Abstract
Methyl-Seq was recently developed as a targeted approach to assess DNA methylation (DNAm) at a genome-wide level in human. We adapted it for mouse and sought to examine DNAm differences across liver and 2 brain regions: cortex and hippocampus. A custom hybridization array was designed to isolate 99 Mb of CpG islands, shores, shelves, and regulatory elements in the mouse genome. This was followed by bisulfite conversion and sequencing on the Illumina HiSeq2000. The majority of differentially methylated cytosines (DMCs) were present at greater than expected frequency in introns, intergenic regions, near CpG islands, and transcriptional enhancers. Liver-specific enhancers were observed to be methylated in cortex, while cortex specific enhancers were methylated in the liver. Interestingly, commonly shared enhancers were differentially methylated between the liver and cortex. Gene ontology and pathway analysis showed that genes that were hypomethylated in the cortex and hippocampus were enriched for neuronal components and neuronal function. In contrast, genes that were hypomethylated in the liver were enriched for cellular components important for liver function. Bisulfite-pyrosequencing validation of 75 DMCs from 19 different loci showed a correlation of r = 0.87 with Methyl-Seq data. We also identified genes involved in neurodevelopment that were not previously reported to be differentially methylated across brain regions. This platform constitutes a valuable tool for future genome-wide studies involving mouse models of disease.
Abbreviations
Apcdd1 | = | Adenomatous Polyposis Coli Down-Regulated 1 |
ChIP | = | Chromatin immunoprecipitation |
DMCs | = | Differentially methylated cytosines (DMCs) |
DMRs | = | Differentially methylated regions |
DNAm | = | DNA methylation |
FDR | = | False discovery rate |
GFAP | = | Glial fibrillary acidic protein |
Gb | = | Gigabases |
GO | = | Gene ontology |
H3K4me1 | = | Histone marks histone 3 lysine 4 monomethylation |
H3K27ac | = | Histone 3 lysine 27 acetylation |
KEGG | = | Kyoto Encyclopedia of Genes and Genomes |
MAP | = | Mitogen activated protein |
Msx1 | = | msh homeobox1 |
PAVIS | = | Peak Annotation and Visualization |
RV | = | Range of variation |
TFBS | = | Transcription factor binding sites |
UTR | = | Untranslated regions |
Introduction
DNA methylation (DNAm) is an epigenetic mechanism that has been observed to contribute to a variety of phenotypes. Studies have noted its role in developmentCitation1 and its contribution to cellCitation2,3 and tissue-specificCitation4,5 differentiation. DNAm patterns have also been observed to vary between individuals.Citation6,7 Importantly, DNAm is not static, but can be altered by environmental influences, and thus provides a mechanism through which external stimuli or exposures can affect phenotypes. For example, studies on early life stressors have observed that changes to DNAm patterns in genes related to the hypothalamic-pituitary-adrenal axis, which is involved in the physiological stress response, are associated with anxiety and mood disorders.Citation8,9 Further, abnormalities in the DNAm pattern can also contribute to a variety of other diseases, including cancer and imprinting disorders, such as Prader-Willi syndrome and Angelman syndrome.Citation10 Due to its involvement in both development and disease, a variety of methods have been developed to study DNAm at a genome-wide level.
Currently, 3 different approaches are commonly used in conjunction with next-generation sequencing platforms to investigate genome-wide DNAm patterns. These approaches rely on either protein-affinity enrichment of methylated regions, bisulfite conversion, or methylated cytosine-sensitive restriction enzymes. Although widely used, these methods suffer from several limitations. For example, protein affinity-based enrichment of methylated cytosine approaches have been reported to differentially enrich for regions by varying CpG density, and they do not provide base-pair resolution of methylated cytosines.Citation11,12 In contrast, methods using methylated cytosine-sensitive restriction enzymes can provide base-pair resolution, but enrich for CpG dense regions.Citation11 Although these 2 approaches can be used complementarily, limited starting material and increased cost of sequencing may be prohibitive. An alternative unbiased method to detecting methylated CpGs at base-pair resolution is whole genome bisulfite sequencing. However, for this approach, obtaining adequate sequencing depth requires a substantial elevation in sequencing cost. In addition, 70–80% of the sequenced reads do not provide useful information on DNA methylation.Citation13 Given these limitations, a method that provides cost-effective detection of CpG methylation at base-pair resolution without bias of CpG dense and poor regions would have advantages for the study of genome-wide DNAm. Genome-wide targeted capture technology offers these advantages.
The genome-wide targeted capture approach uses DNA or RNA baits that contain complementary sequences of targeted regions to enrich these candidate regions for next-generation sequencing. This approach has been widely used in exome sequencing studiesCitation14,15 to identify rare variations. It has more recently been adapted to investigate DNAm at base-pair resolution through bisulfite conversion of the human genome.Citation16,17 As baits can be designed against regions of interest, this eliminates any bias for CpG dense or poor regions while enabling sequencing reactions to be focused on regions of interest, thus improving yield per sequencing cost. Despite these benefits, only a small number of studies to date have used this approach in humans,Citation16,17 and no study has yet used it in mouse. This study describes the adaptation of Methyl-Seq for the mouse methylome and demonstrates its ability to reliably detect DNAm by investigating differences between tissue types. It has also revealed novel tissue-specific differences in DNAm patterns of genes involved in neurodevelopment. This is currently the only bait-based assay for the mouse methylome. This study provides the foundation for future use of this assay in studying genome-wide DNAm signatures in mouse models of disease.
Results
Single-base resolution maps of 5-methylcytosines from targeted bisulfite sequencing of liver and cortex and hippocampus
Single base-pair resolution maps of 5-methylcytosine was generated by bisulfite-sequencing of targeted captured DNA from 3 different tissues (liver, cortex, and hippocampal) each performed in triplicate. Genomic regions captured by this assay are summarized in . This generated an average of ∼81 million, 77 million, and 77 million reads for cortex, hippocampus, and liver, respectively, which uniquely aligned to the bisulfite-converted mouse reference genome (mm9/NCBI37). Eighty-five percent (cortex), 67% (hippocampus) and 70% (liver) of aligned reads remained after deduplication, which covered ∼75% of targeted regions. Approximately 25% of targeted regions had zero coverage, suggesting that baits were unable to isolate these regions. From these filtered reads, 6.4 gigabases (Gb) (cortex), 4.9 Gb (hippocampus) and 5.0 Gb (liver) were present. The mean coverage of targeted regions was 54× (cortex), 42× (hippocampus) and 44× (liver). About 73% of targeted bases were observed to have coverage of at least 10×. As a measure of efficiency of the targeted capture assay, targeted regions were observed to be ∼20-fold more abundant than genomic background for all tissues. An overview of these results is described in .
Table 1. Mouse methylome target capture design. There was approximately 10 Mb of target overlap between data repositories resulting in a final hybridization capture array targeting 99 Mb across the murine genome. TFBS: transcription factor binding sites.
Table 2. Overview of Methyl-Seq data for different tissues. Values shown are the average of 3 independent samples from each tissue. Values shown were obtained from Picard HsMetrics and FastQC analysis. Filtering mentioned in this table involves deduplication of aligned reads.
We report here on our evaluation of tissue-specific differentially methylated cytosines (DMCs) in the CpG context. A total of ∼1.3 million CpGs were commonly captured in all tissues with a minimum coverage of 10× and good quality base calls (< Q30). Correlation analysis showed a tight reproducibility of methylation levels between replicates of cortex (Pearson's r = 0.97, P < 2.2 × 10−16) (), hippocampus (Pearson's r = 0.97, P < 2.2 × 10−16) (), and liver (Pearson's r = 0.96, P < 2.2 × 10−16) (). A high correlation in methylation was observed between cortex and hippocampus (Pearson's r = 0.98, P < 2.2 × 10−16) (). In contrast, reduced correlation was observed between brain tissues and the liver (Pearson's r = 0.84, P < 2.2 × 10−16) (). About 85% of CpGs showed some degree of methylation in both brain and liver tissues. Between tissues, CpG methylation was observed to be significantly higher in the cortex (42% ± 35%, mean ± SD) than hippocampus (40% ± 35%, mean ± SD) (P = 1.15 × 10−11). CpG methylation in both brain tissues were higher than in the liver (38% ± 34%, mean ± SD) (P < 2.2 × 10−16). We also analyzed tissue differences using hierarchical clustering and principle component analysis approaches. These, seen in Figure S1 (A–B), further demonstrated distinct tissue-specific clustering of methylation patterns. A histogram reflecting the variance within each tissue type is provided in Figure S2 (A–C).
Figure 1. Reproducibility of cortex, hippocampus, and liver replicates. (A) Cortex. (B) Hippocampus. (C) Liver. Figures shown are scatter plots of comparisons between DNA methylation levels of tissue replicates. Data shown are a representative of one of the 3 comparisons between replicates of each tissue.
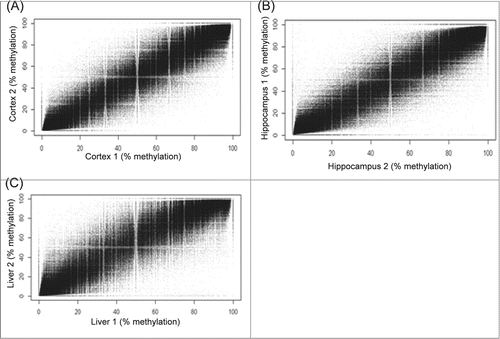
Figure 2. Comparison of DNA methylation level between different tissues. (A) Hippocampus vs. Cortex. (B) Cortex vs. Liver (C) Hippocampus vs. Liver. Figures shown are scatter plots between different tissues using average DNA methylation levels from triplicates of each tissue tissue.
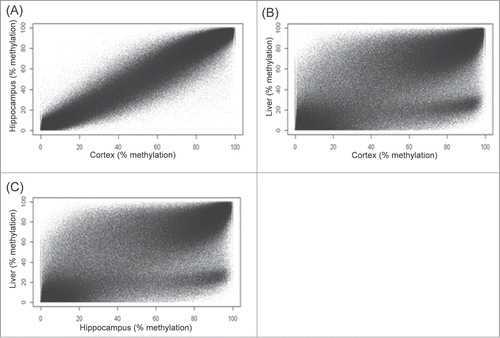
To determine the technical variation for this assay, targeted capture followed by bisulfite-sequencing was performed on DNA derived from cortex and liver in technical triplicates. Sequencing data was aligned to the bisulfite-converted mouse genome and deduplicated as mentioned above. Methylated cytosines in targeted regions with a minimum coverage of 10× and of good base quality (<Q30) across triplicates were used to determine the range of variation (RV) between technical triplicates. This was calculated by determining the difference between the maximum and minimum methylation level for every methylated CpG. The RV was similar across the different tissues (10% ± 10%, median ± IQR). An RV in the 95th percentile, reflecting a range of 27%, was used as a threshold for detecting tissue-specific methylation differences as this removes 95% of technical variation from the assay. Using these screening criteria, tissue-specific DMCs in the CpG context were identified between hippocampus and liver (N = 186,213), cortex and liver (N = 197,519), and cortex and hippocampus (N = 14,519) respectively. The absolute methylation difference for DMCs in the CpG context was not significantly different between the cortex vs. liver (44% ± 13%, mean ± SD) as compared to the hippocampus vs. liver (44% ± 13%, mean ± SD) after Bonferroni correction for multiple testing. However, the absolute methylation difference was significantly higher (P < 2.2 × 10−16) for these 2 comparisons as compared to the cortex vs. hippocampus (33% ± 6%, mean ± SD).
Distribution of differentially methylated cytosines in genomic and CpG contexts
Previous studies have shown that the distribution of DMCs between cell and tissue types can vary between genomic contexts.Citation4,18 To determine the genomic distribution of DMCs among these regions, DMCs were assigned to their targeted capture baits that had been mapped to these functional genomic contexts. For all tissue comparisons, DMCs in the CpG context were present in significantly greater numbers than expected in the introns and intergenic regions downstream of genes (≥1 bp downstream of transcriptional termination site as previously describedCitation19) (). For 3′ untranslated regions (UTR), DMCs in the CpG context were present in significantly greater numbers than expected in the brain vs. liver comparisons, but not in the cortex vs. hippocampus comparison. In contrast, DMCs in promoters, exons, and 5′ UTR were significantly fewer than expected in all tissue comparisons (). Likewise, DMCs in upstream intergenic (>2,000 bps upstream of transcriptional start site) regions were present in significantly smaller numbers than expected in the brain vs. liver comparisons, but not in the cortex vs. hippocampus comparison which showed no significant difference between observed and expected numbers (). Similarly, the distribution of DMCs was assessed according to previously defined CpG contexts, namely CpG islands, CpG shores, CpG shelves, and CpG open sea.Citation18,20 The fewest DMCs were observed in CpG islands for all tissue comparisons, and these numbers were significantly smaller than expected (). In contrast, more DMCs were present in CpG contexts outside of CpG islands such as CpG shores, CpG shelves and CpG open sea, and these numbers were significantly greater than expected (). However, between cortex and hippocampus, the number of DMCs in CpG shores was significantly less than expected ().
Figure 3. Distribution of DMCs in the CpG context across genomic annotations. Chi-Square was performed to determine if the number of observed DMCs deviated from the expected number of DMCs for each genomic category. (A) Hippocampus vs. Liver, (B) Cortex vs. Liver, and (C) Hippocampus vs. Cortex. ***: P < 0.001, N.S.: not significant. P-values remained significant after Sidak's post-hoc multiple comparison test.
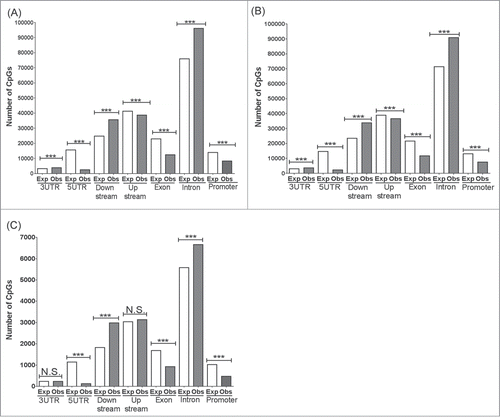
Figure 4. Distribution of DMCs across CpG contexts. Chi-Square was performed to determine if the number of observed DMCs deviated from the expected number of DMCs for each CpG context. (A) Hippocampus vs. Liver, (B) Cortex vs. Liver and (C) Hippocampus vs. Cortex. ***: P < 0.0001. P-values remained significant after Sidak's post-hoc multiple comparison test.
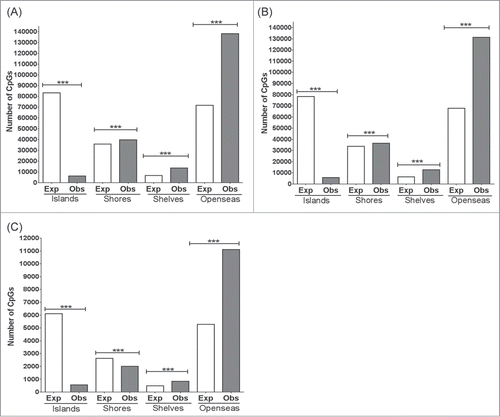
Functional annotation of differentially methylated cytosines between liver and brain tissues
To determine how tissue-specific DMCs might contribute to cellular differences between brain and liver tissues, DMCs in the CpG contexts were assigned to the nearest gene and analyzed by gene ontology (GO) term enrichment of cellular components. After correcting the enrichment P-values for multiple testing, genes that were more methylated in the CpG context in the liver compared to cortex and hippocampus had significant enrichment of GO terms for neuronal components, as would be expected given the correlation of increased methylation with reduced expression and thus functional deactivation. These included (only cortex vs. liver result is shown for simplicity): neuronal projection (P = 5.9 × 10−17), microtubule cytoskeleton (P = 9.5 × 10−9), synapse part (P = 1.1 × 10−10), dendrite (P = 5.8 × 10−10), postsynaptic density (P = 3.3 × 10−7), and axon (P = 1.3 × 10−5). In line with these cellular differences, molecular signaling pathways were also observed to be different between the liver and brain tissues. Pathway analysis showed that genes which were more methylated in the CpG context in the liver were significantly enriched in signaling pathways known to be involved in neuronal function including the mitogen activated protein (MAP) kinase signaling pathway (P = 3.6 × 10−10), the Wnt signaling pathway (P = 1.7 × 10−9), axon guidance (P = 1.7 × 10−8), the Erb signaling pathway (P = 1.7 × 10−6), and the neurotrophin pathway (P = 1.9 × 10−5). These differences remained significant after multiple corrections.
Distribution of differentially methylated cytosines between enhancer regions
Many studies have shown that enhancers play an important role in regulating tissue-specific gene expression. To determine if differential methylation patterns at enhancer regions contribute to phenotypic differences, the distribution of DMCs were mapped to enhancers shared between the cortex and liver, cortex-specific enhancers, liver-specific enhancers, and regions outside of these categories. Enhancers were identified using the histone marks histone 3 lysine 4 mono-methylation (H3K4me1) and histone 3 lysine 27 acetylation (H3K27ac) > 2 kb from the transcriptional start site.Citation21,22 Histone marks based on cortex and liver of adult C57/Bl6 mice were retrieved from the UCSC Genome Browser. DMCs were present in significantly greater numbers than expected in enhancers shared between the cortex and liver (P = 2.6 × 10−15) (), cortex-specific enhancers (P < 1.0 × 10−15) () and liver-specific enhancers (P = 1.0 × 10−15) (). In contrast, DMCs were present in significantly lower numbers than expected in regions outside of these enhancer categories (). Of those DMCs mapped to cortex-specific enhancers, 86% were more highly methylated in the liver, while 14% were more highly methylated in the cortex. Similarly, of those DMCs mapped to liver-specific enhancers, 96% were more highly methylated in the cortex, while 4% were more highly methylated in the liver. Of those present in enhancers shared between the cortex and liver, 68% were more highly methylated in the cortex while 32% were more highly methylated in the liver. We did not include the hippocampus for this analysis, as the data for H3K4me1 and H3K27ac marks for adult C57/Bl6 hippocampus is unavailable.
Figure 5. Distribution of DMCs across enhancer regions. Chi-Square was performed to determine if the number of observed DMCs deviated from the expected number of DMCs for each enhancer context. (A) Enhancers shared between cortex and liver. (B) Enhancers present in the cortex. (C) Enhancers present in the liver. (D) Enhancers in other regions. ***: P < 0.0001. P-values remained significant after Sidak's post-hoc multiple comparison test.
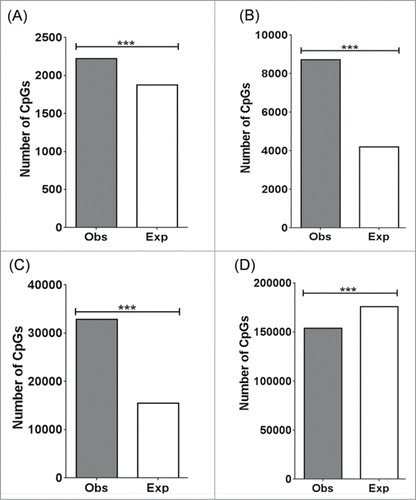
Validation of differentially methylated cytosines by bisulfite-pyrosequencing
To evaluate whether Methyl-Seq reliably detects DNAm differences between tissues, a selection of methylated CpGs that were the most highly statistically significant were interrogated by bisulfite-pyrosequencing using Methyl-Seq samples. CpGs investigated in this study were selected from regions containing clusters of DMCs, i.e., differentially methylated regions (DMRs), ranked by number of DMCs (). As expected, comparison of these DMRs to histone modifications (H3K4me1, H3K4me3 and H3K27ac) and DNase I hypersensitive sites annotated in the UCSC Genome Browser showed that every one of the 19 regions contained hallmarks of at least on of these regulatory genomic features.Citation23 A good correlation in DNAm differences between tissues was observed between Methyl-Seq and bisulfite-pyrosequencing data (Pearson's r = 0.84, P < 2.2 × 10−16). In a separate experiment, another cohort of mouse tissues was used to validate tissue-specific DMCs detected by Methyl-Seq. From a total of 75 tissue-specific DMCs, ∼86% showed significant tissue-specific DNAm differences; all in the same direction as observed by Methyl-Seq (), with an overall correlation of 0.87 (P < 2.2 × 10−16) when comparing tissue-specific DNAm differences between the 2 platforms. There was, however, variation in the magnitude of tissue-specific differences between the 2 platforms. For example, tissue-specific methylation differences observed in Gm13345 and Zfp2865 varied by ∼8% between Methyl-Seq and bisulfite-pyrosequencing detection. Tissue-specific methylation differences observed in Sox9 showed variation of 17%–24% between Methyl-Seq and bisulfite-pyrosequencing. The average variation detected between the 2 approaches was 20% ± 12% (mean ± SD). To determine the relationship between methylation levels and gene expression levels for validated differentially methylated genes, expression data from the mouse cortex, hippocampus and liver from GNF Expression Atlas 2 was obtained using the UCSC Genome Browser. Expression data was available for 15 of the 17 validated genes. Of the 15 genes, 11 genes showed reduced gene expression in tissues that had increased methylation. In contrast, 4 genes (Neat1, Nr1d1, Espn, Potla) did not follow this trend. Neat1 did not show any difference in gene expression between the cortex and liver even though the cortex was more highly methylated than the liver. Similarly, Pot1a did not show any difference in gene expression between the cortex and hippocampus even though the hippocampus was more highly methylated than the cortex. Nr1d1 and Espn showed higher expression in the liver than cortex although their methylation level was higher in the liver.
Table 3. Differentially methylated regions (DMRs) identified from Methyl-Seq for bisulfite-pyrosequencing validation. Differentially methylated cytosines (DMCs) were clustered as DMRs and ranked by number of DMCs; DMC density is calculated by the number of DMCs divided by the length of the DMR.
Table 4. Comparison of Methyl-Seq with bisulfite-pyrosequencing validation. Bisulfite pyrosequencing was performed on a separate cohort of liver and brain tissues at CpG dinucleotide locations described above. (A) Cortex vs Liver, (B) Hippocampus vs Liver and (C) Cortex vs. Hippocampus data shown of average percentage methylation are mean ± SEM of methylation level detected at Cs in the CpG context at the corresponding location in cortical and hippocampal tissues. Methyl-Seq, N = 3. Bisulfite-pyrosequencing, N = 8. P-values for Methyl-Seq and bisulfite-pyrosequencing data were corrected for multiple testing by the sliding linear model method and Holm-Šídák test, respectively.
Brain region-specific methylation differences
In the Methyl-Seq experiment, a total of 14,519 DMCs were identified in the comparison between cortex and hippocampus. Our validation work described above examined regions in 9 genes that showed differences in methylation between these 2 brain regions. Significant differences by bisulfite pyrosequencing were observed in 7 of these. More methylation was seen in cortex than hippocampus for CpGs in 5 genes: Prtg, Apcdd1, Gm13345, and Zfp825, and Cmtm1. Conversely, more methylation was seen in hippocampus than cortex for CpGs in 2 genes: Cacbn4 and Pot1a.
Discussion
Mice are commonly used to model different paradigms of disease. To this end, Methyl-Seq baits were developed to enable a targeted approach to studying patterns of the mouse methylome. To determine the validity of this assay, we investigated DNAm patterns between tissues that are functionally and structurally different from each other, with the expectation that such distinct tissues would maximize the observed methylation differences. Indeed, data from Methyl-Seq did reveal about 10 times more DMCs and a larger magnitude of difference between the brain and liver tissues compared to differences between the cortex and hippocampus, which are more similar. Strikingly, a disproportionate number of these DMCs reside in intronic and intergenic regions. Similar observations have been reported in previous genome-wide DNAm studies that investigated cell-type and tissue-specific DNAm differences.Citation4,18 Similarly, DMCs were also observed predominantly outside of CpG islands in CpG shores, shelves and open sea, as has been similarly observed in other genome-wide DNAm studies.Citation4,18,20 Together, these observations suggest that changes to DNAm patterns in introns, intergenic regions, and in regions outside of CpG islands may play an important role in tissue-specific expression of genes. Importantly, this study highlights that the current mouse Methyl-Seq assay can adequately capture regions where DNAm differences are present between tissues.
Although we did not investigate the function of these non-coding regions, many other studies have clearly demonstrated the involvement of non-coding regions in mediating tissue-specific gene expression. For example, an intron present in a gene ∼1 Mb upstream of the sonic hedgehog gene was shown to mediate limb bud expression of sonic hedgehog.Citation24 In an independent study, a conserved non-coding element ∼42 kb upstream of the galanin gene was observed to drive promoter expression in the paraventricular nucleus, arcuate nucleus and amygdala where the galanin peptide is expressed.Citation25 Our assay was designed to capture such genomic regions with putative regulatory function, and the finding that many of them contain tissue-specific DMCs suggests that DNAm may influence tissue-specific expression of their corresponding target genes. Indeed, the DMRs within which validated DMCs are located contain histone modifications and DNase I hypersensitivity sites, which are hallmarks of transcriptional regulatory features.Citation23 Furthermore, liver-specific enhancers, where regions are enriched for histone marks of active enhancers H3K4me1 and H3K27ac in the liver but depleted in the cortex, are highly methylated in the cortex. Likewise, cortex-specific enhancers are highly methylated in the liver. Interestingly, enhancers that are commonly shared between tissues also displayed tissue-specific methylation differences. This suggests that tissue-specific differences may not only be mediated by the suppression of tissue-specific enhancers, but may also involve varying methylation patterns within shared enhancers.
To demonstrate the utility of the new Methyl-Seq assay, we performed tests of gene ontology (GO) annotation enrichment on differentially methylated genes to determine whether they were enriched for terms that reflect the cellular identity of brain and liver tissues. This was indeed observed. Genes that are hypomethylated in the brain are distinctly enriched in neuronal components. In contrast, genes that are hypomethylated in the liver are enriched in cellular components prevalent in the liver, such as the microbody, or that play a role in the metabolic function of the liver. Similarly, pathway analyses also show that genes that are hypomethylated in the brain are enriched in signaling pathways involved in neuronal function. For example, the Wnt signaling and neurotrophin pathways have been shown to play a role in neurogenesis and arborization of neurites, which are important cellular processes that affect behavior and cognition.Citation26,27 Likewise, the Erb signaling pathway has been observed to mediate specification of adult neural stem cells into oligodendrocyte lineage.Citation28 In contrast, genes that are hypomethylated in the liver are enriched in pathways involved in various liver functions including protein metabolism, haematopoietic cell lineage and complement and coagulation cascades. The reliability of Methyl-Seq at detecting DMCs was demonstrated by its high correlation with the same DMCs detected by bisulfite-pyrosequencing in a separate cohort of mice. In addition, genes that were validated as differentially methylated also generally showed decreased gene expression in tissues where they were more highly methylated. There were exceptions, suggesting that, for some of these genes, other factors, such as histone modifications, may be involved in controlling their expression. Together, these observations underscore the reliability of the assay at detecting differentially methylated cytosines.
As a separate goal, we aimed to extend prior observations about brain-specific differences.Citation4,29 Previous genome-wide DNAm studies have observed a number of tissue-specific differentially methylated genes in the brain involved in cellular mechanisms and signaling pathways, such as neurotransmission, neurotrophins and Wnt signaling, that have been implicated in neuropsychiatric disorders.Citation4,29,30 In this study, we have identified genes with a role in neurodevelopment that have not previously been reported to be differentially methylated across brain regions. For example, the Adenomatous Polyposis Coli Downregulated 1 (Apcdd1) gene is observed to be hypomethylated in the mouse hippocampus relative to the mouse cortex. This gene is a negative regulator of the Wnt signaling pathway and has a migratory role in astrogliogenesis.Citation30 Similarly, the msh homeobox1 (Msx1) gene is hypomethylated in the cortex relative to the hippocampus. Although this gene is known to be involved in orofacial development,Citation31 disruption of this gene has also been observed to impair brain development resulting in mice that have hydrocephalus at birth among other neuroanatomical defects.Citation32 Due to their involvement in brain development, it may be of interest to explore whether methylation changes in human to orthologous regions of the DMRs of these genes may be involved in neurodevelopmental disorders.
The present study has several limitations. First, while our Methyl-Seq data showed good consistency with bisulfite-pyrosequencing validation results, they were not in perfect agreement. The magnitude of difference in DNAm varied between methods. This likely reflects variation in methylation detection between platforms. It is also worth noting that the Methyl-Seq assay is designed to detect DNAm levels on the negative strand, which is a default design parameter of Agilent's SureSelect target enrichment system. Although every attempt is made to validate DNAm changes on the negative strand by bisulfite-pyrosequencing, this is not always possible due to limitations in primer designs with sequences of low complexity. Thus, it is possible that discrepancies between the 2 platforms may be due to the effect of hemi-methylation. None of the genes reported are, however, currently known to be hemi-methylated. Second, while our Methyl-Seq assay covers about 4% of the mouse genome with annotated regulatory features or with regions where differential methylation is known to occur, there remain many more regions where relevant DNAm variation between tissues might exist. These will likely only be covered when whole genome sequencing for methylation status becomes affordable. Third, due to the increasing awareness of methylation outside of the CpG context, an external control should be spiked into the Methyl-Seq library preps to provide accurate detection of bisulfite conversion error rates. This is presently not performed using the standard Agilent Methyl-Seq protocol. In this study, bisulfite conversion error rates were computed using methylation levels detected from random cytosines in the non-CpG context within the sample. Although a slightly elevated bisulfite error rate was observed in brain tissue compared to liver tissue (1.5% vs 0.8%), which is in line with elevated methylation levels of the non-CpG context in the brain,Citation33 this had little impact on our ability to accurately detect tissue-specific DMCs and DMRs, as observed from our robust validation result.
In summary, we have demonstrated the robustness of this platform at detecting DNAm differences between varying mouse tissues. We have also shown the ability of this platform to identify novel DMCs and DMRs. The platform may be a valuable tool in future studies for examining DNAm changes in various mouse models of disease including those involving exposure to environmental stimuli such as stress, medications and substance abuse.
Material and Methods
Tissue extraction from mice
Adult C57/Bl6 mice (>12 weeks) were purchased from Jackson Laboratories. Mice were sacrificed followed by immediate extraction of the brain and liver. Cortices and hippocampi were microdissected from the brain and retained for the study. For Methyl-Seq experiments 3 biological replicates of these tissues were used. For subsequent bisulfite-pyrosequencing validation, 8 biological replicates of these tissues were used from a separate mouse cohort.
DNA extraction
DNA extraction was performed using the MasterPureTM DNA extraction kit (Cat. No. MCD85201 Epicenter, an Illumina company, USA) as described in the manufacturer's protocol. Briefly, tissue was lysed in 600 μl of tissue and cell lysis solution with 2 μl of proteinase K (50 μg/μl). The reaction was briefly vortexed and incubated at 65°C for 30 min. The reaction was then incubated with 2 μl of RNase A (5 μg/μl) at 37°C for 30 min. After incubation at 4°C for 5 min, reaction was mixed with 350 μl of MPC protein precipitation reagent and centrifuged at 4°C for 10 min at 14,000 rpm. The supernatant was mixed with an equal volume of isopropanol and centrifuged at 4°C for 10 min at 14,000 rpm. The pellet was washed in 70% ethanol twice before air-drying for 10 min to remove ethanol residue prior to being dissolved in Tris-EDTA buffer.
Design of the custom RNA hybridization bait library
Recent studies have highlighted the importance of the non-coding genome for regulating gene expression.Citation34 We have designed baits to not only capture RefSeq genes, but to also include putative regulatory sequences, as DNA methylation patterns in both types of genomic regions may reveal important functionally relevant information. The commercially available Agilent SurePrint® oligo manufacturing and SureSelect® hybridization target enrichment technology uses biotinylated 120 base pair RNA baits to selectively hybridize with complimentary DNA sequences that have been randomly fragmented. Agilent offers a fully customizable bait design option through their eArray® online utility (https://earray.chem.agilent.com/earray/). Using this utility, we designed a custom hybridization array that targets 99 Mb of CpG islands, shores, shelves and regulatory elements in the mouse genome based on criteria described below. This is the most comprehensive murine methylome hybridization capture sequencing array currently reported.
Using the publicly available databases from the National Center for Biotechnology Information (NCBI-NIH) (http://www.ncbi.nlm.nih.gov), the University of California Santa Cruz (UCSC) Genome Browser (http://genome.ucsc.edu) and Ensembl (European Bioinformatics Institute/Wellcome Trust Sanger Institute) (http://www.ensembl.org), genomic coordinates for all known CpG islands, shores and shelves in the mouse genome (mm9) were obtained. Verification of each coordinate set was carried out against previously published reports of the predicted CpG islands in the mouse genome.Citation35-41 Additionally, we included tissue-specific differentially methylated regions (DMRs),Citation20 DNase I hypersensitive sites, and regulatory elements such as promoters, enhancers and transcription factor binding sites (). The combined total mouse methylome design included ∼201,788 targets and ∼110 Mb of bases covered by 297,773 baits. Due to some overlap between target regions, the final product was 99 Mb. shows the composition of each of the categories included in the target design. Of the 297,773 baits that were designed, ∼75% of them successfully isolated targeted regions for sequencing. Of these, ∼51% were present in gene bodies covering 17,190 genes with an average of 80 CpGs per gene. This capture library can be obtained from Agilent (Cat. No. 931052). An annotated table of each bait probe can be found in Table S1. Table S2 contains a list of probe baits that did not generate usable data.
Methyl-Seq library preparation
Methyl-Seq was performed using Agilent SureSelect Methyl-Seq kit (Cat. No. G9651A) as described in the manufacturer's protocol (Methyl-Seq protocol, version A.1, April 2012). Briefly, 3 μg of DNA was used for the library prep. From this, >550 ng of adaptor ligated DNA was used for the hybridization capture and the final concentration of indexed library was 8–15 nM. The following changes to minimize loss of DNA in the process of enzymatic reactions. AMPure XP beads (Cat. No. A63880, Beckman Coulter, USA) were incubated with DNA reaction mix for 10 min at room temperature prior to pelleting by magnetization. AMPure XP beads were then washed twice with 80% ethanol and dried at 37°C for 5 min. DNA was dissolved in water for 10 min at 37°C. The amount of water used for dissolving is as described in the manufacturer's protocol. AMPure XP beads were retained in solution for adenylation and end-repair reaction. After these reactions, an equal volume of binding buffer (20% PEG 8000/2.5M NaCl) was added to the reaction mix (1:1 ratio) to enable the AMPure XP beads to rebind to DNA.Citation42 The reaction was incubated at room temperature for 10 min. DNA was subsequently purified as described above. AMPure XP beads were not retained after this step. Concentration and sizes of DNA fragments after shearing, after adaptation ligation, and after indexing, were analyzed by Agilent Bioanalyzer 2100, and were observed to be within the range advised by the manufacturer's protocol. Greater than 550 ng of adaptor ligated DNA was used for the hybridization capture as described in the manufacturer's protocol. This protocol is described in detail in the supplementary material and methods. The Agilent protocol used in this study can be found here: http://www.medicine.uiowa.edu/psychiatry/SureSelect%20Methyl-Seq%20protocol%20version%20A.1.pdf
Bisulfite conversion
Bisulfite conversion was performed using EZ DNA Methylation-GoldTM (Zymo Research, USA) as described in the manufacturer's instructions. DNA was eluted in 12 μl of elution buffer.
Next-generation sequencing
Sequencing was performed by 100 bp paired-end sequencing by Illumina HiSeq2000 as described in the manufacturer's protocol. Quality and concentration of library preparation were determined by bioanalyzer using a high sensitivity DNA kit (Cat. No. 50674626, Agilent, USA) and a KAPA real-time PCR assay (Cat. No. #KK4835, KAPA biosystem, USA). For HiSeq, 3 samples were multiplexed in a lane to a final concentration of 12 pmole for sequencing. This provided a cluster density between 600–700 K/mm2. To overcome color imbalance inherent to low complexity in a bisulfite-converted genome, 1% of phiX genome was spiked into the reaction. In addition, a control lane containing regular genome that was not bisulfite converted was processed in the same flow cell as bisulfite converted libraries. Q30 scores of bases from HiSeq reactions were within the threshold recommended by the manufacturer.
Nested PCR for validation experiment
Validation of differentially methylated cytosines as detected by Methyl-Seq was performed by bisulfite pyrosequencing. To accomplish this we first designed nested PCR primer sets using Primer 343 and Pyromark assay design SW 2.0 (Cat. No. 9019077, Qiagen, USA). Due to the low complexity of bisulfite converted DNA, nested PCR was performed to promote specific amplification of targeted sequence. Primers were purchased from Integrated DNA Technology, USA. For inner PCR primer sets, one of the inner primers was biotinylated and HPLC purified as indicated in Table S3. Outer PCR amplification was performed with 1 μl of bisulfite converted DNA using either Taq polymerase with Thermopol® buffer (Cat. No. M0267S, New England Biolabs, USA) or the PyroMark PCR kit (Cat. No. 978703, Qiagen, USA). Inner PCR amplification was performed using 2 μl of outer PCR product. Thermal cycling conditions were performed as described in the manufacturers’ instructions. For thermopol PCR, annealing temperatures for outer and inner primer sets were 57°C and 53°C, respectively. For Pyromark PCR, both inner and primer sets were annealed at 56°C. The extension times for both PCR kits were 45 sec and 30 sec for inner and outer primer sets, respectively. The number of thermal cycles was 30 and 40 for inner and outer primer sets, respectively. The PCR products were electrophoresed on a 1% agarose gel to check for product specificity. Table S3 describes the primer sets used for the amplification.
Pyrosequencing
Pyrosequencing was performed using capillary dispensing tips on the PyroMark Q96 MD (Qiagen, USA) as described in the manufacturer's instructions. PyroMark CpG software (Cat. No. 9019067, Qiagen, USA) was used in this assay. Bisulfite conversion was shown to be efficient for all samples as the fluorescence signal by cytosine in a non-CpG context was ≤ 1% of the signal produced by thymine. Sequence specificity was also ascertained by introducing a base that is not in the target sequence. As an additional quality control, we investigated DNA methylation levels at the promoter of the glial fibrillary acidic protein (GFAP) gene in NeuN+ and NeuN- cells. NeuN+ cells showed higher methylation in the GFAP promoter than NeuN- cells (Fig. S3). This experiment demonstrated the reliability of the nested PCR bisulfite-pyrosequencing assay.
Data analysis
Bisulfite sequencing data from HiSeq were trimmed using Trim Galore! (http://www.bioinformatics.babraham.ac.uk/projects/trim_galore/) to remove standard Illumina paired-end adaptors using the settings –paired -t -q 30.Citation44 Data were subsequently aligned to the mouse genome mm9 using Bismark and Bowtie2 with default settings and deduplicated using a Bismark perl script.Citation45 Mapping efficiency was ∼84%. Bisulfite error rate was estimated from the non-CpG context giving an average of ∼0.9% in liver and 1.5% in cortex and hippocampus. These estimates are comparable to the bisulfite conversion error rate of ∼1% previously reported in other studies.Citation46 A higher bisulfite conversion error rate from brain tissues is expected as methylation in the non-CpG context has been observed to be elevated in the brain.Citation47,48 Data were subsequently analyzed using the R package MethylKit.Citation49 Only cytosines with a minimal coverage of 10× and phred quality score of Q30 in the CpG context were analyzed. The 5-methylcytosine maps and lists of tissue-specific differentially methylated cytosines can be found at http://www.medicine.uiowa.edu/Psychiatry/Cortex_Hippocampus_Liver_DNA_methylation_map.7z and http://www.medicine.uiowa.edu/Psychiatry/Tissue_specific_DMCs.7z, respectively.
Analysis of differential methylation
Differentially methylated cytosines (DMCs) were considered as cytosines whose difference in methylation level between 2 tissues was > 27% (see below for rationale in selecting this threshold), with the q-value for the difference ≤ 0.05. The initial P-values of DMCs were calculated using logistic regression by comparing the number of methylated reads (Cs) and unmethylated reads (Ts) between the different tissues. The depth of coverage (Cs and Ts) per CpG was ≥ 10× with a median of ∼40–50×, values that were consistent between tissue comparisons. P-values were converted to q-values by MethylKit using the sliding linear model method to correct for multiple comparisons. Differentially methylated regions (DMRs) were determined as targeted regions with > 1 DMCs. For DMR analysis, we began with the default approach used in MethylKit, in which the number of Cs and Ts for all CpGs in a targeted region were combined, with Ns again reflecting the number of reads per CpG. Counts (Cs and Ts) were then divided by the total number of CpGs in the targeted region to produce an average number of Cs and Ts for the region. The median depth of coverage for the averaged region was ∼40–50×, values that were consistent between tissue comparisons. P-values were then calculated by comparing the number of Cs and Ts between tissues using logistic regression as described above and corrected for multiple testing by false discovery rate (FDR). DNAm differences identified by bisulfite pyrosequencing were analyzed by either Student's t-test or one-way ANOVA with Sidak's post-hoc multiple comparison test using Graphpad prism. For comparisons of absolute methylation levels between different tissues, the Mann-Whitney test with Bonferroni correction was employed.
Annotation
Differentially methylated cytosines (DMCs) were mapped to different CpG contexts (i.e., CpG islands, CpG shores, CpG shelves and CpG open sea), genomic contexts (i.e., promoters, exons, introns, and intergenic regions) and enhancers by overlapping DMCs to targeted capture baits mapped to the CpG contexts, genomic contexts or enhancers. Coordinates of CpG islands from the mm9 genome were extracted from the UCSC Genome Browser using Galaxy.Citation50-52 CpG shores were identified as 2 kb regions flanking CpG islands as previously defined.Citation20 Likewise, CpG shelves were considered as regions between 2–4 kb flanking CpG shores,Citation53 while CpG open sea was considered as regions beyond CpG shelves.Citation18 The coordinates of promoters for the mm9 genome were extracted from the UCSC Genome Browser. Promoter coordinates were considered as 2 kb regions upstream of annotated transcription start sites of RefSeq genes with annotated 5′ untranslated regions. Baits that do not overlap promoter coordinates were mapped to exons, introns and intergenic regions using Peak Annotation and Visualization (PAVIS).Citation19 Coordinates of cortex and liver enhancers were retrieved from the UCSC Genome Browser defined by peaks of histone 3 lysine 4 mono-methylation (H3K4me1) and histone 3 lysine 27 acetylation (H3K27ac) obtained from chromatin immunoprecipitation (ChIP)-seq of the cortex and liver of adult (8 weeks) C57/Bl6 mice. We note that the sex of the mice and collection procedures used were not available. As H3K4me1 and H3K27ac partially overlap promoters, only H3K4me1 and H3K27ac peaks which were >2 kb from the transcriptional start site were used. The expected number of differentially methylated CpGs in each of the CpG contexts, genomic categories, and enhancer regions was calculated as follows. First, Methyl-Seq baits were mapped to the different CpG contexts, genomic categories, and enhancer regions. Sequences for the baits were retrieved using Galaxy. The number of CpGs mapped to each CpG context/ genomic category was calculated and expressed as a ratio of the total number of CpGs in all CpG contexts, genomic categories, or enhancer regions. This ratio was then multiplied by the total number of DMCs observed to determine the expected number of DMCs for each CpG context, genomic category, and enhancer region.
Since most previous studies have focused on methylation in the CpG context, we chose to evaluate tissue-specific differentially methylated cytosines (DMCs) in the CpG context for this study. Additional data on CHG and CHH contexts (where H is A, T or C) was generated. Given the unique challenges in analyzing and interpreting those data, we chose to consider them in a subsequent report.
Gene Ontology and Pathway Analysis
Gene ontology enrichment and pathway analysis using the Kyoto Encyclopedia of Genes and Genomes (KEGG) database was performed using the functional annotation tools of DAVID bioinformatics resources version 6.7.Citation54,55 Reported P-values have been corrected for multiple testing using the Benjamini-Hochberg method.
Disclosure of Potential Conflicts of Interest
No potential conflicts of interest were disclosed.
Supplemental_Material.zip
Download Zip (12 MB)Supplemental Material
Supplemental data for this article can be accessed on the publisher's website.
References
- Smith ZD, Meissner A. DNA methylation: roles in mammalian development. Nat Rev Genet 2013; 14:204-20; PMID:23400093; http://dx.doi.org/10.1038/nrg3354
- Kozlenkov A, Roussos P, Timashpolsky A, Barbu M, Rudchenko S, Bibikova M, Klotzle B, Byne W, Lyddon R, Di Narzo AF, et al. Differences in DNA methylation between human neuronal and glial cells are concentrated in enhancers and non-CpG sites. Nucleic Acids Res 2013; 42(1):109-27.
- Zhang B, Zhou Y, Lin N, Lowdon RF, Hong C, Nagarajan RP, Cheng JB, Li D, Stevens M, Lee HJ, et al. Functional DNA methylation differences between tissues, cell types, and across individuals discovered using the M&M algorithm. Genome Res 2013; 23:1522-40; PMID:23804400; http://dx.doi.org/10.1101/gr.156539.113
- Lee RS, Tamashiro KL, Aryee MJ, Murakami P, Seifuddin F, Herb B, Huo Y, Rongione M, Feinberg AP, Moran TH, et al. Adaptation of the CHARM DNA methylation platform for the rat genome reveals novel brain region-specific differences. Epigenetics 2011; 6:1378-90; PMID:22048247; http://dx.doi.org/10.4161/epi.6.11.18072
- Irizarry RA, Ladd-Acosta C, Wen B, Wu ZJ, Montano C, Onyango P, Cui HM, Gabo K, Rongione M, Webster M, et al. The human colon cancer methylome shows similar hypo- and hypermethylation at conserved tissue-specific CpG island shores. Nat Genet 2009; 41:178-86; PMID:19151715; http://dx.doi.org/10.1038/ng.298
- Davies M, Volta M, Pidsley R, Lunnon K, Dixit A, Lovestone S, Coarfa C, Harris RA, Milosavljevic A, Troakes C, et al. Functional annotation of the human brain methylome identifies tissue-specific epigenetic variation across brain and blood. Genome Biol 2012; 13:R43; PMID:22703893; http://dx.doi.org/10.1186/gb-2012-13-6-r43
- Heyn H, Moran S, Hernando-Herraez I, Sayols S, Gomez A, Sandoval J, Monk D, Hata K, Marques-Bonet T, Wang L, et al. DNA methylation contributes to natural human variation. Genome Res 2013; 23:1363-72; PMID:23908385; http://dx.doi.org/10.1101/gr.154187.112
- Klengel T, Mehta D, Anacker C, Rex-Haffner M, Pruessner JC, Pariante CM, Pace TW, Mercer KB, Mayberg HS, Bradley B, et al. Allele-specific FKBP5 DNA demethylation mediates gene-childhood trauma interactions. Nat Neurosci 2013; 16:33-41; PMID:23201972; http://dx.doi.org/10.1038/nn.3275
- Murgatroyd C, Patchev AV, Wu Y, Micale V, Bockmuhl Y, Fischer D, Holsboer F, Wotjak CT, Almeida OF, Spengler D. Dynamic DNA methylation programs persistent adverse effects of early-life stress. Nat Neurosci 2009; 12:1559-66; PMID:19898468; http://dx.doi.org/10.1038/nn.2436
- Robertson KD. DNA methylation and human disease. Nat Rev Genet 2005; 6:597-610; PMID:16136652; http://dx.doi.org/10.1038/nrg1655
- Harris RA, Wang T, Coarfa C, Nagarajan RP, Hong C, Downey SL, Johnson BE, Fouse SD, Delaney A, Zhao Y, et al. Comparison of sequencing-based methods to profile DNA methylation and identification of monoallelic epigenetic modifications. Nat Biotechnol 2010; 28:1097-105; PMID:20852635; http://dx.doi.org/10.1038/nbt.1682
- Nair SS, Coolen MW, Stirzaker C, Song JZ, Statham AL, Strbenac D, Robinson MD, Clark SJ. Comparison of methyl-DNA immunoprecipitation (MeDIP) and methyl-CpG binding domain (MBD) protein capture for genome-wide DNA methylation analysis reveal CpG sequence coverage bias. Epigenetics 2011; 6:34-44; PMID:20818161; http://dx.doi.org/10.4161/epi.6.1.13313
- Ziller MJ, Gu H, Muller F, Donaghey J, Tsai LT, Kohlbacher O, De Jager PL, Rosen ED, Bennett DA, Bernstein BE, et al. Charting a dynamic DNA methylation landscape of the human genome. Nature 2013; 500:477-81; PMID:23925113; http://dx.doi.org/10.1038/nature12433
- Girotto G, Abdulhadi K, Buniello A, Vozzi D, Licastro D, d'Eustacchio A, Vuckovic D, Alkowari MK, Steel KP, Badii R, et al. Linkage Study and Exome Sequencing Identify a BDP1 Mutation Associated with Hereditary Hearing Loss. PloS One 2013; 8:e80323.
- Madhavan S, Gusev Y, Natarajan TG, Song L, Bhuvaneshwar K, Gauba R, Pandey A, Haddad BR, Goerlitz D, Cheema AK, et al. Genome-wide multi-omics profiling of colorectal cancer identifies immune determinants strongly associated with relapse. Front Genet 2013; 4:236; PMID:24312117
- Ivanov M, Kals M, Kacevska M, Metspalu A, Ingelman-Sundberg M, Milani L. In-solution hybrid capture of bisulfite-converted DNA for targeted bisulfite sequencing of 174 ADME genes. Nucleic Acids Res 2013; 41:e72; PMID:23325842; http://dx.doi.org/10.1093/nar/gks1467
- Wang J, Jiang H, Ji G, Gao F, Wu M, Sun J, Luo H, Wu J, Wu R, Zhang X. High resolution profiling of human exon methylation by liquid hybridization capture-based bisulfite sequencing. BMC Genomics 2011; 12:597; PMID:22151801; http://dx.doi.org/10.1186/1471-2164-12-597
- Sandoval J, Heyn HA, Moran S, Serra-Musach J, Pujana MA, Bibikova M, Esteller M. Validation of a DNA methylation microarray for 450,000 CpG sites in the human genome. Epigenetics 2011; 6:692-702; PMID:21593595; http://dx.doi.org/10.4161/epi.6.6.16196
- Huang W, Loganantharaj R, Schroeder B, Fargo D, Li L. PAVIS: a tool for Peak Annotation and Visualization. Bioinformatics 2013; 29:3097-9; PMID:24008416; http://dx.doi.org/10.1093/bioinformatics/btt520
- Irizarry R, Ladd-Acosta C, Wen B, Wu Z, Montano C, Onyango P, Cui H, Gabo K, Rongione M, Webster M, et al. The human colon cancer methylome shows similar hypo- and hypermethylation at conserved tissue-specific CpG island shores. Nat Genet 2009; 41:178-86; PMID:19151715; http://dx.doi.org/10.1038/ng.298
- Ernst J, Kheradpour P, Mikkelsen TS, Shoresh N, Ward LD, Epstein CB, Zhang X, Wang L, Issner R, Coyne M, et al. Mapping and analysis of chromatin state dynamics in nine human cell types. Nature 2011; 473:43-9; PMID:21441907; http://dx.doi.org/10.1038/nature09906
- Kozlenkov A, Roussos P, Timashpolsky A, Barbu M, Rudchenko S, Bibikova M, Klotzle B, Byne W, Lyddon R, Di Narzo AF, et al. Differences in DNA methylation between human neuronal and glial cells are concentrated in enhancers and non-CpG sites. Nucleic Acids Res 2014; 42:109-27; PMID:24057217; http://dx.doi.org/10.1093/nar/gkt838
- Consortium TEP. An integrated encyclopedia of DNA elements in the human genome. Nature 2012; 489:57-74; PMID:22955616; http://dx.doi.org/10.1038/nature11247
- Lettice LA, Hill AE, Devenney PS, Hill RE. Point mutations in a distant sonic hedgehog cis-regulator generate a variable regulatory output responsible for preaxial polydactyly. Hum Mol Genet 2008; 17:978-85; PMID:18156157; http://dx.doi.org/10.1093/hmg/ddm370
- Davidson S, Lear M, Shanley L, Hing B, Baizan-Edge A, Herwig A, Quinn JP, Breen G, McGuffin P, Starkey A, et al. Differential activity by polymorphic variants of a remote enhancer that supports galanin expression in the hypothalamus and amygdala: implications for obesity, depression and alcoholism. Neuropsychopharmacology 2011; 36:2211-21; PMID:21716262; http://dx.doi.org/10.1038/npp.2011.93
- Matrisciano F, Busceti CL, Bucci D, Orlando R, Caruso A, Molinaro G, Cappuccio I, Riozzi B, Gradini R, Motolese M, et al. Induction of the Wnt antagonist dickkopf-1 is involved in stress-induced hippocampal damage. PloS One 2011; 6:e16447; PMID:21304589; http://dx.doi.org/10.1371/journal.pone.0016447
- Murray PS, Holmes PV. An overview of brain-derived neurotrophic factor and implications for excitotoxic vulnerability in the hippocampus. Intl J Pept 2011; 2011:654085; PMID:21966294; http://dx.doi.org/10.1155/2011/654085
- Galvez-Contreras AY, Quinones-Hinojosa A, Gonzalez-Perez O. The role of EGFR and ErbB family related proteins in the oligodendrocyte specification in germinal niches of the adult mammalian brain. Front Cell Neurosci 2013; 7:258; PMID:24381541; http://dx.doi.org/10.3389/fncel.2013.00258
- Ladd-Acosta C, Pevsner J, Sabunciyan S, Yolken RH, Webster MJ, Dinkins T, Callinan PA, Fan J-B, Potash JB, Feinberg AP. DNA methylation signatures within the human brain. A J Hum Genet 2007; 81:1304-15; http://dx.doi.org/10.1086/524110
- Xin Y, Chanrion B, Liu MM, Galfalvy H, Costa R, Ilievski B, Rosoklija G, Arango V, Dwork AJ, Mann JJ, et al. Genome-wide divergence of DNA methylation marks in cerebral and cerebellar cortices. PloS One 2010; 5:e11357; PMID:20596539; http://dx.doi.org/10.1371/journal.pone.0011357
- van den Boogaard MJH, Dorland M, Beemer FA, van Amstel HKP. MSX1 mutation is associated with orofacial clefting and tooth agenesis in humans. Nat Genet 2000; 24:342-3; PMID:10742093; http://dx.doi.org/10.1038/74155
- Ramos C, Fernandez-Llebrez P, Bach A, Robert B, Soriano E. Msx1 disruption leads to diencephalon defects and hydrocephalus. Dev Dyn 2004; 230:446-60; PMID:15188430; http://dx.doi.org/10.1002/dvdy.20070
- Varley KE, Gertz J, Bowling KM, Parker SL, Reddy TE, Pauli-Behn F, Cross MK, Williams BA, Stamatoyannopoulos JA, Crawford GE, et al. Dynamic DNA methylation across diverse human cell lines and tissues. Genome Res 2013; 23:555-67; PMID:23325432; http://dx.doi.org/10.1101/gr.147942.112
- Yue F, Cheng Y, Breschi A, Vierstra J, Wu WS, Ryba T, Sandstrom R, Ma ZH, Davis C, Pope BD, et al. A comparative encyclopedia of DNA elements in the mouse genome. Nature 2014; 515:355-64; PMID:25409824; http://dx.doi.org/10.1038/nature13992
- Antequera F, Bird A. Number of CpG islands and genes in human and mouse. Proc Natl Acad Sci U S A 1993; 90:11995-9; PMID:7505451; http://dx.doi.org/10.1073/pnas.90.24.11995
- Barrera LO, Li Z, Smith AD, Arden KC, Cavenee WK, Zhang MQ, Green RD, Ren B. Genome-wide mapping and analysis of active promoters in mouse embryonic stem cells and adult organs. Genome Res 2008; 18:46-59; PMID:18042645; http://dx.doi.org/10.1101/gr.6654808
- Jiang C, Han L, Su B, Li WH, Zhao Z. Features and trend of loss of promoter-associated CpG islands in the human and mouse genomes. Mol Biol Evol 2007; 24:1991-2000; PMID:17591602; http://dx.doi.org/10.1093/molbev/msm128
- Piechota M, Korostynski M, Przewlocki R. Identification of cis-regulatory elements in the mammalian genome: the cREMaG database. PloS One 2010; 5:e12465.
- Sharov AA, Dudekula DB, Ko MS. CisView: a browser and database of cis-regulatory modules predicted in the mouse genome. DNA Res 2006; 13:123-34; PMID:16980320; http://dx.doi.org/10.1093/dnares/dsl005
- Simmen MW. Genome-scale relationships between cytosine methylation and dinucleotide abundances in animals. Genomics 2008; 92:33-40; PMID:18485662; http://dx.doi.org/10.1016/j.ygeno.2008.03.009
- Xuan ZY, Zhao F, Wang JH, Chen GX, Zhang MQ. Genome-wide promoter extraction and analysis in human, mouse, and rat. Genome Biol 2005; 6:R72; PMID:16086854; http://dx.doi.org/10.1186/gb-2005-6-8-r72
- Hawkins TL, Oconnormorin T, Roy A, Santillan C. DNA purification and isolation using a solid-phase. Nucleic Acids Res 1994; 22:4543-4; PMID:7971285; http://dx.doi.org/10.1093/nar/22.21.4543
- Untergasser A, Cutcutache I, Koressaar T, Ye J, Faircloth BC, Remm M, Rozen SG. Primer3-new capabilities and interfaces. Nucleic Acids Res 2012; 40:e115; PMID:22730293; http://dx.doi.org/10.1093/nar/gks596
- Trim Galore! F.K. http://www.bioinformatics.babraham.ac.uk/projects/download.html#trim_galore
- Krueger F, Andrews SR. Bismark: a flexible aligner and methylation caller for Bisulfite-Seq applications. Bioinformatics 2011; 27:1571-2; PMID:21493656; http://dx.doi.org/10.1093/bioinformatics/btr167
- Lister R, Pelizzola M, Dowen RH, Hawkins RD, Hon G, Tonti-Filippini J, Nery JR, Lee L, Ye Z, Ngo QM, et al. Human DNA methylomes at base resolution show widespread epigenomic differences. Nature 2009; 462:315-22; PMID:19829295; http://dx.doi.org/10.1038/nature08514
- Lister R, Mukamel EA, Nery JR, Urich M, Puddifoot CA, Johnson ND, Lucero J, Huang Y, Dwork AJ, Schultz MD, et al. Global epigenomic reconfiguration during mammalian brain development. Science 2013; 341:1237905; PMID:23828890; http://dx.doi.org/10.1126/science.1237905
- Guo JU, Su Y, Shin JH, Shin J, Li H, Xie B, Zhong C, Hu S, Le T, Fan G, et al. Distribution, recognition and regulation of non-CpG methylation in the adult mammalian brain. Nat Neurosci 2014; 17:215-22; PMID:24362762; http://dx.doi.org/10.1038/nn.3607
- Akalin A, Kormaksson M, Li S, Garrett-Bakelman FE, Figueroa ME, Melnick A, Mason CE. methylKit: a comprehensive R package for the analysis of genome-wide DNA methylation profiles. Genome Biol 2012; 13:R87; PMID:23034086; http://dx.doi.org/10.1186/gb-2012-13-10-r87
- Goecks J, Nekrutenko A, Taylor J, Galaxy T. Galaxy: a comprehensive approach for supporting accessible, reproducible, and transparent computational research in the life sciences. Genome Biol 2010; 11:R86; PMID:20738864; http://dx.doi.org/10.1186/gb-2010-11-8-r86
- Blankenberg D, Von Kuster G, Coraor N, Ananda G, Lazarus R, Mangan M, Nekrutenko A, Taylor J. Galaxy: a web-based genome analysis tool for experimentalists. Curr Protoc Mol Biol 2010; Chapter 19:Unit 19 0 1-21; PMID:20069535
- Giardine B, Riemer C, Hardison RC, Burhans R, Elnitski L, Shah P, Zhang Y, Blankenberg D, Albert I, Taylor J, et al. Galaxy: a platform for interactive large-scale genome analysis. Genome Res 2005; 15:1451-5; PMID:16169926; http://dx.doi.org/10.1101/gr.4086505
- Heyn H, Esteller M. DNA methylation profiling in the clinic: applications and challenges. Nat Rev Genet 2012; 13:679-92; PMID:22945394; http://dx.doi.org/10.1038/nrg3270
- Huang DW, Sherman BT, Lempicki RA. Systematic and integrative analysis of large gene lists using DAVID bioinformatics resources. Nat Protoc 2009; 4:44-57; PMID:19131956; http://dx.doi.org/10.1038/nprot.2008.211
- Huang DW, Sherman BT, Lempicki RA. Bioinformatics enrichment tools: paths toward the comprehensive functional analysis of large gene lists. Nucleic Acids Res 2009; 37:1-13; PMID:19033363; http://dx.doi.org/10.1093/nar/gkn923