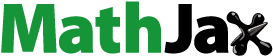
Abstract
The international literature indicates a wide interest in the distances public transport users walk to access their services. Urban and transport planners seek information on acceptable walking distances (AWD) in the provision of minimum levels of service coverage. This study uses a large database from Melbourne, Australia, to analyze trip length frequency distributions (TLFD) of walking access and egress to consider AWD in a multimodal public transportation system and to examine tolerable walking distances (TWD). AWD provides a guide to planners about stop/station locations for desirable minimum service coverage. TWD is a representative maximum walking distance for assessing the effectiveness of that service provision and can be used in conjunction with AWD. A statistical distribution function for walking distances can facilitate the use of regional values for AWD and TWD in transit service planning. The Burr Type XII distribution is shown to provide a good fit to the observed data. This provides a valuable tool for the analysis of percentile walking distances and suggests a general framework for the study of AWD and TWD in any city or region.
1. Introduction
Considering low carbon mobility and public health issues associated with travel activity in our cities, active transport - walking and cycling - is increasingly important in urban transport. This paper focuses on a specific aspect of active transport usage: access to scheduled public transport. Many journeys require access to transit services since the traveler must first board the transit service at a stop or station (access) and then alight at another stop/station near their destination (egress). In addition, transit trips often require transfers between lines or services, which are generally accomplished on foot.
A longstanding question in urban and transport planning concerns the reasonable distances that transit users may be expected to cover in accessing services. Many developed countries have adopted the 400 m (0.25 mile) and 800 m (0.5 mile) distances, respectively, as default planning goals for bus and rail transit (walking) catchment areas. Planners, transit agencies and researchers use these transit catchment area criteria to prescribe regulations or formulate policies. The transit catchment areas are believed to broadly represent how far people are willing to walk to take transit. Even though these distances may vary, depending on the location and travel purpose, they may provide good starting points in developing transit-related policies. The 400 m/800 m distances may be seen as representing acceptable walking distances and are part of a minimum level of service provision, but there is substantial evidence in the research literature (Agrawal et al., Citation2008; Alshalalfah & Shalaby, Citation2007; Daniels & Mulley, Citation2013; Ker & Ginn, Citation2003; O'Sullivan & Morrall, Citation1996) that many people are prepared to walk further than those distances. Thus, the concept of a tolerable walking distance to transit arises. The aim of this paper is to use actual walking behavior to examine how acceptable and tolerable distances may compare and may be used as a pair of parameters in transit service planning.
Transport planners systematically collect data on people’s travel behavior as a core resource for the planning, provision, design and operation of transport infrastructure and services in urban areas. A longstanding data collection method is through large-scale surveys called Household Travel Surveys (HTS). Modern HTS methods include the collection of survey respondents’ activities, as well as their travel movements. It is known that this method produces better and more accurate data on travel movements. It is also recognized that interactions between members of a household strongly influence their behavior, which is why the household is used as a basis for analysis. With the use of new technologies such as Global Positioning Systems (GPS) and smart travel cards, activity-travel diaries can be used to collect travel and activity data for individual household members. Data on travel and associated activities are collected for at least one day, although some surveys collect data for longer periods. Travel days in most modern surveys cover all days of the week, including weekends. The HTS data can thus be used to obtain a comprehensive picture of the travel movements and behavior of a sampled population. Data relating to the usage of active transport modes can be extracted from HTS databases, e.g. Durand et al. (Citation2016) and Fairnie et al. (Citation2016). This was the approach adopted in this study to examine the active transport participation of a metropolitan population, focussing on access to public transport services. This participation can be represented by statistical distributions (trip length frequency distributions, TLFD), and this paper aims to use these distributions in consideration of acceptable and tolerable walking distances. It does so by considering the distances walked in access/egress to/from public transport services and how these may be described in a generalized fashion.
2. Literature review
Active transport is an integral part of sustainable transport, as well as offering potential health and social benefits (Van Soest et al., Citation2020). In other words, health benefits can be achieved, among other things, through a shift away from private motorized transport to more active transport. In addition to reducing the need for other modes of transportation, active transport promotes more human activity on the streets, which can enhance community safety and wellbeing. Low-carbon urban design and planning should result in opportunities to increase active transport modes at both the local precinct and the broader urban regional scale. Policies that aim to increase the proportion of trips that are taken by active modes of travel, such as public transport, bicycling and walking, will likely increase a population’s activity levels in a way that can be sustained over the long run (Grisé et al., Citation2019; Philp & Taylor, Citation2017). Walking is the primary access mode for trips from home to public transport, and walking distance has a significant impact on public transport use (Daniels & Mulley, Citation2013).
Wasfi et al. (Citation2013) studied daily walking activity in Montreal. In addition to confirming public transport’s role in support of active transport, they also found that suburban rail users could meet a reasonable portion of recommended health activity levels by commuting. Morency et al. (Citation2011) used HTS data from Montreal to study walking distances to public transport and assess the actual level of physical activity undertaken. The Montreal travel data indicated that a public transport trip involved 1,250 steps on average (covering walking access to, egress from, and transfer during the trip). Thus, a return trip corresponded to 2,500 steps, or 25% of the daily recommended physical activity (10,000 steps). According to Morency et al. (Citation2011), active transport studies should also take socio-demographic factors into account. The ability to use these modes and the benefits accrued may be affected by physical and mental capabilities. Cole et al. (Citation2006) found that gender and age played a significant role in walking activity levels. These issues were explored by Taylor and Thompson (Citation2019).
Access to public transport is a key consideration in urban planning and design. This is especially true for low-carbon development when using public transport instead of private vehicles becomes a major concern. Certain ‘rule of thumb’ metrics have been recognized in urban and transport planning as indicators of a minimum level of access to public transport when walking from home to the nearest transit service (the acceptable walking distance). In Australia, as in many other developed countries, these distances are commonly accepted as 400 m to a bus stop and 800 m to a train station. American researchers reported similar results (e.g. Hoback et al., Citation2008). Although the origins of these values are unclear, they might represent walking times of five and ten minutes, respectively. In addition, the distances are usually taken to be in a straight line, so actual distances traversed may be longer, depending on the network layout. Ker and Ginn (Citation2003) concluded that people walked significantly more than 800 m to their train station in Perth, Australia, based on observations made at five suburban stations. Durand et al. (Citation2016) reported similar findings for California. Chia et al. (Citation2016) argued that socioeconomic status also affected walking time. Land-use planners have used these metrics widely in the design of walkable cities and transit-oriented developments (TODs) (Daniels & Mulley Citation2013; Kaszczyszyn & Sypion-Dutkowska Citation2019; Noh et al. Citation2021; Pueboobpaphan et al. Citation2022).
O'Sullivan and Morrall (Citation1996) studied walking access to bus and light rail transit (LRT) services in Calgary, Canada. They found that people walked further to reach an LRT station than to a bus stop. The average walking distance to suburban stations was 649 m (75th percentile 840 m), while at CBD stations, the average distance was 326 m with 75th percentile 419 m. Dill (Citation2003) in San Francisco, Crowley et al. (Citation2009) in Toronto, Hess (Citation2012) in New York, and García-Palomares et al., (Citation2013) in Madrid found similar results concerning station location. Stations located further from the CBD have larger pedestrian catchments. García-Palomares et al. (Citation2013) also found that young adults, immigrants, and transit captives were willing to walk longer distances and were less sensitive to the effect of distance when accessing public transport. Hoback et al. (Citation2008) reported average walking distances of 1.3 km total (i.e. access plus egress) per round trip for public transport riders in Detroit, while El-Geneidy et al. (Citation2014) reported 85th percentile walking distances of 524 m to access bus transport and 1.259 km for rail in Montreal. Rastogi and Rao (Citation2003) found a mean walking distance of 910 m and an 85th percentile distance of 1.25 km in a study of suburban rail access in Mumbai. According to BTS (Citation2014), 79% of walking access trips to Sydney rail stations were one kilometer or less, and a further 17% were between one and two kms. These distances are indicative of the tolerable distances that some travelers will undertake to access transit services.
Burke and Brown (Citation2007) used HTS data to analyze walking trips in Brisbane. They produced empirical statistical distributions of walking distances for home-based and non-home-based travel. Single-mode walk trips (i.e. trips made entirely on foot) tended to be longer than walk trip segments (e.g. walking trips to access public transport), although there were twice as many walk-trip segments accessing public transport as there were single-mode walk trips. For home-based single-mode walk trips, the median trip distance was 780 m, with the 85th percentile at 1.45 km, while the median and 85th percentile walk distances to access public transport from home were 600 m and 1.30 km, respectively. The median and 85th percentile egress distances were 470 m and 1.09 km, respectively.
Van Soest et al. (Citation2020) provided an international overview and a synthesis of the factors likely to affect access walking distances. They considered a wide range of factors that have been suggested as influencing walking behavior in transit usage, which they classified as socioeconomic, transit-related, environmental and journey-related factors. Different factors have been proposed by the different research studies cited, with some contradictory findings. Indeed, Van Soest et al. (Citation2020) concluded that there were few clearly established factors and that social attitudes and culture played underlying roles, interacting with many of the suggested factors. Other factors could be explained in terms of physical phenomena: for example, train users walked farther than bus users because train stations tend to be further apart than bus stops (see Vuchic (Citation2007), also Sarker et al. (Citation2020) and Tennøy et al. (Citation2022)). Station/stop function was also identified as a factor, in that terminals tended to attract longer distance walks than mid-line boarding points. Higher density of stops (i.e. stops closer together) led to shorter walking distances. Trips to and from work tended to show longer walking distances than those for other trip purposes.
A useful descriptor of travel behavior in an urban area is the trip length frequency distribution (TLFD). This statistical distribution shows the relative distribution of travel by travel cost, time or distance for a particular purpose or by a given socioeconomic group in a study region. The distribution indicates the range of travel costs incurred and the intensity of travel activity at each travel cost. The TLFD may be represented either by a histogram or by a cumulative distribution function (CDF) plot, where the latter indicates the probability of trip lengths equal to or less than each value of travel cost. The CDF is useful for comparing TLFD for different criteria (e.g. trip purpose, travel mode, or socioeconomic groups). It is possible to develop sets of TLFD for different criteria, and when combined with information on the choice of travel modes, develop a broad picture of travel behavior in a region. Regarding active travel, Rastogi and Rao (Citation2003) developed TLFD for walking and cycling access to suburban rail services in Mumbai, while Burke and Brown (Citation2007) reported TFLD for walking in Brisbane. Given the strong skew to the upper tail seen in their TLFD, Burke and Brown suggested the possible use of the Gamma distribution to describe their data, although they did not fit any theoretical distributions to the data.
This literature review has made frequent reference to the term acceptable walking distance (AWD), with a range of perspectives on what this distance means and no clear definition. Some researchers have identified AWD with the supposed ‘rule of thumb’ of 400 m/800 m for access distance to the nearest bus stop/train station. Others have seen AWD merely as the distance(s) people are prepared to walk to access public transport services. This paper proposes some more formality in the use of the term. Here, AWD is to be seen as a planning criterion for the provision of the minimum level of access to urban public transport services (the 400 m/800 m rule could then be used as AWD). In a transit service planning context AWD is then a walking distance representing an aspirational planning parameter to indicate good access to transit services. On the other hand, the empirical results cited above—and in the case study section of this paper, see below—indicate that many travelers are prepared to walk much further than this AWD, and so we propose the consideration of a second access walking parameter, the tolerable walking distance (TWD). TWD for transit service planning is proposed as a representative maximum walking distance to be used by planners in assessing the effectiveness of transit service provision in an area. We note that the concept of tolerable walking distances has been applied in other areas of research on pedestrian behavior (e.g. students walking to school) as ‘critical walking distances’ in terms of the extremities to which pedestrian trips may be made (i.e. the far upper tail of the distribution of walking distances), e.g. Ermagun et al. (Citation2016). In this case, tolerable walking distance is used as an indicator of potential walking distances that pedestrians may undertake, whether by desire or necessity. That is not the intention nor use in transit service planning of the TWD suggested in this paper.
Given the range of factors suggested in the literature as influencing walking distance, further consideration is needed on how to select AWD and TWD for transit service planning in a given city or region. Besides personal factors such as age, gender and socioeconomic status, the factors include city size, transit mode, climate, environment, walkability and culture. To cover these factors, Pueboobpaphan et al. (Citation2022), Tennøy et al. (Citation2022), and (implicitly) Tsioulianos et al. (Citation2020) suggested the use of percentile values selected from the TLFD for walking distances as appropriate metrics for AWD: Pueboobpaphan et al. suggested use of 65th-70th percentiles while Tennøy et al. suggested the 75th percentileFootnote1. The analysis presented later in this paper explores this percentile approach, using percentile values determined from a theoretical statistical distribution fitted to access and egress walking distances.
3. Melbourne case study
The analysis in this paper uses the Victorian Integrated Survey of Travel and Activity (VISTA) HTS database (DEJTR, Citation2016) for Melbourne to describe and analyze access to public transport using active travel modes. Melbourne is the capital of the State of Victoria and is now Australia’s largest city, having displaced Sydney in 2022. It has a highly developed multi-modal public transportation system dating from the late nineteenth century. The major public modes are suburban heavy rail, light rail (trams) and buses. The icon of the Melbourne public transport system is the extensive tram network that serves the CBD and surrounding suburbs, mostly within 10 km of the city center. shows the Melbourne metropolitan region and the major public transportation networks covering it, including the suburban rail network, the tram network and the major bus routes.
The VISTA database is a major HTS conducted in Melbourne and regional centers in Victoria. It contains comprehensive data on the daily travel and activity patterns of households and persons, including data on travel for all purposes by individuals from all socioeconomic groups, as well as data on the use of active transport modes for their own purposes and as part of trip chains (i.e. travel by all modes that can include trip segments of walking and cycling). Thus, the database contains data on travel by active modes and covers all modes of travel for all purposes over all days of the week by all inhabitants of the study region. The VISTA database comprises the following five tables:
Household table holds household variables such as household size and structure, number of vehicles and bicycles in the household, household income, dwelling type and dwelling location
Person table includes personal variables such as age, gender, occupation/activity type and licensed driver status for each individual in the household
Vehicle table contains information on each vehicle available to the household, including vehicle type, make, year of manufacture, fuel/energy type, engine type and capacity
Stops table includes data on all ‘stops’ or trip segments (sometimes called ‘unlinked trips’) undertaken by each individual in the survey. A trip segment is for travel between successive decision points (nodes), where the traveler either changes activity at the decision point (e.g. arrives at work, school or shopping center) or changes mode of travel (e.g. walks to a bus stop (trip segment i) and then catches a bus (trip segment i + 1) to the next decision point). Data recorded for each trip segment include origin (start point) and destination (endpoint), the purpose of travel on the trip segment, the purpose of activity at the endpoint, mode of travel used, vehicle occupancy (number of people in a private vehicle), departure time, arrival time, travel time, travel distance, time of day and day of week
Trips table provides the combinations of trip segments into ‘linked trips’, giving information on travel undertaken between activity points by an individual. A journey by public transport might involve at least three trip segments: e.g. a walk trip segment from home to the bus stop, a trip segment by bus, and a final trip segment on foot to the destination.
Thus, the Stops table includes all travel activity and how, where, why, when and by which means it was undertaken. The table includes all walking and cycling activities undertaken by survey respondents and provides the most comprehensive overview of active transport usage. The Trips table provides the context for the travel and identifies active travel trip segments used as access and egress in a public transport journey.
VISTA data for residents of the metropolitan Melbourne region was used in the analysis. This comprised 11,061 households containing a total of 28,705 individuals. A set of weighting factors for households, persons, vehicles, stops, and linked trips enabled the sample data to be scaled to reflect the full population and its aggregate behavior. The VISTA database thus represents a population of about 3,830,000 people in the Melbourne metropolitan region, living in 1,365,000 households (2.80 persons/hhld), owning 2,315,000 motor vehicles (including 1,825,000 cars) and 1,840,000 bicycles (household ownership rates 1.70 veh/hhld, 1.34 cars/hhld, 1.35 bicycles/hhld).
4. Data analysis
The analysis focuses on the use of walking as access to (and egress from) public transport, i.e. it considered all walking ’stops’ and the extracted walking components from all linked trips that used public transport. Population-based TLFD by distance and time were derived for these cases. An overall picture of travel in Melbourne, with a focus on active travel, was presented by Taylor and Thompson (Citation2019). The results of their analysis showed the dominance of the private car, particularly the ‘vehicle driver’ mode. This mode accounted for nearly half of the trip numbers, over 60% of the travel distance, and just over half of the travel time. Trip numbers indicate travel decisions, travel distance (as person-km of travel, PKT) indicates travel effort, and travel time (as person-hours of travel, PHT) indicates travel exposure. The number of trips taken by walking was the second most popular mode (22%), just ahead of trips taken by vehicle passengers (21%). The latter was, nevertheless, the second most used mode in terms of distance and time. Only 2.0% of travel distance was covered by walking, but 12.7% of travel time was spent walking. Distances traveled on foot would be expected to be much less than those for wheeled modes, but walking was the third highest mode in terms of travel time. Public transport, taken as the combination of train, tram and bus, accounted for 8.2% of trip numbers, 10.7% of travel distance and 10.6% of travel time. The train was the predominant mode of public transportation in the region, especially for long-distance travel. Bicycling accounted for 1.5% of trips, 1.0% of distance traveled, and 2.1% of travel time. See Taylor and Thompson (Citation2019) for further discussion of these results.
4.1. Trip length frequency distributions
As previously indicated, trip length frequency distributions (TLFDs) indicate a study region’s range of travel activity. TLFDs were derived for the public transportation modes of bus, train and tram, along with distributions for the active modes. For the study of access to public transport, specific distributions of walking access and egress distances were extracted for journeys by public transport.
VISTA’s network travel distances are calculated from the information provided in travel-activity diaries by survey respondents about their origins and destinations, based on computed mode-specific network distances to the nearest 10 m. This paper focuses on the TLFDs for travel distances and considers both the distances traveled on the transit vehicles and the distances traveled on foot in access to and egress from those vehicles.
A notable feature of the observed TLFDs is that the distributions are skewed to the upper tail. The asymmetric nature of the underlying distributions, with this skew, means that the average value is much higher than the median value. The median may then be a better indicator of ‘normal’ travel behavior. Thus, there is some proportion of transit users who are prepared to walk considerable distances as part of their journeys, which is important when considering acceptable and tolerable walking distances.
4.2. TLFD for public transport modes
Given that public transport is considered complementary to the active modes of walking and cycling, and in some cases, as an active transport mode in its own right, the present study looked at the TFLD for the three transit modes. Access to public transport, especially on foot but perhaps also by bicycleFootnote2, is recognized as an important component of, and factor influencing, the use of transit. Additionally, it provides people with an opportunity to engage in healthy physical activity on a daily basis. Further, it is helpful to understand how public modes are used in terms of distance traveled and travel time spent. shows the TLFDs for distances traveled by each of the transit modes. Note that there is no inclusion of access or egress components in these TLFDs, as they represent those parts of an individual’s journey spent onboard a public transport service vehicle. The journey components, access and egress, where made on foot, are examined in more detail below.
shows that tram trips are relatively short, whereas train trips are longer– reflecting the topology of Melbourne’s public transportation network (see ). Rail lines radiate out from the city center to terminals at the edges of the metropolitan area. The tram network primarily serves the CBD and inner suburbs. Buses serve the middle and outer suburbs. All of the distributions in are strongly skewed to the right.
4.3. Access to public transport
Public transport is an important consideration in urban planning and design, especially for low-carbon urban developments where public transport is preferable to private cars. VISTA data analysis provides a baseline view of how far people are willing to walk to access transit. Due to the small number of cycling access trip segments in VISTA, it was not possible to derive cycling access TLFDs. The VISTA Trips table was used to extract all trips involving any or all segments of trips involving active transport access to, or egress from, a public transport mode (bus, train, or tram). The active transport segments of those trips were then analyzed. shows the combined set of observed access and egress walking distance distributions for travel by the public transport modes of bus, train and tram in Melbourne.
Figure 3. Cumulative distributions (TFLD) for access and egress walking distances in public transport trips in Melbourne.
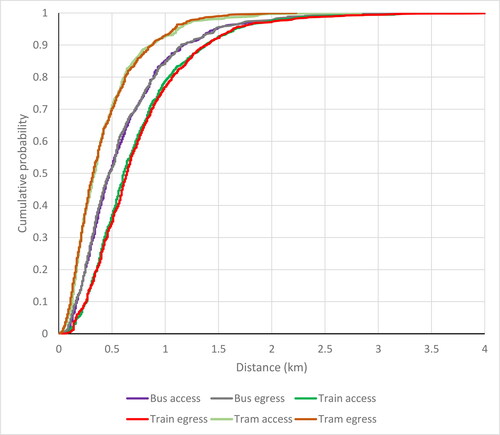
There are three main observations from . As a first observation, the distributions are all skewed to the upper tail. Secondly, the distributions of access and egress distances for each mode are very similar, almost indistinguishable. Finally, the different modes have quite different distributions in terms of walking range. The walking distance to the train is generally longer than that to the bus, which in turn is longer than that to the tram. According to the literature review and based on the way that the service infrastructure is designed (Vuchic, Citation2007), longer walking distances are expected for train services, for railway stations tend to be further apart than bus and tram stops. shows the summary statistics for the distributions. The mean walking distances are (1) bus access 0.61 km, egress 0.61 km, (2) train access 0.70 km, egress 0.72 km, and (3) tram access 0.43 km, egress 0.42 km, while the median values are: (1) bus access 0.48 km, egress 0.47 km, (2) train access 0.59 km, egress 0.62 km, and (3) tram access 0.34 km, egress 0.33 km. The corresponding 90th percentile distances are as follows: (1) bus access 1.17 km, egress 1.16 km, (2) train access 1.30 km, egress 1.30 km, and (3) tram access 0.86 km, egress 0.87 km. These results are comparable with those found by Burke and Brown (Citation2007) for Brisbane, although the Brisbane access distances are longer. The Canadian studies cited previously (El-Geneidy et al. Citation2014; O'Sullivan and Morrall Citation1996) provided distances slightly shorter than the Australian results, with the exception of the 85th percentile access distance to rail in Montreal (1.26 km). Walking access distances for trains always exceed those for trams and buses, with tram access distances always being shorter than bus access distances (). The results for bus and tram reflect the available service coverage in the areas served by these two modes. The tram network provides dense coverage, while the bus network, predominant in the outer suburbs, is much sparser. Note the percentages of trips less than the ‘rule of thumb’ distances of 400 m and 800 m: only 40% of access walks to buses were less than 400 m, but about 60% of walks to tram were less than this value. About two-thirds of access and egress walks for trains were under 800 m. The walking distance statistics in are ‘revealed preference’ data, representing the travel behavior required of travelers using the respective transit modes. They thus reflect tolerable walking distances but not necessarily acceptable distances from a planning perspective.
Table 1. Summary statistics for observed distributions of access and egress walking distances for Melbourne public transport trips.
5. Distribution fitting for the TFLDs
The empirical TLFDs are interesting in their own right, but there is value in considering a generalization of the TFLDs by fitting a theoretical statistical distribution to the data, which may then provide an approach for comparisons between cities when considering acceptable and tolerable walking distances. Given the strong skew in all of the distributions in , distributions accommodating skewed data are required. Different candidate distributions exist, such as the log-normal and Gamma distributions, but these distributions may pose difficulties with the mathematical representation of their cumulative density functions (CDF), given the need to consider percentile values for the TFLDs.
The Burr Type XII distribution (Burr, Citation1942)—henceforth termed the Burr distribution—provides a useful statistical distribution for a random variate x from a skewed distribution. This distribution is finding increasing applications in transportation studies, e.g. Guessous et al. (Citation2014), Taylor (Citation2017), Low et al. (Citation2023) and Polosin et al. (Citation2023). The probability density function (pdf) f(x, θ, c, k) of the 3-parameter version of the Burr distribution is (Tadikamalla, Citation1980):
where random variable x ≥ 0, θ > 0 is a scaling parameter and c > 0 and k > 0 are shape parameters. The CDF F(x, θ, c, k) is given by
(1)
(1)
The Burr distribution has a flexible shape and is well-behaved algebraically. The distribution is easy to use and eminently suited to calculating percentile values, which is a basic requirement for consideration of AWD and TWD. Indeed, an exact analytic function for its inverse CDF (F−1(P)) exists. Given the CDF defined by EquationEquation (1)(1)
(1) , we can solve for a given value of F(x, θ, c, k) for percentile P (0 ≤ p < 1) i.e.
from which
(2)
(2)
where xp is the Pth percentile value of x. Exact values of specific percentiles are thus defined in terms of the parameters θ, c and k. For example, the median is
and the 90th percentile value is
Indeed the Burr distribution is ideally suited to studies involving percentiles as the descriptors of a random variate x. Best-fit parameters for the Burr distribution can be found using maximum likelihood (Taylor, Citation2017). The resulting parameters for each of the six distributions shown in are given in . and compare the fitted distributions with the observed data for access () and egress () and show both probability density functions and cumulative distribution functions for each public transport mode. also indicates the results of statistical goodness-of-fit tests for each data set. Three tests were used: Kolmogorov-Smirnov, Chi-square and Anderson-Darling. The first of these is the weakest test; the last is the most stringent. The test results indicate that the fitted Burr distributions were good fits for the bus and train access and egress, but the results were less clear for tram access and egress due to the particular ‘peakiness’ (lepto-kurtosis) of these two distributions. The upper tails of all the fitted Burr distributions were very close to those for the observed data, as seen by visual inspection. This is important for considerations of percentile values for the upper tails. shows all of the six fitted distributions together in both CDF () and pdf () formats. The similarity of the access and egress distributions is apparent for each mode. The lepto-kurtotic form of the tram access and egress distributions, compared to those for the other modes, is also apparent. may be compared directly to , which shows the CDFs for the observed data for the three modes.
Figure 4. Comparison of fitted Burr distributions and observed distributions for access walking distances by public transport mode: probability density (pdf) and cumulative distribution (CDF) curves.
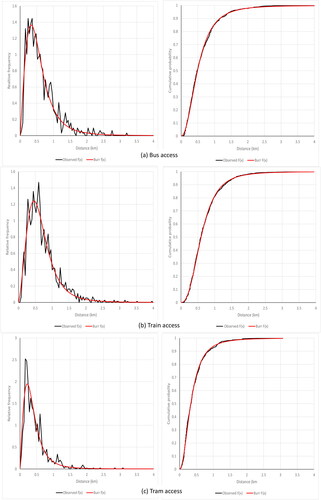
Figure 5. Comparison of fitted Burr distributions and observed distributions for egress walking distances by public transport mode: probability density (pdf) and cumulative distribution (CDF) curves.
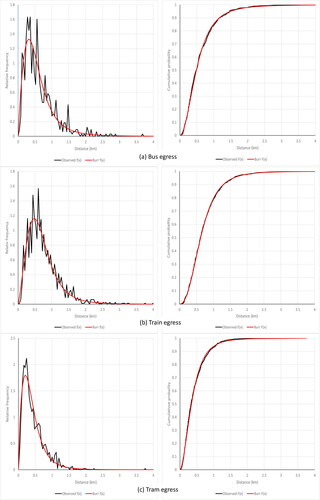
Figure 6. Fitted Burr distributions for walking access and egress distances for public transport trips in Melbourne – (a) cumulative distribution functions, (b) probability density functions.
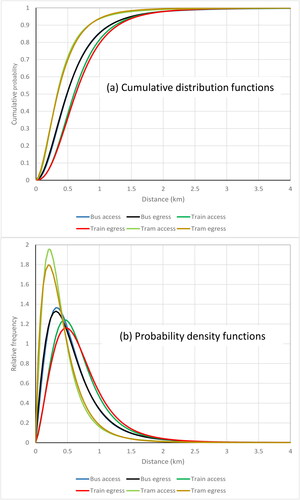
Table 2. Burr distribution best fit parameters for Melbourne access and egress walking distances.
6. Percentiles and walking distances
An analysis of walking distances, percentiles and other statistics can be undertaken given the fitted Burr distributions. A first point of interest is the percentile values corresponding to the ‘rule of thumb’ 400 m (bus and tram) and 800 m (train) walking distances. For the tram, about 60% (60.0% access, 58.5% egress) of users walked up to 400 m, while bus users tended to walk further. Only about 39% of bus users walked 400 m or less (38.7% access, 39.6% egress). Just over two-thirds of train users walked up to 800 m (69.4% access, 66.5% egress). In terms of the average walking distances for each mode:
62.7% of bus users walked up to the average access/egress distance of 610 m
60.8% of train users walked up to the average access distance of 700 m, and 59.6% walked up to the average egress distance of 720 m
63.9% of tram users walked up to the average access distance of 430 m, with 61.1% walking up to the average egress distance of 420 m.
These percentages reflect the skew in the walking distributions, with more than half of the travelers walking less than the average distance. The median walking distances were bus access and egress 480 m, train access 590 m, train egress 620 m, tram access 330 m and tram egress 340 m. As noted previously, tram users tended to walk shorter distances than those of the other two modes. These results also need to be put into perspective. Using data from PTV (Citation2012), the following mean spacings between bus and tram stops and train stations in Melbourne are:
bus stops 345 m
train stations 1844 m (with a range of 418 m - 10.45 km)
tram stops 285 m (range 186–392 m).
These figures reflect the geographic form of the Melbourne public transport system (), with the tram network located in and around the CBD and corresponding higher population density. The bus network predominates in the middle and outer suburbs, with generally lower population density. The rail network is radial, flowing out from the city center and with stations tending to be further apart in the outer suburbs.
Some of the recent international studies cited above have suggested the use of percentile values in ascertaining acceptable walking distances as a reflection of city size and local culture. For example, Pueboobpaphan et al. (Citation2022) suggested the choice of 65–70th percentile values for AWD, while Tennøy et al. (Citation2022) suggested the 75th percentile. shows the walking distances for these percentile values in Melbourne, calculated from the fitted Burr distributions. It also shows the 50th, 60th and 67th percentile values, along with the 80th, 85th and 90th percentiles. The table suggests that the differences in distances between percentiles increase substantially from the 75th percentile onwards, reflecting the long tails in the walking distance distributions. The 67th percentile values, representing the distances walked by two of three transit users, are 640 m access and egress for bus, 770 m access and 810 m egress for train, and 460 m access and 470 m egress for tram. The train distances are reasonably close to the 800 m value previously cited as a planning parameter for this mode, while the bus distance is well above the 400 m value. The tram distances are slightly above this figure. Considering the 60th percentile, representing the distance walked by three of five users, the distances are 580 m access and egress for bus, 690 m access and 720 m egress for train, and 400 m access, 450 m egress for tram. If planners were to seek acceptable walking distances based on percentiles and constituting amenable walking distances for a good part of the population, then this evidence would suggest that a percentile in the range of 60–70% might be suitable, and perhaps the 60th percentile values might be a good choice.
Table 3. Percentile access and egress walking distances from fitted Burr distributions for Melbourne public transport trips.
On the other hand, a tolerable walking distance, as defined in this paper, would be a representative maximum distance planners should be prepared to accept for walking access (and would not want to exceed). Considering the percentile distances in , the 80th percentile values might be good candidates for TWD. This table shows the percentile distances for access and egress walking for each transport mode, covering the percentiles (at five per cent intervals) from the median onwards (i.e. the upper tail of the distribution). It also shows the incremental differences in distances between successive percentiles, indicating that these differences tend to increase rapidly from the 80th percentile upwards. This is also seen in the plots of the CDFs for the distributions, shown in . The 80th percentiles identify the distances that one in five users are prepared to exceed in walking for transit service by each mode. These distances are as follows:
for bus, 800 m access and 870 m egress
for train, 970 m access and 1,010 m egress
for tram, 600 m access and 620 m egress.
7. Discussion and conclusions
As demonstrated by the Van Soest et al. (Citation2020) review, there is widespread research interest in walking access to public transport services. A wide range of factors influencing walking distances has been postulated, but there are a few factors, especially related to socioeconomic and travel behavior, that seem to provide a common thread. Indeed, a review of the various research studies indicates that there are many conflicting findings, suggesting that local culture and social norms play an important role. The factors that do appear to be common across the studies are physical factors, such as transit service mode, city size, climate and environment, and perhaps urban walkability. Urban and transport planners have long sought criteria and parameters to employ in transit service planning and design, and many have used the 400 m/800m walking distance parameters for bus/train stops and stations as a ‘rule of thumb’ in the provision of a minimum level of service, with no clear insight as to the source of these distances. In addition, those studies that have observed walking distance by transit users have consistently found that substantial proportions of users walk distances well in excess of these guideline values.
In recent times, this has led to the consideration of the acceptable walking distance (AWD) concept for access to transit services, although there is not much consistency in what AWD actually is. For some researchers, AWD is linked to the 400 m/800 m distance to the nearest bus stop/train station. Others have seen AWD more in terms of the observed distances people are prepared to walk to access transport services. Researchers such as Pueboobpaphan et al. (Citation2022) and Tennøy et al. (Citation2022) have suggested the use of percentile values from walking distance distributions in selecting an AWD.
This paper proposes a more formal definition of AWD to be taken as a planning criterion for the provision of a desirable minimum level of access to urban public transport services. Given that many users are prepared to walk much further than this AWD, a second access walking parameter, the tolerable walking distance (TWD), is also proposed to be used in conjunction with AWD. TWD is taken as a representative maximum walking distance to be used by planners in assessing the effectiveness of transit service provision in an area and is based on observed walking distances. It should always exceed AWD. A useful way to assess service effectiveness using these two planning parameters would be to construct the catchments around a stop/station corresponding to the values of AWD and TWD. The two parameters could be used to identify core (AWD) and marginal (TWD) catchment areas around stops and stations for transit, and thus indicate the proportions of a population with good or fair access to specific transit services.
Formulating a statistical distribution (a trip length frequency distribution) to describe walking distances is a necessary step in selecting suitable values for AWD and TWD. This study used a large sample of walking access and egress distances in a multimodal public transport system to find and fit suitable distributions to observed data. This data set included walking distances for users of three modes with very different operating characteristics: bus, train and tram. The Burr (Type XII) distribution was found to provide a good fit for these data. It was able to model the shape of the distribution, including the observed skew to the upper tail, and its mathematical form facilitates the computation of percentile distance values. The data analysis undertaken suggested that the 60th percentile walking distance could be used to set AWD and the 80th percentile for TWD. The former represents the distances walked by up to three in five people, the latter the distances for up to four in five people. For the Melbourne metropolitan area, suitable values for AWD would be bus 580 m, train 700 m, and tram 400 m, rounding off from the data in . Corresponding values for TWD are then bus 860 m, train 1.00 km, and tram 600 m.
The analysis described above considers walking distance parameters (AWD and TWD) based on the observed behavior (revealed preferences) of travelers in a given community seeking to access transit services of a given, established configuration (i.e. including the specific stop and station locations and spacings provided for established services). The examination of observed walking distances provides planners with empirical support for the previously hypothesized ‘rules of thumb’ suggestions of 400 m/800 m walking distances in providing a minimum standard of access for transit users. A deeper question for planners may also be raised, which is: what suitable access walking distances would make transit ridership more attractive and thus assist in achieving lower carbon footprints for urban transport. In keeping with the terminology adopted in this paper, such a walking distance might be termed a ‘reasonable walking distance’ (RWD). The determination of RWD is perhaps a question for stated preference studies allied with research on the physical comfort and ease of accomplishment of walking for existing and potential transit users. This requires the study of the physical and physiological characteristics of the users and of the walkability of an urban area. It would also relate to the acceptable levels of transit service provision, including service frequency, route travel times (as affected by stop density along a route), and reliability of those travel times (and hence adherence to published schedules). On this basis, planners could design transit services to maximize usage in a given community. This is an area for future multidisciplinary research. The following conclusions may then be drawn:
Walking access to and egress from public transport services is an important consideration in transit service planning and the design of service catchment areas
Planners require guidelines about the choice of suitable walking distances for this consideration, and these distances need to be related to the walking behaviors of a city or regional population. Choice of an AWD as a parameter is therefore required, with planners also being cognizant of the expectation that some travelers will be prepared to walk distances greater than the AWD, which by design should be less than a second distance parameter, the tolerable walking distance
Realistic selection of parametric walking distances should be based on evidence of walking behavior in a given city or region. The observed distributions of walking behavior are skewed to the upper tail, and so percentile values from these distributions should be used in parameter selection
A suitable statistical distribution for walking distances is the Burr distribution, which has been shown to provide a good fit to observed data and which can be readily used to ascertain percentile values
The international research suggests percentiles in the range of 60–75% for AWD and perhaps 80–90% for TWD. This study proposes the use of the 60th percentile value in the selection of AWD and the 80th percentile value in the selection of TWD
The planning parameters AWD and TWD can be used to establish core and marginal catchments around transit stops and stations, to indicate the proportions of a local population with good (AWD) and fair (TWD) access to specific transit services
Further research is required to establish optimal walking distances for use in planning for increased levels of active transport featuring enhanced transit usage
Another area for further research would be on the potential effects of the seasons (winter, summer, rainy, dry, etc) on walking distances.
Acknowledgements
We gratefully acknowledge the support of the Cooperative Research Centre for Low Carbon Living (www.lowcarbonlivingcrc.com.au), which funded the research project on which this paper is based. In addition, the authors wish to thank the Victorian Department of Transport and Planning for its kind support of this study through the provision of the VISTA database and advice about its contents and use. See https://dtp.vic.gov.au/about/data-and-research/vista. The analysis, its results and their interpretations are, however, strictly those of the authors.
Disclosure statement
No potential conflict of interest was reported by the author(s).
Notes
1 The 75th percentile would represent the distances walked by up to three in four people in a given community. Selecting a percentile representing the distances walked by up to two in three people (i.e. 66.7%) would fit within the range suggested by Pueboobpaphan et al.
2 Analysis of the VISTA data found very small numbers of public transport access trips by bicycle, with statistical analysis infeasible because of the tiny recorded sample sizes (of the order sample size n ≤ 10 for each of the public transport modes).
References
- Agrawal, A., Schlossberg, M., & Irvin, K. (2008). How far, by which route and why? A spatial analysis of pedestrian preference. Journal of Urban Design, 13(1), 81–98. https://doi.org/10.1080/13574800701804074
- Alshalalfah, B. W., & Shalaby, A. S. (2007). Case study: Relationship of walk access distance to transit with service, travel, and personal characteristics. Journal of Urban Planning and Development, 133(2), 114–118. https://doi.org/10.1061/(ASCE)0733-9488(2007)133:2(114)
- BTS. (2014). Train statistics 2014: Everything you need to know about Sydney trains and NSW TrainLink (9th ed.). NSW Bureau of Transport Statistics.
- Burke, M., & Brown, A. L. (2007). Distances people walk for transport. Road and Transport Research, 16(3), 16–28.
- Burr, I. W. (1942). Cumulative frequency functions. The Annals of Mathematical Statistics, 13(2), 215–232. https://doi.org/10.1214/aoms/1177731607
- Chia, J., Lee, J., & Kamruzzaman, M. (2016). Walking to public transit: Exploring variations by socioeconomic status. International Journal of Sustainable Transportation, 10(9), 805–814. https://doi.org/10.1080/15568318.2016.1156792
- Cole, C., Leslie, E., Bauman, A., Donald, M., & Owen, N. (2006). Socio-demographic variations in walking for transport and for recreation or exercise among adult Australians. Journal of Physical Activity & Health, 3(2), 164–178. https://doi.org/10.1123/jpah.3.2.164
- Crowley, D. F., Shalaby, A. S., & Zarei, H. (2009). Access walking distance, transit use, and transit-oriented development in North York City Center, Toronto, Canada. Transportation Research Record: Journal of the Transportation Research Board, 2110(1), 96–105. https://doi.org/10.3141/2110-12
- Daniels, R., & Mulley, C. (2013). Explaining walking distance to public transport. The dominance of public transport supply. Journal of Transport and Land Use, 6(2), 5–20. https://doi.org/10.5198/jtlu.v6i2.308
- DEJTR. (2016). VISTA Online: Web based analysis tool for the Victorian Integrated Survey of Travel and Activity. http://economicdevelopment.vic.gov.au/transport/research-and-data/vista
- Dill, J. (2003). Transit use and proximity to rail: Results from large employment sites in the San Francisco, California, Bay Area. Transportation Research Record: Journal of the Transportation Research Board, 1835(1), 19–24. https://doi.org/10.3141/1835-03
- Durand, C. P., Tang, X., Gabriel, K. P., Sener, I. N., Oluyomi, A. O., Knell, G., Porter, A. K., Oelscher, D. M., & Kohl, H. W. (2016). The association of trip distance with walking to reach public transit: Data from the California Household Travel Survey. Journal of Transport & Health, 3(2), 154–160. https://doi.org/10.1016/j.jth.2015.08.007
- El-Geneidy, A., Grimsrud, M., Wasfi, R., Tétreault, P., & Surprenant-Legault, J. (2014). New evidence on walking distances to transit stops: Identifying redundancies and gaps using variable service areas. Transportation, 41(1), 193–210. https://doi.org/10.1007/s11116-013-9508-z
- Ermagun, A., Samimi, A., & Rashidi, T. H. (2016). How far is too far? Providing safe and comfortable walking environments. Transportation Research Record: Journal of the Transportation Research Board, 2586(1), 72–82. https://doi.org/10.3141/2586-08
- Fairnie, G. A., Wilby, D. J. R., & Saunders, L. E. (2016). Active travel in London: The role of travel survey data in describing population physical activity. Journal of Transport & Health, 3(2), 161–172. https://doi.org/10.1016/j.jth.2016.02.003
- García-Palomares, J. C., Gutiérrez, J., & Cardozo, O. D. (2013). Walking accessibility to public transport: An analysis based on microdata and GIS. Environment and Planning B: Planning and Design, 40(6), 1087–1102. https://doi.org/10.1068/b39008
- Grisé, E., Wasfi, R., Ross, N. A., & El-Geneidy, A. (2019). Evaluating methods for measuring daily walking to public transport: Balancing accuracy and data availability. Journal of Transport & Health, 15, 100638. https://doi.org/10.1016/j.jth.2019.100638
- Guessous, Y., Aron, M., Bhouri, N., & Cohen, S. (2014). Estimating travel time distribution under different traffic conditions. Transportation Research Procedia, 3, 339–348. https://doi.org/10.1016/j.trpro.2014.10.014
- Hess, D. B. (2012). Walking to the bus: Perceived versus actual walking distance to bus stops for older adults. Transportation, 39(2), 247–266. https://doi.org/10.1007/s11116-011-9341-1
- Hoback, A., Anderson, S., & Dutta, U. (2008). True walking distance to transit. Transportation Planning and Technology, 31(6), 681–692. https://doi.org/10.1080/03081060802492785
- Kaszczyszyn, P., & Sypion-Dutkowska, N. (2019). Walking access to public transportation stops for city residents. A comparison of methods. Sustainability, 11(14), 3758. https://doi.org/10.3390/su11143758
- Ker, I., & Ginn, S. (2003). Myths and realities in walkable catchments: The case of walking and transit. Road and Transport Research, 12(2), 69–80.
- Low, V. J. M., Khoo, H. L., & Khoo, W. C. (2023). On the prediction of intermediate-to-long term bus section travel time with the Burr mixture autoregressive model. Transportmetrica A: Transport Science, 20(3). https://doi.org/10.1080/23249935.2023.2181023
- Morency, C., Trépanier, M., & Demers, M. (2011). Walking to transit: An unexpected source of physical activity. Transport Policy, 18(6), 800–806. https://doi.org/10.1016/j.tranpol.2011.03.010
- Noh, N., Mohamad, D., & Abdul-Hamid, A. H. (2021). Acceptable walking distance accessible to the nearest bus stop considering the service coverage [Paper presentation]. Proceedings of International Congress of Advanced Technology and Engineering (ICOTEN), Institute of Electrical and, Electronics Engineers (IEEE). https://doi.org/10.1109/ICOTEN52080.2021.9493435
- O'Sullivan, S., & Morrall, J. (1996). Walking distances to and from light-rail transit stations. Transportation Research Record: Journal of the Transportation Research Board, 1538(1), 19–26. https://doi.org/10.3141/1538-03
- Philp, M., & Taylor, M. A. P. (2017). A research agenda for low carbon mobility: Issues for new world cities. International Journal of Sustainable Transportation, 11(1), 49–58. https://doi.org/10.1080/15568318.2015.1106261
- Polosin, V. G., Mitroshin, A. N., & Gerashchenko, S. I. (2023). Burr Type XII distribution in traffic control systems. Transportation Research Procedia, 68, 433–440. https://doi.org/10.1016/j.trpro.2023.02.058
- PTV. (2012). Facts and figures. Public Transport Victoria. Retrieved March 19, 2023, from https://web.archive.org/web/20121214015704/http:/corp.ptv.vic.gov.au/managing-victoria-s-public-transport-network/facts-and-figures
- Pueboobpaphan, R., Pueboobpaphan, S., & Sukhotra, S. (2022). Acceptable walking distance to transit stations in Bangkok, Thailand: Application of a stated preference technique. Journal of Transport Geography, 99, 103296. https://doi.org/10.1016/j.jtrangeo.2022.103296
- Rastogi, R., & Rao, K. V. (2003). Travel characteristics of commuters accessing transit: Case study. Journal of Transportation Engineering, 129(6), 684–694. https://doi.org/10.1061/(ASCE)0733-947X(2003)129:6(684)
- Sarker, R. I., Mailer, M., & Sikder, S. K. (2020). Walking to a public transport station: Empirical evidence on willingness and acceptance in Munich, Germany. Smart and Sustainable Built Environment, 9(1), 38–53. https://doi.org/10.1108/SASBE-07-2017-0031
- Tadikamalla, P. R. (1980). A look at the Burr and related distributions. International Statistical Review / Revue Internationale de Statistique, 48(3), 337. https://doi.org/10.2307/1402945
- Taylor, M. A. P. (2017). Fosgerau’s travel time reliability ratio and the Burr distribution. Transportation Research Part B: Methodological, 97, 50–63. https://doi.org/10.1016/j.trb.2016.12.001
- Taylor, M. A. P., & Thompson, S. M. (2019). An analysis of active transport in Melbourne: Baseline activity for assessment of low carbon mobility interventions. Urban Policy and Research, 37(1), 62–81. https://doi.org/10.1080/08111146.2018.1437031
- Tennøy, A., Knapskog, M., & Wolday, F. (2022). Walking distances to public transport in smaller and larger Norwegian cities. Transportation Research Part D: Transport and Environment, 103, 103169. https://doi.org/10.1016/j.trd.2022.103169
- Tsioulianos, C., Basbas, S., & Georgiadis, G. (2020). How do passenger and trip attributes affect walking distances to bus public transport stops? Evidence from university students in Greece. Spatium, 44(44), 12–21. https://doi.org/10.2298/SPAT2044012T
- Van Soest, D., Tight, M. R., & Rogers, C. D. F. (2020). Exploring the distances people walk to access public transport. Transport Reviews, 40(2), 160–182. https://doi.org/10.1080/01441647.2019.1575491
- Vuchic, V. R. (2007). Urban transit systems and technology. John Wiley and Sons.
- Wasfi, R. A., Ross, N. A., & El-Geneidy, A. M. (2013). Achieving recommended daily physical activity levels through commuting by public transportation: Unpacking individual and contextual influences. Health & Place, 23, 18–25. https://doi.org/10.1016/j.healthplace.2013.04.006
- Zhao, J., & Deng, W. (2013). Relationship of walk access distance to rapid rail transit stations with personal characteristics and station context. Journal of Urban Planning and Development, 139(4), 311–321. https://doi.org/10.1061/(ASCE)UP.1943-5444.0000155