeted chemical probes. ACS Chem Biol. 2019;14(12):2691–2700. doi: 10.1021/acschembio.9b00631
- Velagapudi SP, Gallo SM, Disney MD, et al. Sequence-based design of bioactive small molecules that target precursor microRnas. Nat Chem Biol. 2014;10(4):291–297. doi: 10.1038/nchembio.1452
- O’Boyle NM, Banck M, James CA, et al. Open Babel: an open chemical toolbox. J Cheminform. 2011;3(1):33. doi: 10.1186/1758-2946-3-33
- Gilson MK, Liu T, Baitaluk M, et al. BindingDB in 2015: a public database for medicinal chemistry, computational chemistry and systems pharmacology. Nucleic Acids Res. 2016;44(D1):D1045–53. doi: 10.1093/nar/gkv1072
- Liu T, Lin Y, Wen X, et al. BindingDB: a web-accessible database of experimentally determined protein-ligand binding affinities. Nucleic Acids Res. 2007;35(Database issue):D198–201. doi: 10.1093/nar/gkl999
- Yang Y, Zhan J, Zhou Y, et al. SPOT-Ligand: fast and effective structure-based virtual screening by binding homology search according to ligand and receptor similarity. J Comput Chem. 2016;37(18):1734–1739. doi: 10.1002/jcc.24380
- Hu J, Liu Z, Yu D-J, et al. LS-align: an atom-level, flexible ligand structural alignment algorithm for high-throughput virtual screening. Bioinformatics. 2018;34(13):2209–2218. doi: 10.1093/bioinformatics/bty081
- Fan C, Wong P-P, Zhao H, et al. DStruBTarget: integrating binding affinity with structure similarity for ligand-binding protein prediction. J Chem Inf Model. 2020;60(1):400–409. doi: 10.1021/acs.jcim.9b00717
- Ying Song SZ, Niu Z, Zhang-Hua F, et al., Communicative representation learning on attributed molecular graphs. Proceedings of the Twenty-Ninth International Joint Conference on Artificial Intelligence (IJCAI-20 2019, (IJCAI-20), Yokohama, Japan, 7.
- Delaney JS. ESOL: estimating aqueous solubility directly from molecular structure. J Chem Inf Comput Sci. 2004;44(3):1000–1005. doi: 10.1021/ci034243x
- Mobley DL, Guthrie JP. FreeSolv: a database of experimental and calculated hydration free energies, with input files. J Comput Aided Mol Des. 2014;28(7):711–720. doi: 10.1007/s10822-014-9747-x
- Knox C, Law V, Jewison T, et al. DrugBank 3.0: a comprehensive resource for ‘omics’ research on drugs. Nucleic Acids Research. 2011;39(Database issue):D1035–41. doi: 10.1093/nar/gkq1126
- Law V, Knox C, Djoumbou Y, et al. DrugBank 4.0: shedding new light on drug metabolism. Nucleic Acids Res. 2014;42(Database issue):D1091–7. doi: 10.1093/nar/gkt1068
- Wishart DS, Feunang YD, Guo AC, et al. DrugBank 5.0: a major update to the DrugBank database for 2018. Nucleic Acids Res. 2018;46(D1):D1074–D1082. doi: 10.1093/nar/gkx1037
- Wishart DS, Knox C, Guo AC, et al. DrugBank: a knowledgebase for drugs, drug actions and drug targets. Nucleic Acids Res. 2008;36(Database issue):D901–6. doi: 10.1093/nar/gkm958
- Wishart DS, Knox C, Guo AC, et al. DrugBank: a comprehensive resource for in silico drug discovery and exploration. Nucleic Acids Res. 2006;34(Database issue):D668–72. doi: 10.1093/nar/gkj067
- Lipinski CA. Lead- and drug-like compounds: the rule-of-five revolution. Drug Discov Today Technol. 2004;1(4):337–341. doi: 10.1016/j.ddtec.2004.11.007
- Yu AM, Choi YH, Tu M-J, et al. RNA drugs and RNA targets for small molecules: principles, progress, and challenges. Pharmacol Rev. 2020;72(4):862–898. doi: 10.1124/pr.120.019554
- Barry G. Integrating the roles of long and small non-coding RNA in brain function and disease. Mol Psychiatry. 2014;19(4):410–416. doi: 10.1038/mp.2013.196
- Blount KF, Megyola C, Plummer M, et al. Novel riboswitch-binding flavin analog that protects mice against Clostridium difficile infection without inhibiting cecal flora. Antimicrob Agents Chemother. 2015;59(9):5736–5746. doi: 10.1128/AAC.01282-15
- Arney JW, Weeks KM. RNA-Ligand interactions quantified by surface plasmon resonance with reference subtraction. Biochemistry. 2022;61(15):1625–1632. doi: 10.1021/acs.biochem.2c00177
- Bernacchi S, Ennifar E. Analysis of the HIV-1 genomic RNA dimerization initiation site binding to aminoglycoside antibiotics using isothermal titration calorimetry. Methods Mol Biol. 2020;2113:237–250.
- Chang Z, Zheng YY, Mathivanan J, et al. Fluorescence-based binding characterization of small molecule ligands targeting CUG RNA repeats. Int J Mol Sci. 2022;23(6):3321. doi: 10.3390/ijms23063321
- Menichelli E, Lam BJ, Wang Y, et al. Discovery of small molecules that target a tertiary-structured RNA. Proc Natl Acad Sci U S A. 2022;119(48):e2213117119. doi: 10.1073/pnas.2213117119
- Zeller MJ, Nuthanakanti A, Li K, et al. Subsite ligand recognition and cooperativity in the TPP riboswitch: implications for fragment-linking in RNA ligand discovery. ACS Chem Biol. 2022;17(2):438–448. doi: 10.1021/acschembio.1c00880
- Agoni C, Olotu FA, Ramharack P, et al. Druggability and drug-likeness concepts in drug design: are biomodelling and predictive tools having their say? J Mol Model. 2020;26(6):120. doi: 10.1007/s00894-020-04385-6
- Hoffman AS. Hydrogels for biomedical applications. Adv Drug Deliv Rev. 2002;54(1):3–12. doi: 10.1016/S0169-409X(01)00239-3
- Gil-Ramirez A, Caz V, Martin-Hernandez R, et al. Modulation of cholesterol-related gene expression by ergosterol and ergosterol-enriched extracts obtained from Agaricus bisporus. Eur J Nutr. 2016;55(3):1041–1057. doi: 10.1007/s00394-015-0918-x
- Hassan SE, Fouda A, Radwan AA, et al. Endophytic actinomycetes Streptomyces spp mediated biosynthesis of copper oxide nanoparticles as a promising tool for biotechnological applications. J Biol Inorg Chem. 2019;24(3):377–393. doi: 10.1007/s00775-019-01654-5
- Hou Y, Li X, Liu X, et al. Transcriptomic responses of Caco-2 cells to Lactobacillus rhamnosus GG and Lactobacillus plantarum J26 against oxidative stress. J Dairy Sci. 2019;102(9):7684–7696. doi: 10.3168/jds.2019-16332
- Katayama S, Xu X, Fan MZ, et al. Antioxidative stress activity of oligophosphopeptides derived from hen egg yolk phosvitin in Caco-2 cells. J Agric Food Chem. 2006;54(3):773–778. doi: 10.1021/jf052280d
- Lindemann G, et al. Limited heat-shock protein 72 induction in Caco-2 cells by L-glutamine. Digestion. 2001;64(2):81–86. doi: 10.1159/000048844
- Yu M, Wang Q, Ma Y, et al. Aryl hydrocarbon receptor activation modulates intestinal epithelial barrier function by maintaining tight junction integrity. Int J Biol Sci. 2018;14(1):69–77. doi: 10.7150/ijbs.22259
- Gu S, Papadopoulou N, Nasir O, et al. Activation of membrane androgen receptors in colon cancer inhibits the prosurvival signals Akt/bad in vitro and in vivo and blocks migration via vinculin/actin signaling. Mol Med. 2011;17(1–2):48–58. doi: 10.2119/molmed.2010.00120
- Swank GM, Lu Q, Xu D-Z, et al. Effect of acute-phase and heat-shock stress on apoptosis in intestinal epithelial cells (Caco-2). Crit Care Med. 1998;26(7):1213–1217. doi: 10.1097/00003246-199807000-00023
- Hermann T. Small molecules targeting viral RNA. Wiley Interdiscip Rev RNA. 2016;7(6):726–743. doi: 10.1002/wrna.1373