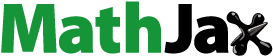
ABSTRACT
Choosing a specialty school involves huge challenges for families. This research aims to understand the behavioral process that leads families to choose among various specialty school programs. Discrete choice models, based on revealed preferences data from middle school applications in a large school district in Florida, are estimated using both, the top and all-ranked alternatives. This study returns insights into geographic settings, theme specialties and socioeconomic characteristics of applicants, schools and their relationships. Distance from home, the school’s academic quality, the school’s theme specialty, and the high percentage of applicant’s own race are found to be the strongest factors related to the decision to apply.
Introduction
Over recent decades, many countries have embarked on educational reforms aimed at increasing access to education, improving equality, and increasing efficiency and effectiveness in education. The “Learning for all” Education Sector 2020 Strategy from the World Bank was proposed with the goal “not only that all kids attend schools, but also that they learn” (World Bank Group, Citation2011). One of the goals is to promote equality in education, ensuring that all students, and not only the most privileged, acquire the knowledge and skills that are needed to succeed in life.
Meanwhile, debate persists as to whether or not school choice policies render greater equality in education. In countries such as Chile, most parents include in their list of choices only schools with similar student demographics (Elacqua, Schneider, & Buckley, Citation2006). In Ghana, students from low-performing elementary schools apply to less selective secondary schools than students from high-performing schools (Ajayi, Citation2011). In Colombia, students are extremely segregated by social class, and elite private institutions do not participate in voucher programs, available only for the poorest families (Arenas, Citation2004).
Most parents in the United States send their children to the assigned school in the attendance zone, according to the place of residence. However, when using attendance areas, school population tend to embrace concentrations of students of similar characteristics, and such schools tend to have segregated enrollment bodies (Willie, Edwards, & Alves, Citation2002). Cities such as Chicago, Tampa, Missouri, St. Louis, and Kansas City must observe strict racial guidelines for the placement of students in city schools. Some school districts offer choice options to deal with potential student segregation. In such a choice plan, families state their preferences for the schools to which they wish their children to attend.
School choice provides parents the ability to access an education marketplace to choose the school that best suits their child’s academic needs. The portfolio of available options varies among geographic locations, but in the United States most of the states include public schools, private schools, charter schools, virtual schools, home schools, or other public school options. In 2015 more than 27% of U.S. Florida’s K–12 public school students have chosen a school other than the one to which they were assigned, and 11% of Florida students were enrolled in a private school (FDOE, Citation2015).
Magnet schools began in the United States in the 1970s in large urban districts across the nation as one means of reducing racial segregation and reducing minority group isolation in public schools. The top reason for the creation of magnet schools was to promote racial, ethnic, and socioeconomic student diversity (Blank, Citation1983; Butler, Carr, Toma, & Zimmer, Citation2013; DOE, Citation2004; Rossell, Citation1991; Smrekar, Citation2009; Smrekar & Honey, Citation2015; Taylor, Phillips, & Goldring, Citation2010).
Traditionally, magnet schools seek to achieve voluntary desegregation and, consequently, they are found in neighborhoods that have large minority populations (Frankenberg & Le, Citation2008). Magnet schools draw students from across geographical boundaries, breaking down residential segregation patterns associated with traditional residential base assignments (Esposito, Citation2010). Their unique educational curricula and well-known specialty themes are intended to attract nonminority students of varying social, economic, ethnic, and racial backgrounds, and who would otherwise attend a traditional neighborhood school. The concept behind this approach is to attract students, instead of forcing them to attend desegregated schools (Rossell, Citation1991).
However, after more than 40 years, magnet schools have evolved far beyond from aiming to achieve racially integrated schools. In the previous 10 years, the main purpose of magnet schools has shifted “to provide families with the option of choosing a school that matches their child’s interests” (FDOE, Citation2005). Nowadays, what most distinguishes magnet schools from other schools is the focus in a specialty theme and high standards of achievement.
The U.S. Magnet schools concept is not unique; a similar type of schools can be found in other countries. For instance, “alternative schools” in Canada (Bosetti, Citation2004), “specialized schools” in Russia (Poder, Lauri, Ivaniushina, & Alexandrov, Citation2016), “specialist schools” in England (DfES, Citation2005). Hereafter, in this article, the term “specialty schools” refers to any of these types of schools.
Specialty themes must be relevant and appealing to parents. For the Student Placement Office in school districts, two challenging questions arise: Which themes are appealing? And where should these themes be placed? Some approaches include identifying unique curricular or instructional themes that have been successful in other districts or applying focus groups and surveys involving parents to investigate what types of programs they are interested in for their children. This study offers another systematic approach to detect appealing specialty themes and is based on statistical methods for analyzing the demand for current specialty programs by estimating discrete choice models.
The research on school choice has tended to focus on parents’ reasons for choosing schools or programs (Teske & Schneider, Citation2001). Hamilton and Guin (Citation2005) condense multiple surveys of the literature revealing insights on what, specifically, parents look for in schools. They report that parents want to find more effective educational programs, followed by religious education and a better match in cultural and family values. Bosetti (Citation2004) finds that parents employ a “mixture of rationalities” and they look for strong academic reputation, teaching style, special programs, shared values and beliefs, and smaller class size. Burgess, Greaves, Vignoles, and Wilson (Citation2014) show that the three most important factors are academic attainment, school socioeconomic composition, and travel distance.
Surprisingly, few studies include “theme specialty” as main criteria for a school or program selection. One of them, a study that analyzes students choosing a gymnasium school in Germany, reports that the theme specialty, in subjects such as sciences, languages, and music/arts, is one of the leading attractors (Müller, Haase, & Seidel, Citation2012). Aligned with that, this research claims that specialty themes are expected to have a high influence on the selection of specialty schools.
Additionally, school choice demand is geographically distributed over space. The distribution of facilities that offer educational services, and the location of the families who might place demand for such services, all takes place in a geographic context. Research suggests that parents tend to give priority to geography in the criteria for their selection (Bell, Citation2009). Because some specialty schools provide free school transportation regardless of the distance from home, understanding the way geographical location impacts choice in the selection of specialty schools is, therefore, essential. Consequently, it is expected that as well as theme specialty, geographic context also has a strong influence on the school and program selection.
This study aims to determine the set of factors that influence how families choose among public school choice options, and particularly to understand the behavioral process that leads families to choose the programs offered by specialty schools. Specific research questions arise in this context:
What is the influence of school quality and spatial elements on the choice of a specialty school program?
How do the demographic characteristics of students and schools influence parents’ choice of a specialty school program?
How important is it for an applicant to be with others of his or her own race or ethnicity? For instance, do White applicants apply to schools in which the majority students are White?
What theme specialties are preferred by applicants, both in general and by each of the socioeconomic groups, based particularly on race or ethnicity? For instance, do White applicants apply to International Baccalaureate (IB) programs?
The main contribution of this research is to provide an answer to question four, to extend understanding of the interrelations among the geographic context, specialty themes, and socioeconomic characteristics. Most of the other factors influencing the choice of school reported in the literature have already been well studied in other choice settings, but not for specialty schools. This study will uncover these interrelations for specialty school choice in a large, highly diverse and representative school district that had faced strong segregation issues in the past. It differs from previous research in this field also in the methods, since it uses Rank-Ordered Models (ROL) and Revealed Preferences (RP) data. Compared with standard widely used methods, this combination generates smaller standard errors in the estimations and delivers greater accuracy in the construction of a picture of realistic scenarios and identification of behavior patterns.
Theoretical framework and research on parental preferences
Rational choice theory suggests that rational parents choose the best school according to their personal preferences and values (utility), and the constraints they face. Parents invoke a decision rule to process all the information available and maximize their utility by making trade-offs among the attributes characterizing the alternatives. The aggregate behavior results from the sum of the behavior of individual parents, and is a function of the frequency that they choose this school over another school, in repeated choices. This focus on utility-maximization is theoretically strong and is robust; it provides a good description of the choice behavior, even if parents use different rules (Koppelman & Bhat, Citation2006).
Discrete choice models are extensively used to investigate individual-level decision making. In the traditional approach, the decision makers are asked to select only one—the most preferred option—from a set of available alternatives. In such case, researchers have the option to estimate the demand for schools using standard discrete choice models, such as the Multinomial Logit (MNL), or the Conditional Logit Model (CLM), the latter selected for this study.
However, when filling the application form for a new school, families are often asked to rank-order all or the most suitable alternatives, from best to worst. The ranking of the school programs provides much more information than simply knowing which school is most preferred. In such a case, the ROL model is the standard tool to analyze ranked data, because of its ease of computation. Consequently, this study also estimates a ROL model.
Choice modeling attempts to model the decision process of the individual by using two main methods (Beggs, Cardell, & Hausman, Citation1981; Train, Citation2009): Stated Preferences (SP) and Revealed Preferences (RP). There are advantages and limitations to each one. SP uses choices under experimental conditions and relies on survey respondents making choices over hypothetical choice scenarios, studying what parents think they would look for when choosing a school. SP has shown to be problematic for capturing real choice behavior. Researchers such as Hamilton and Guin (Citation2005), Elacqua et al. (Citation2006), and Hausman (Citation2011) raised the concern about the validity of the analysis reported by surveys. In short, parents say they are seeking strong academic programs, but they actually choose on the basis of race, ethnicity, and socioeconomic status.
In contrast, RP uses observations on actual choices already made by parents to infer true preferences. RP has been selected for this investigation, based on the assumption that parents’ true preferences can be revealed by their habits and their values captured in the school applications, and in the final enrollment decisions made by them. Nevertheless, RP faces multiple objections, being the most important (Hausman, Citation2011; Train, Citation2009): (a) it limits preferences to those alternatives that currently exist or existed historically, (b) there may be insufficient variation in relevant factors, and (c) preferences influence choices conditional on beliefs.
Top reasons for choosing schools
Many countries of the Organisation for Economic Co-operation and Development (OECD) are working to increase choice and competition in the educational system (Musset, Citation2012). The process of choosing schools is complicated, and there is no consensus on a set of specific factors and methods to analyze such choices. The literature suggests that the main five broad categories of parents’ reasons for choosing schools are related to (a) academic aspects of schooling, (b) discipline and safety, (c) geographic location and transportation, (d) race/ethnicity and the socioeconomic composition of schools, and (e) religion and values.
Preferences for academic aspects of schooling
Several studies report academic quality of the school as an essential choice criterion for parents when selecting schools. Private school choice researchers hypothesize that “market-oriented programs will have positive effects on student achievement” (Sude, DeAngelis, & Wolf, Citation2018). Research shows that families that use scholarships to attend private schools list academic quality on the top (Cohn, Citation1997). Scholarship programs allow qualified parents to use public funds to cover the cost of tuition of a private school and are targeted to a highly disadvantaged population of students (Wolf, Citation2008; Wolf et al., Citation2013).
However, parents’ definitions of school quality might not be related exclusively to test scores, but instead might be related to a broader concept. A study in Washington, DC reports that vouchers users, after some years in the program, list academic considerations such as teacher quality and class size as relevant factors (Stewart & Wolf, Citation2014).
Archbald and Kaplan (Citation2004) argue that specialty schools foster healthy competition, forcing them to try harder to satisfy parents. However, it is not clear whether this effort raises academic achievement. An RP study lead by Hastings, Kane, and Staiger (Citation2009) in Charlotte-Mecklenburg, North Carolina, finds that the academic gains from attending a first-choice school are positive for parents who place a high weight on academics; however, parents placing little value on academics experienced declines in achievement.
Since specialty schools offer a variety of philosophies, specializations, and curriculums, another argument is that they contribute to improve the fit between the schools’ programs and the parents’ preferences, since not all parents want the same education (Archbald & Kaplan, Citation2004). A national online survey reports that parents prefer strong core curriculum in reading and math, and emphasis on Science, Technology, Engineering and Math (STEM) education; nonacademic school characteristics and diversity are considered less important (Zeehandelaar & Winkler, Citation2013).
Other work had demonstrated that parental preferences for school quality might vary according to socioeconomic background. For instance, Goldring and Haussman (Citation1999) in St. Louis show that parents who choose magnet schools are of upper socioeconomic status, have little concern about distance, and have preference for academic reasons and special programs. Dissimilarly, parents who choose nonmagnet schools are likely to be minority and lower income families, and they give preference to distance, convenience, and values.
Preferences for discipline and safety
Other research has been conducted on parents’ reasons for choosing a school and reporting about factors related to discipline and safety. Latino magnet school choosers in Nashville, Tennessee, considered academic factors and safety to be high priorities when choosing a school (Taylor et al., Citation2010). A survey-based study of low-income parents in Washington, DC shows that in the beginning of the journey, private voucher users indicate that safety factors and school characteristics—such as religion, foreign languages, ethnic diversity, and school location—were the most relevant parent motivations for school choice (Stewart & Wolf, Citation2014). In contrast, in Milwaukee, Wisconsin, the preference for public school versus private schools for families awarded with a school voucher program was not associated with the availability of special programs, the quality or availability of school facilities, and the preference for school safety (Cheng, Trivitt, & Wolf, Citation2016).
Preferences for race/ethnicity and the socioeconomic composition of schools
A large body of research examines the role that socioeconomic status, race, and ethnicity play in school enrollment decisions. An influential study in Washington based on Internet search patterns suggests that race is fundamentally important to parents and they value demographic information much more highly than they admit when responding to surveys (Schneider & Buckley, Citation2002). In Minneapolis, Minnesota, parents prefer schools close to home, and they value social matching, selecting schools where their children are well matched, both ethnically and racially (Glazerman, Citation1998).
In Montgomery County, Maryland, magnet elementary schools’ parents are concerned about their children attending schools with others of the same race (Henig, Citation1990). One more study that examines magnet school application (RP) data from Philadelphia indicates that wealthier families avoid schools with high poverty rates, White families avoid schools with high percentages of non-White students, whereas non-White families show no such racial sensitivity (Saporito, Citation2003).
One RP study by Hasting and colleagues (Citation2009) estimate an exploded-mixed logit model in Charlotte, North Carolina. They find that parents have preferences for schools where their race is the majority, and higher socioeconomic status’ parents are more likely to choose higher-performing schools. Similarly, a broader RP longitudinal study in the United States by Butler and colleagues (Citation2013) estimate a modified CLM. They conclude that magnet schools “are considered an attractive option to minorities (Black and Hispanic); however, having a large proportion of minorities reduces the parents’ attractiveness of these schools” (Butler et al., Citation2013).
Freedom of choice is presented as the basis for an equitable educational system. However, some authors have found that charter schools increase racial segregation (Bifulco & Ladd, Citation2007) and the potential to further stratify schools along racial and socioeconomic profiles, allowing a mechanism to escape racial integration (Cobb & Glass, Citation1999). Sohoni and Saporito (Citation2009) examine levels of racial segregation in private, charter, and magnet schools. Their results show that “public schools would be less racially segregated if all children living in a school district attended their local, neighborhood schools.”
In contrast, other groups of researchers provide significant evidence that school choice could improve racial integration. Ritter, Jensen, Kisida, and Bowen (Citation2016) analyze the effects of charter school transfers on school integration in Little Rock, Arkansas, and show that most transfers improve integration levels at the traditional public schools they left. A study of the student migration patterns from public to private schools analyze the impacts of the Louisiana Scholarship Program (LSP). They show that “the vast majority (82%) of LSP transfers have reduced racial stratification in the voucher students’ former public schools” (Egalite, Mills, & Wolf, Citation2017).
Preferences for geographic location and transportation
Several papers have reported on the role of geography in the selection of schools. It has generally been hypothesized that families prefer schools that are close to home. Harris and Larsen (Citation2014) investigate post-Hurricane Katrina school reforms’ data in New Orleans, estimating CLM and ROL models. They conclude that the role of extracurricular activities and indirect costs, such as traveling distance, are as important as perceived academic quality.
Hastings, Kane, and Staiger (Citation2005) using RP data from Charlotte, North Carolina, estimate mixed logit models and find that parents prefer schools close to home, rather than schools with high academic achievement, and preference for school test scores increase with income and parents’ own academic ability. Similarly, in Philadelphia parents apply to schools where the theme fits their child’s interests, is located near to home, and with similar racial composition (Neild, Citation2005).
Likewise, in Denver, Colorado, and Washington, DC, Teske, Fitzpatrick, and O’Brien (Citation2009) report that modal time to school for students attending public neighborhood schools is much lower than for charter, magnet, or private students. In Washington, DC Jacobs (Citation2011) shows that parents are more inclined to select charter schools based on distance to their residential location than just on academic perception. As a result, some scholars assert that the number of new charter schools has increased dramatically in the last decade, particularly in places where race appears to have a high impact with low student achievement and growing heterogeneity (Saultz, Fitzpatrick, & Jacobsen, Citation2015).
Koedel and colleagues (Citation2009) show that the provision of busing and geographic preferences in San Diego, California, seem to be important factors in promoting integration, and families appear to use school choice programs to attend schools with more socioeconomic advantaged peers. Bell (Citation2009) shows that Detroit’ parents are willing to travel long distances when a first choice school best fit their child’s needs. A study in Minnesota concludes that school choice policy for magnet schools is responsible for longer commuting distances (Wilson, Marshall, Wilson, & Krizek, Citation2010).
Preferences for religion and values
An area of research that has not been much studied is the role of parents’ religion and values when choosing a school. The little evidence concentrates on private schools.
Scafidi and Kelly (Citation2013) surveyed private school GOAL scholarship recipients in Georgia, and list as priorities the learning environment, student discipline, religious education, improved school safety, and smaller class sizes.
Another survey study from Nashville finds that income and informal networks are the main factors when considering private schools, and parental involvement when considering public magnet schools (Goldring & Phillips, Citation2008). In Milwaukee, Wisconsin, using MNL, researchers demonstrate that brands influence the choices of parents; for example, parents who value religion education tend to choose faith-based schools (Cheng et al., Citation2016).
Summary
Several studies seem to indicate that parental preferences are multidimensional: parents are faced to weigh the importance of one specific factor relative to other factors, and they may take into consideration all of the factors simultaneously (Goldring & Haussman, Citation1999). The studies discussed previously offer some insight into parents’ and students’ choice behavior for selecting schools. This work has benefited from them by outlining the list of diverse factors that need to be considered in this study. However, they deliver mixed results. There is no consensus on the factors that influence the decision to apply. Furthermore, it leaves some questions unanswered, in particular, regarding specialty schools: What are the most preferred theme specialties? Are there theme specialties that are preferred by specific socioeconomic groups?
Understanding what families value seems to be related to the context in which families are making decisions (Hamilton & Guin, Citation2005). Therefore, we investigate what this context is for specialty schools, determining what parents actually want, to improve decision making on the supply-side. Schools need information about parental preferences and the reasons for choices in order to respond effectively to choice (Manna, Citation2002).
Data
The case study is the School District of Hillsborough County (SDHC), which serves the city of Tampa and its metropolitan area. Hillsborough County is located on Florida’s west coast, totals 3,280 km2 with over 1.2 million people, and it is organized in four jurisdictions including the City of Tampa, Temple Terrace, Plant City, and the unincorporated area. SDHC is the eighth largest school district in the United States, serving more than 206,000 students in about 279 K–12 public schools (DOE, Citation2014-15). SDHC Magnet schools accommodate the 8.5% of the total school district population with approximately 17,700 enrolled students in the 2015–2016 academic year. Magnet programs are the most popular options among the choice alternatives offered by the school district. In 2015, approximately 54% of a total of 30,200 choice applications requested admission to a magnet school.
The focus of this study is the choice of magnet programs at middle (sixth, seventh, and eighth) school grades. Enrollment in magnet schools is on a voluntary basis, and it is not determined by the location of the students since magnet schools are boundary free. Like most magnet programs operating in the United States, families who wish their children to attend a magnet school must submit an application during the annual open enrollment period. SDHC offers multiple application periods during the school year, as early as November from the previous school year to which parents want to apply. The first application period was selected for this study since it contains the most desirable attempt made by families to secure admission into the schools.
Every year a joint team between Choice Options and Magnet Schools areas at SDHC develop a marketing plan and school assignment process to adequately inform parents and students about open school alternatives for the following school year. Selection for middle magnet schools is made by a computer lottery if the number of places available at the school and grade is oversubscribed, as is usually the case. This study assumes that this random assignment makes it infeasible for parents to be strategic about their choices. Strategic behavior has been found to be problematic because parents do not reveal their true preferences; parents may rank another school first when they have low acceptance probability at their most-preferred school (Calsamiglia & GüEll, Citation2014; Haan, Gautier, Oosterbeek, & Klaauw, Citation2015).
SDHC magnet middle school locations are presented in . Most of the middle schools are concentrated in the downtown area, where large minority populations live. Points on the map represent the applicants’ home locations, which are geographically distributed around the county (broken-line). While some applicants only have to travel 0.1 miles to go to a specialized school, others have to travel 31 miles. On average, applicants are willing to travel 9 miles.
Figure 1. Geographic location of School District of Hillsborough County (SDHC) magnet middle school programs (2015–2016). describes the school programs (S1 to S12).
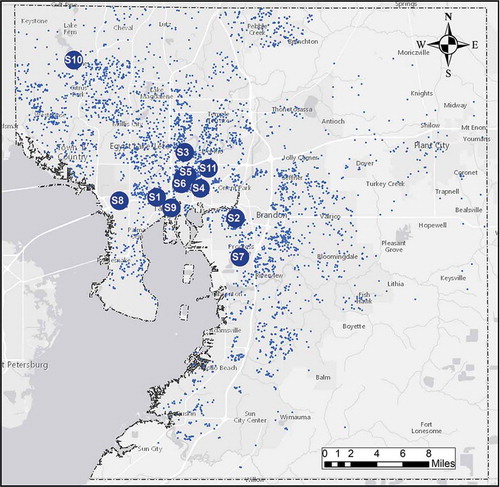
Unlike the extensive literature based on SP, our analysis of revealed preferences consists of geocoded applications for the academic period 2015–2016. This geographic dataset contains secure and anonymized applicant’s records including location and demographic details. The set of independent variables available for the study and initially included in the models is presented in . School-level data provides information about the characteristics of available school programs and geographic boundaries of school service areas. The dataset was enriched with external data sources: First, the county’s Tax Assessors Office parcel data characterizing the property. Second, school-level data from the U.S. Department of Education’s National Center for Education Statistics (NCES). Third, measures of school quality were obtained from the Florida Department of Education (FDOE).
Table 1. Set of variables available for the study.
Table 2. School District of Hillsborough County (SDHC) school characteristics for magnet middle school programs for the 2015–2016 academic year.
shows simple information about the SDHC magnet middle school dataset including 7,798 ranked choices made by 3,723 applicants to 12 magnet middle school programs for the academic year 2015–2016. Starting from the 1960s, Federal courts ruled that transportation should be used to eliminate segregation (Borman et al., Citation2004). Thus, magnet school choice options in SDHC provide free transportation to and from school locations.
The school district serves students from diverse ethnic, religious, and socioeconomic backgrounds. The eligibility for free and reduced-price meals in the school is used as a proxy for the socioeconomic status of a student. In 2015, 60% of all enrolled students were eligible to participate in the free and reduced-price school meals program.
The school program with the highest number of ranked-first applications is S10 (Walker, 16%). The IB is the most preferred specialty for ranked-first applications, with 36% of them. Most (nine out of 12) schools are high performance (A score). Half of the schools are Title-I schools, that is, schools with high percentages of economically disadvantaged students, defined as eligible for free or reduced-price meals.
SDHC has a long history of racial segregation (Groulx, Citation2016; Stretesky & Lynch, Citation2002) with historical dissimilarity indexes in 1970 of 79.9%, in 1980 of 72.6%, and in 1990 of 69.7% (Massey & Denton, Citation1998). In 2001 SDHC obtained “unitary status,” being released from court supervision, and moved from mandatory to voluntary desegregation plans during the 1990s (Borman et al., Citation2004). presents the racial/ethnic mix for both, magnet middle enrolled population and the application pool for each of the school programs in 2015. The District’s enrolled population is distributed as follows: 21% of the students are Black, 36% are White, 35% are Hispanic, and 8% of students are from other races/ethnicities.
Table 3. SDHC magnet middle enrolled population and applications by race/ethnicity for the 2015-2016 academic year.
is a map that shows cluster locations and extent of the high or low number of applications for each race/ethnicity. Visual analysis indicates observed spatial clustering of applicants from the same race/ethnicity in all cases. The locations of statistically significant hot (dark red) and cold (dark blue) spots indicate that the observed spatial clustering of the high or low amount of applicants of the same race/ethnicity in a given area is more pronounced than one would expect in a random distribution. Minorities (Black and Hispanic) are concentrated closer to downtown areas, where most of the magnet middle schools are located. If the distance is also a strong attractor, it might explain the higher level of applications for specialty schools for these two groups.
Methods
To model a student’s utility for a school program, this study first estimates a CLM, using only the top-ranked alternative. The demand for school locations is measured by deriving utility values of each applicant. As a result, the choice probabilities for each alternative are obtained (McFadden, Citation1973).
Using the notation from Allison and Christakis (Citation1994), the utility is the sum of a systematic component
and a random component
. The
term is decomposed into a linear function of a set of explanatory variables
where and
are column vectors of measured variables;
,
,
are row vectors of coefficient to be estimated. We estimate the following equation:
The vector contains case-specific explanatory variables describing the applicant’s demographic characteristics, that vary across applicants but do not vary over alternatives (school programs), including applicant’s home value (
) and applicant’s lunch status (
); the
vector contains alternative-specific explanatory variables describing the school programs characteristics, that vary across alternatives but do not vary over applicants, including school grade (
), school title-I status (
), and school specialty theme (
); and the
vector contains interaction variables, which describe a relation between alternative
and applicant
, such as the distance between applicant’s home to school (
); school’s percentage of the applicant’s race (
); a poverty interaction (
) between applicant’s lunch status (
) and school title-I status (
); and an interaction (
), between applicant’s race (
) and school specialty theme (
).
Second, using all the ranking information available, we estimate a ROL. ROL was first presented by Beggs and colleagues (Citation1981) and was further developed by Hausman and Ruud (Citation1987). Chapman and Staelin (Citation1982) named it the “Exploded Logit Model.” For the ranked model, the probability of observing specific ranking can be expressed as a sequential decision in which the applicant first chooses the most preferred alternative, and then the most preferred alternative from the rest, and so on. (Train, Citation2009). Taking the products of all these probabilities, this likelihood for a single applicant is given by (Beggs et al., Citation1981; Chapman & Staelin, Citation1982)
where each of the terms in the product has the form of a CLM. In specialty schools, applicants are constrained to rank only the top preferred alternatives, and tied ranks are not possible. This model is particularly suitable when the ranking information is incomplete at the bottom, as in this case. This model is solved using maximum likelihood estimation, and standard errors are reported.
More choice observations are available for analysis when ranking information is taken into account. Consequently, the precision of the utility model parameter estimates is improved (Chapman & Staelin, Citation1982). This ranking information also offers the possibility to use small size samples for a specified precision (Hausman & Ruud, Citation1987). Researchers use it widely since preferences can be estimated more efficiently by using the ordinal ranking of the alternatives on the choice set, allowing for separate preference parameters across individuals, and incorporating population variation modeled by case-specific socioeconomic variables.
Both, the CLM and ROL models impose the assumption of the independence of irrelevant alternatives (IIA). IIA assumes that the choices among the alternatives for an applicant are independent of each other (Long & Freese, Citation2006). This condition is undesirable, in particular when the choice set contains alternatives that are close substitutes (Ben-Akiva & Lerman, Citation1985). Some researchers argue that in the case of school choice this restriction is less problematic because there are separate preference parameters for each individual (for instance, distance from home) and school characteristics that make each alternative distinct (Harris & Larsen, Citation2014). In contrast, some geographers suggest that IIA is unlikely to hold in spatial choice applications. Some schools may be better substitutes because of spatial proximity to the selected school (Haynes & Fotheringham, Citation1990; Müller et al., Citation2012).
There are known shortcomings in the models used in this study. ROL relies on the ability of the parents to rank all the alternatives, even if there are many. Consequently, several variations of the ROL model have been proposed; see, for example, Fok, Paap, and Van Dijk (Citation2010), Allison and Christakis (Citation1994).
Results
This study investigates which of the schools’ attributes are valued by parents, and how these attributes are weighted depending on the type of specialty school to which they apply. Both CLM and ROL models were obtained, implementing the methods described in Section 4. The final model was achieved using a forward selection approach, by systematic testing of independent variables in terms of differences in log-likelihood ratios. Some variables were not significant at the 95% confidence level, and hence not included in the final model. The set of independent variables included in the final model is presented in .
Table 4. Model results for applicant’s preferences for magnet middle school programs. Ranked-first (left) vs. all Ranked-all (right) applications for 2015-2016 academic year.
The interpretation of significance is at the 0.05 level (95% confidence level). All the numbers are in contrast with the reference category, namely the IB at Walker Middle School (S10), and hence omitted from the expressions. Therefore, the coefficients for the included indicators are interpreted relative to the excluded category, which serves as a point of reference. The interpretations assume that the values for the other alternatives remain constant.
shows the coefficients, the z-statistic, associated p-values and the factor change in odds (e^b) for the CLM (left) using only the top-ranked school program, and ROL (right), using all ranking information available. Although the variables that contributed to the logistic regression for both models are the same, there is no direct way to compare the measures of fit between the two models because the models are different. However, it is possible to check that although the parameters of the models look similar in terms of sign and magnitude, the standard errors based on the CLM are much larger than ROL. Due to space limitations and similarity of the coefficients between the two models, we only read the results of the ROL model.
ROL uses more choice observations collected from individuals than CLM—not only the first and most desired choice—leading to an improved precision in the parameter estimates, without adding extra work or computation to the estimation task. Adding ranking information in the school choice marketplace is realistic, given the fact that the top-ranked option might not be available to a particular family.
Specific interpretations are possible when transforming the estimates into factor change coefficients, as shown in (see column e^b). To address the research questions designed for the study, the estimates from the ROL will be used.
Research Question 1:
What is the influence of school quality and spatial elements on the choice of a specialty school program?
Overall, the longer the distance from home to school, the less likely that school program will be chosen, as expected. Each additional mile in the distance from home to school is associated with a lower school ranking by a factor of 0.808 (19.1%). In New Orleans, a similar study by Harris and Larsen (Citation2014) reported a decrease of 40% for each additional mile, suggesting that Hillsborough families are willing to commute longer distances than New Orleans families.
School quality results show that selecting a school with intermediate performance (score C) or with low performance (score F), are both associated with a lower school ranking by a factor of 0.403 (59.7%) or 0.537 (46.3%) respectively, when compared with a high performance (score A) school.
Research Question 2:
How do the demographic characteristics of students and schools influence parents’ choice of a specialty school program?
In general, raising the parents’ home value is related to ranking all the other alternatives ahead of S10 (Walker). However, the effect of higher home value is about the same for most of the schools. More specific interpretations for each alternative are also possible. For example, a million dollar increase in an applicant’s home value is related to an increase in the odds of ranking S1 (Steward) higher than S10 by a factor of 1.013 (1.3%).
The analysis of the applicant’s preferences for schools having a significant number of students of his own poverty level reveals a positive outcome. In general, not affluent applicants seem to prefer deprived schools. Indeed, applying to schools with high—versus low—student concentrations of poverty is related to a higher ranking of these schools by a factor of 1.6070 (68.7%). Moreover, the results from the poverty interaction indicate that for an applicant, being—versus not being—eligible for free or reduced-price meals, and applying for a school with high—versus low—student concentrations of poverty, is associated with ranking these schools higher by a factor of 1.622 (62.2%).
Deprived applicants (eligible for free or reduced-price meals), when compared with affluent applicants (not eligible), are less likely to rank schools S1, S3, S4, S5, S8, and S11 higher than S10. More specific interpretations for each alternative are also possible. For example, for an applicant, being deprived is associated with a lower ranking of S1 (Steward) than S10 by a factor of 0.513 (48.7%).
Research Question 3:
How important it is for an applicant to be with others of his or her own race or ethnicity? For instance, do White applicants apply to schools in which the majority students are White?
Results from this model variable are very significant in magnitude and statistical significance. Having a school with a large proportion of students of the applicant’s own race is associated with an alternative being preferred by the applicant. Every one-point increase in the percentage of the own applicant’s race at a given school is related with ranking that school program ahead by a factor of 3.199 (219.9%). Our results can be compared with other scholar’s findings. For instance, Ibraimovic and Hess (Citation2017) using SP and MNL models show that people take account of the ethnic characteristics of their current residential locations when choosing their neighborhood of residence.
Research Question 4:
What theme specialties are preferred by applicants, both in general and by each of the socioeconomic groups, based particularly on race or ethnicity?
Our results show that specialty theme is an important attractor for specialty schools. Student interests and talents are significantly related to the choice of specialized schools. The reference category is “Other specialty” (OTH). For a school, having specialty in International Baccalaureate (IB), Performing Arts (ART) or Math/Sciences (MAT)—instead of an OTH specialty—is associated with higher school ranking, by a factor of 6.323 (532.3%) for IB, by a factor of 4.243 (324.3%) for ART; and by a factor of 3.369 (236.9%) for MAT.
The analysis of the interactions for specialties and races follows. This kind of exploration can be performed using all combinations of bases in school specialties and student races. Overall, our results indicate that there are only a few significant relationships. We nevertheless discuss some cases. Using only significant variables, “other specialty” (OTH) and “Black race” (BLA) as reference levels, preferences for specialties by different races are analyzed based on the level of significance.
First, selecting an IB school versus a school with other (OTH) specialty, is associated with: a higher school ranking by a factor of 1.6460 (64.5%) for applicants of other races; a lower school ranking by a factor of 0.790 (21%) for Hispanic applicants; and a lower school ranking by a factor of 0.756 (24.4%) for White applicants. Second, selecting an ART school versus other (OTH) specialty, is related with a lower school ranking: by a factor of 0.682 (31.8%) for applicants of other races; by a factor of 0.716 (28.4%) for Hispanic applicants; and by a factor of 0.624 (37.6%) for White applicants. Other interactions were not significant.
Conclusions
Specialty schools can be distinguished from traditional schools because they offer deeper coverage of certain subjects in which they specialize. Internationally, it has been widely recognized that learning needs to be encouraged early and consistently, and specific technical or vocational skills are critical to success in the labor market (World Bank Group, Citation2011). In England, specialist schools were introduced to increase choice and promote diversity, and are a popular option for parents due to the specialist school status (West & Noden, Citation2000). Indeed, nowadays in England, 88% of the state-funded secondary schools are specialist schools (DfES, Citation2005).
Discrete choice models statistically relate the choice made by each applicant to both, the attributes of the applicant and the attributes of the school programs available to the applicant. This study uses RP data to estimate CLM and ROL models that describe the likelihood of an applicant to choose any of the available specialty schools, as a function of several independent variables. Few previous studies have developed statistical models to investigate and predict school choice for specialty schools using rank-ordered models. Previous studies employed descriptive statistics (Archbald, Citation2004; Goldring & Haussman, Citation1999; Hausman & Goldring, Citation2000; Zimmerman & Vaughan, Citation2013), binary logit regressions (Kelleher, Smyth, & McEldowney, Citation2016), linear regressions (Davis, Citation2014; Exley, Citation2009; Henig, Citation1990), and other logit models (Burgess et al., Citation2014; Dixon & Humble, Citation2017; Glazerman, Citation1998; Lee Lauen, Citation2009). Compared with standard widely used methods, such as CLM and MNL, ROL models generate smaller standard errors and hence greater accuracy in the estimations. By describing how parents rank the school programs, rather than just specifying the school program that they like best, richer information is available for the estimation.
The case study is based on applications for places to magnet middle schools in Hillsborough, Florida, a county with a long history of efforts to achieve desegregation (Borman et al., Citation2004; Shircliffe, Citation2002). In summary, this study’s results suggest that applicants to middle specialty schools in Hillsborough County prefer schools located close to their home and with high academic performance. Applicants who are eligible for free or reduced-price meals prefer Title I schools, that is, schools with high student concentrations of poverty. Applicants are very attracted to schools with high percentage of pupils who are of their own race. They prefer theme specialties in this order: IB, performing arts, science/math, and other specialties. In general, the race is not a highly significant predictor of specialty themes, with only a few exceptions. Applicants from other races seem to be strongly attracted to IB and science/math programs. Black applicants have a powerful attraction for performing arts and IB programs.
In this framework, we explore interactions between school choice, geographical space, theme specialties, and socioeconomic characteristics of schools and families choosing specialty schools. Since elementary and middle school have no particular entry criteria, it is expected that specialized schools would accommodate a diverse body of students from various backgrounds and locations. The application pool for each school would be representative of the community demographics.
However, if applicants are attracted to schools in which their own race or socioeconomic status is in the majority, then choice would not contribute to improving diversity. From the school’s point of view, the opposite situation would be ideal: lower enrollments for a specific race or socioeconomic status are expected to receive high demand in applicants for this group. Our findings indicate that race and poverty levels are both powerful attractors. The parent behavior related to preferences for schools with high percentage of pupils who are of their own race observed in this study also reflects that found in other studies (Glazerman, Citation1998; Henig, Citation1990; Neild, Citation2005). It seems that parents’ preferences expressed in the applications for specialty schools in Hillsborough County contribute to promoting segregation instead of helping to diminish it. This kind of segregation was modeled by Schelling (Citation1969) showing that individuals might tend to self-segregate, even when they have no explicit desire to do so.
Discrete choice models estimated in this study are quite capable of determining the relative importance of school attributes, such as school academic quality and school theme specialty, versus families’ socioeconomic characteristics, such as home value and free lunch eligibility, and their interactions. One additional fundamental property of choice models is their ability to forecast consumer demand (Beggs et al., Citation1981). In the future, this model has the potential to predict the demand for new schools, offering new or existing theme specialties. This kind of spatial predictive analytics has been shown to be effective for analyzing the school network configuration in cities such as Dresden in Germany (Müller, Haase, & Kless, Citation2009).
Future research will look into models to support the design of strategies for improving school choice from the supply side, to find the location of schools and themes according to the demand, and by investigating how different student assignment policies could be designed to promote diversity at various (school and district) geographical levels. This understanding is crucial in assessing current reforms and implementing future policy changes (Shi, Citation2015).
References
- Ajayi, K. (2011, March 31–April 2). A welfare analysis of school choice reforms in Ghana. Paper presented at the Annual Meeting Program of the Population Association of America (PAA), Washington, DC.
- Allison, P. D., & Christakis, N. A. (1994). Logit models for sets of ranked items. Sociological Methodology, 24, 199–228. doi:10.2307/270983
- Archbald, D. A. (2004). School choice, magnet schools, and the liberation model: An empirical study. Sociology of Education, 77(4), 283–310. doi:10.1177/003804070407700402
- Archbald, D. A., & Kaplan, D. (2004). Parent choice versus attendance area assignment to schools: Does magnet-based school choice affect NAEP scores? International Journal of Educational Policy, Research, and Practice: Reconceptualizing Childhood Studies, 5(1), 3–35.
- Arenas, A. (2004). Privatization and vouchers in Colombia and Chile. International Review of Education, 50(3–4), 379–395. doi:10.1007/s11159-004-2629-z
- Beggs, S., Cardell, S., & Hausman, J. (1981). Assessing the potential demand for electric cars. Journal of Econometrics, 17(1), 1–19. doi:10.1016/0304-4076(81)90056-7
- Bell, C. (2009). Geography in parental choice. American Journal of Education, 115(4), 493–521. doi:10.1086/599779
- Ben-Akiva, M., & Lerman, S. R. (1985). Discrete choice analysis: Theory and application to travel demand. Cambridge, MA: MIT Press.
- Bifulco, R., & Ladd, H. F. (2007). School choice, racial segregation, and test-score gaps: Evidence from North Carolina’s Charter School Program. Journal of Policy Analysis and Management, 26(1), 31–56. doi:10.1002/pam.20226
- Blank, R. K. (1983). Survey of magnet schools. Analyzing a model for quality integrated education. Final report of a national study. Retrieved from http://files.eric.ed.gov/fulltext/ED236304.pdf
- Borman, K. M., Eitle, T. M., Michael, D., Eitle, D., Lee, R., Johnson, L., … Shircliffe, B. (2004). Accountability in a postdesegregation era: The continuing significance of racial segregation in Florida’s schools. American Educational Research Journal, 41(3), 605–631. doi:10.3102/00028312041003605
- Bosetti, L. (2004). Determinants of school choice: Understanding how parents choose elementary schools in Alberta. Journal of Education Policy, 19(4), 387–405. doi:10.1080/0268093042000227465
- Burgess, S., Greaves, E., Vignoles, A., & Wilson, D. (2014). What parents want: School preferences and school choice. The Economic Journal., 125(587), 1262–1289. doi:10.1111/ecoj.12153
- Butler, J. S., Carr, D. A., Toma, E. F., & Zimmer, R. (2013). Choice in a world of new school types. Journal of Policy Analysis and Management, 32(4), 785–806. doi:10.1002/pam.21711
- Calsamiglia, C., & GüEll, M. (2014). The illusion of school choice empirical evidence from Barcelona. Minneapolis, MN: Federal Reserve Bank of Minneapolis, Research Dep. Retrieved from http://www.minneapolisfed.org/publications_papers/pub_display.cfm?id=5317
- Chapman, R. G., & Staelin, R. (1982). Exploiting rank ordered choice set data within the stochastic utility model. Journal of Marketing Research, 19(3), 288–301. doi:10.2307/3151563
- Cheng, A., Trivitt, J. R., & Wolf, P. J. (2016). School choice and the branding of Milwaukee private schools. Social Science Quarterly, 97(2), 362–375. doi:10.1111/ssqu.12222
- Cobb, C. D., & Glass, G. V. (1999). Ethnic segregation in Arizona charter schools. Education Policy Analysis Archives, 7. doi:10.14507/epaa.v7n1.1999
- Cohn, E. (1997). Market approaches to education: Vouchers and school choice (1st ed.). Oxford, UK: Pergamon.
- Davis, T. M. (2014). School choice and segregation. Tracking racial equity in magnet schools. Education and Urban Society, 46(4), 399–433. doi:10.1177/0013124512448672
- DfES. (2005). 14–19 education and skills Hispanic paper. London, UK: Author. Retrieved from http://www.educationengland.org.uk/documents/pdfs/2005-white-paper-14-19-education-and-skills.pdf
- Dixon, P., & Humble, S. (2017). How school choice is framed by parental preferences and family characteristics: A study of western area, Sierra Leone. Journal of School Choice, 11(1), 95–110. doi:10.1080/15582159.2016.1238432
- DOE. (2004). Creating successful magnet programs. Washington, DC: U.S. Department of Education, Office of Innovation and Improvement. Retrieved from https://www2.ed.gov/admins/comm/choice/magnet/report.pdf
- DOE. (2014-15). U.S. Department of Education, National Center for Education Statistics (NCES), Common Core of Data (CCD). Retrieved from https://nces.ed.gov/ccd/ccddata.asp
- Egalite, A. J., Mills, J. N., & Wolf, P. J. (2017). The impact of targeted school vouchers on racial stratification in Louisiana schools. Education and Urban Society, 49(3), 271–296. doi:10.1177/0013124516643760
- Elacqua, G., Schneider, M., & Buckley, J. (2006). School choice in Chile: Is it class or the classroom? Journal of Policy Analysis and Management, 25(3), 577–601. doi:10.1002/pam.20192
- Esposito, C. L. (2010). School type and mathematics achievement: A comparison of magnet and public secondary schools using the Educational Longitudinal Study of 2002 Data Set (PhD). University of Connecticut, UMI, ProQuest, Ann Harbor, Michigan (UMI Number: 3447464)
- Exley, S. R. (2009). Exploring pupil segregation between specialist and non-specialist schools. Oxford Review of Education, 35(4), 451–470. doi:10.1080/03054980902989948
- FDOE. (2005). Choosing a school for your child in Florida. Tallahassee, FL: Office of Independent Education and Parental Choice, Florida Department of Education. Retrieved from https://www.floridaschoolchoice.org/information/private_schools/files/choosing_school.pdf
- FDOE. (2015). School choice options. Preparing students for success. Retrieved from http://www.fldoe.org/core/fileparse.php/5606/urlt/2015ChoiceOptionsBook.pdf
- Fok, D., Paap, R., & Van Dijk, B. (2010). A rank-ordered logit model with unobserved heterogeneity in ranking capabilities. Journal of Applied Econometrics, 27(5), 831–846. doi:10.1002/jae.1223
- Frankenberg, E., & Le, C. Q. (2008). The post parents involved challenge: Confronting extralegal obstacles to integration. Ohio State Law Journal, 69(5), 1015–1072.
- Glazerman, S. M. (1998, April 13–17). School quality and social stratification: The determinants and consequences of parental school choice. Paper presented at the Annual Meeting of the American Educational Research Association, San Diego, CA.
- Goldring, E., & Haussman, C. (1999). Reasons for parental choice of urban schools. Journal of Education Policy, 14(5), 469–490. doi:10.1080/026809399286161
- Goldring, E. B., & Phillips, K. J. R. (2008). Parent preferences and parent choices: The public–private decision about school choice. Journal of Education Policy, 23(3), 209–230. doi:10.1080/02680930801987844
- Groulx, T. (2016). Influences of segregation and desegregation on the bands at historically Black high schools of Hillsborough County, Florida. Journal of Historical Research in Music Education, 37(2), 129–149. doi:10.1177/1536600616638793
- Haan, M. D., Gautier, P., Oosterbeek, H., & Klaauw, B. V. D. (2015). The performance of school assignment mechanisms in practice. London, UK: Centre for Economic Policy Research.
- Hamilton, L., & Guin, K. (2005). Understanding how families choose schools. In J. Betts & T. Loveless (Eds.), Getting choice right: Ensuring equity and efficiency in education policy (pp. 40–60). Washington, DC: Brookings Institution Press.
- Harris, D. N., & Larsen, M. (2014). What schools do families want (and why)? School demand and information before and after the New Orleans post-Katrina school reforms. In T. U. Education Research Alliance for New Orleans (Series ed., pp. 66). Retrieved from http://educationresearchalliancenola.org/files/publications/Technical-Report-Final-Combined.pdf
- Hastings, J., Kane, T. J., & Staiger, D. (2005). Parental preferences and school competition: Evidence from a public school choice program (Working Paper 11805). Retrieved from http://www.nber.org/papers/w11805 doi:10.3386/w11805
- Hastings, J. S., Kane, T. J., & Staiger, D. O. (2009). Heterogeneous preferences and the efficacy of public school choice. Retrieved from http://people.virginia.edu/~sns5r/microwkshp/hastings.pdf
- Hausman, C., & Goldring, E. (2000). Parent involvement, influence, and satisfaction in Magnet schools: Do reasons for choice matter? The Urban Review, 32(2), 105–121. doi:10.1023/A:1005121214860
- Hausman, D. (2011). Preference, value, choice, and welfare. Economics and Philosophy, 30(1), 99–103. doi:10.1017/CBO9781139058537
- Hausman, J. A., & Ruud, P. A. (1987). Specifying and testing econometric models for rank-ordered data. Journal of Econometrics, 34(1–2), 83–104. doi:10.1016/0304-4076(87)90068-6
- Haynes, K. E., & Fotheringham, S. (1990). The impact of space on the application of discrete choice models. The Review of Regional Studies, 20(2), 39–49.
- Henig, J. R. (1990). Choice in public schools: An analysis of transfer requests among Magnet schools. Social Science Quarterly, 71(1), 69–82.
- Ibraimovic, T., & Hess, S. (2017). Changes in the ethnic composition of neighbourhoods: Analysis of household’s response and asymmetric preference structures. Paper in Regional Science, 96(4), 759–784. doi:10.1111/pirs.12230
- Jacobs, N. (2011). Understanding school choice. Education and Urban Society, 45(4), 459–482. doi:10.1177/0013124511413388
- Kelleher, L., Smyth, A., & McEldowney, M. (2016). Cultural attitudes, parental aspirations, and socioeconomic influence on post-primary school selection in Northern Ireland. Journal of School Choice, 10(2), 200–226. doi:10.1080/15582159.2016.1153378
- Koedel, C., Betts, J. R., Rice, L. A., & Zau, A. C. (2009). The integrating and segregating effects of school choice. Peabody Journal of Education, 84(2), 110–129. doi:10.1080/01619560902810096
- Koppelman, F. S., & Bhat, C. (2006). A self instructing course in mode choice modeling: Multinomial and nested logit models. Retrieved from http://www.ce.utexas.edu/prof/bhat/COURSES/LM_Draft_060131Final-060630.pdf
- Lee Lauen, D. (2009). To choose or not to choose: High school choice and graduation in Chicago. Educational Evaluation and Policy Analysis, 31(3), 179–199. doi:10.3102/0162373709339058
- Long, J. S., & Freese, J. (2006). Regression models for categorical dependent variables using Stata (2nd ed.). College Station, TX: Stata Press.
- Manna, P. F. (2002). The signals parents send when they choose their children’s schools. Educational Policy, 16(3), 425–447. doi:10.1177/08904802016003004
- Massey, D. S., & Denton, N. A. (1998). American apartheid: Segregation and the making of the underclass. Cambridge, MA: Harvard University Press.
- McFadden, D. (1973). Chapter 4: Conditional Logit analysis of qualitative choice behavior. In P. Zarembka (Ed.), Frontiers in econometrics (pp. 105–142). New York: Wiley.
- Müller, S., Haase, K., & Kless, S. (2009). A multiperiod school location planning approach with free school choice. Environment & Planning A, 41(12), 2929–2945. doi:10.1068/a40285
- Müller, S., Haase, K., & Seidel, F. (2012). Exposing unobserved spatial similarity: Evidence from German school choice data. Geographical Analysis, 44(1), 65–86. doi:10.1111/j.1538-4632.2011.00836.x
- Musset, P. (2012). School choice and equity: Current policies in OECD countries and a literature review. OECD Education Working Papers, 51. doi:10.1787/5k9fq23507vc-en
- Neild, R. (2005). Parent management of school choice in a large urban district. Urban Education, 40(3), 270–297. doi:10.1177/0042085905274538
- Poder, K., Lauri, T., Ivaniushina, V., & Alexandrov, D. (2016). Family background and school choice in cities of Russia and Estonia: Selective agenda of the Soviet past and present. Studies of Transition States and Societies, 8(3), 5–28.
- Ritter, G. W., Jensen, N. C., Kisida, B., & Bowen, D. H. (2016). Urban school choice and integration: The effect of charter schools in Little Rock. Education and Urban Society, 48(6), 535–555. doi:10.1177/0013124514546219
- Rossell, C. (1991). The carrot or the stick for school desegregation policy: Magnet schools or forced busing. Social Forces, 69(3), 943–946. doi:10.2307/2579501
- Saporito, S. (2003). Private choices, public consequences: Magnet school choice and segregation by race and poverty. Social Problems, 50(2), 181–203. doi:10.1525/sp.2003.50.2.181
- Saultz, A., Fitzpatrick, D., & Jacobsen, R. (2015). Exploring the supply side: Factors related to charter school openings in NYC. Journal of School Choice, 9(3), 446–466. doi:10.1080/15582159.2015.1028829
- Scafidi, B., & Kelly, J. (2013). More than scores: An analysis of why and how parents choose private schools. Retrieved from https://www.edchoice.org/research/more-than-scores/
- Schelling, T. C. (1969). Models of segregation. The American Economic Review, 59(2), 488–493.
- Schneider, M., & Buckley, J. (2002). What do parents want from schools? Evidence from the Internet. Educational Evaluation and Policy Analysis, 24(2), 133–144. doi:10.3102/01623737024002133
- Shi, P. (2015). Guiding school-choice reform through novel applications of operations research. Interfaces, 45(2), 117–132. doi:10.1287/inte.2014.0781
- Shircliffe, B. J. (2002). Desegregation and the historically Black high school: The establishment of Howard W. Blake in Tampa, Florida. The Urban Review: Issues and Ideas in Public Education, 34(2), 135–158. doi:10.1023/A:1015362316709
- Smrekar, C. (2009). Beyond the tipping point: Issues of racial diversity in magnet schools following unitary status. Peabody Journal of Education, 84(2), 209–226. doi:10.1080/01619560902810153
- Smrekar, C., & Honey, N. (2015). The desegregation aims and demographic contexts of magnet schools: How parents choose and why siting policies matter. Peabody Journal of Education, 90(1), 128–155. doi:10.1080/0161956x.2015.988545
- Sohoni, D., & Saporito, S. (2009). Mapping school segregation: Using GIS to explore racial segregation between schools and their corresponding attendance areas. American Journal of Education, 115(4), 569–600. doi:10.1086/599782
- Stewart, T., & Wolf, P. J. (2014). The school choice journey: School vouchers and the empowerment of urban families. New York, NY: Palgrave MacMillan.
- Stretesky, P. B., & Lynch, M. J. (2002). Environme/ntal hazards and school segregation in Hillsborough County, Florida, 1987–1999. The Sociological Quarterly, 43(4), 553–573. doi:10.1111/j.1533-8525.2002.tb00066.x
- Sude, Y., DeAngelis, C. A., & Wolf, P. J. (2018). Supplying choice: An analysis of school participation decisions in voucher programs in Washington, DC, Indiana and Louisiana. Journal of School Choice–International Research and Reform., 12(1), 8–33. doi:10.1080/15582159.2017.1345232
- Taylor, K., Phillips, K., & Goldring, E. (2010). Latino parents’ choice of magnet school: How school choice differs across racial and ethnic boundaries. Education and Urban Society, 42(6), 758–789. doi:10.1177/0013124510370943
- Teske, P., Fitzpatrick, J., & O’Brien, T. (2009). Drivers of choice: Parents, transportation and school choice. Seattle, Washington: Center on Reinventing Public Education (CRPE), University of Washington Bothell.
- Teske, P., & Schneider, M. (2001). What research can tell policymakers about school choice. Journal of Policy Analysis and Management, 20(4), 609–631. doi:10.1002/pam.1020
- Train, K. E. (2009). Chapter 7–Variations on theme: Ranked data. In K. E. Train (Ed.), Discrete choice methods with simulation (2nd ed., pp. 173–182). New York, NY: Cambridge University Press.
- West, A., & Noden, P. (2000). School reform in England: Increasing choice and diversity through specialist schools? Paper presented at the Annual Meeting of the American Educational Research Association, New Orleans, LA. Retrieved from http://files.eric.ed.gov/fulltext/ED445417.pdf
- Willie, C., Edwards, R., & Alves, M. (2002). Student diversity, choice, and school improvement. WestPort, CT: Bergin & Garvey.
- Wilson, E. J., Marshall, J., Wilson, R., & Krizek, K. J. (2010). By foot, bus or car: Children’s school travel and school choice policy. Environment and Planning, 42(9), 2168–2185. doi:10.1068/a435
- Wolf, P. J. (2008). School voucher programs: What the research says about parental school choice. Brigham Young University Law Review, 2(9), 415–446.
- Wolf, P. J., Kisida, B., Gutmann, B., Puma, M., Eissa, N., & Rizzo, L. (2013). School vouchers and student outcomes: Experimental evidence from Washington, DC. Journal of Policy Analysis and Management, 32(2), 246–270. doi:10.1002/pam.21691
- World Bank Group. (2011). Learning for all: Investing in people’s knowledge and skills to promote development. Washington, D.C.: World Bank Group Education Strategy 2020.
- Zeehandelaar, D., & Winkler, A. M. (2013). What parents want: Education preferences and trade-offs. A National Survey of K-12 parents. Retrieved from https://edexcellence.net/publications/what-parents-want.html
- Zimmerman, J. M., & Vaughan, D. Y. (2013). School choice outcomes in post-Katrina New Orleans. Journal of School Choice, 7(2), 163–181. doi:10.1080/15582159.2013.788959