ABSTRACT
Autophagy summarizes evolutionarily conserved, intracellular degradation processes targeting cytoplasmic material for lysosomal degradation. These encompass constitutive processes as well as stress responses, which are often found dysregulated in diseases. Autophagy pathways help in the clearance of damaged organelles, protein aggregates and macromolecules, mediating their recycling and maintaining cellular homeostasis. Protein-protein interaction networks contribute to autophagosome biogenesis, substrate loading, vesicular trafficking and fusion, protein translocations across membranes and degradation in lysosomes. Hypothesis-free proteomic approaches tremendously helped in the functional characterization of protein-protein interactions to uncover molecular mechanisms regulating autophagy. In this review, we elaborate on the importance of understanding protein-protein-interactions of varying affinities and on the strengths of mass spectrometry-based proteomic approaches to study these, generating new mechanistic insights into autophagy regulation. We discuss in detail affinity purification approaches and recent developments in proximity labeling coupled to mass spectrometry, which uncovered molecular principles of autophagy mechanisms.
Abbreviations: AMPK: AMP-activated protein kinase; AP-MS: affinity purification-mass spectrometry; APEX2: ascorbate peroxidase-2; ATG: autophagy related; BioID: proximity-dependent biotin identification; ER: endoplasmic reticulum; GFP: green fluorescent protein; iTRAQ: isobaric tag for relative and absolute quantification; MS: mass spectrometry; PCA: protein-fragment complementation assay; PL-MS: proximity labeling-mass spectrometry; PtdIns3P: phosphatidylinositol-3-phosphate; PTM: posttranslational modification; PUP-IT: pupylation-based interaction tagging; RFP: red fluorescent protein; SILAC: stable isotope labeling by amino acids in cell culture; TAP: tandem affinity purification; TMT: tandem mass tag.
Introduction
The majority of proteins are degraded via two pathways: the ubiquitin proteasome system and autophagy. In comparison to the specific degradation of the ubiquitin proteasome system, autophagy is thought to degrade substrates nonselectively and selectively due to cargo receptors [Citation1]. Thus, autophagy summarizes constitutive lysosomal degradation pathways and stimulus-dependent stress responses to preserve cellular homeostasis. Macromolecules, protein aggregates and organelles are degraded and recycled to accomplish the energy demands of the cell and to restock basic building blocks for anabolic processes. Autophagy acts in general in a cytoprotective manner and its dysregulation has been linked to various diseases such as neurodegeneration, cancer and metabolic syndrome [Citation2]. Autophagy has been broadly categorized into three different subtypes: macroautophagy, microautophagy and chaperone-mediated autophagy, which differ in the mode of transport and delivery of substrates for lysosomal degradation. Microautophagy describes an invagination of endosomal or lysosomal membranes that engulf cytoplasmic substrates for degradation [Citation3]. Chaperone-mediated autophagy is probably the most selective subtype of autophagy. Recognition and unfolding of substrates carrying KFERQ-like motifs by cytosolic chaperone HSPA8/HSC70 (heat shock protein family A [Hsp70] member 8) determines this selectivity. Unfolded proteins are translocated across lysosomal membranes for degradation by LAMP2A, a lysosomal membrane protein [Citation4,Citation5]. In a process termed endosomal microautophagy, substrates carrying the KFERQ motif are selectively recognized by cytosolic HSPA8 chaperones and targeted for degradation to late endosomes instead of the lysosomes via binding to phosphatidylserine on endosomal membranes [Citation6]. Macroautophagy, hereafter referred to as autophagy, is an intracellular degradation pathway that starts with the formation of a double-membraned vesicle called autophagosome, which enwraps cytoplasm targeted for lysosomal degradation. Autophagosome biogenesis consists of distinct hierarchical phases starting with the formation of a cup-shaped membrane called phagophore, followed by elongation of the phagophore, maturation, closure, fusion with endosomes, and finally with lysosomes leading to the formation of autolysosomes [Citation7,Citation8]. Autophagy has been shown to play a critical role in selectively clearing damaged organelles (mitochondria, peroxisomes etc.), infectious agents and protein aggregates [Citation2].
Over the last few decades, more than 40 autophagy-related (ATG) genes/proteins have been reported in yeast. Most of these are conserved between yeast and mammals and have crucial roles in the progress of autophagy [Citation9]. The canonical core pathway is regulated by six conserved protein complexes [Citation10]: (i) the ULK1 (unc-51 like autophagy activating kinase 1)-ULK2 complex, which is critical for autophagy initiation; (ii) the ATG9 system, which provides membranes for autophagosome generation; (iii) the class III phosphatidylinositol 3-kinase complex (PtdIns3K), which phosphorylates the lipid phosphatidylinositol (PtdIns) generating PtdIns-3-phosphate (PtdIns3P) that serves as binding site for protein recruitment; (iv) the ATG2-WIPI complex, which is important for membrane expansion, (v) the ATG12 and (vi) Atg8-family proteins ubiquitin-like conjugation systems. The latter being important for phagophore expansion and cargo recruitment [Citation11]. We will discuss the roles of these complexes in more detail in the following paragraphs ().
Figure 1. Schematic representation of autophagosome biogenesis and maturation. MTORC1 inhibits autophagy via its inhibitory phosphorylations on ULK1 and ATG13 under nutrient-rich conditions. Under stress, autophagy is activated via the formation of an active tetrameric ULK1 initiation complex to promote autophagosome nucleation. PtdIns3K complex I catalyzes the production of PtdIns3Ps, which contributes to phagophore nucleation and omegasome formation. PtdIns3P-binding proteins like ZFYVE1/DFCP1 and WIPIs decorate the omegasomes. WIPI2 interaction with ATG16L1 mediates the recruitment of the ATG12–ATG5-ATG16L1 complex for the conjugation of LC3-I to PE and phagophore expansion and maturation. Additionally, lipid sources from ATG9 vesicles, ATG2A/B recruited by WIPIs and from cell membranes collectively help in expanding the phagophore membrane. Double-membraned autophagosomes fuse with lysosomes to form autolysosomes and their content is degraded by lysosomal hydrolases
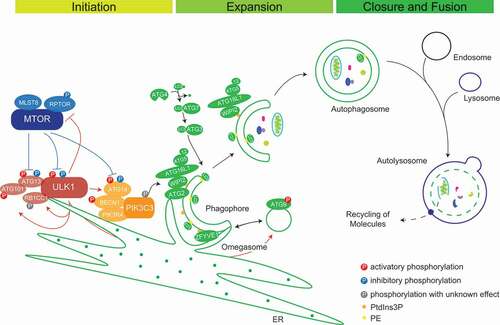
Protein complexes regulating autophagy
Initiation of autophagy is executed by two kinase complexes, the ULK1 complex, the mammalian homolog of yeast Atg1 complex, and secondly, the PtdIns3K complex [Citation12]. ULK1 (or its homolog ULK2), a Ser/Thr kinase, phosphorylates itself, and its complex members ATG13, ATG101 and RB1CC1/FIP200. Together, they form the active tetrameric autophagy initiation complex, e.g., in response to starvation [Citation13,Citation14]. Endoplasmic reticulum (ER) recruitment of this complex happens through direct interaction of ULK1 and RB1CC1 via their FFAT motifs with ER membrane proteins VAPA (VAMP associated protein A)-VAPB, forming active phagophore initiation sites on the ER membrane. Additionally, VAPs interact with WD repeat-containing protein WIPI2; a membrane-associated protein forming a tethering complex and strengthening ER-phagophore contact, making ER an essential organelle for autophagosome biogenesis [Citation15]. ATG9, a multi-membrane spanning protein, predominantly localizes at the trans-Golgi network and endosomes. It is postulated to organize a lipid source for phagophore generation and expansion due to its colocalization to the phagophore membrane [Citation16]. ATG9-containing vesicles fuse with ATG16L1-containing vesicles to progressively promote autophagosome biogenesis [Citation17] ().
There are two distinct PtdIns3K complexes. Complex I promotes autophagy and consists of the lipid kinase PIK3C3/VPS34, the adaptor protein PIK3R4/VPS15, ATG14, which helps in ER membrane tethering, the stabilizing subunit BECN1/Beclin-1, and the accessory subunit NRBF2. In complex II, UVRAG replaces ATG14 and NRBF2. Complex II takes over functions in later stages of autophagy [Citation12]. ULK1 helps recruiting the PtdIns3K complex I to active initiation sites by phosphorylating the following proteins: (a) AMBRA1 on amino acid residues Ser465 and Ser635 to release the tethered PIK3C3-BECN1 complex toward the ER from AMBRA1, which is bound to microtubules via DYNLL1 and DYNLL2 (dynein light chain LC8-type 1/2) [Citation18], (b) PIK3C3 on Ser249 [Citation18,Citation19], (c) BECN1 on Ser15 (human)/Ser14 (murine) stabilizing the complex [Citation20], and (d) ATG14 on Ser29 activating autophagy by increasing PIK3C3 activity [Citation21]. The PtdIns3K complex increases production of PtdIns3P at phagophore formation sites, where PtdIns3P binding protein ZFYVE1/DFCP1 accumulates, leading to crescent-shaped growing phagophores called omegasomes [Citation22]. These structures provide strong platforms for the binding of WIPI1 and WIPI2 involved in nascent autophagosome biogenesis [Citation23]. WDR45B/WIPI3 and WDR45/WIPI4 positively affect signaling events up- and downstream of PtdIns3P production, controlling the size of autophagosomes [Citation24]. In addition, human homologs of yeast lipid transfer protein Atg2 (ATG2A and ATG2B) decorate phagophores via their interaction with WIPIs [Citation24]. ATG2s are involved in maintaining a membrane tether or contact site between ER and phagophores leading to expansion of the growing phagophores by direct lipid transfer [Citation25]. Later, they help in autophagosome membrane closure [Citation26] ().
Ubiquitin-like modifiers (UBLs) play important roles in autophagosomal cargo recruitment and maturation. ATG12 and the family of Atg8 homologs are characterized by ubiquitin folds as UBLs. As ubiquitin, respective proteins are activated and transferred to target proteins by sets of sequential reactions [Citation27]. ATG7, a ubiquitin E1-like enzyme, activates the C-terminal glycine of ATG12 and hands it over to ATG10, an E2-like enzyme. ATG10 transfers ATG12 to its target ATG5, which in turn binds to ATG16L1 to form the ATG12–ATG5-ATG16L1 trimeric complex [Citation27–29]. Atg8 homologs are UBLs classified into two subfamilies: the LC3 family consisting of MAP1LC3A, LC3B, LC3B2 and LC3C and the GABARAP family consisting of GABARAP, GABARAPL1, and GABARAPL2 (hereafter collectively referred to as LC3s) [Citation30]. LC3s are anchored in membranes by conjugation to phosphatidylethanolamine (PE). First, cleavage at the C terminus by ATG4B proteases exposes a terminal glycine residue and activates LC3 (LC3-I) to get bound by ATG7 [Citation31]. The E2-like enzyme ATG3 and the ATG12–ATG5-ATG16L1 complex, which has E3 ligase activity, transfer LC3-I (cytosolic) to PE (LC3-II). This happens at active sites of autophagosome biogenesis due to recruitment of the ATG12–ATG5-ATG16L1 complex via the interaction between ATG16L1 and WIPI2 [Citation29]. LC3s are predominantly involved in the selective capture of substrates for degradation. Autophagy receptors bind to LC3s via so-called LC3-interacting regions (LIRs) [Citation32]. However, LC3s also help in the maturation and closure of nascent autophagosomes [Citation33]. In general, autophagosomes fuse with endosomes to form amphisomes before they finally fuse with lysosomes to form autolysosomes for degradation of their contents [Citation34]. Fusion of mature autophagosomes with lysosomes is carried out in a concerted manner by the action of RAB and SNARE proteins and membrane tethering complexes, most importantly by RAB7A, STX17, SNAP29, VAMP8 and the HOPS complex [Citation35].
Delicate balance between anabolism and catabolism is essential for the survival of cells. Anabolism is positively regulated by the master regulator of cell growth MTORC1, a conserved Ser/Thr kinase complex consisting of the kinase MTOR (mechanistic target of rapamycin kinase), and the regulatory subunits MLST8 and RPTOR. MTORC2, composed of MTOR, RICTOR and MLST8, is thought to positively modulate cell proliferation [Citation36]. MTORC1 is well known to inhibit catabolic pathways, including autophagy where inhibitory phosphorylations of Ser637 and Ser757 on ULK1 and Ser258 on ATG13 prevent them from forming the active autophagy initiation complex. Nutrient deprivation or rapamycin treatment inhibit MTORC1 activity, thus enabling the activation of autophagy via dephosphorylation of MTORC1 sites and subsequent activating phosphorylations of, for example, ATG13 by ULK1. Under starvation conditions, dephosphorylation of MTORC1 target sites on ULK1 is carried out by the heterotrimeric PP2A protein phosphatase, consisting of the catalytic subunit PPP2CA, the scaffolding subunit PPP2R1B/PRL65, and the regulatory subunit PPP2R2A/B55alpha [Citation37]. Additionally, the energy demand activates AMP-activated protein kinase (AMPK), an AMP:ATP ratio sensor. AMPK activates the ULK1 complex by phosphorylating Ser317, Ser659, and Ser777 on ULK1 itself and Ser224 on ATG13. Active ULK1 catalyzes autophosphorylations on different sites, among others Thr180, Ser1042, and Thr1046 [Citation14].
The dynamic organization of sequential events in autophagy is highly regulated by protein-protein interactions (PPIs) and post-translational modifications (PTMs) happening at the right spatial and temporal resolution. To study PPIs in vitro and in vivo, different techniques have been used [Citation38]. The strength of the interactions, either strong/permanent or weak/transient, determine the efficient usage of a specific method. In order to obtain a global, unbiased picture of PPIs, mass spectrometry (MS)-based proteomic approaches studying PPIs and neighborhoods have gained much attention lately [Citation39]. New developments allow the study of strong and weak interactions, supporting the construction of hierarchical networks. Co-immunoprecipitation methods like tandem affinity purification (TAP) enrich strong interactions. To capture weak interactions, crosslinking coupled to affinity purification (AP), proximity labeling (PL) and bio-orthogonal chemistries coupled to MS are now widely used. In the following chapters, we summarize the different MS-based methods to identify PPIs of different strengths and highlight their usage to study the regulation of autophagy.
Protein-protein interactions and their regulation
Dynamic PPIs regulate virtually all biological processes, e.g., metabolism, DNA replication, protein synthesis, as well as autophagy. Interactions can range from simple binary interactions to complex multimeric interactomes and are essential to maintain efficient functioning of cells thereby balancing cell physiology. Hence, understanding these interactions is crucial for revealing molecular functions and disease-associated mechanisms in order to design potent therapeutics [Citation40]. Based on stability, PPIs can be classified into obligate, when binding partners are not stable by themselves, and non-obligate interactions of otherwise stable protomers. Based on binding affinity, i.e. the temporal profile of interactions, PPIs can be classified into permanent and transient interactions [Citation41]. However, often PPIs do not fall into a static classification and a continuum exists between quasi-permanent and transient interactions. As a given protein can also interact with different proteins and form several distinct complexes in vivo, the discrimination between obligate and non-obligate interactions may not be straight forward and depend on a given (patho)physiological condition [Citation42,Citation43]. In addition, permanent interactions often involve proteins that are unstable as monomers and that function in complexes. Thus, due to experimental limitations and in order to simplify a classification based on in vivo observations, only obligate PPIs might be regarded as quasi-permanent (). In contrast, structurally stable proteins that interact with different proteins by undergoing association and dissociation reactions form transient/non-obligate PPIs, i.e. time-limited interactions. Based on affinity and temporal quality, transient interactions can be further classified as strong and weak transient interactions. Moreover, protein complexes are classified based on composition as homo-oligomeric (having identical protein members) and hetero-oligomeric complexes (having non-identical protein members).
Figure 2. Classification of protein-protein interactions based on stability and binding affinity. Affinity is inversely proportional to the dissociation constant Kd. Based on stability and binding affinity, PPIs can be classified into quasi-permanent/obligate and transient/non-obligate interactions. In contrast to permanent complexes, transient complexes are dynamic with proteins associating and dissociating. Transient complexes can be subclassified as strong and weak based on affinity and temporal profile of interactions. Moreover, PPIs can be classified based on composition as homo-oligomers with identical proteins interacting and hetero-oligomers with non-identical chains interacting. Often, interactions change due to physiological conditions rather reflecting a continuous than a “static” classification
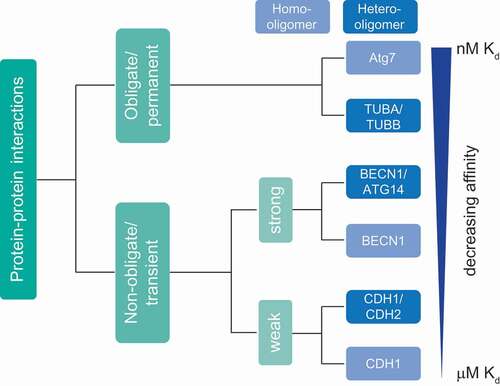
Homo-oligomers generally form highly stable, permanent structures and are symmetric in nature. They also form scaffolds for stable interactions with other macromolecules. For example, yeast Atg7 is an E1-like enzyme that forms a functionally-active homo-dimeric complex (Kd = 1 nM). As described above, ATG7 plays a major role in the two ubiquitin-like conjugation pathways involved in autophagy: ATG12–ATG5-ATG16L1 and LC3–PE generation [Citation44]. TUBA (tubulin alpha) and TUBB (tubulin beta) form a quasi-permanent heterodimer (Kd ≈ 84 nM [54–123 nM]), which polymerizes to form dynamic microtubules. These help in autophagosome formation, motility and fusion with lysosomes [Citation43,Citation45,Citation46]. Another example of a quasi-permanent, homo-oligomeric complex is CASTOR1 (cytosolic arginine sensor of MTORC1), a protein that forms a stable homodimer and acts as an amino acid sensor by binding to arginine [Citation47].
In contrast, transient complexes associate and dissociate based on physiological conditions. Proteins can function either independently or by forming a complex [Citation42,Citation48,Citation49]. Transient complexes have a wide range of affinities and temporal stabilities, depending on the requirements in cells. Hetero-oligomers often form transient complexes of varying stability. Based on their characteristics, transient complexes are classified into strong and weak transient interactions. A strong transient interaction would have a higher affinity and longer lifetime compared to a weak transient interaction. For instance, ATG12 is activated by ATG3 forming a strong, transient hetero-oligomeric complex (Kd = 50 nM) [Citation50]. BECN1, a protein that forms a strong transient homodimer, remains inactive in nutrient-rich conditions. However, upon a physiological stress stimulus leading to activation of autophagy, a BECN1 monomer can interact strongly with ATG14 (Kd = 3.2 µM) to form an active hetero-oligomeric PtdIns3K class-III complex [Citation51]. Weak transient interactions are continuously broken and formed and difficult to capture by biochemical/analytical approaches. For example, cadherins are adhesion proteins, which mediate cell-cell communication. Expression of different cadherins in distinct cell types helps in spatial positioning of respective cells during development and maintaining their 3-D architecture, crucial for tissue function. In order to maintain specific cell-cell communication, CDH1/E-cadherin forms weak homodimers (Kd = 160 µM). CDH1 forms also hetero-oligomers with CDH2/N-cadherin, which interact stronger, mediating contacts of different cell types. Autophagy ensures optimal cell growth via regulating the abundance of CDH1, a protein involved in CTNNB1 (catenin beta 1)-WNT signaling [Citation52–54] (). SNXs (sorting nexins), a class of peripheral membrane proteins important for endosomal sorting, can oligomerize via their Bin/Amphiphysin/Rvs (BAR) domains to perform vesicle to tubular membrane remodeling. SNX4 forms weak homodimers to perform tubule formation of membranes. Under certain physiological conditions such as autophagy, SNX4 can form a hetero-oligomeric complex with SNX7 to mediate autophagosome assembly and maturation [Citation55–57]. Overall, the above examples highlight the variety of affinities of protein-protein interactions important for autophagy.
Structural features of proteins, such as motifs and domains, modulate an array of interactions. In addition, differential interactions can be modulated by factors such as pH, compartmentalization, local concentration of ions, and covalent modifications like phosphorylation and ubiquitination. The above features collectively impart specificity to PPIs and mediate oligomerization of proteins meant to interact in a crowded environment [Citation42,Citation58].
Mass spectrometry-based proteomic approaches to study protein-protein interactions
Due to the importance of PPIs, numerous cell biological and biochemical methods exist to study them in vitro and in vivo. This review cannot comprehensively summarize all approaches in detail (e.g., see [Citation59] for more technical details), but we would like to list important, well-established examples of in vitro and in vivo methods, focusing on MS-based approaches. In vitro methods are techniques carried out in a controlled environment after cell lysis and allow the analysis of binary as well as oligomeric interactions. Examples are affinity purifications, size-exclusion chromatography, protein arrays, enzyme-linked immunosorbent assay, and biophysical methods such as isothermal calorimetry, microscale thermophoresis, and surface plasmon resonance. Powerful MS-based in vitro methods are: AP-MS, chemical cross-linking MS (XL-MS), co-fractionation coupled to MS (CoFrac-MS) and PL-MS methods. In vivo methods are techniques carried out in a living organism and commonly address binary interactions [Citation60]. Examples are yeast two-hybrid analyses or image-based analyses, such as bimolecular fluorescence complementation, Förster resonance energy transfer, bioluminescence resonance energy transfer, and proximity ligation assays [Citation61].
MS-based proteomic studies are widely used to understand mechanisms in biological pathways by analyzing PPIs. Technological advancements in terms of MS-instrumentation, liquid chromatography (LC), sample preparation methods, techniques used to enrich interactomes, high throughput analyses, and bioinformatics data interpretation have greatly increased the usage of MS-based approaches. The ultimate goal of proteomic studies is to comprehensively characterize the proteome, protein expression levels, PTMs, and build functionally relevant protein networks. Based on the nature of protein identifications and characterizations, two approaches are discriminated: top-down and bottom-up proteomics [Citation62].
In top-down proteomics, intact proteins are ionized, followed by fragmentation and measurement in a high-resolution mass spectrometer. This approach provides a complete characterization of proteoforms including PTMs. The bottom-up proteomic approach involves chemical or enzymatic digestion of proteins into peptides prior to ionization and MS measurement. This method is easily automatable and generally helps in the identification of large numbers of proteins compared to top-down approaches, which require a more sophisticated front-end biochemistry to generate samples for MS analyses [Citation63].
Different strategies in MS sample acquisition exist: data-dependent acquisition (DDA), data-independent acquisition (DIA), both unbiased discovery methods, and directed/targeted proteomics approaches. These strategies differ in the depth or coverage of protein identifications. DDA is based on a selection and identification of the most abundant ions in the sample. In DIA, a relatively new method, ions are not selected. Entire groups of ions are measured, generating a “digital map” of the entire sample [Citation39,Citation64–70]. Whereas DIA approaches lead to less missing values across experiments, data interpretation is more challenging. Targeted proteomics approaches deal with the specific isolation and measurement of predefined ions, making it more reproducible compared to discovery proteomics experiments.
The aforementioned strategies have advantages and dis-advantages, leading to trade-offs in sensitivity, specificity, reproducibility, accuracy, and dynamic range (for more information on these strategies, see [Citation39,Citation64–70]). In general, different tools or methods are available for every step in the experiment starting from sample isolation and preparation, MS data acquisition, data analysis, and statistical interpretation. Given the applicability of MS in a wide range of biological questions, choosing the right combination of tools is essential for answering the complex biochemical questions underlying autophagy regulation.
Quantitative proteomics
As signal intensities recorded by MS depend on ionization properties of respective biomolecules, MS is not a truly quantitative analytical approach. Therefore, quantitative proteomics strategies have been developed that allow a systematic quantification of samples revealing changes between measured proteomes. Quantitative information is key to characterize molecular pathways both at protein and PTM level. Quantification can be performed in two ways, either absolute or relative. Absolute quantification is possible by comparing signal intensities of biomolecules with signals of known amounts of respective synthetic/purified standard substances. Relative quantification is performed by comparing ion intensities between different samples. Quantification of proteins or peptides can be performed label-free or via stable isotope labeling strategies, the latter being more accurate as samples can be analyzed in single MS experiments. Labeling approaches add tags that differ in mass but do not interfere with ionization properties. Tags can be incorporated at protein or peptide level, enzymatically, chemically, or metabolically [Citation71].
Metabolic labeling summarizes strategies that commonly lead to the in vivo incorporation of 13C and 15N isotope-labeled metabolites such as amino acids. In one of the most common approaches, stable isotope labeling by amino acids in cell culture (SILAC), labeled lysine (K) and arginine (R) variants are used. Commonly three different SILAC labels are used to perform relative quantifications between three biological conditions [Citation72,Citation73]. SILAC is majorly used in mammalian cell culture studies. Metabolic labeling is advantageous as it allows mixing of cells prior to biochemical perturbations, thus improving quantification accuracy. Enzymatic labeling is being carried out during MS sample preparation, most commonly used in bottom-up proteomics experiments. For example, the use of 18O-labeled water allows the incorporation of 18O to neo-C-termini generated by proteolytic digestion [Citation74,Citation75]. Chemical methods utilize the availability of reactive N-termini and amino acid side chains to incorporate chemical groups. Isobaric mass tags are linked to reactive N-terminal and epsilon amino groups of lysine residues via N-hydroxysuccinimide (NHS) chemistry. These tags are made up of a peptide reactive group, a stable isotope-labeled reporter ion, and a balancer group. Prominent examples are isobaric tags for relative and absolute quantification (iTRAQ) and tandem mass tag (TMT) labeling. These tags have similar chemical structures, differing in positions, numbers, and combinations of 13C and 15N isotopes, and can be highly multiplexed [Citation76,Citation77]. Currently, up to 16 samples can be quantified in a single experiment on a routine basis [Citation78,Citation79]. Due to new bioinformatics approaches, label-free quantification based on peptide precursor (DDA) or fragment ion (DIA) intensities is a widely used and cheap method for robust relative quantification [Citation68,Citation80]. The aforementioned methods have advantages and disadvantages pertaining to the nature of the quantification method, sample type, multiplexing capacity, cost-effectiveness, quantification accuracy, sensitivity, and proteome coverage [Citation68,Citation81,Citation82].
Affinity purification-mass spectrometry to study protein-protein interactions
AP-MS is one of the widely used methods to identify and characterize PPIs. The principle of this method is to isolate a protein of interest (referred to as bait protein) and to identify/quantify its interacting proteins by MS. For AP, either an antibody recognizing the endogenous protein or the expression of a tagged-protein variant in combination with a tag-recognizing structure are commonly used. Affinity tags or antibodies are captured by other macromolecules covalently attached to a solid matrix such as agarose beads. Macromolecules can be oligonucleotides (DNA/RNA), proteins, such as proteins A and G binding the Fc parts of antibodies, peptides and lipids [Citation83–86]. In general, antibodies are coupled to beads and are used to capture tagged or endogenous bait proteins ().
Figure 3. AP-MS approaches. (A) AP of endogenous proteins is a method to identify bait interactors using bait-specific antibodies, which are coupled to beads. (B) Single-tagged bait proteins are expressed in cells and affinity purified using a tag-reactive antibody. (C) The TAP-tag consists of two biochemical tags used for purification. In the classical TAP, the first tag is the ZZ domain of protein-A followed by a TEV protease cleavage site and a second tag. This method involves two consecutive steps of purification
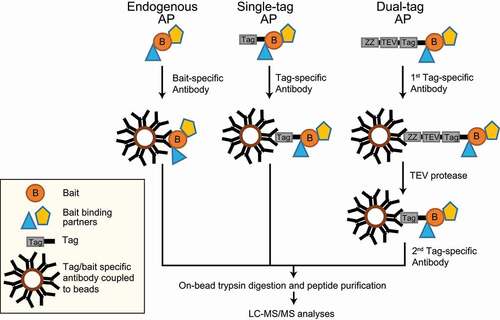
We will briefly explain the two most common AP approaches: (i) antibody-based immunoprecipitation of endogenous proteins and (ii) affinity tags, with critical examples related to mammalian autophagy. In antibody-based purifications, the endogenous bait along with its binding partners can be enriched in their native environment. For instance, autophagy induction by the tumor suppressor protein CDKN2A/ARF, which is a growth suppressor localizing to the nucleolus under growth conditions and to mitochondria under autophagy-inducing conditions, was discovered by an AP-MS approach. Endogenous CDKN2A and its binding partners were enriched using an anti-CDKN2A antibody prior to MS measurements. Under autophagy conditions, CDKN2A interacted with BCL2L1/Bcl-XL at mitochondria, interfering with the BCL2L1-BECN1 interaction and freeing BECN1 to bind to the PIK3C3 complex to promote autophagosome biogenesis [Citation87]. In general, immunoprecipitations of endogenous proteins coupled to MS generate information on native interactions of baits, though there are shortcomings to this method. Firstly, antibodies can bind to different bait isoforms or splice variants, reducing the resolution of interactions. Secondly, protein interactions can be disrupted due to competition between an antibody and interacting proteins, thereby losing information on such interactions. Thirdly, testing and choosing the right high-quality antibody for the immunoprecipitation can be cost-intensive. Finally, a control antibody might not represent the same background binders even though the antibody is species and isotype-matched [Citation88].
Years of research and tremendous improvements in protein engineering helped in developing an array of affinity tags differing in size, length, and affinity to study interactomes. These affinity tags can be classified as protein- and peptide-based tags. There are plenty of single-peptide affinity tags: HA (hemagglutinin), FLAG, MYC/c-myc, 6x/8x His, 2x SBP (streptavidin-binding peptide), and CBP (calmodulin-binding peptide), to name a few. In addition, there are protein tags e.g., GFP (green fluorescent protein), MBP (maltose binding protein), and GST (glutathione-S-transferase) [Citation89]. Next to single tags dual tags exist, consisting of a protein domain/peptide, a cleavage site, and a second peptide tag collectively used for TAP [Citation90]. These are widely used two-step protein purification methods to isolate rather clean protein complexes in yeast. In its initial variant, the tag consists of two IgG-binding units or Z-domains from protein A followed by a tobacco etch virus (TEV) protease cleavage site and a CBP peptide. This purification involves the usage of IgG-coupled beads, which capture the protein A domain, followed by cleavage and release of the complex by TEV protease. Using calmodulin-coupled beads, the CBP-tagged protein complex is captured and purified in a second step. Though this strategy works well in yeast cells and significantly reduces nonspecific background proteins, it was rather inefficient in mammalian cells. Another TAP tag called GS-TAP was developed to purify proteins from mammalian cells [Citation91]. The tag consists of a protein G domain, a TEV protease cleavage site, and an SBP peptide. One advantage of using this tag is the 10-fold increased efficiency of bait purification; moreover, the two-step purification can be skipped using streptavidin beads and eluting the bait with biotin [Citation91].
Aforementioned tags were used in many studies to uncover PPIs modulating sequential events in autophagosome biogenesis. Here, we focus on studies performed in mammalian cells and refer the reader to some excellent articles explaining in detail the studies that have so far been carried out in yeast to understand autophagy [Citation92–95]. A list of crucial AP-MS studies that helped in uncovering the functional network of interactions modulating different events in autophagy using ATG proteins expressed in mammalian cell lines are listed in . A seminal AP-MS study on autophagy-related proteins and proteins involved in autophagosome biogenesis utilized the expression of 65 HA-tagged bait proteins, of which 32 were primary and 33 secondary baits, in HEK293T cells. Primary baits were chosen based on their functional links to autophagy and vesicle trafficking. To validate the interaction network of primary baits, secondary baits were chosen based on high interconnectivity with primary baits and functional domains or gene ontology (GO) terms linked to autophagy. Bait proteins and interaction partners were purified using anti-HA beads prior to MS measurements. The analysis of this large-scale proteomics dataset identified 2,553 potential interactors, of which 409 high-confidence candidate interaction proteins with 759 interactions were shortlisted, which revealed a global interaction network involved in mammalian autophagy. These interactions revealed the involvement of proteins with various functionalities, among others protein kinases, PtdIns3P-binding proteins, lipid transport proteins, lipid kinases, and protein ubiquitination machinery. New functional links between various proteins were revealed, as for example, association of ULK1 and ULK2 with AMPK, thus indicating a crosstalk in energy sensing. The authors performed extensive AP-MS analyses of Atg8 homologs comparing nutrient-rich and autophagy-inducing (Torin-1 treatment which inhibits MTORC1) conditions. Interestingly, the extensive overlap of interactomes from ATG8 homologs showed a functional redundancy between these proteins. This overlap of interactions is likely due to the presence of a conserved LIR docking site in ATG8 homologs interacting with cargo receptors/substrates having a conserved hydrophobic LIR [Citation96]. GST-tagged LC3 variants were also used to identify interacting partners by LC-tandem mass spectrometry (MS/MS): e.g., GST-tagged LC3B bound to glutathione-sepharose led to the identification of FYCO1 as novel LIR-dependent LC3B interactor. FYCO1 was shown to also bind RAB7A and PtdIns3P and to promote microtubule-dependent transport of autophagic vesicles [Citation97]. GST-tagged GABARAPL1 was employed to characterize its interaction with HSP90 family members, HSP90AA1 activity being critical for GABARAPL1 stability [Citation98].
Table 1. AP-MS studies of ATG proteins
Moreover, AP-MS approaches were used to analyze organellar compositions, representing a valuable alternative approach for the characterization of cellular stress-response pathways. SILAC-labeled MCF-7 cells expressing eGFP-LC3 were utilized to compare autophagosome proteome changes upon different stress conditions. Vesicle fractionations in combination with anti-GFP-based enrichments, were coupled to MS analyses to reveal stimulus-dependent autophagosome proteomes [Citation99]. Affinity-purified lysosomes were used to profile proteome changes under nutrient-rich and autophagy conditions. The authors expressed 3x HA-tagged TMEM192, a lysosomal membrane protein, in HEK293T cells and performed anti-HA AP to enrich lysosomes prior MS-based identification and quantification. They identified a change in the localization of a protein called NUFIP1 (nuclear FMR1 interacting protein 1), which shifted from the nucleus toward lysosomes/autophagosomes during starvation-induced autophagy and suggested that NUFIP1 might act as a potential receptor for ribophagy [Citation100]. However, in a recent unbiased approach that studied the contributions of protein translation and degradation to ribosomal protein abundance, the role of NUFIP1 in ribosomal protein degradation could not be confirmed, questioning its function in ribophagy [Citation101].
Post-translational modifications such as phosphorylation, ubiquitination, and sumoylation modulate interactions between autophagy proteins at a particular spatial and temporal resolution [Citation102]. For example, AP-MS studies were performed to identify phosphorylation sites on ULK1. N-terminal TAP-tagged mouse ULK1 was expressed and purified from HEK293T cells prior to LC-MS/MS measurements. A total of 16 novel phosphorylation sites on ULK1 were identified, which also included autophosphorylation sites. The authors suggested that phosphorylation at Ser867 and Ser913 of ULK1 might promote its association with ATG13 and RB1CC1 to form an active autophagy initiation complex [Citation103].
AP methods coupled to either western blot or MS allow analyses of protein complexes in all organelles and compartments without the requirement of prefractionation. The aforementioned examples highlight the robustness of these approaches in identifying PPIs and PPI-modulating PTMs to shed light on the molecular mechanisms in autophagy pathways. However, these approaches also have shortcomings. In general, co-purifying contaminants or nonspecific proteins, either binding to the solid matrix or the antibody, are also enriched, making it sometimes hard to distinguish false and true interactors [Citation104,Citation105]. Usage of quantitative proteomic approaches and analyzing data against contaminant repositories, such as Contaminant Repository for Affinity Purification MS Data (CRAPome), can help in identifying contaminants [Citation106]. Due to overexpression, bait proteins are prone to problems like altered localization, protein misfolding, and aggregation. However, bait levels can be controlled via inducible promoters. In addition, CRISPR approaches are now being used to perform genome editing, enabling the expression of tagged proteins at endogenous levels [Citation107]. Usage of either CRISPR knockout or RNAi approaches that remove the endogenous protein can be efficiently used as negative controls [Citation86,Citation88,Citation108]. Also, the localization of the affinity tag, either at the N- or C-terminus of the bait, has to be critically evaluated. In rare cases, internal tags have been used to address specific biochemical questions such as linear ubiquitination [Citation109]. For the analysis of endogenous proteins, choosing the right mammalian cell line is essential. Commonly, cells expressing high amounts of bait proteins simplify subsequent mechanistic studies.
Critically, AP-MS data are commonly binary and do, therefore, neither yield insights into the structure (and stoichiometry) of complexes nor differentiate between direct and indirect interaction partners. Importantly, AP can isolate only stable or strong bait interactors with nanomolar or higher affinities; thus, dynamic, weak, and transient interactions are often missed [Citation110].
Proximity labeling-mass spectrometry to study weak protein-protein interactions and protein neighborhoods
PL-MS-based quantitative proteomics enables the identification and characterization of weak transient PPIs and neighborhoods. To this end, different classes of enzymes, such as biotin ligases, PTM ligases, and peroxidases, are used to label protein neighborhoods () [Citation111–113]. These enzymes are fused to bait proteins and catalyze the covalent transfer of a chemical group to proximal proteins. Biotin ligases are enzymes that catalyze, in an ATP-dependent reaction, the conversion of biotin to a reactive biotinoyl-5ʹAMP intermediate. Biotinoyl-5ʹAMP reacts with exposed lysine residues of proximal proteins, leading to the covalent attachment of biotin within an estimated radius of 10 nm around the enzyme [Citation114,Citation115]. A classical proximity-labeling technique, called proximity-dependent biotin identification (BioID), utilizes the biotin ligase BirA, a monomeric 35.4-kDa protein, from Escherichia coli that has been further engineered to BirAR118G to improve its catalytic activity (also referred to as BirA*, with “*” indicating its promiscuous activity). Cells expressing BioID-fused bait proteins are incubated with biotin for 16–24 h to reach maximum labeling efficiency. This relatively long incubation time is required to generate sufficient proximally biotinylated proteins, which can then be subsequently enriched using classical high-affinity streptavidin or neutravidin beads prior to LC-MS/MS sample preparation and measurements () [Citation116].
Figure 4. Proximity labeling enzymes to study protein neighborhoods. Proximity labeling ligases can be classified into three types based on their activity: biotin ligases, PTM ligases and peroxidases. Biotin ligases (BioID, BioID2, AirID and BASU) catalyze the conversion of biotin to a reactive biotin intermediate, which labels lysine residues of proximal proteins. In presence of H2O2, peroxidases (APEX2 and HRP) convert biotin phenol to biotin-phenoxyl radical, which labels electron-rich amino acid residues such as Tyr. PTM ligases add peptide/protein tags to proximal proteins
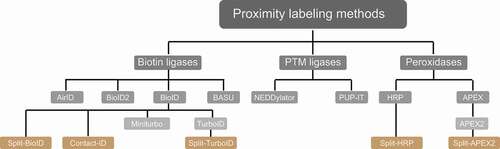
Figure 5. PL-MS approaches. (A) Biotin ligases and peroxidases generate reactive biotin/biotin-phenol intermediates, respectively, which tag proximal proteins. (B) Split variants of biotin ligases and peroxidases are used to identify proximal proteins, while two bait proteins interact to form a complex. Interactions of proteins bring the N/C terminal fragments of the ligases in close proximity allowing the formation of an active PL holo-enzyme. After cell lysis, biotinylated proteins are enriched using classical streptavidin-based enrichment prior to bottom-up LC-MS/MS analysis
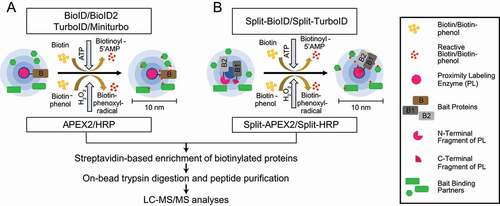
Introducing further mutations to BioID improved its labeling efficiency several-fold and led to two other commonly used biotin ligases called TurboID and miniTurbo [Citation117]. Compared to wild-type BirA, the improved 35 kDa TurboID variant contains a total of 15 mutations, amongst these a substitution of Arg118 to Ser. By deleting the N-terminal DNA-binding domain, a slightly smaller version of TurboID of 28 kDa, called miniTurbo, has also been generated. MiniTurbo harbors 13 out of the 15 mutations of TurboID. These two tags help in identifying snapshots of protein interactions using labeling times of only 5–15 min (). A drawback of TurboID is its high affinity toward biotin, which can lead to the usage of endogenous biotin, thereby disrupting biotin-dependent metabolic pathways and leading to toxicity in certain model organisms [Citation117]. A smaller version of BirA of 28 kDa, BioID2, which naturally lacks the N-terminal DNA-binding domain, was identified in the thermophile Aquifex aeolicus. The interesting feature of this enzyme is the reduced background of DNA/chromatin interacting proteins due to absence of the DNA-binding domain [Citation118]. Overall, labeling time and activity of BioID2 are comparable to the one of BioID. Another biotin ligase variant, termed BirA from Bacillus subtilis (BASU), was engineered without DNA-binding domain. This ligase was used to identify novel RNA-binding and proximal proteins; the respective approach was termed RNA-protein interaction detection (RaPID) [Citation119]. For this, BASU was fused to the λN peptide, which binds bacteriophage lambda BoxB stem-loops. BoxB stem-loops are synthesized to flank the respective RNA-of-interest. Binding of the ligase to the stem-loop structure led to biotinylation of proteins binding to the specific RNA-of-interest within a 10 nm radius. At labeling times of 1 min, BASU showed more than >30-fold increased signal-to-noise ratios compared to BioID and BioID2 [Citation119]. One of the most recent technical developments is the generation of ancestral BirA for proximity-dependent biotin identification (AirID). Metagenome data and ancestral sequence reconstruction coupled to site-directed mutagenesis gave rise to this BirA variant with 82% sequence similarity to BioID, which labels proteins in vitro or in vivo in 3 h using lower concentrations of biotin than in the original approach [Citation120].
Table 2. Commonly used PL methods
PTM ligases are enzymes that commonly add tags onto lysine residues of bait-proximal proteins. Pupylation-based interaction tagging (PUP-IT) is a method that was developed to identify PPIs at membranes. This approach involves the expression of a bait protein fused to the bacterial Pup ligase PafA and co-expression of a Pup (prokaryotic ubiquitin-like protein) variant with a C-terminal Gly-Gly-Glu sequence. The Pup ligase catalyzes the phosphorylation of the C-terminal Glu residue, leading to its activation and conjugation to lysine residues of proteins that are in proximity to the bait. Due to the low diffusible nature of the activated Pup tag a smaller labeling radius is achieved, thus reducing background. The major shortcoming of this method is the large size of ~54 kDa of the ligase and its low catalytic activity, which render it inadequate to capture snapshots of PPIs [Citation121]. Neddylation by the 76 amino acids large protein NEDD8 is a ubiquitin-like modification, which has been exploited to identify PPIs. For this, a modified version of the NEDD8 E2-conjugating enzyme UBE2M/UBC12 called NEDDylator is fused to a protein or a small molecule. The specific tagging of NEDD8 to proximal proteins happens via the nucleophilic attack of prey lysine epsilon amino groups to thioester-bound NEDD8 on the bait-NEDDylator. This confirms the direct contact between bait and prey proteins, which ensures neddylation of potential binding partners. This tool has been successfully coupled to SILAC-based quantitative MS to identify small molecule-protein and protein-protein interactions in mammalian cells [Citation122,Citation123].
Peroxidases are oxidoreductases that catalyze redox reactions in the presence of hydrogen peroxide. This includes APEX (ascorbate peroxidase) and HRP (horseradish peroxidase) enzymes, which catalyze the conversion of biotin-phenol to biotin-phenoxyl radical in the presence of hydrogen peroxide (H2O2) (). This membrane-impermeable reactive radical covalently labels electron-rich amino acids, majorly Tyr and to some extent Trp, Cys, and Phe, of proteins proximal to the bait within a 10 nm radius. APEX was initially identified in pea and engineered to reduce its dimerization property and improve its catalytic activity. This enzyme contributes to polymerization and deposition of diaminobenzidine for electron microscopy (EM) studies. APEX2 from soybean, a 28-kDa enzyme, was engineered to improve its catalytic activity allowing labeling times of 1 min or less in mammalian cells [Citation124–126]. HRP is another peroxidase of 44 kDa used for both EM and PL studies [Citation127]. Due to its inactivity in cytosol, it is majorly used to study cell surface molecules and secretory pathways targeting the enzyme via either ligands or antibodies to cell surfaces, using techniques like selective proteomic proximity labeling assay using tyramide (SPPLAT) and enzyme-mediated activation of radical sources (EMARS) [Citation128]. Alternatively, antibody-conjugated HRP was used intracellularly in fixed tissues and cells to perform PL of bait proteins in techniques such as biotinylation by antibody recognition (BAR) [Citation129]. Overall, due to its usage in EM studies and its fast labeling time, APEX2 is the most widely used technique revealing snapshots of PPIs and enabling analyses of stimulus-dependent interactomes. However, APEX2 is less active compared to HRP and sensitive to H2O2-mediated inhibition.
Notably, tremendous improvements have been made in protein fragment complementation assays (PCA) coupled to PL approaches. Split variants of labeling enzymes were generated using inactive N- and C-terminal fragments of BioID or APEX2 (). The respective fragments are fused to two different baits known to interact with each other. Interaction between the baits enables the reconstitution of the holo-enzyme allowing biotinylation of proximal proteins. This technique enables identification of interactions depending on interaction of two bait proteins at a specific spatial and temporal resolution. Split-BioID was the first variant of this approach [Citation130]; however, longer labeling time and lower activity of the reconstituted complex made this split version non-suitable to study dynamic interactions [Citation131]. These hurdles were resolved with the recent discovery of split versions based on HRP, APEX2, and TurboID. Split HRP was used, for example, to study cell-cell interactions [Citation132]. The initially made split-APEX2 was less active compared to its full-length variant and led to a second split version with additional nine mutations, which improved activity and specificity [Citation133,Citation134]. Also recently, a version of split-TurboID was developed for the analyses of PPI of complexes, organelle and cell contact sites [Citation135]. For more technical information, we would like to refer readers to recent reviews [Citation111–113]. In the remainder of this manuscript, we focus on studies using PL approaches coupled to MS to understand autophagy mechanisms.
Up to date, only a few studies utilized PL coupled to MS-based quantitative proteomics to uncover the roles of proteins involved in autophagy (). TBC1D14, a TBC domain containing protein, has a strong effect on the structure and function of recycling endosomes and negatively regulates the formation of autophagosome upon overexpression [Citation136]. BioID coupled to MS analysis of TBC1D14 identified its interaction with TRAPPC8, a subunit of trafficking protein particle III (TRAPP-III), a multimeric protein complex with guanine exchange factor (GEF)-activity toward RAB1B, promoting its GTP-loaded state. TRAPP-III regulates the cycling of ATG9 from early endosomes and the Golgi apparatus to the ATG9 compartment. Overexpression of TBC1D14 led to mislocalization of TRAPPC8 onto recycling endosome tubules, to a fragmented Golgi apparatus, and to disruption of the Golgi ATG9 pool leading to inhibition of autophagosome formation [Citation137].
Table 3. PL-MS studies in autophagy research
APEX2 labeling of human ATG8 homologs coupled to MS-based quantitative proteomics was used to analyze autophagosome content. APEX2-LC3C labeling identified a reproducible interaction of LC3C with a protein called MTX1 (metaxin 1). MTX1 binds to SAMM50 located on the outer mitochondrial membrane. Together with MTX2 bound to the cytosolic face of MTX1, these proteins form the sorting and assembly machinery (SAM) complex. This complex, together with the mitochondrial contact site and cristae junction organizing system (MICOS), maintain cristae structure, mitochondrial morphology, and homeostasis. Colocalization studies and functional biochemical analyses of MTX1 revealed its role in autophagic clearance of parts of damaged mitochondria in a piecemeal fashion via LC3C and SQSTM1 [Citation107]. Recently, new roles of LC3s in protein secretion were identified using PL-MS. RNA-binding proteins and small non-coding RNAs were shown to be packed into extracellular vesicles in a MAP1LC3B and LC3-conjugation-machinery-dependent manner [Citation138]. Also, GABARAP was identified in extracellular vesicles using cells expressing APEX2-GABARAP. Interestingly, these extracellular vesicles also contained proteins with which GABARAP was shown to interact inside autophagosomes, further supporting a crosstalk between autophagy and protein secretion [Citation139].
APEX2 labeling of the mitophagy receptors OPTN, OPTND474N a ubiquitin-binding defective mutant, and TAX1BP1 in HeLa cells coupled to TMT (8/9 plex)-based quantitative MS analysis was performed comparing antimycin A/oligomycin A (inducing depolarization of mitochondria) treatments for 1 and 3 h with non-treated cells to identify proteins proximal to the receptors during mitophagy. In combination with a CRISPR-based genetic screen coupled to mitophagy flux assays, HK2 (hexokinase-2) was characterized as a scaffold, forming a 700-kDa complex consisting of PINK1-PRKN and other ubiquitinated proteins, essential for the clearance of damaged mitochondria [Citation140].
The role of galectins in maintenance, repair, removal, and biogenesis of lysosomes upon injury has been extensively characterized using APEX2 labeling coupled to quantitative MS. Galectins are beta-galactoside binding proteins with an intrinsic carbohydrate recognition domain (CRD). This property helps in sensing membrane damage due to exposure of membrane glycans. LGALS8 (galectin 8) was shown to induce autophagy upon endomembrane damage by regulating MTORC1 activity via changes in the activation state of RRAG GTPases [Citation141]. APEX2-LGALS9 labeling revealed its role in lysosomal damage sensing via activation of AMPK. Upon lysosomal damage, LGALS9 displaces the deubiquitinase USP9X from MAP3K7/TAK1 kinase, thereby promoting K63-linked polyubiquitination and activation of the kinase. MAP3K7 in turn activates AMPK and thereby autophagy by phosphorylation of Thr172 [Citation142]. Previously, LGALS3 was known to promote TRIM16 based autophagic removal of damaged lysosomes and activate TFEB, a transcription factor for lysosome biogenesis [Citation143]. In addition, APEX2-LGALS3 labeling was performed to understand its role in the repair and clearance of endomembrane damage via autophagy. LGALS3 helped in recruiting the ESCRT component PDCD6IP/ALIX upon lysosomal membrane damage. LGALS3 promoted the interaction of PDCD6IP with CHMP4B, which collectively mediated scission and closure of lysosomal membranes [Citation144].
Thus, the aforementioned examples show the impact of PL-based MS in understanding autophagy-relevant mechanisms. PL provides information about the proximal proteome change around the bait of interest at a given spatial and temporal resolution. However, PL methods reveal many proximal neighbors and potentially interacting proteins leading to an inherently high background. Therefore, the controls used to distinguish true versus false-positive interactors are critical. Commonly, five different types of controls are employed: (i) cells without any PL enzyme treated with biotin/biotin-phenol, (ii) the enzyme fused to an unrelated protein such as GFP or RFP, (iii) the enzyme fused to an inactive or mutant version of the respective bait, (iv) the enzyme fused to a compartment-specific, unrelated protein, which localizes similarly to the bait, and (v) cells with the free PL enzyme [Citation111,Citation145]. Given the variety of controls with their intrinsic pros and cons, choosing the right control for the desired PL experiment is still a debate in the field. We see large differences in enrichments of baits and their binding partners depending on the used control conditions (unpublished data). Whereas control (i) leads to high enrichment rates, the number of false positives appears high. In contrast, due to the high activity of free enzymes in control (v), enrichment rates are low, and the number of false-negatives appears high, i.e., weak transient interactions seem to be lost. Thus, we favor controls (ii)-(iv) in which PL enzyme-tagged bait proteins are compared to PL enzyme-tagged control/unrelated proteins. By using inducible expression systems, PL enzyme-bait protein amounts can be titrated to avoid high expression levels, which again increases background signals of nonspecifically enriched proteins. Additionally, one should consider that BioID-based approaches rely on the availability of accessible lysine residues and APEX2 methods on electron-rich amino acid residues, i.e., tyrosine, in proximal proteins. Importantly, problems could arise from toxicity issues related to overexpressed PL enzymes e.g., via protein aggregation, mislocalization, and functional inactivation of baits due to the fused PL enzyme. Hence, experimental characterizations of PL enzymes and controls are essential to design meaningful experiments for studying complex biological questions.
Conclusions and outlook
Over two decades, there has been a significant increase in the understanding of mechanisms regulating autophagy. Technological improvements contributed to the expanding list of autophagy-related and -associated proteins. MS-based proteomics studies helped tremendously in identifying and characterizing relevant proteins. Overall, various factors influence the identification of PPIs, like the range of affinities and composition of complexes, next to intrinsic methodological shortcomings of the used analytical approach. Thus, in-depth knowledge of potential methods and understanding of influential experimental factors that might modulate PPIs is essential for choosing appropriate approaches to characterize any PPI. Here, we introduced various MS-based methods available to study PPIs, explained in detail principles and applications of AP-MS- and PL-MS-based approaches, and listed examples of autophagy relevant studies. There are still various unanswered, mechanistic questions like: which membrane sources are employed under which conditions for autophagosome biogenesis? Which factors influence the localization of autophagy initiation complexes at ER sites? Which triggering factors regulate the balance between selective and nonselective autophagy, and how do proteins modulate autophagosome size and shape under these conditions? Extensive characterizations of PTMs modulating PPIs are essential for understanding the above questions. In the future, it will be essential to combine the analyses of PTMs and PPIs. The increasing scanning speed and sensitivity of mass spectrometers will help in generating more detailed views of the regulation of PTMs. Both DIA and DDA methods will likely contribute to generating truly comprehensive datasets that will also be useful for systems biology-based approaches. However, the limited dynamic range of mass spectrometers still poses a challenge to be addressed. Modified peptides still have to be enriched, but enrichment approaches often differ depending on which PTMs are analyzed, impeding the study of PTM crosstalk. Top-down proteomics approaches might partially address this issue. Finally, the growing field of MS-based lipidomics will be essential to fully understand the membrane dynamics underlying autophagy. Thus, we believe that cutting-edge MS approaches will continue to help to address autophagy-related questions and lead to a comprehensive understanding of autophagy-related processes.
Acknowledgments
We apologize to all colleagues and research labs whose work was not covered in this review due to space constraints. We thank M. Stumpe and D. Kressler of the Proteomics Platform Fribourg for carefully reading the manuscript. The research leading to this review is generously supported by the Swiss National Science Foundation and the canton of Fribourg.
Disclosure statement
The authors declare no competing interests
Additional information
Funding
References
- Mizushima N, Klionsky DJ. Protein turnover via autophagy: implications for metabolism. Annu Rev Nutr. 2007;27:19–40.
- Rubinsztein DC, Bento CF, Deretic V. Therapeutic targeting of autophagy in neurodegenerative and infectious diseases. J Exp Med. 2015;212(7):979–990.
- Levine B, Kroemer G. Autophagy in the pathogenesis of disease.Cell. 2008 [cited 2008 Jan 11];132(1):27–42.
- Chiang HL, Terlecky SR, Plant CP, et al. A role for a 70-kilodalton heat shock protein in lysosomal degradation of intracellular proteins. Science. 1989 Oct 20;246(4928):382–385.
- Cuervo AM, Wong E. Chaperone-mediated autophagy: roles in disease and aging. Cell Res. 2014 Jan;24(1):92–104.
- Kaushik S, Cuervo AM. Chaperone-mediated autophagy: a unique way to enter the lysosome world. Trends Cell Biol. 2012;22(8):407–417.
- Mintern JD, Villadangos JA. Autophagy and mechanisms of effective immunity. Front Immunol. 2012;3:60.
- Nakatogawa H. Mechanisms governing autophagosome biogenesis. Nat Rev Mol Cell Biol. 2020 May 5;21(8):439–458.
- Ohsumi Y. Historical landmarks of autophagy research. Cell Res. 2014 Jan;24(1):9–23.
- Klionsky DJ, Baehrecke EH, Brumell JH, et al. A comprehensive glossary of autophagy-related molecules and processes (2nd edition). Autophagy. 2011 Nov;7(11):1273–1294.
- Dikic I, Elazar Z. Mechanism and medical implications of mammalian autophagy. Nat Rev Mol Cell Biol. 2018 June;19(6):349–364.
- Rostislavleva K, Soler N, Ohashi Y, et al. Structure and flexibility of the endosomal Vps34 complex reveals the basis of its function on membranes. Science (New York, NY). 2015;350(6257):aac7365–aac7365.
- McAlpine F, Williamson LE, Tooze SA, et al. Regulation of nutrient-sensitive autophagy by uncoordinated 51-like kinases 1 and 2. Autophagy. 2013 Mar;9(3):361–373.
- Zachari M, Ganley IG. The mammalian ULK1 complex and autophagy initiation. Essays Biochem. 2017;61(6):585–596.
- Zhao YG, Liu N, Miao G, et al. The ER contact proteins VAPA/B interact with multiple autophagy proteins to modulate autophagosome biogenesis. Curr Biol. 2018 Apr 23;28(8):1234–1245.e4.
- Ungermann C, Reggiori F. Atg9 proteins, not so different after all. Autophagy. 2018;14(8):1456–1459.
- Puri C, Renna M, Bento CF, et al. Diverse autophagosome membrane sources coalesce in recycling endosomes. Cell. 2013 Sep 12;154(6):1285–1299.
- Di Bartolomeo S, Corazzari M, Nazio F, et al. The dynamic interaction of AMBRA1 with the dynein motor complex regulates mammalian autophagy. J Cell Biol. 2010 Oct 4;191(1):155–168.
- Egan DF, Chun MG, Vamos M, et al. Small molecule inhibition of the autophagy kinase ULK1 and identification of ULK1 substrates. Mol Cell. 2015 July 16;59(2):285–297.
- Russell RC, Tian Y, Yuan H, et al. ULK1 induces autophagy by phosphorylating Beclin-1 and activating VPS34 lipid kinase. Nat Cell Biol. 2013 July;15(7):741–750.
- Wold MS, Lim J, Lachance V, et al. ULK1-mediated phosphorylation of ATG14 promotes autophagy and is impaired in Huntington’s disease models. Mol Neurodegener. 2016 Dec 9;11(1):76.
- Axe EL, Walker SA, Manifava M, et al. Autophagosome formation from membrane compartments enriched in phosphatidylinositol 3-phosphate and dynamically connected to the endoplasmic reticulum. J Cell Biol. 2008;182(4):685–701.
- Proikas-Cezanne T, Takacs Z, Donnes P, et al. WIPI proteins: essential PtdIns3P effectors at the nascent autophagosome. J Cell Sci. 2015 Jan 15;128(2):207–217.
- Bakula D, Müller AJ, Zuleger T, et al. WIPI3 and WIPI4 β-propellers are scaffolds for LKB1-AMPK-TSC signalling circuits in the control of autophagy. Nat Commun. 2017;8:15637.
- Valverde DP, Yu S, Boggavarapu V, et al. ATG2 transports lipids to promote autophagosome biogenesis. J Cell Biol. 2019 June 3;218(6):1787–1798.
- Zhang H. Lipid transfer at ER-isolation membrane contacts. Nat Rev Mol Cell Biol. 2020 Mar;21(3):121.
- Geng J, Klionsky DJ. The Atg8 and Atg12 ubiquitin-like conjugation systems in macroautophagy. ‘Protein modifications: beyond the usual suspects’ review series. EMBO Rep. 2008;9(9):859–864.
- Mizushima N, Noda T, Yoshimori T, et al. A protein conjugation system essential for autophagy. Nature. 1998 Sep 24;395(6700):395–398.
- Dooley HC, Razi M, Polson HE, et al. WIPI2 links LC3 conjugation with PI3P, autophagosome formation, and pathogen clearance by recruiting Atg12-5-16L1. Mol Cell. 2014 July 17;55(2):238–252.
- Weidberg H, Shvets E, Shpilka T, et al. LC3 and GATE-16/GABARAP subfamilies are both essential yet act differently in autophagosome biogenesis. EMBO J. 2010 June 2;29(11):1792–1802.
- Kirisako T, Baba M, Ishihara N, et al. Formation process of autophagosome is traced with Apg8/Aut7p in yeast. J Cell Biol. 1999 Oct 18;147(2):435–446.
- Birgisdottir ÅB, Lamark T, Johansen T. The LIR motif - crucial for selective autophagy. J Cell Sci. 2013 Aug 1;126(Pt 15):3237–3247.
- Wild P, McEwan DG, Dikic I. The LC3 interactome at a glance. J Cell Sci. 2014 Jan 1;127(Pt 1):3–9.
- Klionsky DJ, Eskelinen E-L, Deretic V. Autophagosomes, phagosomes, autolysosomes, phagolysosomes, autophagolysosomes … wait, I’m confused. Autophagy. 2014;10(4):549–551.
- Nakamura S, Yoshimori T. New insights into autophagosome-lysosome fusion. J Cell Sci. 2017 Apr 1;130(7):1209–1216.
- Saxton RA, Sabatini DM. mTOR signaling in growth, metabolism, and disease. Cell. 2017 Mar 9;168(6):960–976.
- Wong PM, Feng Y, Wang J, et al. Regulation of autophagy by coordinated action of mTORC1 and protein phosphatase 2A. Nat Commun. 2015 Aug 27;6:8048.
- Podobnik M, Krasevec N, Bedina Zavec A, et al. How to study protein-protein interactions. Acta Chim Slov. 2016;63(3):424–439.
- Bensimon A, Heck AJ, Aebersold R. Mass spectrometry-based proteomics and network biology. Annu Rev Biochem. 2012;81:379–405.
- Perkins JR, Diboun I, Dessailly BH, et al. Transient protein-protein interactions: structural, functional, and network properties. Structure. 2010 Oct 13;18(10):1233–1243.
- Nooren IM, Thornton JM. Diversity of protein-protein interactions. EMBO J. 2003 July 15;22(14):3486–3492.
- Nooren IMA, Thornton JM. Structural characterisation and functional significance of transient protein–protein interactions.J Mol Biol. 2003 [cited 2003 Jan 31];325(5):991–1018.
- Acuner Ozbabacan SE, Engin HB, Gursoy A, et al. Transient protein-protein interactions. Protein Eng Des Sel. 2011 Sep;24(9):635–648.
- Noda Nobuo N, Satoo K, Fujioka Y, et al. Structural basis of Atg8 activation by a homodimeric E1, Atg7. Mol Cell. 2011;44(3):462–475.
- Montecinos-Franjola F, Schuck P, Sackett DL. Tubulin Dimer reversible dissociation: affinity, kinetics, and demonstration of a stable monomer. J Biol Chem. 2016;291(17):9281–9294.
- Mackeh R, Perdiz D, Lorin S, et al. Autophagy and microtubules – new story, old players. J Cell Sci. 2013;126(5):1071.
- Saxton RA, Chantranupong L, Knockenhauer KE, et al. Mechanism of arginine sensing by CASTOR1 upstream of mTORC1. Nature. 2016;536(7615):229–233.
- Jones S, Thornton JM. Analysis of protein-protein interaction sites using surface patches. J Mol Biol. 1997 Sep 12;272(1):121–132.
- Lo Conte L, Chothia C, Janin J. The atomic structure of protein-protein recognition sites. J Mol Biol. 1999 Feb 5;285(5):2177–2198.
- Metlagel Z, Otomo C, Takaesu G, et al. Structural basis of ATG3 recognition by the autophagic ubiquitin-like protein ATG12. Proc Natl Acad Sci U S A. 2013;110(47):18844–18849.
- Li X, He L, Che KH, et al. Imperfect interface of Beclin1 coiled-coil domain regulates homodimer and heterodimer formation with Atg14L and UVRAG. Nat Commun. 2012;3:662.
- Katsamba P, Carroll K, Ahlsen G, et al. Linking molecular affinity and cellular specificity in cadherin-mediated adhesion. Proc Natl Acad Sci U S A. 2009;106(28):11594–11599.
- Fontenete S, Peña-Jimenez D, Perez-Moreno M. Heterocellular cadherin connections: coordinating adhesive cues in homeostasis and cancer. F1000Res. 2017;6:1010.
- Nighot P, Ma T. Role of autophagy in the regulation of epithelial cell junctions. Tissue Barriers. 2016;4(3):e1171284–e1171284.
- Antón Z, Betin VMS, Simonetti B, et al. A heterodimeric SNX4: SNX7SNX-BAR autophagy complex coordinates ATG9A trafficking for efficient autophagosome assembly. J Cell Sci. bioRxiv. 2020;133(14):jcs246306. doi:https://doi.org/10.1242/jcs.246306.
- van Weering JRT, Sessions RB, Traer CJ, et al. Molecular basis for SNX-BAR-mediated assembly of distinct endosomal sorting tubules. EMBO J. 2012 [cited 2012 Nov 28];31(23):4466–4480.
- Ma M, Kumar S, Purushothaman L, et al. Lipid trafficking by yeast Snx4 family SNX-BAR proteins promotes autophagy and vacuole membrane fusion. Mol Biol Cell. 2018;29(18):2190–2200.
- Keskin O, Gursoy A, Ma B, et al. Principles of protein-protein interactions: what are the preferred ways for proteins to interact? Chem Rev. 2008 Apr;108(4):1225–1244.
- Gagarinova A, Phanse S, Cygler M, et al. Insights from protein-protein interaction studies on bacterial pathogenesis. Expert Rev Proteomics. 2017 [cited 2017 Sep 2];14(9):779–797.
- Yu H, Braun P, Yildirim MA, et al. High-quality binary protein interaction map of the yeast interactome network. Science (New York, NY). 2008;322(5898):104–110.
- Hayes S, Malacrida B, Kiely M, et al. Studying protein–protein interactions: progress, pitfalls and solutions. Biochem Soc Trans. 2016;44(4):994–1004.
- Timp W, Timp G. Beyond mass spectrometry, the next step in proteomics. Sci Adv. 2020;6(2):eaax8978.
- Han X, Aslanian A, Yates JR 3rd. Mass spectrometry for proteomics. Curr Opin Chem Biol. 2008;12(5):483–490.
- Aebersold R, Mann M. Mass-spectrometric exploration of proteome structure and function. Nature. 2016 Sep 15;537(7620):347–355.
- Gillet LC, Leitner A, Aebersold R. Mass spectrometry applied to bottom-up proteomics: entering the high-throughput era for hypothesis testing.Ann Rev Anal Chem. 2016 [cited 2016 June 12];9(1):449–472.
- Aebersold R, Mann M. Mass spectrometry-based proteomics. Nature. 2003 Mar 13;422(6928):198–207.
- Ludwig C, Gillet L, Rosenberger G, et al. Data-independent acquisition-based SWATH-MS for quantitative proteomics: a tutorial. Mol Syst Biol. 2018 [cited 2018 Aug 1];14(8):e8126.
- Bantscheff M, Schirle M, Sweetman G, et al. Quantitative mass spectrometry in proteomics: a critical review. Anal Bioanal Chem. 2007 [cited 2007 Oct 1];389(4):1017–1031.
- Doerr A. Mass spectrometry–based targeted proteomics.Nat Methods. 2013 [cited 2013 Jan 1];10(1):23.
- Cox J, Mann M. Quantitative, high-resolution proteomics for data-driven systems biology. Annu Rev Biochem. 2011;80:273–299.
- Merrill AE, Coon JJ. Quantifying proteomes and their post-translational modifications by stable isotope label-based mass spectrometry. Curr Opin Chem Biol. 2013 Oct;17(5):779–786.
- Wheatley DN, Scott L, Lamb J, et al. Single amino acid (arginine) restriction: growth and death of cultured HeLa and human diploid fibroblasts. Cell Physiol Biochem. 2000;10(1–2):37–55.
- Ong SE, Blagoev B, Kratchmarova I, et al. Stable isotope labeling by amino acids in cell culture, SILAC, as a simple and accurate approach to expression proteomics. Mol Cell Proteomics. 2002 May;1(5):376–386.
- Reynolds KJ, Yao X, Fenselau C. Proteolytic 18O labeling for comparative proteomics: evaluation of endoprotease Glu-C as the catalytic agent. J Proteome Res. 2002 Jan–Feb;1(1):27–33.
- Yao X, Freas A, Ramirez J, et al. Proteolytic 18O labeling for comparative proteomics: model studies with two serotypes of adenovirus. Anal Chem. 2001 [cited 2001 July 1];73(13):2836–2842.
- Thompson A, Schäfer J, Kuhn K, et al. Tandem mass tags: a novel quantification strategy for comparative analysis of complex protein mixtures by MS/MS. Anal Chem. 2003 Apr 15;75(8):1895–1904.
- Ross PL, Huang YN, Marchese JN, et al. Multiplexed protein quantitation in Saccharomyces cerevisiae using amine-reactive isobaric tagging reagents. Mol Cell Proteomics. 2004 Dec;3(12):1154–1169.
- Plubell DL, Wilmarth PA, Zhao Y, et al. Extended multiplexing of tandem mass tags (TMT) labeling reveals age and high fat diet specific proteome changes in mouse epididymal adipose tissue. Mol Cell Proteomics. 2017;16(5):873–890.
- Thompson A, Wölmer N, Koncarevic S, et al. TMTpro: design, synthesis, and initial evaluation of a proline-based isobaric 16-plex tandem mass tag reagent set. Anal Chem. 2019 [cited 2019 Dec 17];91(24):15941–15950.
- Neilson KA, Ali NA, Muralidharan S, et al. Less label, more free: approaches in label-free quantitative mass spectrometry. Proteomics. 2011 Feb;11(4):535–553.
- Bantscheff M, Lemeer S, Savitski MM, et al. Quantitative mass spectrometry in proteomics: critical review update from 2007 to the present. Anal Bioanal Chem. 2012 Sep;404(4):939–965.
- Ankney JA, Muneer A, Chen X. Relative and absolute quantitation in mass spectrometry–based proteomics.Ann Rev Anal Chem. 2016 [cited 2018 June 12];11(1):49–77.
- Lambert JP, Pawson T, Gingras AC. Mapping physical interactions within chromatin by proteomic approaches. Proteomics. 2012 May;12(10):1609–1622.
- Slobodin B, Gerst JE. RaPID: an aptamer-based mRNA affinity purification technique for the identification of RNA and protein factors present in ribonucleoprotein complexes. Methods Mol Biol. 2011;714:387–406.
- Tsai BP, Wang X, Huang L, et al. Quantitative profiling of in vivo-assembled RNA-protein complexes using a novel integrated proteomic approach. Mol Cell Proteomics. 2011 Apr;10(4):M110.007385.
- Oeffinger M. Two steps forward–one step back: advances in affinity purification mass spectrometry of macromolecular complexes. Proteomics. 2012 May;12(10):1591–1608.
- Pimkina J, Humbey O, Zilfou JT, et al. ARF induces autophagy by virtue of interaction with Bcl-xl. J Biol Chem. 2009 Jan 30;284(5):2803–2810.
- Dunham WH, Mullin M, Gingras AC. Affinity-purification coupled to mass spectrometry: basic principles and strategies. Proteomics. 2012 May;12(10):1576–1590.
- Terpe K. Overview of tag protein fusions: from molecular and biochemical fundamentals to commercial systems. Appl Microbiol Biotechnol. 2003 Jan;60(5):523–533.
- Rigaut G, Shevchenko A, Rutz B, et al. A generic protein purification method for protein complex characterization and proteome exploration. Nat Biotechnol. 1999 Oct;17(10):1030–1032.
- Burckstummer T, Bennett KL, Preradovic A, et al. An efficient tandem affinity purification procedure for interaction proteomics in mammalian cells. Nat Methods. 2006 Dec;3(12):1013–1019.
- Kolkman A, Daran-Lapujade P, Fullaondo A, et al. Proteome analysis of yeast response to various nutrient limitations. Mol Syst Biol. 2006;2:2006.0026.
- Becker AC, Bunkenborg J, Eisenberg T, et al. Friend or food. Autophagy. 2012 [cited 2012 June 23];8(6):995–996.
- Reggiori F, Klionsky DJ. Autophagic processes in yeast: mechanism, machinery and regulation. Genetics. 2013;194(2):341–361.
- Farré J-C, Subramani S. Mechanistic insights into selective autophagy pathways: lessons from yeast.Nat Rev Mol Cell Biol. 2016 [cited 2016 Sep 1];17(9):537–552.
- Behrends C, Sowa ME, Gygi SP, et al. Network organization of the human autophagy system. Nature. 2010 [cited 2010 July 1];466(7302):68–76.
- Pankiv S, Alemu EA, Brech A, et al. FYCO1 is a Rab7 effector that binds to LC3 and PI3P to mediate microtubule plus end-directed vesicle transport. J Cell Biol. 2010;188(2):253–269.
- Seguin-Py S, Lucchi G, Croizier S, et al. Identification of HSP90 as a new GABARAPL1 (GEC1)-interacting protein. Biochimie. 2012 Mar;94(3):748–758.
- Dengjel J, Hoyer-Hansen M, Nielsen MO, et al. Identification of autophagosome-associated proteins and regulators by quantitative proteomic analysis and genetic screens. Mol Cell Proteomics. 2012 Mar;11(3):M111.014035.
- Wyant GA, Abu-Remaileh M, Frenkel EM, et al. NUFIP1 is a ribosome receptor for starvation-induced ribophagy. Science (New York, NY). 2018;360(6390):751–758.
- An H, Ordureau A, Korner M, et al. Systematic quantitative analysis of ribosome inventory during nutrient stress. Nature. 2020 July;583(7815):303–309.
- Xie Y, Kang R, Sun X, et al. Posttranslational modification of autophagy-related proteins in macroautophagy. Autophagy. 2015;11(1):28–45.
- Dorsey FC, Rose KL, Coenen S, et al. Mapping the phosphorylation sites of Ulk1. J Proteome Res. 2009 [cited 2009 Nov 6];8(11):5253–5263.
- Morris JH, Knudsen GM, Verschueren E, et al. Affinity purification-mass spectrometry and network analysis to understand protein-protein interactions. Nat Protoc. 2014;9(11):2539–2554.
- Trinkle-Mulcahy L. Resolving protein interactions and complexes by affinity purification followed by label-based quantitative mass spectrometry. Proteomics. 2012 [cited 2012 May 1];12(10):1623–1638.
- Mellacheruvu D, Wright Z, Couzens AL, et al. The CRAPome: a contaminant repository for affinity purification-mass spectrometry data. Nat Methods. 2013 Aug;10(8):730–736.
- Le Guerroué F, Eck F, Jung J, et al. Autophagosomal content profiling reveals an LC3C-dependent piecemeal mitophagy pathway. Mol Cell. 2017 [cited 2017 Nov 16];68(4):786–796.e6.
- Selbach M, Mann M. Protein interaction screening by quantitative immunoprecipitation combined with knockdown (QUICK). Nat Methods. 2006 Dec;3(12):981–983.
- Akimov V, Olsen LCB, Hansen SVF, et al. StUbEx PLUS-A modified stable tagged ubiquitin exchange system for peptide level purification and in-depth mapping of ubiquitination sites. J Proteome Res. 2018 Jan 5;17(1):296–304.
- Schlosshauer M, Baker D. Realistic protein-protein association rates from a simple diffusional model neglecting long-range interactions, free energy barriers, and landscape ruggedness. Protein Sci. 2004 June;13(6):1660–1669.
- Samavarchi-Tehrani P, Samson R, Gingras A-C. Proximity dependent biotinylation: key enzymes and adaptation to proteomics approaches. Mol Cell Proteomics. 2020;19(5):757–773.
- Kim DI, Roux KJ. Filling the void: proximity-based labeling of proteins in living cells. Trends Cell Biol. 2016 Nov;26(11):804–817.
- Trinkle-Mulcahy L. Recent advances in proximity-based labeling methods for interactome mapping. F1000Res. 2019;8:F1000 Faculty Rev–135.
- Kim DI, Birendra KC, Zhu W, et al. Probing nuclear pore complex architecture with proximity-dependent biotinylation. Proc Natl Acad Sci U S A. 2014 June 17;111(24):E2453–61.
- Rees JS, Li X-W, Perrett S, et al. Protein neighbors and proximity proteomics. Mol Cell Proteomics. 2015;14(11):2848–2856.
- Roux KJ, Kim DI, Raida M, et al. A promiscuous biotin ligase fusion protein identifies proximal and interacting proteins in mammalian cells. J Cell Biol. 2012;196(6):801–810.
- Branon TC, Bosch JA, Sanchez AD, et al. Efficient proximity labeling in living cells and organisms with TurboID. Nat Biotechnol. 2018;36(9):880–887.
- Kim DI, Jensen SC, Noble KA, et al. An improved smaller biotin ligase for BioID proximity labeling. Mol Biol Cell. 2016;27(8):1188–1196.
- Ramanathan M, Majzoub K, Rao DS, et al. RNA-protein interaction detection in living cells. Nat Methods. 2018;15(3):207–212.
- Kido K, Yamanaka S, Nakano S, et al. AirID, a novel proximity biotinylation enzyme, for analysis of protein-protein interactions. eLife. 2020;9:e54983.
- Liu Q, Zheng J, Sun W, et al. A proximity-tagging system to identify membrane protein–protein interactions. Nat Methods. 2018 [cited 2018 Sep 1];15(9):715–722.
- Hill ZB, Pollock SB, Zhuang M, et al. Direct proximity tagging of small molecule protein targets using an engineered NEDD8 ligase. J Am Chem Soc. 2016;138(40):13123–13126.
- Zhuang M, Guan S, Wang H, et al. Substrates of IAP ubiquitin ligases identified with a designed orthogonal E3 ligase, the NEDDylator. Mol Cell. 2013 Jan 24;49(2):273–282.
- Martell JD, Deerinck TJ, Sancak Y, et al. Engineered ascorbate peroxidase as a genetically encoded reporter for electron microscopy. Nat Biotechnol. 2012;30(11):1143–1148.
- Rhee H-W, Zou P, Udeshi ND, et al. Proteomic mapping of mitochondria in living cells via spatially restricted enzymatic tagging. Science (New York, NY). 2013;339(6125):1328–1331.
- Lam SS, Martell JD, Kamer KJ, et al. Directed evolution of APEX2 for electron microscopy and proximity labeling. Nat Methods. 2015;12(1):51–54.
- Jiang S, Kotani N, Ohnishi T, et al. A proteomics approach to the cell-surface interactome using the enzyme-mediated activation of radical sources reaction. Proteomics. 2012;12(1):54–62.
- Li X-W, Rees JS, Xue P, et al. New insights into the DT40 B cell receptor cluster using a proteomic proximity labeling assay. J Biol Chem. 2014;289(21):14434–14447.
- Bar DZ, Atkatsh K, Tavarez U, et al. Biotinylation by antibody recognition-a method for proximity labeling. Nat Methods. 2018;15(2):127–133.
- De Munter S, Görnemann J, Derua R, et al. Split-BioID: a proximity biotinylation assay for dimerization-dependent protein interactions. FEBS Lett. 2017;591(2):415–424.
- Schopp IM, Amaya Ramirez CC, Debeljak J, et al. Split-BioID a conditional proteomics approach to monitor the composition of spatiotemporally defined protein complexes. Nat Commun. 2017;8:15690.
- Martell JD, Yamagata M, Deerinck TJ, et al. A split horseradish peroxidase for the detection of intercellular protein-protein interactions and sensitive visualization of synapses. Nat Biotechnol. 2016 July;34(7):774–780.
- Xue M, Hou J, Wang L, et al. Optimizing the fragment complementation of APEX2 for detection of specific protein-protein interactions in live cells. Sci Rep. 2017;7(1):12039.
- Han Y, Branon TC, Martell JD, et al. Directed evolution of split APEX2 peroxidase. ACS Chem Biol. 2019 Apr 19;14(4):619–635.
- Cho KF, Branon TC, Rajeev S, et al. Split-TurboID enables contact-dependent proximity labeling in cells. Proc Natl Acad Sci U S A. 2020 June 2;117(22):12143–12154.
- Longatti A, Lamb CA, Razi M, et al. TBC1D14 regulates autophagosome formation via Rab11- and ULK1-positive recycling endosomes. J Cell Biol. 2012 May 28;197(5):659–675.
- Lamb CA, Nühlen S, Judith D, et al. TBC1D14 regulates autophagy via the TRAPP complex and ATG9 traffic. EMBO J. 2016;35(3):281–301.
- Leidal AM, Huang HH, Marsh T, et al. The LC3-conjugation machinery specifies the loading of RNA-binding proteins into extracellular vesicles. Nat Cell Biol. 2020 Feb;22(2):187–199.
- Sanwald JL, Poschmann G, Stuhler K, et al. The GABARAP co-secretome identified by APEX2-GABARAP proximity labelling of extracellular vesicles. Cells. 2020 June 16; 9(6).
- Heo J-M, Harper NJ, Paulo JA, et al. Integrated proteogenetic analysis reveals the landscape of a mitochondrial-autophagosome synapse during PARK2-dependent mitophagy. Sci Adv. 2019;5(11):eaay4624–eaay4624.
- Jia J, Abudu YP, Claude-Taupin A, et al. Galectins control mTOR in response to endomembrane damage. Mol Cell. 2018;70(1):120–135.e8.
- Jia J, Bissa B, Brecht L, et al. AMPK, a regulator of metabolism and autophagy, is activated by lysosomal damage via a novel galectin-directed ubiquitin signal transduction system. Mol Cell. 2020 [cited 2020 Mar 5];77(5):951–969.e9.
- Chauhan S, Kumar S, Jain A, et al. TRIMs and galectins globally cooperate and TRIM16 and galectin-3 co-direct autophagy in endomembrane damage homeostasis. Dev Cell. 2016 Oct 10;39(1):13–27.
- Jia J, Claude-Taupin A, Gu Y, et al. Galectin-3 coordinates a cellular system for lysosomal repair and removal. Dev Cell. 2020 [cited 2020 Jan 6];52(1):69–87.e8.
- Roux KJ, Kim DI, Burke B, et al. BioID: a screen for protein-protein interactions. Curr Protoc Protein Sci. 2018;91:19.23.1–19.23.15.
- Hara T, Takamura A, Kishi C, et al. FIP200, a ULK-interacting protein, is required for autophagosome formation in mammalian cells. J Cell Biol. 2008;181(3):497–510.
- Ganley IG, Lam DH, Wang J, et al. ULK1.ATG13.FIP200 complex mediates mTOR signaling and is essential for autophagy. J Biol Chem. 2009;284(18):12297–12305.
- Ewing RM, Chu P, Elisma F, et al. Large-scale mapping of human protein-protein interactions by mass spectrometry. Mol Syst Biol. 2007;3:89.
- Matsunaga K, Saitoh T, Tabata K, et al. Two Beclin 1-binding proteins, Atg14L and rubicon, reciprocally regulate autophagy at different stages. Nat Cell Biol. 2009 [cited 2009 Apr 1];11(4):385–396.
- Judith D, Jefferies HBJ, Boeing S, et al. ATG9A shapes the forming autophagosome through Arfaptin 2 and phosphatidylinositol 4-kinase IIIβ. J Cell Biol. 2019;218(5):1634–1652.
- Hosokawa N, Hara T, Kaizuka T, et al. Nutrient-dependent mTORC1 association with the ULK1-Atg13-FIP200 complex required for autophagy. Mol Biol Cell. 2009;20(7):1981–1991.
- Hosokawa N, Sasaki T, Iemura S-I, et al. Atg101, a novel mammalian autophagy protein interacting with Atg13. Autophagy. 2009 [cited 2009 Oct 1];5(7):973–979.
- Huttlin EL, Bruckner RJ, Paulo JA, et al. Architecture of the human interactome defines protein communities and disease networks. Nature. 2017 [cited 2017 May 1];545(7655):505–509.
- Cho KF, Branon TC, Rajeev S, et al. Split-TurboID enables contact-dependent proximity labeling in cells. Proc Nat Acad Sci. 2020;117(22):12143–12154.
- Kwak C, Shin S, Park J-S, et al. Contact-ID, a tool for profiling organelle contact sites, reveals regulatory proteins of mitochondrial-associated membrane formation. Proc Nat Acad Sci. 2020;117(22):12109–12120.
- Davies AK, Itzhak DN, Edgar JR, et al. AP-4 vesicles contribute to spatial control of autophagy via RUSC-dependent peripheral delivery of ATG9A. Nat Commun. 2018 [cited 2018 Sep 27];9(1):3958.
- Martin AP, Jacquemyn M, Lipecka J, et al. STK38 kinase acts as XPO1 gatekeeper regulating the nuclear export of autophagy proteins and other cargoes. EMBO Rep. 2019;20(11):e48150.
- An H, Ordureau A, Paulo JA, et al. TEX264 is an endoplasmic reticulum-resident ATG8-interacting protein critical for ER remodeling during nutrient stress. Mol Cell. 2019 [cited 2019 June 6];74(5):891–908.e10.