Abstract
Prenatal maternal stress (PNMS) in animals and humans predicts obesity and metabolic dysfunction in the offspring. Epigenetic modification of gene function is considered one possible mechanism by which PNMS results in poor outcomes in offspring. Our goal was to determine the role of maternal objective exposure and subjective distress on child BMI and central adiposity at 13½ years of age, and to test the hypothesis that DNA methylation mediates the effect of PNMS on growth. Mothers were pregnant during the January 1998 Quebec ice storm. We assessed their objective exposure and subjective distress in June 1998. At age 13½ their children were weighed and measured (n = 66); a subsample provided blood samples for epigenetic studies (n = 31). Objective and subjective PNMS correlated with central adiposity (waist-to-height ratio); only objective PNMS predicted body mass index (BMI). Bootstrapping analyses showed that the methylation level of genes from established Type-1 and -2 diabetes mellitus pathways showed significant mediation of the effect of objective PNMS on both central adiposity and BMI. However, the negative mediating effects indicate that, although greater objective PNMS predicts greater BMI and adiposity, this effect is dampened by the effects of objective PNMS on DNA methylation, suggesting a protective role of the selected genes from Type-1 and -2 diabetes mellitus pathways. We provide data supporting that DNA methylation is a potential mechanism involved in the long-term adaptation and programming of the genome in response to early adverse environmental factors.
Introduction
The developmental origins of adult health and disease hypothesisCitation1 states that perturbations in early life, including intrauterine exposure to maternal stress, could program metabolic functions and lead to poor health outcomes later in life, such as cardiovascular disease, obesity, and metabolic syndrome. Exposure to high levels of prenatal maternal stress (PNMS) is associated with a perturbation of the hypothalamic-pituitary-adrenal axis,Citation2 the body's central stress modulating mechanism, which is involved in metabolic pathways.Citation3
A growing amount of evidence shows that the induction of stress during pregnancy in animals, or psychological stress during pregnancy in humans, is associated with an increased risk of obesity and metabolic dysfunction in the offspring. In animals, for instance, maternal stress induced a long-lasting disturbance in feeding behavior and dysfunctions related to Type 2 diabetes mellitus in the aged rat.Citation4 Likewise, prenatal stress increased susceptibility to diet-induced obesity in rat offspring.Citation5 In another study, prenatal stress has been shown to increase the obesogenic effects of a high-fat-sucrose diet in a sex-specific manner in rat.Citation6 In mice, exposure to chronic variable stress produced alterations in long-term body weight and energy homeostasis regulation.Citation7 In a human Dutch famine study, adults who were prenatally exposed were found to have increased body weight, BMI, and waist circumference.Citation8 Furthermore, in other studies, maternal bereavement during pregnancy was found to predict a high risk of Type-1 and -2 diabetesCitation9,10 in the offspring in late childhood. More recently, maternal stress during pregnancy or during the first year of life was related to increased risk of the infant being overweight.Citation11 Finally, another study showed that antenatal depression was associated with higher central adiposity in children at the age of 3.Citation12
The animal PNMS literature, with its experimental method, can make clear, causal conclusions; we cannot, however, generalize the results to the more complex stress experience in humans.Citation13 In the human literature, although there is ample evidence of an association between some kinds of prenatal events or conditions and obesity outcomes in children, several issues remain. Retrospective approaches, as in the studies of bereavement, are unable to isolate the active ingredient in the PNMS effect: Are the effects due to the objective hardship experienced as a result of the event, or to the mother's distress? As well, such studies generally fail to assess the severity of the exposure or the severity of the distress, and instead compare exposed and non-exposed groups, a statistical approach that limits power. Next, given that women are not randomly assigned to experience depression or relationship difficulties in pregnancy, studies showing associations between maternal mood or psychosocial stressors and child outcomes cannot disentangle the genetic transmission of maternal traits from effects of the intrauterine environment and from conditions in the postnatal environment. Finally, subjects in retrospective studies may be biased in their reporting of life events since they will be knowledgeable about the outcomes for their child and may be searching for causes.
In order to improve understanding of prenatal stress effects in humans, we need a human model that uses a stressor that is independent of the mother's potential influence, and whose stress severity is randomly distributed. Natural disasters are such events. Project Ice Storm has been following a cohort of children whose mothers were pregnant during the worst natural disaster in Canadian history, the 1998 Quebec ice storm. The strength of this study lies in the independent nature of the stressor, which is not associated with potentially confounding maternal and household characteristics and thereby approximates the random assignment to stress conditions that is possible with laboratory animals. Furthermore, we are able to distinguish between 2 aspects of the maternal stress experience—objective hardship and subjective distress—that is impossible in animal studies and in archive-based or retrospective research. We have previously reported that objective PNMS predicted increased BMI and obesity risk in the Ice Storm cohort at age 5½,Citation14 as well as greater insulin secretion at age 13½.Citation15
The underlying molecular mechanisms responsible for these adverse PNMS effects are still not well understood. Epigenetic modification of gene function is considered to be one mechanism by which PNMS results in poor outcomes in the offspring. DNA methylation is an intensively studied epigenetic mechanism that could be modulated by exposure to a variety of maternal experiences and might participate in processes that “adapt” the genome to stress signals across multiple tissues and explain the broad-ranging effects of early life stress on the fetus.Citation16,17 Although epigenetic processes, such as DNA methylation, are proposed to be strong candidates as underlying mechanisms through which PNMS could affect metabolic health in offspring, there are few studies that are able to conduct empirical tests of these effects using mediation analyses. Baron and Kenny described a mediating effect as the possible effect of a third variable, intervening in an existing relationship between a predictor and an outcome.Citation18
By studying the genome-wide DNA methylation profile in isolated T-cells of the Ice Storm offspring at age 13½, we reported that prenatal maternal objective stress, but not subjective distress, was significantly correlated with methylation of CpG sites on genes related to metabolic function.Citation19 However, whether and to what extent DNA methylation can mediate PNMS effect on metabolic outcomes, such as BMI and central adiposity, remain to be investigated. The goal of the present study was to determine the impact of PNMS on BMI and central adiposity in adolescence at age 13½, and the potential mediating effect of DNA methylation. We hypothesized that children born to mothers who experienced higher levels of PNMS would exhibit higher body mass index (BMI) and central adiposity [waist-to-height ratio (WHtR)] than those born to mothers who experienced lower levels of PNMS, and that these effects would be mediated by DNA methylation of genes involved in metabolic pathways.
Results
Participants' characteristics
Three children were excluded from analyses because of exposure to maternal gestational diabetes. At the time of anthropometric assessment, the remaining 66 children were on average 13.6 y of age (SD = 0.1) and 95.6% were from households in the middle class and above (lower class, 1.5%; lower-middle class, 2.9%; middle class, 32.4%; upper-middle class, 48.5%; upper class, 14.7%). There were 37 boys and 29 girls. Of these, 9 children (13.6%) were classified as obese (8 boys and 1 girl). The data from a subgroup of 31 subjects, for whom both DNA methylation and body measures were available, were used for further DNA methylation mediation analyses. shows means and standard deviations for maternal variables, child variables, and storm-related variables for both data sets.
Table 1. Summary of descriptive statistics of study participants
Correlations
Objective and subjective PNMS were significantly correlated (r = 0.338, P = 0.005). Bivariate Pearson product-moment correlations were analyzed between the 2 outcome variables (BMI and WHtR) and the predictors (). Objective PNMS was significantly correlated with WHtR, with a trend-level correlation with BMI (P = 0.051), with higher objective PNMS predicting higher scores. Subjective PNMS was not significantly correlated with BMI but was positively associated with WHtR, again with greater PNMS associated with larger waists relative to heights. Sex was marginally significantly related to WHtR (P = 0.053) and BMI (P = 0.081), with boys having higher scores than girls. More prenatal maternal life events (other than the ice storm) were significantly associated with larger BMI scores and WHtR.
Table 2. Pearson correlations between outcome measures and predictors (n = 66)
Hierarchical Linear Regression models (n = 66)
Final, trimmed results of the hierarchical multiple regression of WHtR are presented in . In Step 1, objective PNMS was significantly related to WHtR and explained 10.6% of the variance: higher objective PNMS levels predicted larger waists relative to heights. In Step 2, subjective PNMS was also related to the children's WHtRs, accounting for an additional 5.2% of the variance: higher subjective PNMS levels predicted larger waists relative to heights. Finally, in Step 3, the number of life events in pregnancy was also related to WHtR and accounted for an additional 5.2% of the variance: women who experienced more prenatal life events (excluding the ice storm) had children with higher WHtRs. Together, objective PNMS, subjective PNMS, and prenatal life events accounted for 21.0% of the variance in the children's WHtRs. Neither the child's sex nor timing of exposure significantly explained any additional variance in the children's WHtRs.
Table 3. Hierarchical multiple regression of central adiposity (WHtR) (n = 66)
Final, trimmed results of the hierarchical multiple regression of BMI are presented in . Objective PNMS predicted BMI at a strong trend level (P = 0.051) in Step 1, explaining 5.8% of the variance: higher objective PNMS levels predicted higher BMI levels. Life events in pregnancy accounted for an additional 9.7% of the variance in the children's BMI. Together, objective PNMS and prenatal life events accounted for 12.5% of the variance in the children's BMI levels. None of the other predictors (subjective PNMS, child's sex, timing of exposure) significantly explained additional variance in the children's BMI.
Table 4. Hierarchical multiple regression of body mass index (BMI) (n = 66)
Mediation analysis (n = 31)
The bootstrapping analyses for the selected genes from the Type 1 diabetes pathway revealed 17 genes (57 CpG sites) for which there was a negative mediating effect (path a*b) () between objective PNMS and BMI () and 15 genes (51 CpG sites) negatively mediating the effect on central adiposity (). The average effect size (ES) of the mediation analyses for CpG sites in the Type 1 pathway was 0.18 (range: 0.09–0.31) for BMI and 0.18 (range: 0.11–0.31) for central adiposity. Among the selected genes from the Type 2 diabetes pathway, we found 10 genes (16 CpG sites) negatively mediating the effect of objective PNMS on BMI () and 7 genes (11 CpG sites) negatively mediating the effect on central adiposity (). The average ES of the mediation analyses for CpG sites in the Type 2 pathway was 0.16 (range: 0.09–0.26) for BMI and 0.16 (range: 0.12–0.22) for central adiposity. All above CpGs showing significant mediating effects from both Type-1 and -2 diabetes mellitus were arranged into groups (). Track on the screenshot of Integrative Genomics Viewer (IGV) window marked their location (Fig. S1). Table S2 lists the effects of objective PNMS on DNA methylation (path a), the effects of the DNA methylation on BMI and central adiposity controlling for objective PNMS (path b), the direct effects (path c'), and mediating effects (path a*b) for each CpG site. From that table, we observe that, in nearly all cases of mediation, the higher the objective PNMS (Storm32 score), the greater the methylation of the CpG; the greater the methylation of the CpG, the lower the BMI and central adiposity in the adolescent. In other words, although the total and direct effects of objective stress on BMI and WHtRs are positive (greater stress, greater BMI and central adiposity), the mediation via DNA methylation results in limiting the effect of the objective stress (greater stress, greater methylation; greater methylation, lower BMI and central adiposity), suggesting that the DNA methylation of these selected CpGs, at least, protects from the adverse metabolic outcomes. Remarkably, none of the genes we tested were found to mediate the effect of subjective PNMS on BMI or central adiposity.
Figure 1. Mediation analysis on the relationship between exposure to objective/subjective PNMS and growth outcomes. The path coefficients are known as the direct effects, indirect effects, and total effects. The direct effect indicates the effect of a risk factor on an outcome controlling for the mediators; the indirect effect (mediating effect) indicates the effect of the risk factor on an outcome variable through an intervening variable; the total effect is the full effect of risk factor on the outcome. It represents the sum of direct and indirect effects of the path. Path a is the effect of the objective/subjective PNMS (predictor variable) on the DNA methylation (mediator), path b is the effect of the DNA methylation on growth outcomes (outcome variable) controlling for the objective/subjective PNMS, and path c' is the direct effect of the objective/subjective PNMS on growth outcomes controlling for the DNA methylation. The path a*b indicates the indirect effect/mediating effect of objective/subjective PNMS on growth outcomes through DNA methylation (mediator). Path c is the total effect of objective/subjective PNMS on growth and obesity outcomes. This model can be represented by the following equation: c = c'+ a*b.
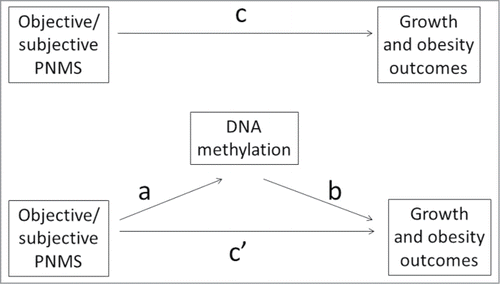
Table 5. Genes and their mediating effects on the impact of objective PNMS on BMI (n = 31)
Table 6. Genes and their mediating effects on the impact of objective PNMS on central adiposity (n = 31)
Table 7. The location of analyzed CpGs that mediates the effect of PNMS on BMI and central adiposity
Discussion
The first aim of this study was to test the effects of objective and subjective PNMS and other risk factors on BMI and central adiposity in 13½ year-old adolescents. The hierarchical multiple regression analyses revealed that objective and subjective PNMS were both independently related to central adiposity, while only objective PNMS was related to BMI. Life events in pregnancy other than the ice storm explained additional, unique variance in central adiposity beyond that explained by objective and subjective ice storm stress. The second aim was to determine the extent to which DNA methylation could mediate the impact of PNMS on these outcomes. The methylation levels of genes selected from established diabetes genes in both Type-1 and -2 diabetes mellitus pathways showed significant negative mediation of the effect of objective PNMS on both central adiposity and BMI, suggesting a protective role of the selected CpGs. This is the first study to determine that DNA methylation mediates the relationship between PNMS due to a natural disaster and growth and adiposity outcomes in human offspring.
A growing body of evidence from human and nonhuman studies shows that PNMS may have a long term effect on prenatal development and on growth and metabolism in offspring.Citation20 Project Ice Storm, a prospective longitudinal study, provides a human model of prenatal stress. Our current analyses, based on 66 children at age 13½, indicated that objective PNMS predicts greater central adiposity and BMI, while subjective PNMS predicted central adiposity. This finding is in accord with our previous studies that observed a significant predictive association between objective PNMS and BMI in the Ice Storm cohort at age 5½,Citation14 and that at age 8½ and 11½ (unpublished data). Together, these results suggest that it is the severity of the women's objective exposure to the ice storm that plays a greater role in predicting BMI than their subjective reactions to the crisis, and that children of mothers experiencing severe hardship in pregnancy could be at greater risk of developing obesity. Moreover, these findings highlight the longevity of the predictive role of PNMS on offspring adiposity in humans.
In our previous report with the same sample, we investigated the genome-wide DNA methylation profile and observed that only objective PNMS was related to methylation, and that the associated methylation patterns correspond to biological pathways that have been linked to metabolic outcomes.Citation19 Therefore, based on these findings, in the current study we were able to analyze the potential mediation of exposure to ice storm and growth outcome in later life by altered DNA methylation. Given that we have shown in this same subgroup of Project Ice Storm participants who agreed to give blood at age 13½, that objective PNMS was associated with greater insulin secretion, which is a feature of insulin resistance, increasing risk for Type 2 diabetes,Citation15 and, given the important role of Type-1 and -2 diabetes mellitus pathways on outcomes such as obesity, insulin resistance and diabetes, we selected genes associated with these pathways to conduct the mediation analyses.
Although both the total effect (c) (data not shown) and the direct effect (c') of objective PNMS on BMI and central adiposity were positive (higher stress, BMI, and central adiposity), the direction of all of the mediation effects were, however, negative (e.g., higher PNMS, higher methylation level; higher methylation level, lower BMI and central adiposity), suggesting that the positive effects of PNMS on child growth outcomes were dampened by DNA methylation. Thus, it seems that the methylation of these CpGs from Type-1 and -2 diabetes mellitus pathways protects the offspring from at-risk metabolic health, rather than explaining how PNMS predicts increased risk of adiposity in offspring. As social and physical environmental factors experienced during the early life period could have a long-lasting effect on offspring's health, DNA methylation might participate in sculpting genome function in response to these environmental signals.Citation16 As such, our data provide preliminary evidence of epigenomic programming of adaptation to the social environment. The fact that the adverse outcomes due to the objective PNMS cannot be explained by the negative mediating effect of DNA methylation is likely due, in part, to the lack of data on DNA methylation in other genes, which make up the Type-1 and -2 diabetes mellitus pathways. For example, the Type 1 diabetes mellitus pathway consists of 120 genes, besides the 19 genes analyzed in the current study; the remaining 101 genes might have positive mediating effects that could explain the positive effects of PNMS on offspring adiposity. A second explanation for the counterintuitive mediation results might be the lack of biological material at birth. As the blood samples were collected at age 13½, we cannot rule out the possibility that postnatal environmental factors could also have modified the offspring's DNA methylation profile in order to adapt to the postnatal environment, which differed from the stress of the prenatal environment. Therefore, future longitudinal studies are needed to differentiate between different timing of DNA methylation changes, and their relative effects on long-term outcomes.
Given that there is a well-established, complex partnership between immune and metabolic regulation, it is not surprising to observe that the majority of the genes whose methylation levels were correlated with both PNMS and child adiposity are involved in the immune system. Three genes, LTA, NFKBIA, and PIK3CD, which showed the greatest mediating effects, are of particular interest. Among all the genes investigated, LTA had the greatest number of significant CpGs, which are involved in both Type-1 and -2 diabetes mellitus pathways. LTA's 17 CpGs are distributed within TSS1500/200, 5′UTR, 1st exon and gene body (). LTA encodes lymphotoxin-α protein, a member of the tumor necrosis factor family, which is a well-identified pro-inflammatory cytokine produced by lymphocytes. Associations between variations in the LTA and metabolic traits have previously been reported. For example, the polymorphism of the LTA was associated with Type 2 diabetesCitation21,22 and other phenotypes of the metabolic syndrome.Citation23 LTA has been reported to be associated with insulin-dependent diabetes mellitus.Citation24 Two CpGs located within gene body in NFKBIA were found to have significant mediation effects. NFKBIA is one member of a family of cellular proteins that function to inhibit the NF-kappa-B. It is well known that, together with its inhibitor (NFKBIA), NF-kappa-B is an important transcription factor that participates in the activation of genes involved in immune responses. It has been shown that one polymorphism, A/G in the 3′UTR region of NFKBIA, could influence the pathogenesis of diabetes mellitus and affect its complications.Citation25 In addition, SNP rs1951276 in the 3′ region of NFKBIA was reported to be associated with obesity-induced insulin resistance.Citation26 Results also involved PIK3CD, which encodes p110delta; this enzyme belongs to the class I PI3K (Phosphatidylinositol 3-kinases), which are key mediators of insulin action. Three CpGs located within 3′UTR in PIK3CD were found to have significant mediation effects in the current study. PIK3CD was reported to be essential to the increased vascular contractile response in a mouse model of Type 1 diabetes.Citation27 Recently, it was observed that the mRNA level of PIK3CD in leukocyte was overexpressed in women with gestational diabetes mellitus.Citation28 To our knowledge, no prior PNMS studies have investigated DNA methylation in these genes. As such, LTA, NFKBIA, and PIK3CD could be considered potential candidate genes for follow-up PNMS studies. Our data highlight the important role of the immune system on obesity outcomes.
The present results are somewhat limited by the small sample size. To approximate the power of our bootstrapping method to detect the methylation mediation effect, we performed a bootstrapping power analysis method similar to the one explained in a recent publicationCitation29 and found that our results are actually underestimates of the number of moderate-to-strong mediations that we would have found with a larger sample. Furthermore, as our original design is not a case-control study design, but rather focused on exploration of “dose-response” relationships, our finding lacks data for the methylation profile of youth who were not exposed (i.e., a control group). In regard to the tissue-specificity, we would have studied DNA methylation in adipose tissue taken from the children, but obtaining blood is obviously a much less invasive procedure. As a viable alternative, blood has been used successfully in epigenetic research in obesity. Additionally, blood serves as crucial material for studying the role of inflammation and immune dysfunction in obesity.Citation30,31 Still, the heterogeneity between blood and adipose tissue is a limitation of our study. Moreover, we were not able to obtain the RNA samples from the subjects due to the low amount of blood collected. As such, the gene expression levels of LTA, NFKBIA, and PIK3CD, which showed greatest mediating effects, need to be carefully examined in further studies. As the DNA methylation and BMI data were taken at the same age (13½), our results do not allow any definitive conclusions about the direction of cause between DNA methylation and BMI. In order to further explore the potential for reverse causality we tested 2 alternative models. Model 1 posited an indirect effect of PNMS on DNA methylation at age 13½ through BMI at age 13½, thus reversing the causal direction. No significant mediation analyses were found in this alternative model, and statistical comparisons of the fit indices showed that our original model was a significantly better fit than the reverse causation model. Our Model 2 posited an indirect effect of PNMS on BMI at the later age of 15½ through DNA methylation at 13½. Despite testing the model with a smaller sample due to attrition, many significant mediation effects were detected. Taken together, we believe these findings offer preliminary support for our causal chain hypothesis.
The present study is strengthened by its prospective design using a unique human model, a natural disaster, through which we can isolate objective and subjective aspects of PNMS and their associations with offspring phenotypes. This is because the objective degree of ice storm exposure was quasi-randomly distributed in the population, and completely independent of the women's control or influence (unlike many common life events), and, therefore, objective PNMS was not biased by genetic or socioeconomic confounding. Based on the high-throughput investigation of DNA methylation across 480,000 individual CpG sites throughout the genome, we were able to conduct a mediation analysis and provided evidence that objective PNMS affects growth outcomes via DNA methylation of genes involved in Type 1 and Type 2 diabetes mellitus pathways. These results support the contention that PNMS has a significant effect on growth outcomes in offspring. Additionally, our study implicates the potential role of DNA methylation as a protective factor for growth outcomes. Taking together, this study contributes new data suggesting DNA methylation could act as an intervening variable between PNMS and growth outcomes, and could help to understand how risk factors such as genetic, environmental, social and biological factors work together to alter risk for some disorders.Citation32
In conclusion, this study has shown that objective PNMS resulting from the 1998 Quebec ice storm predicted BMI and central adiposity in the adolescent offspring. Although we cannot explain the above findings through our observation of the protective mediating effect of selected genes from 2 metabolic pathways, we provide data supporting the hypothesis that DNA methylation is a potential mechanism involved in the long-term adaptation and programming of the genome programs in response to early adverse environmental factors.
Materials and Methods
Participants
Participants were involved in the longitudinal Project Ice Storm,Citation33 which focuses on disaster-related PNMS and child development. Assessments of the women and their children have been conducted several occasions since the families were first contacted in June 1998, 5 months after the storm.Citation34 All mothers were 18 y of age or older at recruitment, and all participants were Francophone Caucasians. At age 13½ (M = 13.6, SD = 0.09), 69 families agreed to a comprehensive assessment of the children's physical, cognitive, motor and behavioral development. The children (38 boys and 31 girls) had been in their first (n = 17), second (n = 18), or third (n = 17) trimester of pregnancy on January 9, 1998 (the peak of the ice storm), or were conceived within 3 months of the storm (n = 17), when maternal stress hormones could still be elevated. As part of a separate wave of data collection, we invited subjects to give a fasting blood sample in October-November 2011. The 31 adolescents who accepted (19 boys, 12 girls) were, on average, 13.3 (SD = 0.29) years of age at the time of blood sampling. All phases of this study were approved by the Research Ethics Board of the Douglas Hospital Research Center. We obtained informed consent from parents at every assessment, and informed assent from children at the age 13½ assessment.
Predictors
Storm-related variables
The degree of objective and subjective PNMS, as well as family demographic variables, was assessed in a postal questionnaire mailed to participants on June 1, 1998. Objective PNMS was calculated using the mothers' responses to questionnaire items tapping into categories of exposure used in other disaster studies: Threat, Loss, Scope, and Change.Citation35 Because each natural disaster presents unique experiences to the exposed population, questions pertaining to each of the 4 categories must be tailor-made. Each of the 4 dimensions was scored on a scale of 0–8, ranging from no exposure to high exposure. A total objective hardship score (Storm32) was computed by summing scores from all 4 dimensions using McFarlane's approach.Citation36 Details of the Storm32 items and scoring are presented elsewhere.Citation37
Subjective PNMS was assessed using the validated French versionCitation38 of the widely used Impact of Event Scale – Revised (IES-R).Citation39 The 22-item scale describes symptoms from 3 categories relevant to post-traumatic stress disorder: Intrusive Thoughts, Hyperarousal, and Avoidance. The IES-R instructions for respondents allow investigators or clinicians to “write in” the traumatic event in question. Participants responded on a 5-point Likert scale, from “Not at all” to “Extremely,” the extent to which each item described how they felt over the preceding 7 d in response to the ice storm crisis. We used the total score in all analyses.
Timing of exposure to the ice storm in utero was calculated using the child's birth date, and the gestational age at birth (as recorded in the birth records) to estimate conception date, then calculating the number of days between conception and January 9, 1998 as the date of the peak of the ice storm. As such, higher numbers indicate exposure later in pregnancy.
Maternal variables
The level of maternal psychological functioning was assessed with a validated French version of the widely-used General Health Questionnaire-28 (GHQ).Citation40 The GHQ is a self-report screening tool for psychiatric symptoms and includes 7 items in each of the depression, anxiety, dysfunction, and somatization sub-scales. Items are scored on a 4-point Likert scale indicating the degree to which each symptom was experienced in the preceding 2 weeks. In the present study, each item was re-scored as either 0 (a rating of 0 or 1) or 1 (a rating of 2 or 3), according to the Goldberg method,Citation40 resulting in a minimum possible score of 0 and a maximum possible score of 28. The total score was used in analyses. The GHQ was included in the June 1998 questionnaire, and also when their children were 13½ years of age.
Exposure to potentially stressful maternal life events was assessed in a questionnaire sent 6 months after each woman's due date, and again at the 13½-year assessment. Women answered the Life Experiences Survey (LES),Citation41 a self-report measure that lists 57 life changes, such as death of a spouse or a promotion at work. To keep the questionnaire length reasonable, we reduced this to 29 events by eliminating items not likely to have occurred in this sample (e.g., combat experience). At the 6-month questionnaire, women indicated events (except the ice storm) that occurred during the 6 months since the baby's due date, the 9 months of pregnancy, and the 3 months before conception. At 13½ years, they reported on events that occurred during the previous 2 y Women gave the approximate date of each event experienced, and rated the impact of each event on a 7-point Likert scale ranging from “extremely negative” to “extremely positive.” The numbers of life events at each assessment were used in analyses.
Data on maternal and paternal age and education, and parental job classification, were collected in June 1998. Socio-economic status (SES) was computed using Hollingshead Index criteria.Citation42
The number of obstetrical complications, including flu with fever, was determined by maternal recall using an adaptation of the scale used by KinneyCitation43 in our 6-month postpartum questionnaire, and verified using hospital records. We used the total number of obstetrical complications experienced by the women that were rated as moderate-to-severe using the McNeil-Sjöström Scale for Obstetric Complications.Citation44
Children's birth weight, birth length, and gestational age were obtained from maternal reports (transcribed from Quebec birth records given at discharge) in the 6-month postpartum questionnaire, and from hospital records. Birth ponderal index was calculated (100 × (birth weight (g)/birth lenghtCitation3 (cm)).
Blood samples and T-cell isolation at 13½ years of age
Blood was collected from 31 subjects for T-cell isolation and DNA extraction using methods which have been described previously.Citation19,45 Briefly, T-cells were isolated from PBMCs by immunomagnetic separation with Dynabeads CD3 (Dynal, Invitrogen). DNA extraction from T-cells was performed using Wizard Genomic DNA Purification kit (Promega) according to the manufacturer's instructions.
Infinium Human Methylation 450 BeadChip Array and data analysis
Infinium HumanMethylation450 BeadChip, an array containing 485,577 probes covering 99% RefSeq genes and 96% of CpG islands, was used to determine DNA methylation levels in T-cells. We then correlated the levels of methylation with objective/subjective PNMS. Probes on chromosomes X and Y were excluded. To avoid artifacts due to hybridization bias, probes with minor allele frequency (MAF) ≥ 5% in the HapMap CEU population were removed. Furthermore, CpGs with an inter-quartile range (IQR) less than 0.10 (i.e., 10% methylation difference) were not analyzed. The remaining 10,553 probes were tested for association with the objective/subjective PNMS. The Benjamini-Hochberg algorithm was used to correct for multiple testing by computing the false discovery rate (FDR), which was set at <0.2. Illumina 450K Methylation BeadChip analyses were completed using standard procedures and described previously.Citation19
Child outcome measures at age 13½
During the face-to-face assessment of the child, height, weight and waist measurements were collected following standard guidelines,Citation46 repeating each measure twice. Standing height was measured without shoes to the nearest 0.1 cm and weight to the nearest 0.1 kg. Waist circumference was measured twice at the level of the umbilicus, to the nearest 0.1 cm. The mean of the 2 measurements was used for analysis. Body Mass Index (BMI, kg/m2) Z-Scores were computed based on World Health Organization growth references.Citation47 Central adiposity was calculated as the ratio of waist circumference (cm) by height (cm).
Selection of candidate genes for testing mediation by methylation
In order to determine the extent to which gene methylation mediates the association between objective hardship or subjective distress and BMI and central adiposity at 13½ years, we tested genes that we have previously shown to have their methylation signatures from isolated T-cells associated with objective hardship levels in this sampleCitation19; subjective PNMS had not correlated with methylation of any CpG sites. We then matched the genes whose methylation had been significantly correlated with PNMS to the Type-1 and -2 diabetes mellitus pathways as classified by IPA software (www.ingenuity.com). In total, 19 genes (64 CpGs) in the Type 1 diabetes mellitus pathway, and 12 genes (19 CpGs) in the Type 2 diabetes mellitus pathway, were selected. Because there were 6 CpGs overlapping between Type-1 and -2 diabetes mellitus pathways, there were 75 unique CpGs which were used for further analysis (Table S1). Additionally, to reduce the risk of missing potentially significant CpG site mediations we tested the associations between outcomes and all remaining CpG sites and none were significant.
Statistical analysis
Pearson product-moment correlations were conducted between the outcome measures and all predictors. Hierarchical linear regression analysis was conducted on the children's WHtR and BMI scores. Because of the relatively small sample size, all non-significant main effects were then trimmed from the equation. Only the final models are presented. All analyses were completed with SPSS 20.0 (SPSS, Chicago, IL).
Mediation analysis and bootstrapping methods
To investigate whether the genes we selected mediated the relationship between exposure to objective hardship, subjective distress and growth outcomes in the subsample of 31 subjects, we conducted a mediation analysis using state-of-the-art bootstrapping.Citation48 Mediation models hypothesize a causal chain in which the independent variable affects the mediator variable, which, in turn, affects the dependent variable. The current mediation analysis included one of the 2 body measurements as the outcome, and either objective or subjective PNMS as the predictor, and DNA methylation levels of CpGs as mediators. The theoretical model of the mediation analysis is presented in .
We used bootstrap methods, one of the routinely used approaches, to determine the significance of mediation effects in the mediation analyses.Citation49 Bootstrapping is a powerful approach because it takes into account that the sampling distribution of the mediated effect is skewed away from 050 and this approach can be applied for small-to-moderate samples (i.e., sample sizes ranging from 20–80 cases).Citation51 Bootstrapping involves randomly resampling with replacement from the dataset to compute the desired statistic in each resample, providing confidence intervals by which the significance of a mediation effect can be accessed. We tested the indirect effects of objective and subjective PNMS on the WHtR and BMI through each CpG site associated with either the Type 1 diabetes pathway (64 CpG sites in 19 genes) or the Type 2 diabetes pathway (19 CpG sites in 12 genes). To that end, 95% bias-corrected bootstrap confidence intervals were computed, as explained by Hayes.Citation52 The PROCESS procedure for SPSSCitation52 was used to conduct the analyses. Each bootstrap resampled the initial sample 10,000 times. In order to be able to recover the same results, the random seed for all bootstraps was fixed at 1509805407, an integer randomly generated between 1 and 2000000000 prior to the first bootstrap. A mediating effect was considered significant if 0 was not included in the bootstrap confidence interval.
The effect size of each CpG site was measured using the method described by Preacher and Kelley.Citation53 According to this approach, effect sizes of mediation analyses can be interpreted in a manner similar to that using the Pearson correlation coefficient r-value.
Disclosure of Potential Conflicts of Interest
No potential conflicts of interest were disclosed.
1063771_Supplemental_Material.zip
Download Zip (344.2 KB)Acknowledgment
We are grateful to families for their continued participation in Project Ice Storm.
Funding
This research was supported by a grant from the Canadian Institute of Health Research (CIHR) to Suzanne King and colleagues.
Supplemental Material
Supplemental data for this article can be accessed on the publisher's website.
References
- Barker DJ. Fetal origins of coronary heart disease. BMJ 1995; 311:171-4; PMID:7613432; http://dx.doi.org/10.1136/bmj.311.6998.171
- Sandman CA, Wadhwa PD, Dunkel-Schetter C, Chicz-DeMet A, Belman J, Porto M, Murata Y, Garite TJ, Crinella FM. Psychobiological influences of stress and HPA regulation on the human fetus and infant birth outcomes. Ann N Y Acad Sci 1994; 739:198-210; PMID:7832474; http://dx.doi.org/10.1111/j.1749-6632.1994.tb19822.x
- Nieuwenhuizen AG, Rutters F. The hypothalamic-pituitary-adrenal-axis in the regulation of energy balance. Physiol Behav 2008; 94:169-77; PMID:18275977; http://dx.doi.org/10.1016/j.physbeh.2007.12.011
- Lesage J, Del-Favero F, Leonhardt M, Louvart H, Maccari S, Vieau D, Darnaudery M. Prenatal stress induces intrauterine growth restriction and programmes glucose intolerance and feeding behaviour disturbances in the aged rat. J Endocrinol 2004; 181:291-6; PMID:15128277; http://dx.doi.org/10.1677/joe.0.1810291
- Tamashiro KL, Terrillion CE, Hyun J, Koenig JI, Moran TH. Prenatal stress or high-fat diet increases susceptibility to diet-induced obesity in rat offspring. Diabetes 2009; 58:1116-25; PMID:19188431; http://dx.doi.org/10.2337/db08-1129
- Paternain L, de la Garza AL, Batlle MA, Milagro FI, Martinez JA, Campion J. Prenatal stress increases the obesogenic effects of a high-fat-sucrose diet in adult rats in a sex-specific manner. Stress 2013; 16:220-32; PMID:22738222; http://dx.doi.org/10.3109/10253890.2012.707708
- Mueller BR, Bale TL. Impact of prenatal stress on long term body weight is dependent on timing and maternal sensitivity. Physiol Behav 2006; 88:605-14; PMID:16828814; http://dx.doi.org/10.1016/j.physbeh.2006.05.019
- Belle D. Poverty and Women's Mental Health. Am Psychol 1990; 45:385-9; http://dx.doi.org/10.1037/0003-066X.45.3.385
- Li J, Olsen J, Vestergaard M, Obel C, Kristensen JK, Virk J. Prenatal exposure to bereavement and type-2 diabetes: a Danish longitudinal population based study. PLoS One 2012; 7:e43508; PMID:22952698; http://dx.doi.org/10.1371/journal.pone.0043508
- Virk J, Li J, Vestergaard M, Obel C, Lu M, Olsen J. Early life disease programming during the preconception and prenatal period: making the link between stressful life events and type-1 diabetes. PLoS ONE 2010; 5:e11523; PMID:20634978; http://dx.doi.org/10.1371/journal.pone.0011523
- Watt TT, Appel L, Roberts K, Flores B, Morris S. Sugar, stress, and the Supplemental Nutrition Assistance Program: early childhood obesity risks among a clinic-based sample of low-income Hispanics. J Community Health 2013; 38:513-20; PMID:23197136; http://dx.doi.org/10.1007/s10900-012-9641-1
- Ertel KA, Koenen KC, Rich-Edwards JW, Gillman MW. Antenatal and postpartum depressive symptoms are differentially associated with early childhood weight and adiposity. Paediatr Perinat Epidemiol 2010; 24:179-89; PMID:20415775; http://dx.doi.org/10.1111/j.1365-3016.2010.01098.x
- Lazarus RS, Folkman S. Stress, appraisal and coping. NY: Springer, 1984
- Dancause KN, Laplante DP, Fraser S, Brunet A, Ciampi A, Schmitz N, King S. Prenatal exposure to a natural disaster increases risk for obesity in 5 ½ year old children. Pediatr Res 2012; 71:126-31; PMID:22289861; http://dx.doi.org/10.1038/pr.2011.18
- Dancause KN, Veru F, Andersen RE, Laplante DP, King S. Prenatal stress due to a natural disaster predicts insulin secretion in adolescence. Early Hum Dev 2013; 89:773-6; PMID:23830724; http://dx.doi.org/10.1016/j.earlhumdev.2013.06.006
- Szyf M. How do environments talk to genes? Nat Neurosci 2013; 16:2-4; PMID:23257925; http://dx.doi.org/10.1038/nn.3286
- Szyf M. DNA methylation, behavior and early life adversity. Journal of genetics and genomics = Yi chuan xue bao 2013; 40:331-8; PMID:23876773; http://dx.doi.org/10.1016/j.jgg.2013.06.004
- Baron RH, Kenny DA. The moderator-mediator variable distinction in social psychological research: Conceptual, strategic, and statistical considerations. J Pers Soc Psychol 1986; 51:1173-82; PMID:3806354; http://dx.doi.org/10.1037/0022-3514.51.6.1173
- Cao-Lei L, Massart R, Suderman M, Machnes Z, Laplante D, Szyf M, King S. DNA methylation signatures of prenatal maternal objective stress exposure to a natural disaster: Project Ice Storm. PLoS ONE 2014; 9:e107653; PMID:25238154; http://dx.doi.org/10.1371/journal.pone.0107653
- Godfrey KM, Inskip HM, Hanson MA. The long term effects of prenatal development on growth and metabolism. Semin Reprod Med 2011; 29:257; PMID:21769765; http://dx.doi.org/10.1055/s-0031-1275518
- Hamid YH, Urhammer SA, Glumer C, Borch-Johnsen K, Jorgensen T, Hansen T, Pedersen O. The common T60N polymorphism of the lymphotoxin-alpha gene is associated with type 2 diabetes and other phenotypes of the metabolic syndrome. Diabetologia 2005; 48:445-51; PMID:15729581; http://dx.doi.org/10.1007/s00125-004-1659-1
- Mahajan A, Tabassum R, Chavali S, Dwivedi OP, Chauhan G, Tandon N, Bharadwaj D. Obesity-dependent association of TNF-LTA locus with type 2 diabetes in North Indians. J Mol Med (Berl) 2010; 88:515-22; PMID:20177654; http://dx.doi.org/10.1007/s00109-010-0594-5
- Phillips CM, Goumidi L, Bertrais S, Ferguson JF, Field MR, Kelly ED, Mehegan J, Peloso GM, Cupples LA, Shen J, et al. Additive effect of polymorphisms in the IL-6, LTA, and TNF-{alpha} genes and plasma fatty acid level modulate risk for the metabolic syndrome and its components. J Clin Endocrinol Metab 2010; 95:1386-94; PMID:20080841; http://dx.doi.org/10.1210/jc.2009-1081
- Feugeas JP, Caillens H, Poirier JC, Charron D, Marcelli-Barge A, Wautier JL. Influence of metabolic and genetic factors on tumour necrosis factor-alpha and lymphotoxin-alpha production in insulin-dependent diabetes mellitus. Diabetes Metab 1997; 23:295-301; PMID:9342542
- Romzova M, Hohenadel D, Kolostova K, Pinterova D, Fojtikova M, Ruzickova S, et al. NFkappaB and its inhibitor IkappaB in relation to type 2 diabetes and its microvascular and atherosclerotic complications. Hum Immunol 2006; 67:706-13.
- Miller MR, Zhang W, Sibbel SP, Langefeld CD, Bowden DW, Haffner SM, et al. Variant in the 3′ region of the IkappaBalpha gene associated with insulin resistance in Hispanic Americans: The IRAS Family Study. Obesity (Silver Spring) 2010; 18:555-62.
- Pinho JF, Medeiros MA, Capettini LS, Rezende BA, Campos PP, Andrade SP, et al. Phosphatidylinositol 3-kinase-delta up-regulates L-type Ca2+ currents and increases vascular contractility in a mouse model of type 1 diabetes. Br J Pharmacol 2010; 161:1458-71.
- Wojcik M, Mac-Marcjanek K, Wozniak LA, Nadel I, Lewinski A, Cypryk K. The association of leukocyte phosphatidylinositol 3-kinase delta overexpression with gestational diabetes mellitus (GDM). Endokrynol Pol 2014; 65:17-24.
- Fritz MS, Mackinnon DP. Required sample size to detect the mediated effect. Psychol Sci 2007; 18:233-9; PMID:17444920; http://dx.doi.org/10.1111/j.1467-9280.2007.01882.x
- Mansuy-Aubert V, Zhou QL, Xie X, Gong Z, Huang JY, Khan AR, Aubert G, Candelaria K, Thomas S, Shin DJ, et al. Imbalance between neutrophil elastase and its inhibitor alpha1-antitrypsin in obesity alters insulin sensitivity, inflammation, and energy expenditure. Cell Metab 2013; 17:534-48; PMID:23562077; http://dx.doi.org/10.1016/j.cmet.2013.03.005
- McGuire TR, Brusnahan SK, Bilek LD, Jackson JD, Kessinger MA, Berger AM, Garvin KL, O'Kane BJ, Tuljapurkar SR, Sharp JG. Inflammation associated with obesity: relationship with blood and bone marrow endothelial cells. Obesity (Silver Spring) 2011; 19:2130-6; PMID:21901025; http://dx.doi.org/10.1038/oby.2011.246
- Kraemer HC, Stice E, Kazdin A, Offord D, Kupfer D. How do risk factors work together? Mediators, moderators, and independent, overlapping, and proxy risk factors. Am J Psychiatry 2001; 158:848-56; PMID:11384888; http://dx.doi.org/10.1176/appi.ajp.158.6.848
- King S, Laplante DP. The effects of prenatal maternal stress on children's cognitive development: Project Ice Storm. Stress 2005; 8:35-45; PMID:16019596; http://dx.doi.org/10.1080/10253890500108391
- King S, Dancause K, Turcotte-Tremblay A-M, Veru F, Laplante DP. Using Natural Disasters to Study the Effects of Prenatal Maternal Stress on Child Health and Development. Birth Defects Research Part C: Embryo Today: Reviews 2012; 96:273-88; http://dx.doi.org/10.1002/bdrc.21026
- Bromet E, Dew MA. Review of Psychiatric Epidemiologic Research on Disasters. Epidemiol Rev 1995; 17:113-9; PMID:8521929
- McFarlane AC. Relationship between psychiatric impairment and a natural disaster: the role of distress. Psychol Med 1988; 18:129-39; PMID:3363033; http://dx.doi.org/10.1017/S0033291700001963
- Laplante DP, Zelazo PR, Brunet A, King S. Functional play at 2 years of age: effects of prenatal maternal stress. Infancy 2007; 12:69-93; http://dx.doi.org/10.1111/j.1532-7078.2007.tb00234.x
- Brunet A, St-Hilaire A, Jehel L, King S. Validation of a French version of the Impact of Event Scale – Revised. Can J Psychiatry 2003; 48:55-60
- Weiss DS, Marmar CR. The Impact of Event Scale – Revised. In: Wilson JP, Keane TM, eds. Assessing psychological trauma and PTSD: A practitioner's handbook. New York: Guilford, 1997:399-411
- Goldberg DP. The Detection of Psychiatric Illness by Questionnaire: A Technique for the Identification and Assessment of Non-Psychiatric Illness. London: Oxford University Press, 1972
- Sarason IG, Johnson JH, Siegel JM. Assessing the impact of life changes: development of the Life Experience Survey. J Consult Clin Psychol 1978; 46:932-46; PMID:701572; http://dx.doi.org/10.1037/0022-006X.46.5.932
- Hollingshead AB. Four-factor index of social status. New Haven: Yale University Press, 1973
- Jacobsen B, Kinney DK. Perinatal complications in adopted and non-adopted samples of schizophrenics and controls. Acta Psychiatr Scand 1980; 62:337-46; http://dx.doi.org/10.1111/j.1600-0447.1980.tb07709.x
- McNeil TF, Sjöström K. The McNeil–Sjöström OC Scale: a Comprehensive Scale for Measuring Obstetric Complications. Department of Psychiatry, Lund University, Malmö General Hospital, Malmö, Sweden, 1995
- Cao-Lei L, Elgbeili G, Massart R, Laplante DP, Szyf M, King S. Pregnant women's cognitive appraisal from a natural disaster affects DNA methylation in their children 13 years later: Project Ice Storm. Translational psychiatry 2015; 5.
- Lohman TG, Roche AF, Martorell R. Anthropometric standardization reference manual. Champaign, IL: Human Kinetics Books, 1988
- World Health Organization. Growth reference data for 5-19 years. Geneva: World Health Organization, 2010
- Krause MR, Serlin RC, Ward SE, Rony RY, Ezenwa MO, Naab F. Testing mediation in nursing research: beyond Baron and Kenny. Nurs Res 2010; 59:288-94; PMID:20467337; http://dx.doi.org/10.1097/NNR.0b013e3181dd26b3
- Preacher KJ, Hayes AF. SPSS and SAS procedures for estimating indirect effects in simple mediation models. Behav Res Methods Instrum Comput 2004; 36:717-31; PMID:15641418; http://dx.doi.org/10.3758/BF03206553
- Shrout PE, Bolger N. Mediation in experimental and nonexperimental studies: new procedures and recommendations. Psychol Methods 2002; 7:422-45; PMID:12530702; http://dx.doi.org/10.1037/1082-989X.7.4.422
- Efron B, Tibshirani R. An introduction to the bootstrap. Boca Raton: Chapman and Hall/CRC, 1993
- Hayes AF. Introduction to mediation, moderation, and conditional process analysis. New York: The Guilford Press, 2013
- Preacher KJ, Kelley K. Effect size measures for mediation models: quantitative strategies for communicating indirect effects. Psychol Methods 2011; 16:93-115; PMID:21500915; http://dx.doi.org/10.1037/a0022658