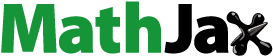
ABSTRACT
The coupling of human, management, mechanical, and environmental risk factors increases the probability of accident occurrence at metro construction sites. The degree of coupling risks at metro construction sites is the degree of interdependence among the risks in subsystems during metro construction projects. This paper uses the coupling relationship between the human and environmental risks to predict the progression in overall risk-level of construction process at Rongjiazhai station of Xi’an Metro Line 5 in China. The coupling model and system dynamics simulation are applied in four steps: develop the coupling model, estimate the weight index for metro construction risk factors, calculate the risk levels, and estimate the degrees of coupling risks. This paper identifies that the change of coupling coefficients of human-environmental factors has a significant influence on the risk-level at metro construction sites. The “psychological and physiological quality,” “natural and working environment,” and “physiological discomfort and working environment” are the most influential factors of the coupling level of human-environmental risks. The simulation results demonstrate that the coupling effects of risk factors should be emphasized in engineering practice. To reduce the risk-level of construction system, the human, mechanical, and environmental risks should be identified, coupled, and monitored in real time.
Introduction
The metro construction is a complex system involving uncertainty, multi-technology, and risks in the construction environment. The coupling of various risks in the construction process leads to accidents. China is investing on the high-speed and metro projects to expand the rail network to 200,000 km over the coming decade, of which over 45,000 km will be high-speed lines (China Urban Rail Transit Association, Citation2016). A total length of over 11,700 km metro network with 300 metro lines is expected to be constructed in Mainland China by the year 2050 that is 10 times of current metro network (Ministry of Commerce of the People’s Republic of China, Citation2015). The unprecedented pace of large-scale metro construction projects endangers the safety of construction environment. There were approximately 80 accidents occurred at the metro construction sites during the period of 2011–2016 in China (Ministry of Housing and Urban Rural Development, Citation2017). A total of five construction workers were died due to the collapse of Hujiamiao section at Xi’an Metro Line 3 in May 2013. An area of about 32 square-meters road surface was collapsed about 1,000 meters east of Nanyang Lake Bridge at the 22nd contract section of Shenyang Metro Line 9 in October 2016. The accident occurrences at metro construction sites are caused by the interaction of human, management, mechanical, and environmental risk factors in the construction process.
The existing safety standards of metro construction in China that include the design specifications, safety control standards, and worker behavior regulations lack the consistency and consolidation (Liu, Citation2011). These safety standards are generally focused on the technical aspects with insignificant attention on the human and environmental risk factors.
The degree of risk coupling can be evaluated quantitatively, but it is difficult to assess the complex relationship among different types of coupling risks under different conditions. The qualitative methods, the systematic and objective means of describing and quantifying the phenomena, can assess the risk interactions, formation mechanisms and interactive coupling effects of risk factors (Longo et al., Citation2015). There are relatively few studies (Pan et al., Citation2019; Wu et al., Citation2016) on system risk coupling evaluation at metro construction sites. The application of coupling mechanism and coupling model in the field of metro construction is not mature due to various factors and complex relationship among different types of coupling risks under different conditions. The complexity of engineering in the metro construction project combined with the independent role of risk factors causes the coupling effect between risk factors, and the consequences are more serious. The safety management of metro construction should combine the coupling mechanism and coupling model in the risk evolution mechanism and take measures to effectively control the metro construction risks and reduce accidents. The coupling degree measurement model can simulate and analyze the future development trend of human-environmental coupling risk of metro construction. The coupling model adjusts the magnitude of coupling coefficients for different risks in the system to change the overall level of human-environmental coupling risks and supports. It is used to determine the key management and control points for the metro construction safety and suggests the relevant risk control. This paper applies the investigation methods, N-K model and SD system dynamics model to explore the coupling effects of human and environmental risk factors in metro construction. The findings of this paper help the construction managers to understand the coupling mechanism of construction risks, manage the coupling risks, and provide a reference for the relevant research on the coupling risks at metro construction projects.
This paper discusses the current studies on the risk assessment of metro construction projects and the SD simulation environment. The details of the project site is discussed followed by stating the coupling model and simulating the human-environmental coupling risks. This paper analyses and discusses the modeling outputs. Finally, the limitations and scope for future studies are illustrated followed by a conclusion section.
Literature review
The risk assessment of metro construction involves uncertainty in identifying, evaluating, and responding the negative effects through avoiding, mitigating, and eliminating strategies (Badalpur & Nurbakhsh, Citation2019). The previous studies (Hyun et al., Citation2015; Seo & Choi, Citation2008; Wang & Chen, Citation2017; Wu et al., Citation2015; Špačková et al., Citation2013) assessed the risk at metro construction sites focusing on planning and reducing the vulnerabilities (Qingjun et al., Citation2020).
Brooker (Citation2006) worked on the coupling risk simulation by combining the risk models and safety goals in air crashes. Ding and Zhou (Citation2012) proposed the application of information technology in identifying and early warning the security risks. Morita and Yoshimura (Citation2013) studied a stochastic matrix model to understand the mechanism of risk coupling expansion (or better hedging) through decentralization and analyzed the stochastic diffusion model of coupled risk around the world. Shyur (Citation2008) used the accident data and safety indicators to quantify the aviation risks caused by human errors. Shyur (Citation2008) predicted the possible accidents through the proportional risk coupling model and urged for monitoring the risk coupling process.
Studies on coupling risk in China are also emerging, mostly for coal mining, ecology, aviation, metro construction, and other related fields (Pan et al., Citation2019; Wu et al., Citation2016; Xu, Citation2012). Wu et al. (Citation2016) first applied the N-K model for the coupling probability statistics that was followed by studies on coupling simulation for the metro construction process. Wu et al. (Citation2016) estimated the probability and effects of risk coupling among human, equipment, management, environment, and other factors on metro construction safety. Xu (Citation2012) investigated the impact of risk coupling associated with different types of construction risks using the finite element method. M. Chen (Citation2016) used the coupling model combined with the inverse and positive cloud to calculate the connection between risk factors. Qiao (Citation2014) used the coupling model to calculate the risk coupling degree of coal mine safety production system. Pan et al. (Citation2019) applied the fuzzy entropy theory to define an index system for the construction safety at shield tunnels. The theory of coupling degree was used to quantify the coupling degree among various factors in the safety risk system (Pan et al., Citation2019).
The degree of risk coupling can be quantitatively evaluated, but it is difficult to assess the complex relationship among different types of coupling risks under different conditions. The qualitative methods, the systematic and objective means of describing and quantifying the phenomena, can assess the risk interactions, formation mechanism, and interactive coupling effects of risk factors (Longo et al., Citation2015). There are relatively few studies (Pan et al., Citation2019; Wu et al., Citation2016) on system risk coupling evaluation at metro construction sites due to the large number of factors, type of risk coupling, and the complex relationship required to be considered. The safety management of metro construction should combine the coupling mechanism and coupling model in the risk evolution mechanism and take measures to effectively control the metro construction risks and reduce accidents. The coupling degree measurement model can simulate and analyze the future development trend of human-environmental coupling risk of metro construction safety. The coupling model adjusts the magnitude of coupling coefficients for different risks in the system to change the overall level of human-environmental coupling risks and supports. It is used to determine the key management and control points for the metro construction safety risk and highlight the relevant risk control suggestions. This paper applies the investigation methods, N-K model and SD system dynamics model to explore the human-environment coupling factors of construction risks.
Method
The SD simulation was used to diagram the human-environmental coupled risk loop, and Vensim PLE software (Vensim, Citation2015) was used to simulate different degrees of risk change. The SD model is a quantitative research method based on the system and information theories and uses the Vensim PLE to simulate the dynamic behavior of complex systems. The Vensim PLE graphically processes the concepts in system dynamics and assigns specific graphics to the concepts in systems to enable the visualization of abstract mathematical system models. The Vensim PLE describes the system structure by means of drawing to present the state and behavior of the system in a graphical manner. The SD model is developed in four steps: build the coupling model, estimate the weight index for the metro construction safety risk, calculate the risk level for each indicators of risks, and estimate the risk coupling degrees.
The accident occurrences at the metro construction sites are caused by the coupling of multiple risk factors. The analysis of inter-connected risk factors considers the understanding of coupling relationship among risk factors, their causes, and the frequency reduction of accidents at construction sites. The N-K model, developed from information theory, is applied to identify the degree of interaction between human and environmental risk factors through statistical classification of historical metro accidents. The coupling risk probability and risk coupling value are later calculated. The probability of accidents at the metro construction site is determined by measuring the magnitude of the risk coupling value. A weight index of the metro construction risks is designed using an Analytical Hierarchy Process (AHP) model. The risk level of indicators is estimated by experts, scoring method and reverse cloud model. The power function is used to estimate different risk coupling degrees.
Project site overview
This study has taken the Rongjiazhai station of the first phase of the Xi’an Metro Line 5 project as the case study. The Rongjiazhai station is located at the intersection of Yanxiang Road, Jieqi Road, and Tingzi Road. The Rongjiazhai station is a two-story underground island station with a two-column three-span cast-in-place concrete frame structure. The total length of Rongjiazhai station is 220.8 m, and the standard section width is 21.2 m. The station has four entrances and exits and two sets of wind pavilions. The total construction area of the station is 13,200 square meters (m2) with 10,348 m2 of main building and 2,852 m2 of auxiliary building. The left line of station is connected to the shield section on the left line, the right line is connected to the dark excavation section, and the double line is provided with the shield receiving wall. The tunnel arch is buried at a depth of 16.9 m to 26 m with the groundwater depth at between 36.30 m and 57.0 m, and the corresponding elevation is 425.43 m to 444.47 m. The Yuejiazhai Station to Changming Road Station section, through the ground fissure f10, should reserve the amount of ground fissure displacement. There may be flammable, explosive, and other toxic and harmful gases such as biogas in the cave.
The collapsible loess foundation in the site is 1–2 layers of plain fill and 2–1 layers of loess-like soil, and the collapsible soil layer is continuously distributed in the site. The buried depth of the collapsible soil layer is less than 10–15 m, and the foundation is wet. The site category is Class II, and the basic intensity of the earthquake is octave. The weather in Xi’an is warm and semi-humid continental monsoon climate with long winter and summer, short spring and autumn, dry springs, hot summers, wet and rainy autumns, cold and dry winters, and significant differences between mountainous and plain climates.
Risk coupling model
The N-K model measures the coupling values of human, mechanical, environmental, and management factors in metro construction, together with the homogeneity factors among human, machine, ring, and tube. The risk indicators in the metro construction safety system are identified reviewing relevant studies in section 2 and illustrated in . The coupling effects of human and environmental risk factors are selected in this study. This study applied the AHP to determine the weight of the first-level index.
Initially, an in-depth analysis of problems was performed for establishing the hierarchical structure model. The decision-making problem was divided into three levels: the upper target level, middle criterion level, and lowest case level. The judgment matrix is constructed, and the index elements at the same level are compared with each other based on their importance degree (EquationEquations 1(1)
(1) -Equation9
(9)
(9) ). EquationEquation 1
(1)
(1) shows the judgment matrix of the first-level index, A where A= {human risk, mechanical risk, environmental risk, management risk}={B1, B2, B3, B4}.
The secondary indicators of human factors (B1) are B1={professional technical ability, educational level, safety production awareness, psychological quality, on-site disposal risk capability, physical discomfort}={B11,B12,B13,B14,B15,B16}. The secondary indicators of environmental factors are B2={deteriorating work environment, complex social environment, unforeseen natural environment, environmental impacts of surrounding buildings}={B21,B22,B23,B24}. The judgment matrices for B1 and B2 are shown in EquationEquation 2(2)
(2) and Equation3
(3)
(3) , respectively.
The AHP method was applied to estimate the weighting indices of human and environmental risk factors () and indicators (
) using EquationEquations 1
(1)
(1) -Equation4
(4)
(4) , respectively (). The equivalent ranges of risk level are assigned in the Xi’an Metro Line 5 project ().
Table 1. Weighting indices of human and environmental factors
Table 2. Definition of safety risk classification for metro construction
A total of five project department personnel and five construction workers from different projects were selected to score the human and environmental risk factors. The personnel from project department have at least a bachelor's degree in relevant disciplines and 5 years of engineering experience in no less than three similar projects.
The purpose of selecting different staff expertises to score is to view problems from different perspectives. The reverse cloud model and Delphi expert scoring method estimate the risk level of indicators (EquationEquations 5(5)
(5) -Equation9
(9)
(9) ). To eliminate the potential selection bias, the scoring process was conducted over several rounds, and invalid scores were excluded. The new expert scoring results are added until the results meet the discrete requirements. The complex number in super entropy indicates that the randomness and fuzziness of scoring data after processing do not meet the requirements, resulting in the minimum of some attribute values of characteristic numbers, which will be in complex form after square root (EquationEquation 9
(9)
(9) ).
If the super entropy (He) digital feature generated by the scores of 10 experts in the inverse cloud is complex, the group randomness and ambiguity do not meet the requirements and the discreteness that leads to the result is complex. Then, the maximum score is eliminated and the new expert score is added. The final 10 expert scores and the inverse of the inverse cloud model (expectation, entropy, and super entropy expressed as Ex, En, and He) after three rounds to eliminate deviation are shown in .
Table 3. Expert scoring and reverse cloud model digital features
Risk coupling degrees are estimated using power functions (EquationEquation 10(10)
(10) and Equation11
(11)
(11) ).
where Exij is the first-class factor for the safety risks of metro construction. All experts of the second-level indicator j under i (r and h indicator codes in ) score the expected value. Aij and Bij are the upper and lower limits of the order parameter.
Uij is the function coefficient of efficacy that indicates the degree to which the indicators of each level reach the target value. The nearer to 0, the larger the difference with the target value. Values of Uij closer to 1 indicate a stronger consistency with the target.
Ui is the orderly contribution degree of each subsystem, and it is the weight of each order parameter. However, this study does not require to calculate the coupling risk under the first-level index; therefore, only Uij between the second-level indicators was calculated.
The coupling model of the system is given in EquationEquation 12(12)
(12) where m refers to the second-level coupling index and Uk
represents the factors those participate in the coupling process. The degrees of coupling are low, moderate, high, and the strongest for
,
,
and Cm = 1, respectively. The construction risk level is proportional to the degree of risk coupling. The defense mechanism of metro construction system can resist the risk impact when the risk system exhibits a low-risk coupling. However, the risk has a certain degree of damage with a medium risk coupling. There is the possibility of a sudden surge to break through the defense mechanism. At high-risk coupling, the metro construction system is in an extremely dangerous state (Qiao, Citation2014). The coupling degree model is shown in EquationEquation 12.
(12)
(12)
The numerical modeling of secondary indicators “professional technical ability (23.6000, 3.1333, 3.4196)” and “culture (24.9000, 3.7098, 5.2518)” under the human risk factor in is used to calculate the degree of coupling between risk indicators (EquationEquations 13(13)
(13) -Equation15
(15)
(15) ). The estimated value of the degree of coupling between professional technical ability and culture is
, corresponding to a medium coupling level. This numerical process is repeated to calculate the degree of coupling of the homogeneous (Equation 16) and heterogeneous (Equation 17) risk factors for the secondary indicators in the causal loop diagram ().
Table 4. Human-environmental risk coupled system SD model variable set and value
Homogeneous factor:
Heterogeneous factor:
Human-environment coupling risk simulation application
The estimated values of numerical characteristics of the inverse cloud model in are taken as the initial values of horizontal variables for the first-level indicators. The results of the coupling degree values from Equations (16) and (17) are taken as the risk coupling coefficients. The higher value of variables indicates the higher risk at the metro construction site. The variables set, perfect variables set, and their values are shown in .
The coupling risk causality loop diagram is developed based on the coupling model indicator that is the basis of the SD model (). There are three main feedback loops in the diagram ().
Psychological quality, production safety consciousness, human risk factors, human-environmental risk level, and psychological quality. This is a positive feedback loop. When the psychological quality of the metro construction personnel increases, their awareness of production safety is relatively high, the probability of human risk factors is reduced, the risk level of human-loop coupling is reduced, and in turn, the psychological quality of people is more stable.
Psychological quality, ability to deal with risks on the spot, human risk factors, human-environmental risk level, and psychological factors. This is a positive feedback loop. The higher the psychological quality, the stronger the ability to deal with risks, the lower the artificial risk factors, the lower the risk level of human-loop coupling, and in turn, the more stable and less disturbance on the psychological quality.
Human-environmental factors coupling risk level, complex social environment, psychological quality, ability to deal with risks in the face of unforeseen natural environment, poor working environment, professional and technical ability, environmental impact of surrounding buildings, environmental risk factors, and human-environmental factors coupling risk level. This is a positive feedback loop. The increase of human-environmental risk level leads to an increase of interpersonal relationship risk in complex social environment that increases the low mood of employees, the risk of psychological quality, and risk of on-the-spot disposal, and decreases the ability of on-the-spot disposal. In case of unpredictable natural environment, the risks will be superimposed, and the hazardous natural environment will make the working environment even worse. The lack of professional skills will make the workers unable to deal with emergencies, thus increasing the risks and encounters in the complex surrounding building environment leading to an increase of the environmental risk. The environmental risks eventually increase the overall human-environmental risk level.
The velocity and flow rate models were built using the Vensim PLE software following the causal loop diagram, the initial level of risk, coupling coefficient of the operation of function, and AHP operation (). The weight indices of all risk indicators were assigned into the software by the reverse cloud generator. The outputs intuitively reflect the variation trend of the total value of human-loop factor risk coupling level in the operating cycle.
Experts evaluated the risk associated with the metro construction project in February 2017. This study considered the 14-month simulation cycle from February 2017 to April 2018. The velocity and flow rate model was constructed using the causal loop diagram and modeling equation, the initial risk level scored by experts, the coupling coefficient of efficacy function operation, and the weights of various indices in AHP operation. The trend of total value of risk coupling level for the human and environmental factors in the set running cycle can be intuitively reflected from the simulation results. If the human-environmental coupling system is in a state of severe and extreme risk, take effective measures to control and reduce the risk.
The 14-month simulation cycle was combined with the coupling relationship of various factors in the flow rate diagram for human-environment loop coupling risk. The risk development level of human-environmental risk coupling system is relatively stable without foreign object interference and is between level I and level II risks). The project has achieved remarkable results in controlling human and environmental risks without breaking the defense mechanisms of each subsystem. Although the project has an effective defense mechanism, once some risk couplings occur, its risk level is in an irreversible state and the risk value will increase continuously and accumulate slowly. The results of operation are analyzed by the cause tree. The growth rate of risk level for each secondary indicator under human and environmental factors is small, and the risk increase level of each secondary index is relatively stable.
Analyses and discussion
The homogeneous and heterogeneous risk coupling coefficients in the human-environmental risk system were adjusted to study the influence of coupling between artificial and environmental risks on the risk level; and only one risk coupling coefficient was adjusted at a time. The adjustment factors for each coupling coefficient are equal to 10%. The influence of coupling coefficients for different homogeneous human factors, homogeneous environmental factors, and heterogeneous human-environmental risk on the value change of the risk coupling level of human-environmental factor (RHZSP) was observed to determine the most influential factors of risk control in risk coupling.
The homogeneity and heterogeneous coupling coefficients were increased by 10% whilst other coupling coefficients remained unchanged. The process was run thirteen times depending on the number of coupling coefficients, and the results were divided into three groups. These groups were the homogeneous human factors (Cr4-r6, Cr4-r3, Cr3-r2, Cr2-r1, Cr4-r5, Cr1-r5), the homogeneous environmental factors (Ch3-h1, Ch4-h1), and the heterogeneous human-environmental factor (Cr6-h1, Cr1-h1, Cr5-h3, Cr4-h2, Cr1-h4).
The influence of incremental coupling coefficient of homogeneous human factors on the change of total risk level can be illustrated by Cr4-r6 increase>Cr3-r2 increase>Cr4-r3 increase>Cr2-r1 increase>Cr1-r5 increase>Cr4-r5 increase. The homogeneous human factor (Cr4-r6) has the fastest growth rate and increased beyond 60 in October corresponding to a risk level IV, indicating the psychological and physiological qualities in the coupling of homogeneous human factors. Coupling (Cr4-r6) has the highest impact on the RHZSP. A 10% decrease of the initial coefficient values results in the decrease in the degree of influence of coupling human factors illustrated by Cr1-r5 decrease > Cr4-r5 decrease > Cr2-r1 decrease > Cr4-r3 decrease > Cr3-r2 decrease > Cr4-r6 decrease. The increasing rate of Cr1-r5 (coupling coefficient of professional technical and on-site disposal risk abilities) is the most obvious followed by Cr4-r5 (coupling coefficient of psychological quality and on-site disposal risk ability); otherwise the increase is relatively stable. The influence of incremental coupling coefficient for homogeneous environmental factors on the change of total risk level can be illustrated by Ch3-h1 increase > Ch4-h1 increase. The unpredictable coupling coefficient (Ch3-h1) between the natural and working environment affects the growth rate of RHZSP. The degree of influence for coupling coefficient of environmental factors considering 10% decrease of initial coefficient values is given by Ch3-h1 decrease > Ch4-h1 decrease. A 10% increase of initial coefficient values leads to a greater impact on the RHZSP, characterized by Ch3-h1, but does not exceed the severity level.
The influence of incremental coupling coefficient of heterogeneous human-environmental factors on the change of total risk level is given by Cr6-h1 increase > Cr4-h1 increase> Cr1-h4 increase > Cr4-h2 increase > Cr5-h3 increase. The coupling of physiological discomfort and working environment factor (Cr6-h1) that exceeds the risk value of 60 (risk level IV) on December is the most important factor for the RHZSP. A 10% decrease of the initial coefficient values affects the degree of influence of coupling human-environmental factors as follows: Cr4-h2 decrease > Cr1-h4 decrease > Cr1-h1 decrease > Cr6-h1 decrease > Cr5-h3 decrease. The increase rate of Cr4-h2 (psychological quality and complex social environment) at the metro construction project is the most obvious followed by Cr1-h4 (professional technical ability and surrounding architectural environment influence). The total horizontal value (RHZSP) has a large impact but does not exceed the risk level IV.
The heterogeneous human-environmental factor risk coupling has the most significant impact on the total risk coupling level. A 10% increase in the initial coupling coefficients results in the coupling coefficients for human-environmental and environmental factors to have the highest and lowest influences on the RHZSP at the construction site of Xi’an Metro Line 5, respectively. However, the coupling coefficients of psychological and physiological quality (Cr4-r6), natural and working environment (Ch3-h1) and physiological discomfort and working environment (Cr6-h1) have the highest impact on the human-environmental factor risk coupling level. Identification and control of these critical factors can reduce the overall risk level at the metro construction site (Rongjiazhai station) of Xi’an Metro Line 5. It is not easy to decrease the risk level after reaching at a certain threshold level, and the increase of risk coupling coefficients rapidly increases the risk value. However, it is obvious that the decrease of risk coupling coefficients may not decrease the overall risk level. A construction site with safe human, mechanical, environmental, and pipe defense system should be established to control the growth of the risk coupling coefficients. The pre-controlled risk management should be strengthened to avoid coupling shocks in the weak system so that the overall system risk level should be increased and should not be reached at risk.
For the construction risk control of human factors, the following measures can be taken: 1) check the experience and qualification certificate of construction workers to ensure that the professional and technical ability meets the standard; 2) pay attention to safety education and training to improve people’s cultural and technical literacy; 3) inculcate the idea of production safety and enhance employees’ awareness of production safety; 4) communicate with employees regularly and pay attention to their work psychological changes; 5) improve the preparation and practice of emergency plans, and improve the ability to deal with risks on the spot; and 6) avoid fatigue and illness during construction and pay attention to workers’ health.
For the construction risk control of environmental factors, the following measures can be taken: 1) improve the working environment and ensure the comfort level of the working environment; 2) carefully study the safety standards and deal with the complex social relations in the metro construction scope; 3) inform in advance in case of bad weather and take measures to ensure safe production; and 4) make preparations in advance to reduce the impact of surrounding building environment on construction.
For the construction risk control of mechanical factors, the following measures can be taken: 1) perform mechanical equipment installation according to the provisions of the original factory; 2) strictly check each link to avoid machine operation failure; 3) identify the dangerous parts of the machinery and check the safety protection device of the equipment; and 4) regular inspection and maintenance of construction equipment.
For the construction risk control of management factors, the following measures can be taken: 1) formulate a reasonable reward and punishment scheme to increase the incentive for worker safety; 2) master basic safety knowledge and strengthen safety education and training for employees; 3) effectively control all production risk factors and improve the on-site safety production management system; 4) pay attention to the people-oriented development concept, build a good corporate safety culture atmosphere; and 5) clarify the rights and responsibilities of all members of the safety inspection team and improve the safety management organization.
Limitations
There are many risk factors associated with the metro construction projects. The inclusion of all risk factors in the coupling simulation is difficult due to the complex relationships among different types of coupling risks under different conditions. The application of coupling mechanism and coupling model in the field of metro construction is not mature. This paper focuses on the specific coupling factors that have the significant impact on the coupling effect of human-environmental risks in metro construction and analyzed the trend of risk development.
A total of five project department personnel and five construction workers from different projects were selected to score the human and environmental risk factors. The purpose of selecting different types of people to score is to view problems from different perspectives. The scoring process was conducted several rounds, and invalid scores were excluded in order to reduce the potential selection bias. Although there might have a certain deviation, the deviation related to statistics was reduced to a very low level based on the existing data. The several rounds of processing scores and excluding the invalid scores reduce the standard deviation between the original scores and processed scores of the human and environmental risk factors. The small sample size undermines the statistical power of SD simulation as it increases the likelihood of a Type II error. The metro construction projects rely on the integrated efforts of several hierarchically linked parities with identical objectives, management systems, and operating procedures (Ghanbaripour et al., Citation2020). Future studies should include the perspectives of large sample size from the linked parties in metro construction projects to improve the statistical accuracy of coupling simulation.
The results of causal loop diagram in the SD model will always be self-reinforcing growth, but there are always limits to growth. Future studies will consider the self-reinforcing feedback loops in the SD model. The metro construction projects are complex and dynamic, and the coupling simulation of human-environmental safety risk factors is difficult to verify. However, this study ensures the correctness of the methods and assumptions, so the results are reasonable. The specific verification problems will be improved in subsequent studies.
Conclusions
The accident occurrences at metro construction sites are caused by the interaction of human, management, mechanical, and environmental risk factors. The increase of coupling risk factors leads to a rapid increase in the overall risk level at a metro construction site. This paper predicts the progression trend of the overall risk level in the construction process at the Rongjiazhai station of Xi’an Metro Line 5 combining the coupling relationship between human and environmental risks. The system dynamics (SD) simulation application was used to diagram the human-environmental coupled risk loop, and the Vensim PLE software was used to simulate different degrees of risk changes. This study identifies that the change of coupling coefficients for human-environmental risk factors coupling coefficients has the highest influence on the risk level at the construction site of Xi’an Metro Line 5. Among different coupling risks of human-environmental factors, this study concludes that psychological and physiological quality, natural and working environment, and physiological discomfort and working environment have the most obvious influences on the coupling level of human-environmental risk factors.
The total value of human-environmental risk coupling level in the remaining 14 months of a bid section of Xi’an Metro Line 5 is simulated dynamically. By adjusting the coupling coefficient of different kinds of risks in the human-environmental risk coupling system, the key risk coupling factors that have the greatest impact on the total value of human-environmental risk coupling level are found. Experts were selected from different projects to understand the risk and safety issues from different perspectives and to improve the universality of the research results.
In the three-factor risk coupling, the human-environment-management factor coupling value is the largest. In the two-factor coupling risk, the human-environmental factor coupling risk is the largest. This shows that human factors and environmental factors have the significant coupling effects. This finding supports the priority factor given in metro construction risk control in recent years. The risk growth of key influencing factors leads to the rapid rise of the overall risk level of the system. The coupling level of homo-human, homo-environment, and hetero-human-environment factors is significantly higher than that of other risk levels. Heterogeneous human-environmental risk coupling has the most significant effect on the total value of risk coupling level. It is not easy to reduce the risk when the risk reaches a certain threshold. A defense mechanism can only be established when the risk level is low. It is necessary to establish a good human, mechanical, environmental, and pipe defense system, strengthen the pre-control of risk management, and avoid the coupling vibration of risk in the weak parts of the metro construction system at a low-level of risk.
This paper details the results of international collaboration among Coventry University (United Kingdom), Xi’an Technological University (China), and Dentsu (Metro Line 5 contractor, China) to analyze, anticipate, and manage the coupling relationship between human and environmental risks during the Xi’an’s metro construction. It proposes and applies a new coupling risk assessment index designed with stakeholders’ involvement. The combination of coupling model and system dynamics simulation reduces uncertainties and accurately assesses the coupling risks of the dynamics of metro construction sites. The work has exploited the expert opinions involved in the construction project and highlighted how the coupling model and system dynamics simulation can improve the safety assessment and management through self-repair, adaptive capacity, recovery, and rapid response capacity.
Disclosure statement
No potential conflict of interest was reported by the authors.
References
- Badalpur, M., & Nurbakhsh, E. (2019). An application of WASPAS method in risk qualitative analysis: A case study of a road construction project in Iran. International Journal of Construction Management, 21(9), 910–918. https://doi.org/10.1080/15623599.2019.1595354
- Brooker, P. (2006). Air traffic management accident risk. Part 1: The limits of realistic modeling. Safety Science, 44(5), 419–450. https://doi.org/10.1016/j.ssci.2005.11.004
- Chen, M. (2016). The study on coupling model of construction risk in shield section of metro tunnel. Southwest Petroleum University.
- China Urban Rail Transit Association. (2016). Statistics and analysis of urban rail transit in 2015. Urban Rapid Rail Transit, 29(4), 6–11. in Chinese.
- Ding, L., & Zhou, C. (2012). Automatic risk identification and safety early-warning of underground construction under complex conditions. Engineering Sciences, 14(12), 85–93.
- Ghanbaripour, A. N., Sher, W., & Yousefi, A. (2020). Critical success factors for subway construction projects – main contractors’ perspectives. International Journal of Construction Management, 20(3), 177–195. https://doi.org/10.1080/15623599.2018.1484843
- Hyun, K. -C., Min, S., Choi, H., Park, J., & Lee, I. -M. (2015). Risk analysis using fault-tree analysis (FTA) and analytic hierarchy process (AHP) applicable to shield TBM tunnels. Tunnelling and Underground Space Technology, 49, 121–129. https://doi.org/10.1016/j.tust.2015.04.007
- Liu, K. D. (2011). Research and application of security system for urbanrail transit system. China Architecture & Building Press (in Chinese).
- Longo, E., Masellis, M., Fondi, G., Cedri, C., Debbia, C., & Pitidis, A. (2015). Qualitative analysis of emergency department reports applied to a pilot project for the prevention of pediatric burns. Annals of Burns and Fire Disasters, 28(4), 247–252.
- Ministry of Commerce of the People’s Republic of China. (2015). Rail transit industry investment promotion report. http://www.fdi.gov.cn/180000012135398_07.html, 2017-3-1 (in Chinese)
- Ministry of Housing and Urban Rural Development of People’s Republic of China. (2017). Accident letters. http://sgxxxt.mohurd.gov.cn/Public/AccidentList.aspx, 2017-2-8 (in Chinese)
- Morita, S., & Yoshimura, J. (2013). Analytical solution of a stochastic model of risk spreading with global coupling. Physical Review E Statistical Nonlinear & Soft Matter Physics, 88(5), 052809. https://doi.org/10.1103/PhysRevE.88.052809
- Pan, H., Gou, J., Wan, Z., Ren, C., Chen, M., Gou, T., & Luo, Z. (2019). Research on coupling degree model of safety risk system for tunnel construction in metro shield zone. Mathematical Problems in Engineering, 2019, 5783938. https://doi.org/10.1155/2019/5783938
- Qiao, W. (2014). Analysis of mine accident risk factor coupling and simulation of coupling risk. China University of Mining and Technology..
- Qingjun, G., Amin, S., Hao, Q., & Haas, O. (2020). Resilience assessment of safety system at metro construction sites applying analytic network process and extension cloud models. Reliability Engineering & System Safety, 201, 106956. https://doi.org/10.1016/j.ress.2020.106956
- Seo, J. W., & Choi, H. H. (2008). Risk-based safety impact assessment methodology for underground construction projects in Korea. Journal of Construction Engineering and Management, 134(1), 72–81. https://doi.org/10.1061/(ASCE)0733-9364(2008)134:1(72)
- Shyur, H. -J. (2008). A quantitative model for aviation safety risk assessment. Computers & Industrial Engineering, 54(1), 34–44. https://doi.org/10.1016/j.cie.2007.06.032
- Špačková, O., Novotná, E., Šejnoha, M., & Šejnoha, J. (2013). Probabilistic models for tunnel construction risk assessment. Advances in Engineering Software, 62–63, 72–84. https://doi.org/10.1016/j.advengsoft.2013.04.002
- Vensim. (2015). Vensim® personal learning edition. Available at: https://vensim.com/vensim-personal-learning-edition/[accessed on 14 July 2019]
- Wang, Z. Z., & Chen, C. (2017). Fuzzy comprehensive Bayesian network-based safety risk assessment for metro construction projects. Tunnelling and Underground Space Technology, 70, 330–342. https://doi.org/10.1016/j.tust.2017.09.012
- Wu, X., Liu, H., Zhang, L., Skibniewski, M. J., Deng, Q., & Teng, J. (2015). A dynamic Bayesian network based approach to safety decision support in tunnel construction. Reliability Engineering & System Safety, 134, 157–168. https://doi.org/10.1016/j.ress.2014.10.021
- Wu, X., Wu, K., & Shen, M. (2016). Research on coupling of safety risks in metro construction based on N-K model. China Safety Science Journal, 26(4), 96–101.
- Xu, K. (2012). Research on risk control of new metro tunnel passing through existing metro station closely. Master’s Thesis. Beijing Jiaotong University.