ABSTRACT
In solar photovoltaic (SPV) systems, optimizing output under various meteorological conditions relies on the Maximum Power Point (MPP) tracking controller. However, the presence of multiple peaks due to partial shading complicates this tracking process. While traditional and soft computing methods are commonly employed for MPP tracking, they face limitations such as the fixed step size in conventional methods and a lack of randomness in soft computing approaches once they reach a certain MPP. To address these challenges, a unique optimization technique known as the Adaptive Jellyfish Search (AJFS) method has been proposed. This method conducts both global and local searches simultaneously in a single step, enhancing the effectiveness of MPP tracking. To evaluate its performance and resilience, zero, weak, moderate, and strong shading patterns are employed in simulations, with comparisons made against traditional Jellyfish Search (JFS) and Particle Swarm Optimization (PSO) techniques. The newly suggested AJFS approach demonstrates significant improvements over traditional methods. It reduces convergence time by 49% and offers additional motivating features, including zero risk of failure, minimized oscillation in power parameters, and enhanced energy production by 1.5%. Moreover, it enables smooth tracking of the MPP, particularly in dynamically changing shading patterns. Overall, the AJFS method presents a promising solution for efficient MPP tracking in SPV systems, overcoming limitations of conventional approaches and demonstrating superior performance under various shading conditions. Its ability to simultaneously conduct global and local searches in a single step makes it well suited for optimizing energy production in real-world scenarios.
Supplementary material
Supplemental data for this article can be accessed online at https://doi.org/10.1080/15567036.2024.2387243
Acknowledgements
The authors acknowledge the Department of Science and Technology (DST), Govt. of India for the financial assistance provided under the DST SERB Project (File No. SRG/2021/002110) to carry out the present work. Amitesh Kumar would like to thank DST SERB for providing a Start-up Research Grant for this project to conduct research at NIT Patna. Balmukund Kumar would like to thank the Ministry of Education and NIT Patna for the research fellowship. The authors are thankful to NIT Patna for providing research facilities.
Disclosure statement
No potential conflict of interest was reported by the author(s).
Additional information
Notes on contributors
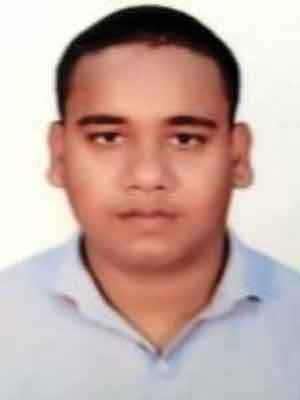
Balmukund Kumar
Balmukund Kumar (Non-member) received an M. Tech degree in Electrical engineering from National Institute of Technology, Durgapur, West Bengal, in 2020. He is currently a Research Scholar at the National Institute of Technology Patna Bihar. His research interests Nature Inspired Algorithms for Optimization applications in Renewable Energy System.
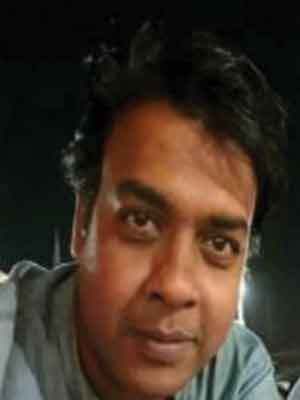
Amitesh Kumar
Amitesh Kumar (Non-member) has done his B.Tech in Electrical Engineering from Indian Institute of Technology, BHU. He did his Ph.D. in Electrical Engineering from Indian Institute of Technology, Indore. He did his Postdoc Research from University of Utah, USA. He has been a Research Fellow awardee from CSIR, Govt. of India. He is currently working as Assistant Professor in Electrical Engineering at National Institute of Technology, Patna.