ABSTRACT
A hybrid workstyle is becoming more common post-COVID-19, and longer occupancy hours at home are increasing household electricity consumption. Small homes are regarded as a potential for improving energy efficiency in the residential sector, and a home consists of mixed-function spaces with dynamic occupancy behaviors. These underpin the opportunity to optimize presence sensing lighting in small homes for energy efficiency and user-behavioral needs. A comprehensive overview of presence sensing approaches, comparing four types of non-wearable sensors connected to home lighting is made. A bibliometric mapping of the reviewed literature visually reinforces a significant research gap that presence sensing studies were not connected to home lighting but inclined toward the commercial and institutional context. Next, a non-exhaustive example of commercially available presence sensing products applicable to residential lighting for small homes is analyzed, and their general characteristics and technologies are synthesized. The literature and product overview identified five significant product knowledge gaps. Incorporating the gathered information leads to the proposal of a conceptual flexible radar-based sensor (prototype design), addressing a wish list with three important criteria to optimize future presence sensing lighting in a mixed-function small home. Future radar sensing studies are expected to develop an anticipatory lighting system that processes real-time multi-user vital signals for smarter localized and personalized lighting options for (small) living environments.
1. Introduction
After the COVID-19 pandemic, a home is no longer limited to a retreat place, where most people leave each morning and return each evening. The pandemic has intensified the importance of achieving desired comfort levels for remote working at home (Tleuken et al. Citation2022) and satisfaction in lighting for mental health (Bergefurt et al. Citation2022). As a hybrid workstyle becomes more common post-pandemic, longer occupancy hours at home are increasing household electricity consumption (Kawka and Cetin Citation2021). Aldubyan and Krarti (Citation2022) reported that stay-home living during the pandemic lockdown period led to high demands for heating/cooling, lighting, and usage of electronic appliances, thus increasing overall household electricity consumption, about 16% more in Saudi Arabia, 14% more in Australia, and up to 25% more in Great Britain when peak demand occurs. With energy prices skyrocketing and remote homeworking expected to be prevalent post-pandemic, energy efficient strategies for the residential sector have become a vital national parliament agenda in Sweden, as it is for many other countries. According to Statistics Sweden, the residential sector (small, multi-family, and holiday houses) consumed almost one-third (28%) of Sweden’s total electricity power in 2019 (Statistics Sweden Citation2021a), and users of small houses were the second-largest final consumers of electricity (Statistics Sweden Citation2021a). Interestingly, home lighting accounted for up to 15% to 30% of the household electricity usage in Sweden (Energimyndigheten Citation2011; Gerhardsson et al. Citation2019; Palacios-Garcia et al. Citation2015), which is almost comparable to 17% in Brazil (de Souza et al. Citation2019), 20% in Cameroon (Enongene et al. Citation2017), and 20% to 35% in Egypt (Attia et al. Citation2017). Hence, identifying optimal approaches to reducing electric lighting energy consumption in (small) residential environments is crucial for operational cost savings through reduced electricity bills and lessening the built environment’s impact on the climate.
1.1. Common electric lighting energy saving strategies for homes
A home lighting system has the potential to achieve energy savings through three common optimizing strategies. The first strategy is by using energy efficient lamps and adopting newer lamp technologies. For example, a simulation study by Moadab et al. (Citation2021) identified that substituting compact fluorescent lamps (CFL) with light-emitting diodes (LED) resulted in 33% to 35% annual energy savings for a Swedish apartment. A study in Spanish homes observed 40% reduction in energy consumption when 50% of the home’s existing lamps were replaced with LED, and the energy saving increased to 65% when 80% of the lamps were LED (Palacios-Garcia et al. Citation2015). The rapidly growing LED technology offers lamps with higher lumen per Watt at lower energy consumption and is spectrally tunable. The newer LED lamp provides more lumens, thus lessening the number of luminaires needed to be switched on, appropriated for the energy savings goal and value-added well-being features. Even though LED lighting consumes less energy, the wasted light practice by leaving unnecessary lights on in unoccupied spaces can be minimized with the following strategies.
The second strategy is to opt for effective automated lighting control systems like occupancy detection or daylight harvesting. This strategy uses sensors to help decrease energy use by turning off the lights, minimizing the extended periods when lights are left on at the highest light output (scene brightness) in unoccupied spaces, or reducing the illumination levels when daylight is available (Safranek et al. Citation2021). Moadab et al. (Citation2021) projected that a smart lighting system that dims the room lighting automatically based on occupancy patterns achieved the lowest energy consumption when compared to a home with conventional CFL and LED lighting. However, the convenience of such implementation at home relies on the timeout period. Setting the sensor with a long time delay may result in energy wastage but ensures the lights are not switched off in occupied spaces (Tiller et al. Citation2010); while shorter time delay offers greater energy savings but could be annoying when the sensor turns off the lighting with users being present (Nguyen and Aiello Citation2013). Another challenge with the shorter timeout is that it may ensue increased maintenance and lamp replacement costs (Dikel et al. Citation2018). This is primarily the case for homes still using older lamp technologies, like fluorescent lamps in Italy (Pompei et al. Citation2022). Nevertheless, the increase in switching frequency is not a concern if LED lamps are used as they are not impervious to lifetime degradation. For an office environment, Dikel et al. (Citation2018) highlighted that reducing the timeout period of a LED lighting control system from 20 minutes to 1 minute resulted in 26% energy savings.
The third strategy is understanding user’s behavior and activities, which according to Nguyen and Aiello (Citation2013) and Moadab et al. (Citation2021), have a significant influence on electric lighting energy consumption. Palacios-Garcia et al. (Citation2015) observed lighting demand peaks in the morning and night during weekdays, but during weekends the morning peak was reduced (due to later wake-up times) and night peak was increased (due to later bedtimes). This coincides with a difference in the activity of household occupancy patterns between weekdays and weekends. Soheilian et al. (Citation2019) observed higher energy consumption during a weekend than a weekday, and for activities requiring task lighting like cooking compared to eating in the kitchen. However, most of the energy saving and sensing technologies focus on the first strategy (lamp technology) and second strategy (occupancy detection) rather than user’s behavior characteristics (Dong et al. Citation2018; Williams et al. Citation2012). Sensing technologies are evolving rapidly over the last few years, adopting to the connected data-driven technologies of the Internet of Things (IoT) to support user’s day-to-day lives in (smart) homes. Therefore, there is a need to study how the sensors as part of a lighting control system can energy-efficiently support user’s behavioral needs in homes.
1.2. Incorporating presence sensing technologies that support energy efficiency for post-pandemic dynamic occupancy behavior in homes
Expanding from the need highlighted in the third strategy, it is also important to consider presence sensing lighting control for the post-pandemic dynamic occupancy behaviors at home, which has yet to be researched for its influence on electric lighting energy consumption. A home consists of mixed-function spaces where different activities are bound to occur in the same room, especially in small homes. Users behave in different ways at various times throughout the day for work, learning, entertainment, or retreat purposes (Chu et al. Citation2022; Ticleanu Citation2021). Lighting a small home with an open-plan layout and dynamic occupancy behaviors can be tricky, especially when determining whether to have bright general lighting in the living room for activities like reading, or pleasant decorative lighting for coziness. Those using automated lighting control systems may have experienced frustration at some point in time when the lighting and its sensing system led to mismatched responsiveness. A user working in a sedentary position could be annoyed if the lighting automatically dims after the pre-set time delay until it is reactivated. The user may dismantle or sabotage the lighting system that causes inconvenience or change his/her behavior to suit the installed lighting system.
Several studies have shown a direct relationship between house size and operational energy use, where small homes (less than 37 m2) are regarded as a potential for improving energy efficiency in the residential sector (Crawford and Stephan Citation2020; Wotton et al. Citation2018). The average floor space per person in a small home ranged from 14 m2 to 31 m2 in some Asian countries (like Hong Kong, Japan, and Singapore) and ranged from 31 m2 to 65 m2 in a number of the EU countries (Wilson Citation2022; Wotton et al. Citation2018). According to Statistics Sweden (Citation2021b), Sweden provides an average living space of 42 m2 per person (). These data underline the opportunity to optimize short-range presence sensing lighting in small homes as input for energy efficient and user-responsive smart lighting systems. A small home with mixed-function spaces and dynamic occupancy behaviors may require alternative presence sensing techniques and product applications than those developed for bathrooms, corridors, and commercial and institutional buildings. Therefore, the main objective of this paper is to compare short-range presence sensing technologies that support energy efficiency and user-behavioral needs in a small home. The research question explores the ideal type of sensors, the localized placement, and quantity for optimizing presence sensing lighting in a mixed-function small home. It queries if optimization could be realized following the “Less is moreFootnote*” concept.
Fig. 1. The average living space per person by dwelling type (range: 29 m2 – 47 m2) based on a survey of 4,776,239 households in Sweden on 31 December 2020 (after Statistics Sweden Citation2021b).
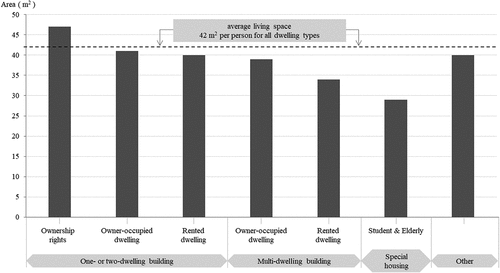
The rest of this paper is organized as follows. Section 2 describes the methodology employed (in three parts) for studying presence sensing approaches, technologies, and products. Section 3 reviews the commonly used sensor-integrated lighting technologies, provides a quick bibliometric analysis of the literature, and synthesizes the currently available presence sensing products. Section 4 discusses the product knowledge gaps and a proposed concept for optimizing future presence sensing lighting in mixed-function small homes. Finally, Section 5 presents the conclusions.
2. Methodology: exploring presence sensing approaches, technologies, and products
The exploration of presence sensing approaches and technologies started with a scoping review of the literature (part 1), followed by a bibliometric mapping of the reviewed literature (part 2) and a synthesis of the current commercially available presence sensing products (part 3).
The PRISMA-ScR checklist for Scoping Reviews is developed to retrieve and refine a literature search (Munn et al. Citation2018; PRISMA-ScR Checklist Citation2021; Tricco et al. Citation2018). The purpose was to get an overview of the presence sensing approaches connected to home lighting, identifying the research developments, knowledge gaps, and recommendations for future studies. A broad search strategy for peer-reviewed research and review articles published in English was adopted, using the online databases Google Scholar, Scopus, Science Direct, Sage, and Web of Science. The search was conducted from January 2022 and was regularly repeated until May 2022 using a fixed set of keywords. First, the overview search used generic keywords such as ‘presence sensing’ AND ‘residential’ AND ‘indoor lighting’ under all fields category, with records dating from 2010 to 2022. Next, relevant records were identified from the title, limited to the first 100 hits randomly sorted by relevance in each database. The abstracts of the identified records were then screened for subject relevance with the objective of this paper. After that, a snowball search was performed from the records included in this review. New reference lists and keywords were incorporated to refine the scoping review. In addition, organizational websites were appraised for commercially available sensing products, recommendations, and research developments (master and doctoral thesis). A total of 102 records were identified by title, and 36 publications were reviewed, as shown in .
Fig. 2. The flow diagram summarizing the conducted scoping review based on the PRISMA-ScR checklist.
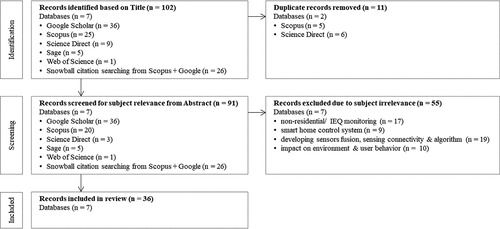
Part 2 of the literature analysis included a bibliometric mapping of the 36 reviewed publications from Part 1. It was conducted to explore the reviewed literature’s knowledge networks and identify important areas/field gaps in a map. A free online program, VOSviewer (version 1.6.18) was used to construct and visualize the bibliometric networks of the 36 reviewed publications (van Eck et al. Citation2010; Zhang et al. Citation2018). The bibliometric analysis threshold was set to three minimum number of occurrences for a term. Terms that contributed to comparative context were gathered as synonyms to avoid repetition of similar terms, and 17 generic words (redundant nouns) were excluded from the analysis. The bibliometric analysis identified 135 keywords that met the threshold of 1,222 terms. Detailed examination and network mapping of specific terms were facilitated by selecting and zooming the terms in VOSviewer. visually presents the current state of research development regarding presence sensing from the title and abstract of the 36 reviewed publications. It reveals a persisting lack of research on presence sensing for homes. This is consistent with Bladh and Krantz (Citation2008), Gerhardsson et al. (Citation2019), and Moadab et al. (Citation2021), who stressed the lack of energy efficient lighting control research in the residential sector.
Fig. 3. Network and density visualization mapping of (a) the most occurred terms currently derived from the title and abstract of the 36 reviewed publications; detailed examination of term (b) occupancy detection; (c) presence detector. The map is best viewed in color available from the online version of the figure.
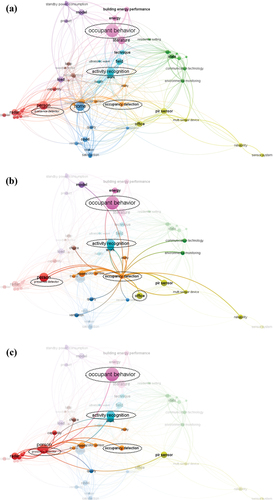
Part 3 of the literature analysis reviewed various off-the-shelf presence sensing technologies applicable to home lighting based on the theoretical information from Part 1. Information about the currently available presence sensing products was gathered from various organizational websites, and their sensing characteristics and technologies have been synthesized.
3. Results: presence sensing approaches, technologies, and products for home lighting
This section presents an overview of the presence sensing approaches connected to home lighting, the output from the bibliometric analysis of the reviewed publications, and the current commercially available presence sensing products.
3.1. Research landscape of presence sensing approaches
A sensor could take the form of a module, device, or subsystem. It detects signals or environmental changes and relays the information as electrical or digital impulses to the micro- or computer processor (Javaid et al. Citation2021). Human presence sensing refers to the sensor technology used to detect if a person is present in the monitored area. Wearable and non-wearable presence sensing devices are used to track and monitor the user’s occupancy information.
Wearable sensors connected to a smart home lighting system are usually employed to monitor the user’s location, body movements, or vital signals (like cardiac heartbeats and respiratory breathing). Commercially available watch-like wearable sensors networked with mobile devices can sense user’s physiological measures, pressure, sleep quality, lifestyle activities and can act as a controller to operate the home lighting apps, making the system user-specific. Although lighting systems are moving toward personalization, the challenges are that wearable sensors may be less feasible and not necessarily be a reliable source of data for a residential context. Users may opt not to wear the sensor at any time while at home and would often experience inconvenience when used during activities like sleeping or bathing. In addition, prolonged usage may cause discomfort or skin irritation to sensitive users.
As for the non-wearable sensors, there are the object-tagged and dense sensing (environment tagged) options, a less intrusive sensing approach for users. Object-tagged sensors are additional sensing devices (bridge, anime robots, or portable lamps) synced to the connected lighting system (Hussain et al. Citation2020). However, it may not be a convenient option as it bounds the user to deploy additional items on top of the lighting system, thus increasing maintenance efforts and cost of installation and operation. Moreover, having a number of object-tagged sensors on standby could indirectly contribute to a slight increase in the grid’s load. A multi-sensor approach of having several object-tagged sensors may provide better coverage for large areas (Yavari et al. Citation2014b) but may not be efficient for small homes due to potential configuration and logical zone overlaps, leading to conflicting and unnecessary lighting output. Alternatively, dense sensing is when sensors are embedded in the luminaire or environment (like tags attached to a wall). Dense sensing may deploy many different sensors, such as motion, pressure, temperature, and proximity sensors in the environment to capture user’s activity. It is becoming a popular sensing option because it gives users independence from additional object-tagged devices or wired to wearable sensors that could alter their behavior (Hussain et al. Citation2020; Woodstock Citation2020).
The operation of non-wearable sensors can be categorized into passive and active sensing. A passive sensor operates by receiving signals to recognize the object’s presence. On the other hand, an active sensor operates by emitting signals and then receiving the signals bounced from the objects. Active sensors are known to consume more energy than passive ones due to the continuous probing of signals within their coverage zone (Woodstock Citation2020). Some of the latest developed sensors have incorporated passive and active sensing to improve their robustness and reliability. This paper compared four types of non-wearable sensors – Visual sensors, and Passive Infrared (PIR) sensors for passive sensing; Ultrasonic sensors, and Radio Detection And Ranging (Radar) sensors for active sensing.
3.1.1. Visual sensors
Visual sensors use imaging techniques such as camera and body recognition scanners to detect stationary and in motion occupancy and user activities (Benezeth et al. Citation2011). Examples of visual sensors include the classical monocular, omnidirectional, RGB-D (depth sensors like the Kinect), and more sophisticated 3D systems (Reinoso and Payá Citation2020). A visual sensor captures the largest quantity of information from the environment and is increasingly used as a source of information to solve diverse tasks which prioritize accuracy. However, they are flagged as invasive in terms of perceived privacy () despite providing the richest information about user’s activities, occupancy, and environmental conditions (Debes et al. Citation2016; Fioranelli et al. Citation2019). Low-resolution cameras have been developed to gather enough information regarding a user’s position and movement status, yet there is still some psychological resistance as personal images are captured (Benezeth et al. Citation2011).
Fig. 4. Classification of sensing technologies as a function of richness of information and perceived privacy (after Debes et al. Citation2016).
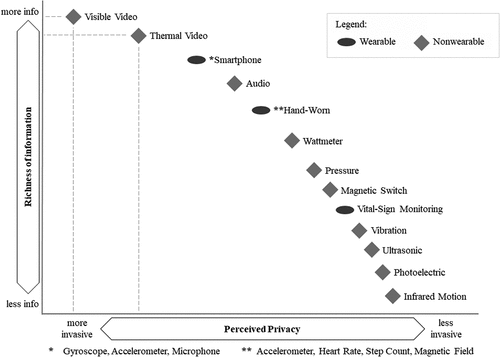
3.1.2. Passive Infrared (PIR) sensor
A Passive Infrared (PIR) sensor is a thermal infrared sensor that detects the movement of objects in its detection zone. As a motion sensor, it operates by sensing the changes in infrared signals (temperature patterns) emitted by various objects in the environment. The sensor has two equal slots made up of pyroelectric material that is very sensitive to infrared radiation. A positive differential change occurs between the two halves when a warm object moves closer to the detection area. Similarly, a negative differential change occurs between both slots when the object leaves the detection area. Both the slots sense the same number of infrared radiation when an object is inactive. Mounting a PIR sensor depends on its field of view (Bachouch et al. Citation2023), and the most common position manufacturers usually recommend is the ceiling-mounted option at the center of the room. An alternate possibility is to place the sensors in the corner of the room, at a height and angle covering most of the user’s torso, the body part that radiates most energy (Extronic Elektronik AB). The advantage of a PIR motion sensor is that it offers accuracy in detecting moving objects, even in dark conditions. It is straightforward and generally consumes less energy, as claimed by the commercial market. However, it has limitations, as it is mainly unable to detect stationary objects or micro movements (for example, typing), which takes up almost 50% of the total occupancy rate (Wu and Wang Citation2021). Furthermore, a PIR sensor is not a presence detector and is prone to false negatives,Footnote† turning the lights off when a user has been idle in a quasi-static position (watching TV or sleeping) after the pre-set time delay (Santra et al. Citation2018; Thorström and Anderson Citation2020). It cannot distinguish humans from animals/non-living objects and thus is susceptible to false positivesFootnote‡ triggered by pets or objects blown by the wind. It is also sensitive to heat fluctuations caused by heating elements or windows and could miss a user’s movement if he/she enters after another person has triggered a heat change in the detection zone (Benezeth et al. Citation2011; Woodstock Citation2020).
3.1.3. Ultrasonic sensors
Ultrasonic waves are elastic waves with frequencies greater than 20,000 Hz, above the range of human hearing of approximately 20 to 20,000 Hz (Ihara Citation2008). An ultrasonic sensor operates by emitting ultrasound waves that are above the human audible frequency (Garcia et al. Citation2019; Yavari et al. Citation2014a), and any moving object within its detection zone disturbs the waves, resulting in activating the lighting (Acuity Brands Citation2017). The distance of an object to the sensor is determined by the time the wave returns to the sensor. One of the outstanding advantages of ultrasonic sensing is its capability to propagate through any media, including solids and liquids, except vacuum (Ihara Citation2008). Consequently, this could trigger false positives when movements are detected from activities in neighboring rooms (Ahmad et al. Citation2016) which could be disadvantageous for small homes. The ultrasonic sensor does not require a fixed line-of-sight like the PIR sensor because the room surfaces reflect the waves (Ahmad et al. Citation2016). Unfortunately, the waves are highly sensitive to reflective surfaces like glass or plastic, leading to false positives (Santra et al. Citation2018). Another drawback of ultrasonic sensors is that certain materials like cloth and foam absorb the waves. This creates a tricky situation for occupancy detection in homes because a user covered in multiple layers of clothing reduces the sensor’s sensitivity in detecting his/her motion consistently (Yavari et al. Citation2014a). There are also health and safety concerns with ultrasonic sensors. Even if the sensor is safe for humans, prolonged exposure to ultrasound may affect users using hearing aids and certain animals with ultrasonic hearing ability (Garcia et al. Citation2019; Yavari et al. Citation2014b). In addition, an ultrasonic sensor has high sampling rates and generates a lot more data than other sensors, making data analysis and interpretation a challenge (Dong et al. Citation2018).
3.1.4. Radio Detection And Ranging (Radar) sensors
Radar was initially associated with the defense and military sectors but is becoming a relevant exploratory sensing technology in the engineering and healthcare sectors due to its contactless detection and tracking of one or more objects. Radar can refer to systems that use microwaves or radio waves (Lucas Citation2019). A radar sensor operates by letting its antenna emit signals in the form of microwaves, which move at the speed of light and are not perceivable by humans, just like wireless computer networks and mobile phones (Adib et al. Citation2015; Guo et al. Citation2019). When the waves hit objects, the signal changes and get reflected to the sensor, similar to an echo. The received signal is then processed to identify the position of the objects, direction of movement, speed, and distance to the sensor. Movement toward the radar causes an increase in the frequency of the transmitted signal, thus resulting in a positive Doppler shift, while movements away from the radar lead to a negative Doppler shift (Fioranelli et al. Citation2019; Kalyanaraman et al. Citation2019). Commercially available radar sensors have an adjustable detection zone ranging from less than one meter to 15 meters and can penetrate through light walls, glass, and wood. The advantage of a radar sensor is that it does not produce optical images or videos of the monitored objects like any visual sensor but offers equally rich information (Shukri and Kamarudin Citation2017). In addition, it detects multiple users’ macro motion (running or walking), micro motion (standing still, watching TV, eating, or working on a computer), and vital signals. Users also do not need to wear, carry, or interact with additional devices, modify their daily routine and behavior, or be aware of its presence, as radar sensor preserves users’ privacy, comfort, and perception (Adib et al. Citation2015; Fioranelli et al. Citation2019; Santra et al. Citation2018).
3.2. Presence sensing knowledge networks and developments
The bibliometric mapping in displays nine scattered clusters represented by different colors. The map is best viewed in color available from the online version of the figure. describes each colored cluster and its respective represented knowledge connections. The mapped visualization indicated a loosely connected knowledge network regarding presence sensing for home lighting. Closely overlapping terms were only concentrated within the red (presence detector) cluster around terms like radar sensor development and health indicator measurements.
Table 1. Clusters of knowledge networks and development derived from the bibliometric mapping.
Based on the density visualization (size of circles), a substantial portion of the reviewed publications focused on two dominant terms: occupant behavior and activity recognition. Both terms had the highest total link strength, each with 23 and 13 times of text data co-occurrence, highlighting key research scopes from the reviewed literature. Interestingly, terms such as occupancy detection and presence detector were less represented in the text data derived from the title and abstract of the reviewed publications, each with just 7 and 3 times co-occurrence. Occupancy detection showed stronger (closer) connections with office, work activity recognition, and the PIR sensor (). It had scattered network connections with multi-sensors exploration and modeling, presence detection, indoor air quality, and energy management. On the other hand, the presence detector had a stronger relation and more frequent co-occurrence with radar and health indicator measurements (). Scattered interactions were observed linked to occupancy detection, color and PIR sensors, work activity recognition, and occupant behavior.
The bibliometric analysis brings to attention that presence sensing studies from the reviewed publications were inclined toward commercial settings (office, workspaces), sensor systems modeling and developments, environmental monitoring, and energy performance research. Neither occupancy detection () nor presence detector () were connected to home lighting. This reinforces a significant research gap – a clear contrast of inadequate connection between current presence detection for lighting residential environments despite the exponential growth in sensing technology. This key finding motivated the completion of this paper, to compare short-range presence sensing home lighting technologies as input for energy efficiency and user-behavior needs.
3.3. Current commercially available presence sensing products
Many types of presence sensing technologies are available off-the-shelf, each with advantages and disadvantages, and the solutions are not one-size-fits-all. presents a non-exhaustive example of commercially available presence sensing products (PIR, Ultrasonic, and Radar sensors) applicable for residential lighting in small homes. Given the rise of smart lighting applications, users live an ‘always on’ online mentality. Most sensors are programmed to be connected to the cloud of the IoT platforms for the user to control the home lighting like turning on/off and dimming for energy savings. These control would less likely pose any threats to user’s privacy at home as they have the least security vulnerabilities that hackers can exploit. In contrast, visual sensors are omitted from the analysis due to concerns about invading the user’s privacy (gathering personal image-based information) in a private home environment. In-home Voice Assistants like Amazon’s Alexa that connects Echo smart speaker to IoT light are also omitted as they are conversational agents (Sciuto et al. Citation2018) and not presence sensors. Moreover, voice assistance poses threats to user’s privacy as the technology gathers user information beyond the user’s knowledge (McLean and Osei-Frimpong Citation2019)
Table 2. Synthesis of some of the general characteristics of commercially available PIR, ultrasonic, and radar sensors applicable for residential lighting in small homes (non-exhaustive examples adapted from various organizational websites).
4. Discussion: product knowledge gaps and proposed future presence sensing lighting concept
Five significant product knowledge gaps have been identified from the literature findings of presence sensing approaches (Section 3.1) to the commercially available sensing products (Section 3.3). They have led the authors to propose a concept for optimizing future presence sensing lighting in mixed-function small homes.
4.1. Five identified product knowledge gaps
First, there are almost no or very limited commercially available presence sensing options for residential layouts, more so for a dynamic occupancy behavior in mixed-function small homes. provides evidence that almost all off-the-shelf sensing products offered various sensing options for the nonresidential market catering to circulation spaces (corridors, stairwells, parking), or commercial and institutional layouts (offices, hotels, conference rooms, classrooms, washrooms, functional rooms, hospital wards, warehouses). This insight is consistent with the research gap identified from the bibliometric analysis discussed in Section 3.2. Some of the mentioned spaces may be relevant in homes (for example, corridors, staircases, bathrooms, and a hotel room as a bedroom); however, the sensing products lacked appropriate characteristics for controlling the lighting according to user’s behavioral needs. Moreover, the sensor’s coverage caters to uniform typologies, controlled and single-functioned layouts instead of mixed-function spaces where a living room could be integrated with the kitchen in a small home.
Secondly, there is a lack of appropriate short-range sensing products to support the varied, mixed-function small home layouts. reveals that currently, most sensing products are broad ranged by distance (ranging from 3 meters to 10 meters) and come with wide detection angles (120° to 360°), except for radar, offering prospects of shorter and narrower coverage. Such broad and wide detection coverage may apply better to large commercial and institutional environments through multi-zone lighting control. Such sensors could be used in small homes with living areas between 37 m2 and 42 m2. However, there are possibilities that the logical zones may overlap, thus unnecessarily switching on almost all the lights in the living, kitchen, and bedroom upon the user’s entry or movement between spaces, defeating the energy savings goal. Since the size and layout of the home are influential variables impacting the performance and placement of presence sensors (Bachouch et al. Citation2023), it is crucial to have customizable sensing with short-range zone sizes.
The third identified product knowledge gap is that there are no alternatives in the placement of sensors except for the often ceiling-mounted option recommended by the manufacturers. The recommendation may not be practical for small homes like a one-room apartment with an open-plan layout. Home ceiling profiles may not necessarily be flat and clear (unobstructed). Homes may have ceiling-mounted suspended features like ceiling fans, pendant lamps, and decorative elements, which could obstruct the line-of-sight of PIR sensors. Ultrasonic sensors may have waves reflected from the suspended features, thus increasing false activation (tripping) (Acuity Brands Citation2017). Furthermore, manufacturers rarely explain the sensor’s efficiency and sensitivity if the sensor is mounted vertically or at an angle. Should the sensors be mounted vertically on a wall, limitations would likely be observed where sidelineFootnote§ detection could be less responsive than any front-lineFootnote** movements for certain sensors (Yavari et al. Citation2014a). Positioning the sensor at the center of the wall may not be aesthetically pleasing, while placing it at the end or corner of a wall may compromise its angular efficiency, making it susceptible to false alarms.
The fourth gap relates to several concerns arising from a multi-sensor approach in a mixed-function small home regarding energy efficiency, cost, maintenance, and complexity in data processing. Homes are preemptively becoming artificially intelligent to optimize operational energy efficiency through multiple sensing devices (Woodstock Citation2020). The development of IoT-connected lighting systems facilitates presence detection by networking all the paired sensing devices to assist the user’s daily life. Users have the option to control and personalize lighting conveniently. Switching on/off, dimming, and changing the lighting’s color temperature have revolutionized from control through wall switches to using apps on paired IoT devices. A multi-sensor approach of the present IoT-connected lighting system enables flexible, localized task tuning illumination when and where needed to encourage energy savings in larger built-up spaces (Pandharipande and Caicedo Citation2015). In addition, having a multi-sensor network offers increased accuracy in occupancy detection and can save energy with shorter timeout periods (Pandharipande and Newsham Citation2018; Tiller et al. Citation2010). However, a multi-sensor approach may not necessarily be efficient for small homes. Informal feedback gathered from various organizational websites and blogs of users living in mixed-functioned small homes hinted at inconvenience with a multi-sensor approach due to the sensor’s broad and wide detection coverage, which activates many other luminaires close by than needed. The effectiveness of an energy efficient approach is lost if unwanted lighting is always illuminating on unnecessary triggers. The next concern with a multi-sensor approach is cost and maintenance. Having more sensors would no longer be cost-effective (Tiller et al. Citation2010). The multi-sensor approach cumulatively increases the number of connected sensing devices, operating concurrently for continuous user detection to provide an accurate representation of the occupancy locations and activities (Chu et al. Citation2022; Hayat et al. Citation2019). As a result, all networked lamps, paired sensing devices, and bridges (hub, gateway, network router) will need to be left on standby 24/7/365 or charged more frequently to manage the user’s daily lighting conditions. Such a practice indirectly consumes electricity and may increase the load on the grid. Onaygil and Erkin (Citation2018) observed that hourly testing of two WiFi-connected smart LED lamps, even when turned off remotely, still draws a constant amount of 1 W standby power consumption due to internet connectivity, thus increasing the energy consumption slightly. It may be product-dependent. Next, following the IEC 62301 standard measurement procedure, Dikel et al. (Citation2019) highlighted 21 of the 30 investigated smart LED lamps draw less than 0.5 W standby power consumption. Although the smart LED lamps reflected the maximum allowable standby power consumption, it is inconclusive to measure the percentage savings achieved by the lamps as other gadgets and paired devices are constantly consuming electricity. Besides, there is concern about collected user data and the complexity of data processing required as part of the multi-sensor IoT enabled smart lighting. Human sensing in a private residential environment matters greatly. The tracked user information must preserve the user’s identity, secure sensitive information, be rational, reliable, and correlate with the user’s intended outcomes (Balvedi et al. Citation2018; Houser Citation2022). Too much real-time data processing may lead to unnecessary noise for user X, while it may be helpful to user Y. Monitoring the user’s presence and activities through multiple sensors may impose added psychological stress, especially in a residential environment. Users who live under constant tracking could alter the originality of their behavior. There is a dearth of empirical evidence to substantiate this concern, and it may be a prospective research area for future studies and product developments.
The fifth gap relates to the lack of sensing technologies that consider user’s data for his/her behavioral needs and comfort at home. There are four categories of lighting systems, and each has its level of control relating to the user’s needs. They include (1) manual system that requires direct user intervention; (2) automatic systems that run by its pre-set parameters and detection; (3) reactive lighting, which responds to external cues; and (4) anticipatory lighting that can sense the user and propose smarter decisions considering the user’s past and expectations (Covarrubias Citation2016). demonstrates that most available sensing measurements are inclined toward motion detection and switching on/off the lighting. Presence detection using the user’s vital signal measurements has been focused on minimizing false alarms and has yet to be personalized for individual needs. Most available sensing technologies represent automatic and reactive lighting systems and have yet to achieve the anticipatory lighting expectations. Even if there is, the system follows predefined patterns in the sensor-based infrastructure model and could function erratically in unfamiliar situations (Navarro et al. Citation2022).
The challenge for future presence sensing technologies in an anticipatory lighting system is to consider approaches to minimize wasted light practice while preserving the user’s behavioral needs and social culture according to the time of the day. For example, the PremiumLight market survey carried out in 12 EU countries highlighted an interesting socially influenced user’s lighting behavior in Sweden. Swedish homes had the most luminaires per home, and only one-third of Swedish residents always turned the lights off, while the majority two-thirds left the lights on in unoccupied rooms (Gerhardsson et al. Citation2019, Citation2021). Gerhardsson et al. (Citation2021) identified several reasons the Swedish residents left the lights on. They include reasons for psychological well-being, safety, and social needs, such as brightening the interior, creating a welcoming and comfortable ambiance upon entry when there is a lack of natural daylight, and reassuring that someone is always at home. Since the residential sector in Sweden consumes much electricity, it is valuable to investigate a reliable anticipatory lighting system with suitable sensors to detect and measure relevant user data within its detection zone; and provide supportive, personalized lighting in an energy efficient way for the majority who prefer leaving the lights on. Here, the connected sensors have to take measurements in real-time and process the data as fast as possible for timely lighting intervention before the user arrives or moves to the next mixed-function space (Covarrubias Citation2016). The sensor has to establish confidence in the quality of the measured data and interpret those data accurately, considering the individual, his/her socially influenced preferences, activity, and time of the day.
4.2. Proposed concept for optimizing future presence sensing lighting: flexible radar-based sensor
Based on the results of the scoping review, bibliographic mapping, limitations of current presence sensing technologies, and identified product knowledge gaps, a wish list is tabulated to optimize future presence sensing lighting for mixed-function small homes. The wish list includes the following criteria:
sensors with short-range presence detection coverage;
presence detection with minimal user data measurements sufficient to support user-behavioral needs while preserving privacy in residential environments; and
simpler real-time users’ data monitoring and processing for a personalized, anticipatory lighting system.
To realize the above wish list, this paper proposes the concept of a flexible radar-based sensor with the following criteria:
Realizing wish list criteria 1: Opting for radar sensors would enable smaller detection zones, switching on/off relevant localized lighting where the user is in a mixed-function space. Radar sensor offers presence detection up to 4 meters and short-range vital signals detection from 0.2 meters to 1.2 meters (Acconeer Citation2019; Thorström and Anderson Citation2020). Pairing the radar module with a Fresnel Zone Plate lens enables a narrow Half Power Beam width of 12° (between 80° to 100°) on the horizontal and vertical plane, respectively. Smaller zonal coverage could assist the daily living of at least two users in a small home with personalized lighting for each person if they are beyond a pre-set distance. For example, suppose one person is cooking in the kitchen and the other is resting in the bedroom in the evening; the flexible radar-based sensor with short-range sensing could configure the lighting to be bright in the kitchen worktop zone while dimmer in the bedroom zone based on each user’s detected vital signal.
Realizing wish list criteria 2: Radar sensing can monitor the user’s breathing and heart rate, which could prompt the anticipated lighting deliverables. Minimizing the real-time tracking to specific user data measurements would lessen the system’s complexity and make it more affordable, saving energy and promoting sustainability. Specific user data measurements could be a strategy to overcome the problems of false alarms caused by non-human objects, dead spots in conventional sensors, and avoid privacy concerns compared to visual sensing (Shukri and Kamarudin Citation2017; Yavari et al. Citation2014b). Focusing real-time sensing on specific parts of the human body (torso) and limiting the type of vital signal measurements could also help reduce user’s indirect psychological stress as fewer personal data (noninvasive to privacy) are permitted to be tracked. Opting for objective vital sensing data measurements could also provide significant empirical evidence for predicting lighting options that better support the user’s mood and behavioral needs according to activity and time of day. Research correlating lighting with mood and behavior from vital signal detection has yet to be explored. For example, it is theoretically hypothesized that activating lighting would be applied during an exercise state (detection of higher breathing rate), and dim/no lighting during sleep (detection of slower breathing rate).
Realizing wish list criteria 3: shows portable light with integrated occupancy sensors co-located at every luminaire. Multiple installations of such battery-powered sensors are flexible, user-friendly add-ons that could detect multi-user in a varied home layout. However, real-time monitoring and processing of multi-user data from multiple sensors at different locations could be complicated (). Interestingly, monitoring real-time multi-user vital signals is achievable using radar techniques, as observed in a study that developed the VitalRadio prototype (Adib et al. Citation2015). The study evidenced that a localized placed VitalRadio device could monitor the vital signals of up to three individuals at the 2-meter, 4-meter, and 6-meter marks in a test lab (8 × 5 meters). The device detected the three users, each at their respective distance from the device, and processed the vital signals of each user. The sensing accuracy of the three individuals was similar to that of a person, and the individuals did not need to face the device or be aware of its presence (Adib et al. Citation2015). Therefore, a conceptual flexible radar sensor prototype design is proposed to detect different zones from a localized placement (), simplifying the real-time detection and processing of multi-user vital signals for users’ personalized, anticipatory lighting. The idea behind the flexible radar sensor prototype is that the lighting follows the user’s movement path instead of providing coverage for the home layout. Detecting vital signals in short-range logical zones along the movement path is sufficient to turn on the lighting in the relevant zones where the user is or moving to in mixed-function small home. More interdisciplinary research is recommended in developing the conceptual flexible radar sensor prototype design, especially in exploring the localized placement (optimal quantity of sensors), directionality, and angle of coverage to monitor multi-user vital signals to support his/her behavioral needs according to the time of the day in a mixed-function small home ().
Fig. 5. Monitoring and processing multi-user data. (a) A complicated real-time presence sensing system with multiple sensors at different locations. (b) The proposed conceptual flexible radar sensor prototype design that detects different zones from a localized placement to simplify the real-time processing of multi-user vital signals. (c) Required factors when designing the proposed conceptual flexible radar sensor prototype for sensing multi-user vital signals to support his/her behavioral needs according to the time of the day.
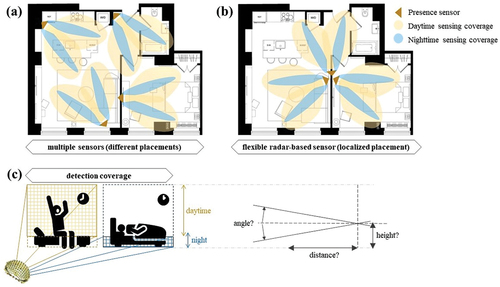
5. Conclusions
The mixed-function spaces in a (small) residential environment with dynamic multi-user occupancy behavior throughout the day can benefit from optimized presence sensing lighting that supports energy efficiency practice and user-behavioral needs. A comprehensive overview of presence sensing approaches is presented, comparing four types of non-wearable sensors (Visual, PIR, Ultrasonic, and Radar) connected to home lighting. Findings from the bibliometric analysis visually reinforced a significant research gap. Studies on presence sensing were not connected to home lighting but were predominantly inclined toward commercial and institutional contexts.
Five significant product knowledge gaps were identified. First, products off-the-shelf are mostly catered for the nonresidential market, and there were almost no or very limited options for residential layouts. Secondly, most products have a broad distance detection and wideangle coverage, lacking appropriate short-range sensing characteristics for a varied, mixed-function small home. Thirdly, there are no alternatives in the placement of sensors other than the recommended ceiling-mounted option. Fourthly, the multiple sensors approach draws several concerns about energy efficiency, cost, maintenance, and complexity in processing the user’s data. The fifth gap observes that sensing technologies are orientated more toward energy efficiency practice and less toward considering the user’s dynamic occupancy and monitoring appropriate data for his/her behavioral needs.
Criteria to optimize future presence sensing lighting in small homes includes prospective insights of a conceptual flexible radar-based sensor (prototype design) that offers short-range presence sensing possibilities and monitors minimal user data to support energy efficiency and his/her behavioral needs in a mixed-function small home. More interdisciplinary research is recommended in exploring the sensor’s localized placement (optimal quantity of sensors), directionality, and angle of coverage to monitor multi-user vital signals according to the time of the day. Findings from future studies are expected to facilitate the development of an anticipatory lighting system with a flexible radar-based sensor that could process real-time multi-user vital signals for smarter localized and personalize lighting options.
Author contributions
RS: Conceptualization, Visualization, Writing (original draft and editing).
TO: Writing (critical review and revision).
MA: Conceptualization, Visualization, Writing (critical review and revision).
All the authors approved the submitted version of this manuscript.
Acknowledgments
The authors thank Mr. Stefan Carlstein from the Jönköping University Library for his technical assistance with VOSviewer.
Disclosure statement
No potential conflict of interest was reported by the author(s).
Additional information
Funding
Notes
* A quote by Mies van der Rohe, a German architect who emphasized simplicity in design by having only the essential items, creating something aesthetically pleasing till it cannot be further reduced to fit the purpose and user’s needs.
† Sensor system fails to detect user(s) when there is one or more present.
‡ Sensor system indicates there is user(s) when there is none present.
§ Any parallel movement alongside the sensor.
** Any perpendicular movement toward and away from the sensor.
References
- Acconeer. 2019. Acconeer Pulsed Coherent Radar (PCR) Module (XM112-XB112). Acconeer. https://docs.acconeer.com/en/latest/sensor_introduction.html#welcome
- Acuity Brands. 2017. Occupancy sensor technologies: microphonics vs. ultrasonic [White Paper]. Acuity control sens switch. https://www.acuitybrands.com/-/media/Files/Acuity/Brands/Controls/SensorSwitch/occupancy.sensor.technologies.whitepaper.pdf
- Adib F, Mao H, Kabelac Z, Katabi D, Miller RC. 2015. Smart homes that monitor breathing and heart rate. In: Proc 33rd Annual ACM Conf Hum Factors Comput Syst (CHI ’15). New York (NY, USA): Association for Computing Machinery (ACM). p. 837–846.
- Ahmad MW, Mourshed M, Mundow D, Sisinni M, Rezgui Y. 2016. Building energy metering and environmental monitoring – a state-of-the-art review and directions for future research. Energy Build. 120:85–102. doi:10.1016/j.enbuild.2016.03.059.
- Aldubyan M, Krarti M. 2022. Impact of stay home living on energy demand of residential buildings: Saudi Arabian case study. Energy. 238:121637. doi:10.1016/j.energy.2021.121637.
- Attia S, Hamdy M, Ezzeldin S. 2017. Twenty-year tracking of lighting savings and power density in the residential sector. Energy Build. 154:113–126. doi:10.1016/j.enbuild.2017.08.041.
- Bachouch RB, Fousseret Y, Parmantier Y. 2023. Optimal sensor placement in smart home using building information modeling: a home support application. Innov Res Biomed Eng. 44(3):100745. doi:10.1016/j.irbm.2022.100745.
- Balvedi BF, Ghisi E, Lamberts R. 2018. A review of occupant behaviour in residential buildings. Energy Build. 174:495–505. doi:10.1016/j.enbuild.2018.06.049.
- Benezeth Y, Laurent H, Emile B, Rosenberger C. 2011. Towards a sensor for detecting human presence and characterizing activity. Energy Build. 43(2–3):305–314. doi:10.1016/j.enbuild.2010.09.014.
- Bergefurt L, Weijs-Perrée M, Appel-Meulenbroek R, Arentze T, de Kort Y. 2022. Satisfaction with activity-support and physical home-workspace characteristics in relation to mental health during the COVID-19 pandemic. J Environ Psychol. 81:101826. doi:10.1016/j.jenvp.2022.101826.
- Bladh M, Krantz H. 2008. Towards a bright future? Household use of electric light: a microlevel study. Energy Policy. 36(9):3521–3530. doi:10.1016/j.enpol.2008.06.001.
- Chu Y, Mitra D, Cetin K, Lajnef N, Altay F, Velipasalar S. 2022. Development and testing of a performance evaluation methodology to assess the reliability of occupancy sensor systems in residential buildings. Energy Build. 268:112148. doi:10.1016/j.enbuild.2022.112148.
- Covarrubias GDS. 2016. Anticipatory lighting control systems. Eindhoven (Netherlands): Eindhoven University of technology (TU/e).
- Crawford RH, Stephan A. 2020. Tiny house, tiny footprint? The potential for tiny houses to reduce residential greenhouse gas emissions. IOP Conf Series. 588, No. 2. Gothenburg (Sweden): IOP Publishing. p. 022073.
- de Souza DF, da Silva PPF, Fontenele LFA, Barbosa GD, de Oliveira Jesus M. 2019. Efficiency, quality, and environmental impacts: a comparative study of residential artificial lighting. Energy Rep. 5:409–424. doi:10.1016/j.egyr.2019.03.009.
- Debes C, Merentitis A, Sukhanov S, Niessen M, Frangiadakis N, Bauer A. 2016. Monitoring activities of daily living in smart homes: understanding human behavior. IEEE Signal Process Mag. 33(2):81–94. doi:10.1109/MSP.2015.2503881.
- Dikel EE, Li YE, Vuotari M, Mancini S. 2019. Evaluating the standby power consumption of smart LED bulbs. Energy Build. 186:71–79. doi:10.1016/j.enbuild.2019.01.019.
- Dikel EE, Newsham GR, Xue H, Valdés JJ. 2018. Potential energy savings from high-resolution sensor controls for LED lighting. Energy Build. 158:43–53. doi:10.1016/j.enbuild.2017.09.048.
- Dong B, Kjærgaard MB, De Simone M, Gunay HB, O’Brien W, Mora D, Dziedzic J, Zhao J. 2018. Sensing and data acquisition. In: Wagner A, O’Brien W, Dong B, editors. Explor Occupant Behav Build Methods Challenges. Cham (Switzerland): Springer International Publishing. p. 77–106.
- Energimyndigheten. 2011. The Swedish Energy Agency’s guide to the new light. Stockholm. http://www.energimyndigheten.se/globalassets/webb-en/belysning_engelska.pdf
- Enongene KE, Murray P, Holland J, Abanda FH. 2017. Energy savings and economic benefits of transition towards efficient lighting in residential buildings in Cameroon. Renew Sustain Energy Rev. 78(April):731–742. doi:10.1016/j.rser.2017.04.068.
- Extronic Elektronik AB. För belysningskonsulter och installatörer. Extronic Elektron AB. www.extronic.se
- Fioranelli F, Le Kernec J, Shah SA. 2019. Radar for health care: recognizing human activities and monitoring vital signs. IEEE Potentials. 38(4):16–23. doi:10.1109/MPOT.2019.2906977.
- Garcia M, Lau EK, Lovald ST, Chang E. 2019. Ultrasound in consumer products: perception and safety assessment. In: Thought Leadersh. San Francisco (USA): Exponent. p. 1–2.
- Gerhardsson KM, Laike T, Johansson M. 2019. Residents’ lamp purchasing behaviour, indoor lighting characteristics and choices in Swedish homes. Indoor Built Environ. 28(7):964–983. doi:10.1177/1420326X18808338.
- Gerhardsson KM, Laike T, Johansson M. 2021. Leaving lights on – a conscious choice or wasted light? Use of indoor lighting in Swedish homes. Indoor Built Environ. 30(6):745–762. doi:10.1177/1420326X20908644.
- Guo H, Zhang N, Shi W, AlQarni S, Wu S, Wang H 2019. Real-time indoor 3D human imaging based on MIMO radar sensing. In: 2019 IEEE Int Conf Multimed Expo. Shanghai (China): IEEE. p. 1408–1413.
- Hayat H, Griffiths T, Brennan D, Lewis RP, Barclay M, Weirman C, Philip B, Searle JR. 2019. The state-of-the-art of sensors and environmental monitoring technologies in buildings. Sensors. 19(17):3648. doi:10.3390/s19173648.
- Houser KW. 2022. To measure is to know … or not. LEUKOS. 18(2):103. doi:10.1080/15502724.2022.2029086.
- Hussain Z, Sheng QZ, Zhang WE. 2020. A review and categorization of techniques on device-free human activity recognition. J Netw Comput Appl. 167:102738. doi:10.1016/j.jnca.2020.102738.
- Ihara I. 2008. Ultrasonic sensing: fundamentals and its applications to nondestructive evaluation. In: Mukhopadhyay S, Huang R, editors. Sensors. Vol. 21. Berlin (Heidelberg): Springer. p. 287–305.
- Javaid M, Haleem A, Rab S, Pratap Singh R, Suman R. 2021. Sensors for daily life: a review. Sensors Int. 2:100121. doi:10.1016/j.sintl.2021.100121.
- Kalyanaraman A, Soltanaghaei E, Whitehouse K 2019. Doorpler: a radar-based system for real-time, low power zone occupancy sensing. In: 2019 IEEE Real-Time Embed Technol Appl Symp. Montreal (QC, Canada): IEEE. p. 42–53.
- Kawka E, Cetin K. 2021. Impacts of COVID-19 on residential building energy use and performance. Build Environ. 205:108200. doi:10.1016/j.buildenv.2021.108200.
- Lucas J. 2019. What are microwaves? Live Sci. https://www.livescience.com/50259-microwaves.html
- McLean G, Osei-Frimpong K. 2019. Hey Alexa … examine the variables influencing the use of artificial intelligent in-home voice assistants. Comput Human Behav. 99:28–37. doi:10.1016/j.chb.2019.05.009.
- Moadab NH, Olsson T, Fischl G, Aries MBC. 2021. Smart versus conventional lighting in apartments - electric lighting energy consumption simulation for three different households. Energy Build. 244:111009. doi:10.1016/j.enbuild.2021.111009.
- Munn Z, Peters MDJ, Stern C, Tufanaru C, McArthur A, Aromataris E. 2018. Systematic review or scoping review? Guidance for authors when choosing between a systematic or scoping review approach. In: Payne H, Koch S, Tantia, J, Fuchs, T, editors. BMC Med Res Methodol. Vol. 18. p. 143.
- Navarro RC, Ruiz AR, Molina FJV, Romero MJS, Chaparro JD, Alises DV, Lopez JCL. 2022. Indoor occupancy estimation for smart utilities: a novel approach based on depth sensors. Build Environ. 222:109406.
- Nguyen TA, Aiello M. 2013. Energy intelligent buildings based on user activity: a survey. Energy Build. 56:244–257. doi:10.1016/j.enbuild.2012.09.005.
- Onaygil S, Erkin E 2018. Smart lighting solutions for residences using IoT infrastructure: advantages, disadvantages and effects on energy saving. In: 2018 Seventh Balk Conf Light. Varna (Bulgaria): IEEE. p. 1–5.
- Palacios-Garcia EJ, Chen A, Santiago I, Bellido-Outeiriño FJ, Flores-Arias JM, Moreno-Munoz A. 2015. Stochastic model for lighting’s electricity consumption in the residential sector: impact of energy saving actions. Energy Build. 89:245–259. doi:10.1016/j.enbuild.2014.12.028.
- Pandharipande A, Caicedo D. 2015. Smart indoor lighting systems with luminaire-based sensing: a review of lighting control approaches. Energy Build. 104:369–377. doi:10.1016/j.enbuild.2015.07.035.
- Pandharipande A, Newsham GR. 2018. Lighting controls: evolution and revolution. Light Res Technol. 50(1):115–128. doi:10.1177/1477153517731909.
- Pompei L, Blaso L, Fumagalli S, Bisegna F. 2022. The impact of key parameters on the energy requirements for artificial lighting in Italian buildings based on standard EN 15193-1:2017. Energy Build. 263:112025. doi:10.1016/j.enbuild.2022.112025.
- Preferred Reporting Items for Systematic reviews and Meta-Analyses extension for Scoping Reviews (PRISMA-ScR) Checklist. 2021. PRISMA. http://prisma-statement.org/Extensions/ScopingReviews
- Reinoso O, Payá L. 2020. Special issue on visual sensors. Sensors. 20(3):910. doi:10.3390/s20030910.
- Safranek S, Wilkerson A, Irvin L, Casey C. 2021. Using occupant interaction with advanced lighting systems to understand opportunities for energy optimization: control data from a hospital NICU. Energy Build. 251:111357. doi:10.1016/j.enbuild.2021.111357.
- Santra A, Ulaganathan RV, Finke T. 2018. Short-range millimetric-wave radar system for occupancy sensing application. IEEE Sensors Lett. 2(3):1–4. doi:10.1109/LSENS.2018.2852263.
- Sciuto A, Saini A, Forlizzi J, Hong JI. 2018. “Hey Alexa, what’s up?”: a mixed-methods studies of in-home conversational agent usage. In: Proceedings of the 2018 Designing Interactive Systems Conference. New York (NY, USA): Association for Computing Machinery (ACM). p. 857–868.
- Shukri S, Kamarudin LM. 2017. Device free localization technology for human detection and counting with RF sensor networks: a review. J Netw Comput Appl. 97:157–174. doi:10.1016/j.jnca.2017.08.014.
- Soheilian M, Moadab NH, Fischl G, Aries MBC. 2019. Comparison of simulated energy consumption by smart and conventional lighting systems in a residential setting. J Phys Conf Ser. 1343(1):012155. doi:10.1088/1742-6596/1343/1/012155.
- Statistics Sweden. 2021a. Electricity in Sweden. Stat Sweden. https://www.statista.com/statistics/1027084/consumption-of-electricity-in-sweden-by-user/
- Statistics Sweden. 2021b. Households’ housing 2020: smallest living space per person in cities. Stat Sweden. https://www.scb.se/en/finding-statistics/statistics-by-subject-area/household-finances/income-and-income-distribution/households-housing/pong/statistical-news/households-housing-2020/
- Thorström M, Anderson G. 2020. Presence detectors and remote heartbeat sensing using radar technology. Lund (Sweden): Lund University.
- Ticleanu C. 2021. Impacts of home lighting on human health. Light Res Technol. 53(5):453–475. doi:10.1177/14771535211021064.
- Tiller D, Guo X, Henze G, Waters C. 2010. Validating the application of occupancy sensor networks for lighting control. Light Res Technol. 42(4):399–414. doi:10.1177/1477153510375524.
- Tleuken A, Turkyilmaz A, Sovetbek M, Durdyev S, Guney M, Tokazhanov G, Wiechetek L, Pastuszak Z, Draghici A, Boatca ME, et al. 2022. Effects of the residential built environment on remote work productivity and satisfaction during COVID-19 lockdowns: an analysis of workers’ perceptions. Build Environ. 219:109234. doi:10.1016/j.buildenv.2022.109234.
- Tricco AC, Lillie E, Zarin W, O’Brien KK, Colquhoun H, Levac D, Moher D, Peters MDJ, Horsley T, Weeks L, et al. 2018. PRISMA Extension for Scoping Reviews (PRISMA-ScR): checklist and explanation. Ann Intern Med. 169(7):467–473. doi:10.7326/M18-0850.
- van Eck NJ, Waltman L. 2010. Software survey: VOSviewer, a computer program for bibliometric mapping. Scientometrics. 84(2):523–538. doi:10.1007/s11192-009-0146-3.
- Williams A, Atkinson B, Garbesi K, Page E, Rubinstein F. 2012. Lighting controls in commercial buildings. LEUKOS. 8(3):161–180. doi:10.1582/LEUKOS.2012.08.03.001.
- Wilson L. 2022. How big is a house? Average house size by country - 2022. Shrink That Footpr. https://shrinkthatfootprint.com/how-big-is-a-house/
- Woodstock T-KAE. 2020. Multisensor fusion for occupancy detection and activity recognition in a smart room. Troy (New York): Rensselaer Polytechnic Institute.
- Wotton J, Skates H, Shutter L. 2018. Tiny house – when size matters. Aust Plan. 55(3–4):209–220. doi:10.1080/07293682.2019.1634112.
- Wu L, Wang Y. 2021. Stationary and moving occupancy detection using the SLEEPIR sensor module and machine learning. IEEE Sens J. 21(13):14701–14708. doi:10.1109/JSEN.2021.3071402.
- Yavari E, Lee A, Pang K, Mccabe NA, Boric-Lubecke O 2014a. Radar and conventional occupancy sensors performance comparison. In: 2014 Asia-Pacific Microw Conf.; Sendai, Japan. p. 444–446.
- Yavari E, Song C, Lubecke V, Boric-Lubecke O. 2014b. Is there anybody in there? IEEE Microw Mag. 15(2):57–64. doi:10.1109/MMM.2013.2296210.
- Zhang Y, Bai X, Mills FP, Pezzey JCV. 2018. Rethinking the role of occupant behavior in building energy performance: a review. Energy Build. 172:279–294. doi:10.1016/j.enbuild.2018.05.017.