Abstract
The primary T-cell-dependent antibody response (TDAR) to antigens is widely used as a functional test in immunotoxicology. TDAR assays can be performed using different antigens, e.g., sheep red blood cells (SRBC) and keyhole limpet hemocyanin (KLH) are presently utilized by different laboratories. The purpose of this study was to apply meta-analysis to compare data from several laboratories using these antigens, in two strains of rats, by multiple routes of immunization and with different primary TDAR assay formats. Although limitations imposed by the scale of the data set and the need to convert some data to an ordinal scale to allow comparisons restrict the scope and strength of the conclusions that can be drawn, the results of this study have shown that the two antigens in different assay formats give comparable results. Similarly, both antigens and assays formats showed the same pattern of response to strong immunotoxicants. These results indicate that standardizing the choice of antigen in TDAR assays may not be critical in the evaluation of potential immunotoxicity.
Keywords :
INTRODUCTION
Meta-analysis is the statistical analysis of a large collection of analytical results from individual studies for the purpose of integrating the findings (Glass, Citation1976). Meta-analysis is used to identify sources of variation among study findings and, when appropriate, to provide an overall measure of effect as a summary of those findings (Berlin et al., 1993). The purpose of the work was to collect data on TDAR tests from a number of pharmaceutical and chemical company laboratories and to determine if these data were suitable for a statistical meta-analysis, to define the primary sources of variation in the data, e.g., immunization routes, strain differences, and to compare the antibody responses to different antigens, specifically, SRBC and KLH. This work was conducted under the auspices of the Immunotoxicology Technical Committee, ILSI-HESI. The statistical analysis was conducted primarily by Kim and Berlin. This proceedings paper represents an abbreviated treatment of this analysis. The full analysis, with detailed methods and discussion is the subject of a paper by Kim et al. (submitted for publication).
METHODS
The companies (Companies contributing data are listed in the acknowledgement section.) submitted data in Microsoft Excel spreadsheets with information on species, strain, number of animals, sex, immunogen, route of immunization, measured endpoints for antibody response (IgM and IgG: concentration, titer, plaque cell counts) and, when available, treatment regimens with known immunosuppressive agents (e.g., cyclophosphamide, cyclosporine and dexamethasone). These raw data were first screened for their suitability for inclusion in the subsequent analysis based on availability of individual animal data and the common antigens (i.e., SRBC or KLH) used in primary (after one immunization) TDAR tests in rats. Next, the data were assessed for common rat strains that were used in studies with SRBC and/or KLH by different routes of administration (intravenous or footpad). Each laboratory followed their own protocols for conducting the assays and dosing the immunosuppresssive agents. No prospective standardization was performed.
Overview of Statistical Methods
The statistical analyses were aimed at addressing the broad question of whether antibody responses are comparable between animals receiving SRBC versus animals receiving KLH. This overall question was addressed first in analyses including only control animals, i.e., those not receiving any immunosuppressive drugs (positive controls). In subsequent analyses, experiments involving positive controls were used to ask whether the measured effects of known, effective, immunosuppressive drugs depend on the choice of antigen.
Analyses Involving Control Rats
Because of the limitations of the available data we had to apply data transformation. Various forms of regression analyses were used to perform the tests of interest. Some of these analyses were conducted using a given form of the measured endpoint, for example, for titers linear regression was used. However, we were unable to run a model that was inclusive of all variables as predictors. Therefore, additional linear regression models were used to analyze standardized measured endpoints. Specifically, as an alternative way of assessing the antigen effect, we chose to standardize the data within each outcome type, which can be done by subtracting the mean and dividing this by the standard deviation. With these standardized scores, similar in nature to a z-score, we could combine the scores from each outcome type and fit a usual linear regression model. To confirm the results of the linear regressions, the scores were parsed into five roughly equal-sized groups and analyzed using an ordinal cumulative logistic regression model on the combined ranks.
Prior to standardizing each outcome variable, we examined its distribution. Except for the titer measurements, other measurement types were highly asymmetric. The natural log within each outcome type was found to have a distribution more closely approximating normality. As a result, the transformation converted all outcome measures to the log scale (log base 2 for titers), and then standardized, within each outcome type, i.e., by subtracting the mean and dividing by the standard deviation separately for log concentration, log plaque, and titer. These values were then parsed into five equal sized groups for the ordinal logistic regression analyses. Once these standardized scores were calculated within each outcome type, a linear regression model was fit and the antigen effect tested, along with the effects of other variables.
Comparison of Drug Effects Between Antigens
We performed meta-analysis of the drug effect studies using the statistical package STATA (Version 8.2) to determine the effect size. The effect size for each antigen-antibody pair was estimated using the standardized difference of the means (on the log scale) between the control and the drug group. The logarithmic scale was preferred to the original scale because the data came closer to meeting the assumption of a normal distribution on the log scale.
To address the issue that the studies used different measurements (concentrations, titers, or plaques), analyses were initially conducted using standardized mean differences (SMDs). These differences are expressed in terms of standard deviation units, which allow inclusion of measurements made on different scales. The overall estimate of the effect is a weighted average of the within-study results. The models used were so-called “random-effects” models, which allow for variability in the drug effects across studies by inflating the estimated variance of the drug effect in proportion to that among-study variability (Berlin et al., Citation1989).
Tests of Significance
A p-value of 0.05 was used in determining the statistical significance of a variable within a model. All models were fit allowing for additional variability among experiments. That is, conditional on all aspects of the experimental design and the animals used, the models allow for additional variation in antibody response attributable to other unmeasured differences among studies. This was accomplished by using Generalized Estimating Equations (Zeger et al., Citation1988). All models were fit using the SAS statistical package (Version 8.1, SAS Institute, Cary, NC) or STATA (Version 8.0, STATA Corporation, College Station, TX).
RESULTS
Specific Comparisons in Control Rats
The results of the analysis of raw, i.e., unstandardized, data for the control rats (Sprague–Dawley and Wistar-Han) immunized with either KLH or SRBC are summarized in . Comparing the responses across antigens, the results suggest that KLH resulted in higher IgM responses than SRBC. Comparing across route of KLH immunization in Sprague–Dawley rats, there was a stronger response from footpad injection than from intravenous (IV) administration for both IgM and IgG.
TABLE 1 Results for control rats (Unstandardized)
Additional comparison of the responses between strains indicates that Wistar-Han rats had slightly stronger IgM responses to SRBC than Sprague–Dawley rats. Similarly, for KLH immunization, Wistar-Han rat IgM responses were higher than those in Sprague–Dawley rats, although lower for IgG antibodies.
The results of the analysis of standardized data are summarized in . While comparative analyses for the responses across antigens showed consistent results without and with standardization of the data, this process enabled analysis of more comparisons, i.e., effect of gender and effect of route of immunization in addition to the effect of strain, than were possible with unstandardized data. The coefficients and the standard errors for the antigen effect in models that included the gender effect were not appreciably different from those from models that did not include the gender effect. For route of administration, the footpad injection of KLH induced greater antibody responses than the intravenous injection of KLH in Sprague–Dawley rats. For strain effects, the IgM response in Wistar-Han appeared stronger than in Sprague–Dawley rats in both SRBC and KLH tests while the standardized IgG responses to KLH in Wistar-Han rats were weaker than in Sprague–Dawley rats.
TABLE 2 Results for control rats (Standardized)
Comparison of Drug Effects in Rats
Meta-analysis of drug effects was conducted for data generated in the common rat strain, Sprague–Dawley, using the same route of immunization, intravenous injection of either SRBC or KLH. The three drugs with enough data to be studied were cyclophosphamide, cyclosporine and dexamethasone. The cyclophosphamide effect can be tested for both SRBC and KLH antigens (IgM and IgG), the cyclosporine effect can be tested only for KLH (IgM and IgG) and the dexamethasone effect only for IgM responses of rats that received SRBC. The results for the drug effects across experiments are shown as Forest plots in and and are summarized in .
FIG. 1 Effects of dexamethasone and cyclophosphamide on rat primary IgM response following intravenous injection of SRBC. Forest plots of standardized log-mean differences for anti-SRBC IgM responses: the area of the box represents how much each study contributes to the meta-analysis (% weight) and the center of the box shows the standardized mean drug effect size (i.e., in standard deviation units, with the underlying data on the log-scale) for each study. The overall effect size and the corresponding confidence interval are summarized at the bottom of the forest plot in a hollow diamond symbol.
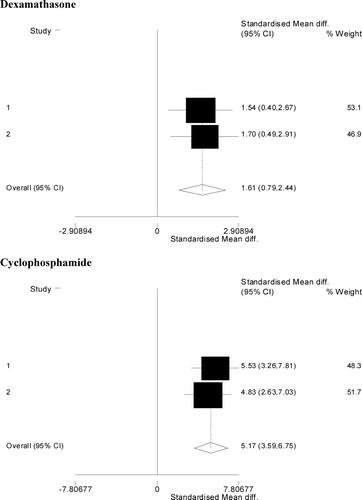
FIG. 2 Effects of cyclophosphamide or cyclosporine on rat primary IgM response following intravenous injection of KLH. Forest plots of standardized log-mean differences for anti-KLH IgM responses: the area of the box represents how much each study contributes to the meta-analysis (% weight) and the center of the box shows the standardized mean drug effect size (i.e., in standard deviation units, with the underlying data on the log-scale) for each study. The overall effect size and the corresponding confidence interval are summarized at the bottom of the forest plot in a hollow diamond symbol.
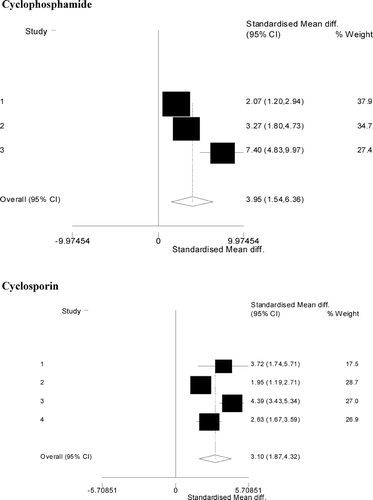
TABLE 3 Results for drug effects
In rats immunized with KLH, the effect of cyclophosphamide on the IgM and IgG response was statistically significant. However, the heterogeneity across the studies was also statistically significant. Similarly, the effect of cyclosporine on IgM but not IgG responses of rats that received KLH is statistically significant and the heterogeneity of treatment effects across studies is also statistically significant.
In rats immunized with SRBC, similar drug effects were found. Cyclophosphamide significantly suppressed the IgM and IgG response to SRBC. However, the heterogeneity across the studies was also statistically significant. For dexamethasone, the IgM response to SRBC was significantly suppressed and no significant heterogeneity across the two studies was found.
We then determined if there was a significant difference in the drug-mediated suppression across the two antigens. The sparseness of the data restricted our analysis to comparing the drug effect between antigens in rats treated with cyclophosphamide. As shown in , the cyclophosphamide effect sizes (in standard deviation units) were: 3.95 (95% CI: 1.54, 6.36) for KLH and 5.17 (95% CI: 3.59, 6.75) for SRBC. Using a random effects regression model for effect sizes, the drug effects do not differ statistically significantly between the antigens. The results using the ordinal logistic model agreed with those of the regression on effect sizes, i.e., the difference in drug effect of cyclophosphamide did not differ significantly between the antigens.
TABLE 4 Results for effect of cyclophosphamide across antigens (random effects regression model)
In summary, the results of the meta-analysis indicate that despite the heterogeneity of effects among studies, there is a large and highly statistically significant drug effect within each antigen-antibody pair. The results also indicate that comparison of drug effects should be done within the same species, the same strain and the same route of antigen administration.
CONCLUSIONS AND RECOMMENDATIONS
No differences in the outcome of SRBC and KLH studies with known immunosuppressive drugs, administered as “positive controls,” indicate that inter-laboratory standardization of TDAR tests may not be necessary. However, more work is needed to determine drug-dose response and value of inclusion of additional endpoints, e.g., primary IgG antibody response in TDAR tests.
The author gratefully acknowledge and thank ILSI HESI Immunotoxicology Technical Committee for coordinating and supporting this work, Drs. Jesse Berlin and Carin Kim for conducting the meta-analyses and representatives of the following listed companies for providing data for the analytical purposes: Dr. Herve Lebrec, 3M Pharmaceticals (Saint Paul, MN); Dr. Hans-Werner Vohr, Bayer AG (Wuppertal, Germany); Dr. Helen Haggerty, Bristol-Myers Squibb Co. (Syracuse, NY); Dr. Pauline Martin, Centocor, R&D (Radnor, PA); Dr. Gregory Ladics, E.I. DuPont Co. (Newark DE); Dr. Michael Woolhiser, The Dow Chemical Company (Midland, MI); Dr. Daniel Wierda, Eli Lilly and Company (Greenfield, IN); Dr. Danuta Herzyk, GlaxoSmithKline (King of Prussia, PA); Dr. Miklos Csato, F. Hoffmann-La Roche Ltd (Basel, Switzerland); Dr. Catherine Kaplanski, Merck Research Laboratories (West Point, PA); Dr. Peter Ulrich, Novartis Pharma AG (Basel, Switzerland); Dr. Joseph Piccotti, Pfizer Global Research and Development (Ann Arbor, MI); Dr. Brigitte Molinier, Sanofi-Aventis (Montpellier, France); Dr. Ian Kimber, Syngenta CTL (Cheshire, United Kingdom) and Dr. Richard Pfeifer, Wyeth Research (Andover, MA).
REFERENCES
- Berlin J. A. Invited commentary: Benefits of heterogeneity in meta-analysis of data from epidemiologic studies. Am. J. Epidemiol. 1995; 142: 383–387
- Berlin J. A., Laird N. M., Sacks H. S., Chalmers T. C. A comparison of statistical methods for combining event rates from clinical trials. Stat. Med. 1989; 8: 141–151
- Glass G. V. Primary, secondary and meta-analysis of research. Educ. Res. 1976; 10: 3–8
- Kim C., Berlin J. A., Bugelski P. J., Haley P., Herzyk D. J. Comparison of immune functional tests using T-dependent antigens in immunotoxicology studies: A meta-analysis. 2007, Submitted
- Zeger S. L., Liang K. Y., Albert P. S. Models for longitudinal data: A generalized estimating equation approach. Biometrics 1988; 44: 1049–1060