Abstract
Reduced representation bisulfite sequencing (RRBS) is a powerful method of DNA methylome profiling that can be applied to single cells. However, no previous report has described how PCR-based duplication-induced artifacts affect the accuracy of this method when measuring DNA methylation levels. For quantifying the effects of duplication-induced artifacts on methylome profiling when using ultra-trace amounts of starting material, we developed a novel method, namely quantitative RRBS (Q-RRBS), in which PCR-induced duplication is excluded through the use of unique molecular identifiers (UMIs). By performing Q-RRBS on varying amounts of starting material, we determined that duplication-induced artifacts were more severe when small quantities of the starting material were used. However, through using the UMIs, we successfully eliminated these artifacts. In addition, Q-RRBS could accurately detect allele-specific methylation in absence of allele-specific genetic variants. Our results demonstrate that Q-RRBS is an optimal strategy for DNA methylation profiling of single cells or samples containing ultra-trace amounts of cells.
Introduction
During embryonic development, tumorigenesis, and aging, the DNA methylome exhibits spatial and temporal distinct patterns within different cell types.Citation1,2 Characterizing the DNA methylome of the pioneer cells of specialized cell populations is essential for revealing the role of DNA methylation in these biological processes. However, because large quantities of starting material are required when using current methods of methylome profiling,Citation3,4 the specific cell types presented in the trace amounts within cell populations have rarely been analyzed.Citation5-8
Recently, reduced representation bisulfite sequencing (RRBS), a highly accurate method of DNA methylome profiling, was optimized for studying the methylome landscape during embryonic development by using both dozens-of-cells (DC) and single-cell (SC) samples as starting materials.Citation9-11 However, to prepare a sufficient quantity of library for next-generation sequencing from the DC or SC samples, excessive PCR amplification (>40 cycles) was performed, which might have resulted in abundant duplication in the data.Citation12 Given that the sequencing libraries for RRBS are based on enzyme-digestion, which generates identical reads derived from multi-copies of the chromosome at the same position, no available strategy can discriminate these reads are derived from distinct copies of the same fragments, or from PCR-induced duplicates. Thus, confusion between the genuine molecular copies and duplicates might bias the results of DNA methylation analyses.
To overcome the aforementioned complication, we developed quantitative RRBS (Q-RRBS), a method in which unique molecular identifiers (UMIs) are used to eliminate PCR-induced duplication. Recently, UMIs were applied for counting individual RNA or DNA molecules in sequencing data of post-amplification libraries.Citation13 UMI labeling of cDNA was successfully employed in library construction for RNA-seq in order to eliminate PCR-induced duplication and determine the true counts of RNA molecules.Citation14 Furthermore, UMIs were also used for eliminating amplification noise in single-cell RNA-seq, and could be well-suited for profiling allele-specific expression patterns when allele-specific genetic variants exist.Citation15 We employed Q-RRBS to analyze—for the first time—the proportions of PCR-induced duplicates present in the sequencing data obtained from 30 ng of genomic DNA and DC and SC samples by using RRBS, and to investigate the effects of this duplication on the accuracy of the DNA methylation data. We further report that our Q-RRBS method could be used for precisely determining allele-specific DNA methylation (ASM) regions even in the absence of heterozygous single nucleotide polymorphisms (SNPs). We therefore propose that our Q-RRBS method is an optimal strategy for DNA methylation profiling of both SC samples and samples containing ultra-trace amounts of starting material.
Results
UMIs can be used to identify PCR-induced duplications during Q-RRBS
The UMIs were used in library preparation of Q-RRBS for counting genuine DNA molecules during DNA methylome profiling of DC and SC samples (). Unlike in the case of single-cell RNA-seq, the library preparation procedure for RRBS involves bisulfite treatment and the use of double-stranded adapters for adapter-ligation. Therefore, we designed a series of adapters that contained 6-base-pair (bp) identifiers featuring alternating arrangements of S/W [where S represents a cytosine (C) or guanine (G), and W an adenine (A) or thymine (T)] at their 3′ ends. This modified strategy provides 2 notable advantages. First, the use of 6-bp S/W identifiers at both the 5′ and 3′ ends yields 4,096 possible combinations of pair-ended identifiers, which is sufficient for inputs of up to hundreds of cells. Second, the adapters were designed such that the Cs and Ts were located at distinct positions within the identifiers, which eliminated the following type of error: if a C and T were located at the same position in 2 different UMIs that are otherwise identical, the C in the original identifier would be converted to a uracil (U) during bisulfite treatment and would thereafter present as a T; this would result in 2 distinct UMIs being combined into one.
Figure 1. Signatures of quantitative reduced representation bisulfite sequencing (Q-RRBS). (A) A schematic of the Q-RRBS method used for starting materials from either a trace amount of cells or single cells. Briefly, we first performed cell lysis, MspI digestion, repairing/A-tailing, UMI-adapter ligation, and bisulfite treatment in single-tube reactions, and then performed amplification, sequencing, and deduplicated analysis. Adapters containing UMIs (unique molecular identifiers) at their 3′ ends are represented by the short, variously colored boxes within the ellipse. The random-labeling step showed that both ends of each double-stranded DNA molecule randomly ligated with one of the 26 distinct UMI adapters. After deduplicated analysis, the 5 identifiers indicated that only 5 molecules were present, whereas the original analysis showed that 20 sequencing reads were present. (B) An example of single-molecular-fragment-derived (SFD) duplicates that contained identical UMIs (6-bp sequences located at both ends of black lines) and aligned to the same position of the genome. This type of SFD duplicates contained 6 fragments that displayed homogeneity of the DNA methylation pattern (same distribution of methylated CpGs in the sequencing reads). Filled circles represent methylated CpGs. (C) Of the 6,306,437 types of SFD duplicates that derived from 6,306,437 positions of the diploid genome, 98.3% displayed homogeneity of the DNA methylation pattern (shown by the example in ) in each type of the SFD duplicates.
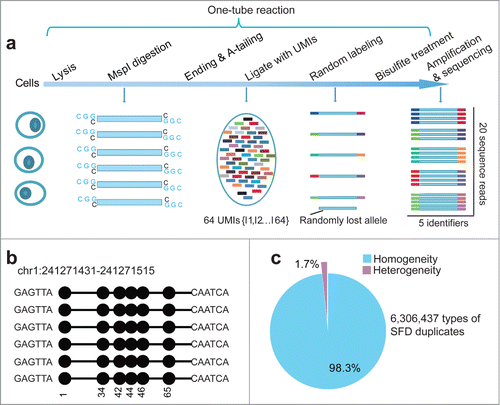
To demonstrate that these UMIs can be used to identify PCR-induced duplications derived during RRBS, we performed Q-RRBS on 30 ng of genomic DNA purified from the MCF-7 cell line (Table S1); this is considered the lowest amount of input sample that can be used in the original RRBS protocol.Citation16 Any sequencing reads that contain identical UMIs and are aligned to the same position of the genome could be considered single-molecular-fragment-derived (SFD) duplicates. Theoretically, these SFD duplicates should exhibit the same distribution of methylated or unmethylated CpGs in the sequencing read of each duplicate, which could be defined as homogeneity of the DNA methylation pattern (). In accord, when we surveyed 6,306,437 types of SFD duplicates that were derived from 6,306,437 positions in the diploid genome, we found that 98.3% of them displayed homogeneity of the DNA methylation pattern in each type of the SFD duplicates (), which indicated successful labeling of the SFD duplicates with our UMIs.
PCR-induced duplications introduce severe artifacts during RRBS of trace amounts of cells
To evaluate the proportion of PCR-induced duplications in RRBS data obtained using different numbers of amplification cycles, we performed RRBS with 2 technical replications on DC samples PCR-amplified for 40 cycles and SC samples PCR-amplified for 45 cycles, respectively (Table S1). We also performed RRBS with 2 technical replications on 30 ng of MCF-7 genomic DNA by using 18 amplification cycles. For each dataset, we aligned the original reads and the deduplicated reads, respectively, for subsequent analyses. From the samples 30 ng-1, 30 ng-2, DC-1, DC-2, SC-1, and SC-2, we obtained 8,563,772, 10,616,633, 11,612,874, 11,217,275, 1,340,399, and 277,612 original reads, and 6,690,079, 7,982,604, 6,563,712, 5,305,022, 271,580, and 123,432 deduplicated reads, respectively (Fig. S1). Therefore, the proportions of PCR-induced reads among the total number of aligned reads were 21.9%, 24.8%, 43.5%, 52.7%, 79.7%, and 55.5% for the samples 30 ng-1, 30 ng-2, DC-1, DC-2, SC-1, and SC-2, respectively. These data indicate that duplication increased as the number of amplification cycles increased.
We next assessed how duplication in RRBS data influenced the results of methylation profiling. By performing the original and deduplicated analysis for each data set, we obtained various CpGs at varying coverage depths (Table S1). For subsequent analyses of methylation levels, we selected CpGs of at least 2×, 5×, and 5× depths (as determined by analyzing the deduplicated reads) from the data obtained for the samples SC, DC, and 30 ng of MCF-7 genomic DNA, respectively. In theory, if a CpG reaches a depth of 2× through deduplicated analysis, its depth in the corresponding original dataset must be ≥2×. Consequently, this phenomenon might introduce deviations from the genuine methylation level of these CpGs, because the depth-increment induced by duplication is not removed. In , we show the genome-wide distribution and a snapshot of duplication-induced deviation between the analyses of the original and deduplicated data. When we distributed the CpGs analyzed in each original and deduplicated data set according to their various methylation levels, we found that—with the exception of sites where the methylation levels were 0 or 1—variability was present in the methylation levels of certain CpGs in the SC-1, DC-1, and 30 ng-1 samples (). These data are consistent with the conclusion that duplication does not affect the outcome of DNA methylation analyses of unmethylated and fully methylated CpGs. Therefore, we plotted the methylation levels of CpGs in the original and deduplicated data that exhibited methylation levels ranging between 20% and 80%, as determined by analyzing the deduplicated reads (). For these analyses, we used only those CpGs for which duplicate coverage was available in the original data, and the results indicated that the severity of the impact of duplication increased as the amount of starting material decreased.
Figure 2. Duplication-induced artifacts in reduced representation bisulfite sequencing (RRBS) data. (A) Deviation of coverage-depth and methylation level between original and deduplicated analysis. In the original and deduplicated analyses, the total original reads and the deduplicated reads were used for subsequent analysis, respectively. The Circos plot on left displays the methylation level and depth of 5,246 CpG sites (differential methylation level > 0.2 between original analysis (blue) and deduplicated analysis (red; coverage ≥ 2). The right plot shows a representative locus (chr1:44,401,940-44,401,987) of significant deviation between the original and deduplicated analysis. (B) Distribution of CpG sites showing different DNA methylation levels in each set of original (blue dotted lines) and deduplicated (orange solid lines) data for the single-cell (SC), dozens-of-cells (DC), and 30 ng of MCF-7 DNA samples. The y-axis shows the number of counts in each range of methylation level. (C) Scatter plots of correlation coefficients between the methylation levels determined by means of deduplicated analysis and original analysis for each sample. We selected CpGs exhibiting methylation level ranging from 20% to 80%, as determined through deduplicated analysis. Horizontal and vertical axes represent the methylation levels of CpGs determined from the deduplicated analysis and original analysis, respectively. Color bars, ranging from pink to blue, represent the increase of CpG density. Correlation coefficients are shown above the scatter plots.
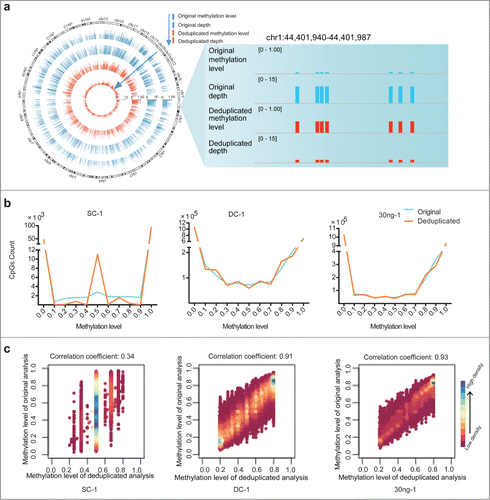
To evaluate the effects of duplication on the accuracy of DNA methylation-level analysis, we compared the differences between the methylation levels of the CpGs in the original and deduplicated datasets. We identified a total of 34,898, 117,009, and 7,357 differentially methylated CpGs (DMCs) between the original and deduplicated analysis of the 30 ng-1, DC-1, and SC-1 sequencing data, which accounted for 5.3%, 13.6%, and 64.0% of the total CpGs covered by the RRBS with depth-cutoffs from deduplicated analysis of 2×, 5×, and 5×, respectively. These results again indicate that PCR-induced duplications introduce progressively more severe artifacts as the amount of starting material decreases. Furthermore, the results also indicate that our Q-RRBS method would therefore be particularly suitable for DNA methylome profiling of SC samples.
Q-RRBS analysis can reveal the chromosomal copy number of the starting material
Our results demonstrated that UMIs can be used for identifying duplications and accurately restoring the coverage for each CpG when using trace amounts of cells or, in particular, SC samples as the starting material. By counting the CpGs at distinct coverage-depths in the deduplicated data from the SC-1 sample, we determined that 85.2%, 12.7%, and 2.1% of the CpGs detected exhibited 1×, 2×, and >2× coverage, respectively (). As indicated by the results in , during library construction for Q-RRBS of SC samples, certain MspI-digested fragments were randomly lost and this resulted in partial absence of diploid and polyploid molecules. A previous study also showed that considerable copy number variation was detected in the HEK293T cell line.Citation17 In order to identify the origin of the covered CpGs in HEK293T cells, we counted CpGs at distinct coverage-depths both from normal diploid regions (NDRs) and abnormal polyploid regions (APRs, copy number >2) (Fig. S2). In the sample SC-1, the CpG numbers in APRs were 745,512, 110,272, and 17,151, and in NDRs were 147,068, 22,790, and 4,137 for 1×, 2×, and >2× depths, respectively. Thus, the ratios of APR-derived CpG numbers to NDR-derived CpG numbers were calculated to be 5.07, 4.84, and 4.15 for coverage-depths of 1×, 2×, and >2×, respectively, in the SC-1 sample. Furthermore, similar ratios were also calculated for SC-2 (Fig. S2). This indicated that the APR-derived CpGs were more likely to be covered in Q-RRBS. Moreover, we counted the total CpGs present within and outside repetitive elements (REs) in the data obtained for HEK293T (human cell line) and N2A (mouse cell line) SC samples of different depths as determined by Q-RRBS. Our results showed non-preferential coverage of CpGs derived from REs when compared to CpGs derived from other regions lacking REs in the data for both HEK293T and N2A cell lines (Fig. S3a-b). However, APRs are frequently associated with segmental duplications, which contain REs in the human genome.Citation18 In SC-1, the numbers of CpGs in APRs that overlapped with REs were 266,219, 36,175, and 5,452, and those in NDRs that overlapped with REs were 59,992, 8,408, and 1,344 for 1×, 2×, and >2× depths, respectively. Thus, the ratios of the numbers of CpGs in APRs that overlapped with REs to the numbers of CpGs in NDRs that overlapped with REs were calculated to be 4.44, 4.30, and 4.06 for coverage-depths of 1×, 2×, and >2×, respectively, in the SC-1 sample. This result indicated that CpGs derived from APRs or APRs that overlapped with REs constituted a large part of the total CpGs covered by Q-RRBS. By exploiting the ability to identify single molecules within the SC samples by using UMIs, we were able to sequentially quantify the molecular copy number of the starting material and determine whether monoalleles or bialleles of specific regions were present in our sequencing data and, thus, we were able to accurately distinguish the methylation patterns of these regions ().
Figure 3. Analysis of quantitative reduced representation bisulfite sequencing (Q-RRBS) data obtained from HEK293T single-cell samples. (A) Bar plots of CpG counts at depths ranging from 1× to 10× after deduplicated analysis for the single-cell sample SC-1. The y-axis shows the counts of the CpGs corresponding to each distinct coverage depth. (B) Examples showing the ability of Q-RRBS to accurately identify the methylation pattern of monoalleles or bialleles for specific regions. The left plot shows that although 9 reads were aligned to the same locus of the genome from the original analysis, only the monoallele of this locus was detected from the deduplicated analysis. The right plot presents the results for certain biallelic loci that were detected by performing Q-RRBS with deduplicated analysis. (C) Circos plot of the methylation state of HEK293T cells and 9 differentiated tissues (thymus, spleen, pancreas, ovary, lung, gastric, esophagus, adrenal gland, and adipose tissues) at previously identified allele-specific DNA methylation (ASM) regions, which were obtained by using Q-RRBS and pooling the data for SC-1 and SC-2 samples. The methylation levels of CpGs from different tissues or HEK293T cells are depicted on different tracks. Red bars represent cell type-specific ASM regions that were intermediately methylated (methylation level: 0.25–0.75) in at least 5 of the 9 tissues. Here, 33 common or cell type-specific ASM regions are annotated in the core, in which the known or reported imprinted genes are shown in red. (D) Identification of maternal or paternal origin for 7 monoallelic methylation regions from the aforementioned 33 ASM regions. (E) Snapshot of methylation levels for a monoallelic methylation region (LOC101927190) in HEK293T cells, sperm, and oocytes.
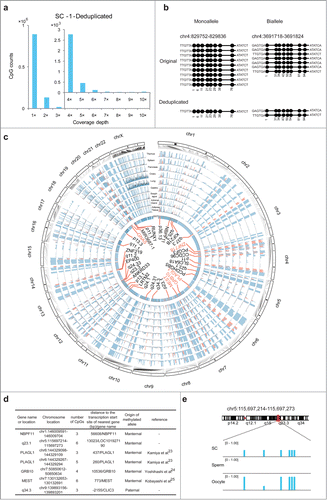
Q-RRBS can be used to identify ASMs in the absence of heterozygous SNPs
In previous studies, heterozygous SNPs were used to identify ASM regions.Citation10,19 However, in such cases, ASM regions lacking a heterozygous SNP would clearly be missed. To identify ASM regions by using Q-RRBS, deduplicated reads containing 2 different paired UMIs that mapped to unique locations were selected for subsequent analysis. Among the 95,579 and 21,378 reads featuring biallelic coverage from the SC-1 and SC-2 samples, respectively, we identified 624 regions in total that harbored at least 3 consecutive CpGs exhibiting the same methylation pattern on one allele, but divergent methylation patterns between 2 alleles (Supplemental Table S2). Four of these ASM regions were known imprinted differential methylation regions (DMRs) (GNAS, PLAGL1, GRB10, and MEST). Although most known imprinted DMRs are common to various cell types, a few of these DMRs are cell type-specific.Citation20,21 Thus, we surveyed the methylation status of the ASM regions identified by screening 9 differentiated tissues (thymus, spleen, pancreas, ovary, lung, gastric, esophagus, adrenal gland, and adipose tissues) by using information obtained from the Roadmap Epigenomics Consortium (http://roadmapepigenomics.org/). We marked each CpG site that was intermediately methylated (methylation level: 0.25–0.75) in at least 5 of the tissues (). As expected, the 4 known imprinted DMRs were common to all 9 tissues. Notably, we found that one ASM region located within the promoter of ZNF718 was also common to all 9 tissues. Although conclusive experimental data are not available for characterizing ZNF718 as an imprinting gene, our findings agree with those of a statistical model developed by Fang et al., which identified ZNF718 as a candidate imprinting gene.Citation22 Nevertheless, our results demonstrate that Q-RRBS can be used to accurately identify ASM regions by using UMIs. Furthermore, we surveyed the ASM regions by using similar criteria (present in >5/9 tissues) and identified another 28 regions (genomic annotations in black font in ) that were cell type-specific ASM regions, which might potentially be identified as imprinted DMRs based on further testing for parent-of-origin-specific effects.
Germline DMRs could be potentially serve as imprinted DMRs. Recently, Okae et al. reported that whole-genome bisulfite sequencing (WGBS) of human sperm and oocytes identified 68.6% (46/67) of the known imprinted DMRs as germline DMRs.Citation21 Thus, based on integrating these WGBS data with the 5 known imprinted DMRs and 28 cell type-specific ASM regions identified by our analyses, we predicted that the methylation of 7 of these regions, which are located upstream of the transcriptional initiation site of 6 genes, might be derived from one of the gametes (). Of these, 3 known imprinted genes, MEST,Citation23 GRB10,Citation24 and PLAGL1,Citation25 and 2 monoallelic methylation regions, which are proximal to the transcriptional start site of NBPF11 and LOC101927190 (), originated from the maternal parent. Conversely, an ASM region localized in the promoter of CLIC3 might be derived from the paternal parent.
Discussion
DNA methylation is a key form of epigenetic modification that plays crucial roles in numerous biological processes, including repression of gene transcription, maintenance of gene imprinting, X-chromosome inactivation, and repression of transposable elements.Citation26-31 RRBS is an effective method of DNA methylome profiling that has been extensively used in comparative research on DNA methylomes in mammalian cells.Citation3 Since the initial development of this method, the RRBS protocol has been modified in order to optimize it for genomic coverage, starting material, and library-construction throughput, which has resulted in new methods such as enhanced RRBS (ERRBS),Citation32 double-enzyme RRBS (dRRBS),Citation33 gel-free and multiplexed RRBS (mRRBS),Citation34 and single-cell RRBS (scRRBS).Citation9 However, each of these methods has failed to address PCR-derived duplication artifacts, which can bias the results of DNA methylation analyses. In this study, we found that 18 cycles of PCR amplification resulted in small, but acceptable, artifacts when 30 ng of genomic DNA was used as the starting material. Conversely, the use of >40 cycles of PCR amplification resulted in large amounts of error when assessing the DNA methylome particularly of single cells.
The ability to analyze the methylomes of single cells continues to attract considerable attention because pioneer cells unfailingly contain a specific marker that is indicated by DNA methylation.Citation1,2 The first single-cell epigenome analysis technique, scRRBS, enables DNA methylation analysis at single-base resolution, and can detect the methylation status of 0.5–1 million CpG sites within the genome of one cell.Citation9 Recently, when a modification of Post-Bisulfite Adaptor Tagging was used, single-cell Bisulfite Sequencing (scBS) was able to measure up to 48.4% of the CpGs present in the mouse genome.Citation35 Moreover, Farlik et al. described single-cell WGBS (scWGBS), which can cumulatively detect 90% of the CpGs in human and mouse genomes when data from several SC methylomes are combined to infer cell-state dynamics;Citation36 furthermore, without the use of redundant pre-amplification and restriction enzymes in paired-end sequencing, this approach provided duplicate read detection as an alternative to using UMIs. Similar to scRRBS, our Q-RRBS method provides less coverage of CpGs in the sequenced genome when compared to scBS and scWGBS. However, Q-RRBS for single cells and scRRBS are relatively more focused on consistent CpG-island and genome profiling, which would be suitable for comparative analysis of DNA methylomes across different single cells. Compared to scRRBS, Q-RRBS more effectively eliminated biased determination of DNA methylation levels by using UMIs. Thus, Q-RRBS is superior to scRRBS and can complement scBS and scWGBS as another methodological choice according to the specific aims of a study.
When analyzing single cells or groups of several cells, such as a zygote or blastula, identifying sequencing read which are derived from specific cells or alleles can be challenging.Citation9 Through the use of UMIs, our method provides an approach to uncover the heterogeneity of the methylation state at specific regions within different cells or alleles. Moreover, Landan et al. have developed a bioinformatics strategy for characterizing epigenetic polymorphisms within cell populations.Citation37 In the case of sequencing-read saturation, we speculate that integration of our Q-RRBS method with this bioinformatics strategy would facilitate the identification of not only cell types, but also the proportions of each cell type within trace cell tissues such as blastulas and islets, in which cells differentiate asynchronously,Citation38-40 and which consist of several different cell types,Citation41 respectively.
Previous studies of ASM regions typically required the presence of heterozygous SNPs within these regions.Citation10 However, our results demonstrated that the UMIs used in our Q-RRBS analyses enabled effective and accurate detection of ASM regions in SC samples independently of the existence of genotypic variation; by contrast, scRRBS, scBS, and scWGBS could not discriminate ASM regions in the absence of heterozygous SNPs. Therefore, our method could likely be used to identify previously unrecognized imprinted DMRs that lack heterozygous SNPs. Notably, we were also able to accurately detect genomic imprinting defects that contribute to human disease syndromes. Thus, by employing Q-RRBs for analyzing, at the SC level, the DNA methylome of embryos to be used for in vitro fertilization, Q-RRBS could potentially be used as a diagnostic tool for avoiding the implantation of cells that could lead to imprinting defect-associated syndromes.
Recently, Shipony et al. developed an RRBS method by using UMI plasmid libraries to achieve genuine quantitative profiling of DNA methylation-pattern distributions within cell populations.Citation42 However, because this method requires at least several nanograms of genomic DNA as starting material and also customized primers and a customized sequencing model on the Illumina sequencer, the applications of this approach might be limited. By contrast, basic RRBS and scRRBS protocols are used in our method with the substitution of UMI adapters. Consequently, Q-RRBS can be more readily manipulated and is more reproducible than the method developed by Shipony et al. In conclusion, Q-RRBS can be widely applied as an effective and accurate method for DNA methylome profiling of SC samples and of samples containing trace amounts of cells.
Materials and Methods
Preparation of UMI adapters
The 5-methylcytosine-modified adapter oligonucleotides used in this study (5′-ACACTCTTCCCTACAGCTCTTCCGATCTSWSWSWT-3′ and 5′-WSWSWSAGATC GGAAGAGCACACGTCT-3′; S = C or G, and W = A or T; all of the Cs in oligo are 5-methylcytosine-modified), which were designed to provide distinct UMIs as identifiers for different DNA fragments, were synthesized by Invitrogen (Shanghai, China). Double-stranded adapters were generated by diluting the single-stranded oligos to a concentration of 10 µM in 10 mM Tris-HCl (pH 8.0), 0.1 mM ethylenediaminetetraacetic acid (EDTA), and 10 mM NaCl, heating to 98°C for 2 min in a heat block, and then allowing the sample to gradually cool to room temperature.
Sample preparation
The human ovarian epithelial cell line T29 was a generous gift from Dr. Jinsong Liu (MD Anderson Cancer Center, University of Texas). MCF-7, N2A, and HEK293T cell lines were obtained from American Type Culture Collection (Manassas, VA, USA). All cells were maintained in Dulbecco's modified Eagle's medium-high glucose (Gibco, Grand Island, NY, USA) supplemented with 10% fetal bovine serum (Gibco) at 37°C with 5% CO2 and a humidified atmosphere. Genomic DNA was extracted from MCF-7 cells by using a DNeasy Blood & Tissue Kit (Qiagen, Venlo, Netherlands), and 20 mg/mL RNase (Qiagen) was used to degrade any RNA contaminants in the DNA samples. DNA integrity was verified by means of agarose gel electrophoresis, and DNA was quantified using a Quant-iT PicoGreen dsDNA Kit (Life Technologies, Carlsbad, CA, USA) and a Qubit 2.0 Fluorometer (Life Technologies).
Q-RRBS library construction
To prepare the libraries for 30-ng DNA input, we used the protocol published by Gu et al.Citation16 Cultured cells were subjected to trypsin digestion, washed in standard phosphate-buffered saline, and then serially diluted and counted. Single cells were individually handpicked (using a mouth pipette) to ensure that only one cell was processed at one time under the microscope. Samples of DC were collected using a micropipettor, according to the cell count. Precisely one cell (SC sample) and approximately DC samples were then transferred into 5 µL of CellsDirect solution (10:1 Resuspension buffer:Lysis enhancer; Invitrogen) and lysed by incubating them at 75°C for 10 min to release naked DNA. The DNA was then spiked with 1% unmethylated lambda DNA (Fermentas, Waltham, MA, USA), which translated to 60 fg for SC and 3 pg for DC given the basic understanding that, according to previous calculations, only 6 pg of DNA is present in a diploid human cell;Citation9,43 next, 15-µL aliquots were fragmented by mixing with 20 units of MspI (New England BioLabs [NEB], Ipswich, MA, USA) and CutSmart buffer (NEB) and incubating for 4 h at 37°C. After incubation, reactions were terminated by adding 0.5 µL of 150 mg/mL protease solution (Qiagen) and incubating at 50°C for 1 h to allow optimal digestion of MspI, and this was followed by 30 min at 75°C for heat inactivation of proteases. To fill in the 5′ overhangs and to adenylate the MspI-digested DNA, 20-µL aliquots were treated with 5 U of Klenow fragment (3′-5′exo-; Enzymatics Inc., Beverly, MA, USA) in the presence of 1 mM dATP, 0.1 mM dGTP, and 0.1 mM dCTP (all 3 from Fermentas). Reactions were incubated for 30 min at 30°C for optimal end repair and then for 30 min at 37°C for optimal A-tailing, after which the reactions were terminated through heat-inactivation (15 min, 75°C).
To generate UMI adapter-linked DNA fragments, 1.5 µL of the double-stranded adapters (1 µM) described above were ligated to the dA-tailed DNA fragments in 25-µL reactions containing 0.5 µL of 2000 U/µL T4 DNA Ligase (Enzymatics), 2.5 µL of 10 mM ATP (Life Technologies), and 0.5 µL of CutSmart buffer (NEB). The ligation reaction was incubated at 16°C overnight and then heat-inactivated (10 min, 65°C). Next, bisulfite conversion was performed in 150-µL reactions by using the EZ DNA Methylation-Lightning™ Kit (Zymo Research, Irvine, CA, USA) according to the manufacturer's standard protocol: 125 µL of ready-to-use Lightning Conversion Reagent was added to 25 µL of a library in a single tube, and bisulfite conversion was performed on the thermocycler as follows: at 98°C for 8 min, then at 54°C for 1 h, and finally at 4°C for temporary storage. After bisulfate treatment, the converted DNA was subjected to on-column desulfonation for at least 15 min at room temperature as per manufacturer instructions, and 3 µg of carrier RNA (Qiagen) was added to the supplied M-Binding Buffer before purification to increase the overall yield. The DNA was subsequently eluted twice from the Zymo spin column by using 11.5 µL of pre-heated (50°C) elution buffer.
The total yield of purified DNA was then subjected to 2 rounds of PCR amplification. All PCR primers are listed in Supplemental Table S3. The initial reactions were performed in 25 μL with PCR Round 1 Primer (final concentration: 300 nM) by using KAPA HiFi HotStart Uracil+ ReadyMix (KAPA Biosystems, Wilmington, MA, USA). The following amplification conditions were used: 2 min at 98°C, followed by 20 cycles of 30 s at 98°C, 30 s at 65°C, and 1 min at 72°C, and a final extension for 7 min at 72°C. PCR products were then size-selected by means of 2% NuSieve 3:1 agarose gel electrophoresis and purified using a MinElute gel extraction kit (Qiagen). After clean-up, the optimal, minimum PCR cycle number required to generate the final libraries was assessed by using diagnostic PCRs for each library. The size-selected DNA fragments were then subjected to a second round of PCR in 25-μL reactions with PCR Round 2 Primer (final concentration: 300 nM) by using KAPA HiFi HotStart ReadyMix (KAPA Biosystems) and the following thermocycler conditions: 98°C for 2 min, followed by 21–25 cycles of 98°C for 30 s, 65°C for 30 s, and 72°C for 1 min, and a final extension for 7 min a 72°C. PCR products were subjected to a final size-selection step on a 2% NuSieve 3:1 agarose gel, and then 2 gel bands, ranging between 200–300 and 300–500 bp, were excised. The final RRBS libraries were recovered from the gel by using the MinElute gel extraction kit and quantified using a Qubit Fluorometer and the Qubit® dsDNA HS Assay Kit (Life Technologies).
Sequencing and alignment
Libraries were quantified using a standard curve-based qPCR assay (KAPA Biosystems) and Agilent Bioanalyzer 2100 (Agilent Technologies, Loveland, CO, USA). The quality-ensured libraries were then loaded on an Illumina HiSeq 2000 analyzer (Illumina, Inc., San Diego, CA, USA) at final concentration of approximately 8 pM with cluster densities at 75–85% of that used in regular sequencing. All clusters that passed the filter were converted into FASTQ files by using a standard Illumina pipeline.
The raw paired-end FASTQ reads were trimmed to remove both the adapter sequences and low-quality bases. The UMI sequences were extracted as tags of the reads. The remaining truncated reads were then aligned to the hg19 human reference genome (downloaded from UCSC Genome Browser) with the Bismark tool.Citation44 (http://www.bioinformatics.bbsrc.ac.uk/projects/bismark/) by using the default parameters and applying a customized pairwise-alignment Perl script. Uniquely aligned reads that contained MspI digestion sites at their ends were retained for further analysis. The 48,502-bp lambda DNA genome was built as an extra reference to calculate the bisulfite conversion rate. For RRBS of 30 ng of starting material, bisulfite conversion rates were calculated as the number of genomic cytosines outside a CpG context that were unconverted, divided by the total number of cytosines outside a CpG context.
To demonstrate that DNA contamination did not occur in our method, we preformed single-cell Q-RRBS not only on human cells, but also mouse cells (Table S4). We used the same parameters and mapped the mouse data to the human genome or the human data to the mouse genome, and found that the mapping rate was low when these approaches was used, which agrees with the scRRBS data obtained by Guo et al.Citation9,10 (Fig. S4). Furthermore, we also used negative controls in which only the carryover buffer was transferred into the lysis buffer, and we performed all of the remaining steps in the same manner as in the case of single cells. This revealed that the contamination in the negative-control samples was also negligible (Fig. S4). These results demonstrated that major DNA-contamination problems do not affect our method.
Bioinformatics analysis
Any original reads that aligned to the same location of the reference and contained the same pair-ended UMIs were considered duplicates. In such cases, only one of the reads was retained and used as the deduplicated read. The original and deduplicated reads were used for subsequent analyses. To estimate the CpG coverage in the RRBS data sets, we simply added up the CpG sites, but only if these CpG sites were captured at least once in the samples. However, when computing the methylation level of the libraries, we selected only the CpG sites that featured coverage-cutoffs after analysis of the deduplicated reads of 2×, 5×, and 5× for the samples SC, DC, and 30 ng of MCF-7 DNA, respectively. The methylation level of each CpG site was estimated as the number of reported Cs (methylated) divided by the total number of reported Cs (methylated) or Ts (unmethylated) at the same position of the reference genome. A CpG site was considered a DMC if a difference existed between the methylation state in the original and deduplicated reads, with an absolute difference of ≥0.1 and a fold-change of ≥1.2. Because 2 copies of each allele are typically present in single cells (one from each parent), the CpGs present in the deduplicated reads from the SC samples covering 2× depth were used for ASM analysis. CpG sites exhibiting ASM were characterized as sites showing 50% methylation, and ASM regions were defined as regions containing at least 3 uninterrupted ASM CpG sites. To investigate the methylation states of known DMRs covered in our Q-RRBS data for SC-1 and SC-2, we examined our sequences for the known DMR regions listed in the Imprinted Gene Databases (http://www.geneimprint.com/site/genes-by-species). Lastly, to further decipher the origins of the ASM regions, we combined our SC data with previous methylation data from germ cells.Citation21
Disclosure of Potential Conflicts of Interest
No potential conflicts of interest were disclosed.
Author Contributions
KW and SD constructed libraries for Q-RRBS. JL, CZ, and HW performed cell culture and micromanipulation for isolating single-cells. XL and FM performed bioinformatics analysis. KW, XL, and WC wrote the manuscript. WC, ZS, and JW conceived the study, participated in its design, and supervised the entire project. All authors read and approved the final manuscript.
1075690_Supplemental_Material.zip
Download Zip (764.7 KB)Acknowledgments
We thank Teng Huajing and Fang Jianhuo for scientific advice that helped improve our manuscript. We thank Editage (http://www.editage.cn/) for their editing of the English in this manuscript.
Supplemental Material
Supplemental data for this article can be accessed on the publisher's website.
Funding
This work was supported by grants from the National High Technology Research and Development Program of China (No. 2012AA02A202, No. 2012AA02A201) and the Key Project of the National 12th Five Year Research Program of China (No. 2012BAI03B02).
References
- Esteller M. Cancer epigenomics: DNA methylomes and histone-modification maps. Nat Rev Genet 2007; 8:286-98; PMID:17339880; http://dx.doi.org/10.1038/nrg2005
- Smith ZD, Chan MM, Mikkelsen TS, Gu H, Gnirke A, Regev A, Meissner A. A unique regulatory phase of DNA methylation in the early mammalian embryo. Nat 2012; 484:339-44; PMID:22456710; http://dx.doi.org/10.1038/nature10960
- Harris RA, Wang T, Coarfa C, Nagarajan RP, Hong C, Downey SL, Johnson BE, Fouse SD, Delaney A, Zhao Y, et al. Comparison of sequencing-based methods to profile DNA methylation and identification of monoallelic epigenetic modifications. Nat Biotechnol 2010; 28:1097-105; PMID:20852635; http://dx.doi.org/10.1038/nbt.1682
- Smallwood SA, Kelsey G. Genome-wide analysis of DNA methylation in low cell numbers by reduced representation bisulfite sequencing. Methods Mol Biol 2012; 925:187-97; PMID:22907498; http://dx.doi.org/10.1007/978-1-62703-011-3_12
- Ramskold D, Luo S, Wang YC, Li R, Deng Q, Faridani OR, Daniels GA, Khrebtukova I, Loring JF, Laurent LC, et al. Full-length mRNA-Seq from single-cell levels of RNA and individual circulating tumor cells. Nat Biotechnol 2012; 30:777-82; PMID:22820318; http://dx.doi.org/10.1038/nbt.2282
- Ni X, Zhuo M, Su Z, Duan J, Gao Y, Wang Z, Zong C, Bai H, Chapman AR, Zhao J, et al. Reproducible copy number variation patterns among single circulating tumor cells of lung cancer patients. Proc Natl Acad Sci USA 2013; 110:21083-8; PMID:24324171; http://dx.doi.org/10.1073/pnas.1320659110
- Potter NE, Ermini L, Papaemmanuil E, Cazzaniga G, Vijayaraghavan G, Titley I, Ford A, Campbell P, Kearney L, Greaves M. Single-cell mutational profiling and clonal phylogeny in cancer. Genome Res 2013; 23:2115-25; PMID:24056532; http://dx.doi.org/10.1101/gr.159913.113
- Awong G, Zuniga-Pflucker JC. Thymus-bound: the many features of T cell progenitors. Front Biosci 2011; 3:961-9; PMID:21622245; http://dx.doi.org/10.2741/200
- Guo H, Zhu P, Wu X, Li X, Wen L, Tang F. Single-cell methylome landscapes of mouse embryonic stem cells and early embryos analyzed using reduced representation bisulfite sequencing. Genome Res 2013; 23:2126-35; PMID:24179143; http://dx.doi.org/10.1101/gr.161679.113
- Guo H, Zhu P, Yan L, Li R, Hu B, Lian Y, Yan J, Ren X, Lin S, Li J, et al. The DNA methylation landscape of human early embryos. Nature 2014; 511:606-10; PMID:25079557; http://dx.doi.org/10.1038/nature13544
- Smith ZD, Chan MM, Humm KC, Karnik R, Mekhoubad S, Regev A, Eggan K, Meissner A. DNA methylation dynamics of the human preimplantation embryo. Nature 2014; 511:611-5; PMID:25079558; http://dx.doi.org/10.1038/nature13581
- Aird D, Ross MG, Chen WS, Danielsson M, Fennell T, Russ C, Jaffe DB, Nusbaum C, Gnirke A. Analyzing and minimizing PCR amplification bias in Illumina sequencing libraries. Genome Biol 2011; 12:R18; PMID:21338519; http://dx.doi.org/10.1186/gb-2011-12-2-r18
- Kivioja T, Vaharautio A, Karlsson K, Bonke M, Enge M, Linnarsson S, Taipale J. Counting absolute numbers of molecules using unique molecular identifiers. Nat Methods 2012; 9:72-4; PMID:22101854; http://dx.doi.org/10.1038/nmeth.1778
- Shiroguchi K, Jia TZ, Sims PA, Xie XS. Digital RNA sequencing minimizes sequence-dependent bias and amplification noise with optimized single-molecule barcodes. Proc Natl Acad Sci USA 2012; 109:1347-52; PMID:22232676; http://dx.doi.org/10.1073/pnas.1118018109
- Islam S, Zeisel A, Joost S, La Manno G, Zajac P, Kasper M, Lonnerberg P, Linnarsson S. Quantitative single-cell RNA-seq with unique molecular identifiers. Nat Methods 2014; 11:163-6; PMID:24363023; http://dx.doi.org/10.1038/nmeth.2772
- Gu H, Bock C, Mikkelsen TS, Jager N, Smith ZD, Tomazou E, Gnirke A, Lander ES, Meissner A. Genome-scale DNA methylation mapping of clinical samples at single-nucleotide resolution. Nat Methods 2010; 7:133-6; PMID:20062050; http://dx.doi.org/10.1038/nmeth.1414
- Lin YC, Boone M, Meuris L, Lemmens I, Van Roy N, Soete A, Reumers J, Moisse M, Plaisance S, Drmanac R, et al. Genome dynamics of the human embryonic kidney 293 lineage in response to cell biology manipulations. Nat Commun 2014; 5:4767; PMID:25182477; http://dx.doi.org/10.1038/ncomms5767
- Redon R, Ishikawa S, Fitch KR, Feuk L, Perry GH, Andrews TD, Fiegler H, Shapero MH, Carson AR, Chen W, et al. Global variation in copy number in the human genome. Nature 2006; 444:444-54; PMID:17122850; http://dx.doi.org/10.1038/nature05329
- Court F, Tayama C, Romanelli V, Martin-Trujillo A, Iglesias-Platas I, Okamura K, Sugahara N, Simon C, Moore H, Harness JV, et al. Genome-wide parent-of-origin DNA methylation analysis reveals the intricacies of human imprinting and suggests a germline methylation-independent mechanism of establishment. Genome Res 2014; 24:554-69; PMID:24402520; http://dx.doi.org/10.1101/gr.164913.113
- Liu J, Yu S, Litman D, Chen W, Weinstein LS. Identification of a methylation imprint mark within the mouse Gnas locus. Mol Cell Biol 2000; 20:5808-17; PMID:10913164; http://dx.doi.org/10.1128/MCB.20.16.5808-5817.2000
- Okae H, Chiba H, Hiura H, Hamada H, Sato A, Utsunomiya T, Kikuchi H, Yoshida H, Tanaka A, Suyama M, et al. Genome-wide analysis of DNA methylation dynamics during early human development. PLoS Genetics 2014; 10:e1004868; PMID:25501653; http://dx.doi.org/10.1371/journal.pgen.1004868
- Fang F, Hodges E, Molaro A, Dean M, Hannon GJ, Smith AD. Genomic landscape of human allele-specific DNA methylation. Proc Natl Acad Sci USA 2012; 109:7332-7; PMID:22523239; http://dx.doi.org/10.1073/pnas.1201310109
- Kobayashi S, Kohda T, Miyoshi N, Kuroiwa Y, Aisaka K, Tsutsumi O, Kaneko-Ishino T, Ishino F. Human PEG1/MEST, an imprinted gene on chromosome 7. Hum Mol Genet 1997; 6:781-6; PMID:9158153; http://dx.doi.org/10.1093/hmg/6.5.781
- Yoshihashi H, Maeyama K, Kosaki R, Ogata T, Tsukahara M, Goto Y, Hata J, Matsuo N, Smith RJ, Kosaki K. Imprinting of human GRB10 and its mutations in two patients with Russell-Silver syndrome. Am J Hum Genet 2000; 67:476-82; PMID:10856193; http://dx.doi.org/10.1086/302997
- Kamiya M, Judson H, Okazaki Y, Kusakabe M, Muramatsu M, Takada S, Takagi N, Arima T, Wake N, Kamimura K, et al. The cell cycle control gene ZAC/PLAGL1 is imprinted–a strong candidate gene for transient neonatal diabetes. Hum Mol Genet 2000; 9:453-60; PMID:10655556; http://dx.doi.org/10.1093/hmg/9.3.453
- Bird A. DNA methylation patterns and epigenetic memory. Genes Dev 2002; 16:6-21; PMID:11782440; http://dx.doi.org/10.1101/gad.947102
- Fulka H, Mrazek M, Tepla O, Fulka J, Jr. DNA methylation pattern in human zygotes and developing embryos. Reproduction 2004; 128:703-8; PMID:15579587; http://dx.doi.org/10.1530/rep.1.00217
- Li E, Beard C, Jaenisch R. Role for DNA methylation in genomic imprinting. Nature 1993; 366:362-5; PMID:8247133; http://dx.doi.org/10.1038/366362a0
- Hackett JA, Surani MA. DNA methylation dynamics during the mammalian life cycle. Philos Trans R Soc Lond Biol Sci 2013; 368:20110328; PMID:23166392; http://dx.doi.org/10.1098/rstb.2011.0328
- Lister R, Pelizzola M, Dowen RH, Hawkins RD, Hon G, Tonti-Filippini J, Nery JR, Lee L, Ye Z, Ngo QM, et al. Human DNA methylomes at base resolution show widespread epigenomic differences. Nature 2009; 462:315-22; PMID:19829295; http://dx.doi.org/10.1038/nature08514
- Wu H, Zhang Y. Early embryos reprogram DNA methylation in two steps. Cell Stem Cell 2012; 10:487-9; PMID:22560071; http://dx.doi.org/10.1016/j.stem.2012.04.012
- Akalin A, Garrett-Bakelman FE, Kormaksson M, Busuttil J, Zhang L, Khrebtukova I, Milne TA, Huang Y, Biswas D, Hess JL, et al. Base-pair resolution DNA methylation sequencing reveals profoundly divergent epigenetic landscapes in acute myeloid leukemia. PLoS Genetics 2012; 8:e1002781; PMID:22737091; http://dx.doi.org/10.1371/journal.pgen.1002781
- Wang J, Xia Y, Li L, Gong D, Yao Y, Luo H, Lu H, Yi N, Wu H, Zhang X, et al. Double restriction-enzyme digestion improves the coverage and accuracy of genome-wide CpG methylation profiling by reduced representation bisulfite sequencing. BMC Genomics 2013; 14:11; PMID:23324053; http://dx.doi.org/10.1186/1471-2164-14-11
- Boyle P, Clement K, Gu H, Smith ZD, Ziller M, Fostel JL, Holmes L, Meldrim J, Kelley F, Gnirke A, et al. Gel-free multiplexed reduced representation bisulfite sequencing for large-scale DNA methylation profiling. Genome Biol 2012; 13:R92; PMID:23034176; http://dx.doi.org/10.1186/gb-2012-13-10-r92
- Smallwood SA, Lee HJ, Angermueller C, Krueger F, Saadeh H, Peat J, Andrews SR, Stegle O, Reik W, Kelsey G. Single-cell genome-wide bisulfite sequencing for assessing epigenetic heterogeneity. Nat Methods 2014; 11:817-20; PMID:25042786; http://dx.doi.org/10.1038/nmeth.3035
- Farlik M, Sheffield NC, Nuzzo A, Datlinger P, Schonegger A, Klughammer J, Bock C. Single-cell DNA methylome sequencing and bioinformatic inference of epigenomic cell-state dynamics. Cell Reports 2015; 10:1386-97; PMID:25732828; http://dx.doi.org/10.1016/j.celrep.2015.02.001
- Landan G, Cohen NM, Mukamel Z, Bar A, Molchadsky A, Brosh R, Horn-Saban S, Zalcenstein DA, Goldfinger N, Zundelevich A, et al. Epigenetic polymorphism and the stochastic formation of differentially methylated regions in normal and cancerous tissues. Nat Genet 2012; 44:1207-14; PMID:23064413; http://dx.doi.org/10.1038/ng.2442
- Tang F, Barbacioru C, Wang Y, Nordman E, Lee C, Xu N, Wang X, Bodeau J, Tuch BB, Siddiqui A, et al. mRNA-Seq whole-transcriptome analysis of a single cell. Nat Methods 2009; 6:377-82; PMID:19349980; http://dx.doi.org/10.1038/nmeth.1315
- Bengtsson M, Stahlberg A, Rorsman P, Kubista M. Gene expression profiling in single cells from the pancreatic islets of Langerhans reveals lognormal distribution of mRNA levels. Genome Res 2005; 15:1388-92; PMID:16204192; http://dx.doi.org/10.1101/gr.3820805
- Webb SE, Miller AL. Ca2+ signaling and early embryonic patterning during the blastula and gastrula periods of zebrafish and Xenopus development. Biochim Biophys Acta 2006; 1763:1192-208; PMID:16962186; http://dx.doi.org/10.1016/j.bbamcr.2006.08.004
- Brissova M, Fowler MJ, Nicholson WE, Chu A, Hirshberg B, Harlan DM, Powers AC. Assessment of human pancreatic islet architecture and composition by laser scanning confocal microscopy. J Histochem Cytochem 2005; 53:1087-97; PMID:15923354; http://dx.doi.org/10.1369/jhc.5C6684.2005
- Shipony Z, Mukamel Z, Cohen NM, Landan G, Chomsky E, Zeliger SR, Fried YC, Ainbinder E, Friedman N, Tanay A. Dynamic and static maintenance of epigenetic memory in pluripotent and somatic cells. Nature 2014; 513:115-9; PMID:25043040; http://dx.doi.org/10.1038/nature13458
- Liang J, Cai W, Sun Z. Single-cell sequencing technologies: current and future. J Genet Genomics 2014; 41:513-28; PMID:25438696; http://dx.doi.org/10.1016/j.jgg.2014.09.005
- Krueger F, Andrews SR. Bismark: a flexible aligner and methylation caller for Bisulfite-Seq applications. Bioinformatics 2011; 27:1571-2; PMID:21493656; http://dx.doi.org/10.1093/bioinformatics/btr167