Abstract
With the goal of studying epigenetic alterations in fibrolamellar hepatocellular carcinoma (FLC) and establish an associated DNA methylation signature, we analyzed LINE-1 methylation in a cohort of FLC and performed next-generation sequencing of DNA methylation in a training set of pure-FLCs and non-cirrhotic hepatocellular carcinomas (nc-HCC). DNA methylation was correlated with gene expression. Furthermore, we established and validated an epigenetic signature differentiating pure-FLC from other HCCs. LINE-1 methylation correlated with shorter recurrence-free survival and overall survival in resected pure-FLC patients. Unsupervised clustering using CG sites located in islands distinguished pure-FLC from nc-HCC. Major DNA methylation changes occurred outside promoters, mainly in gene bodies and intergenic regions located in the vicinity of liver developmental genes (i.e., SMARCA4 and RXRA). Partially methylated domains were more prone to DNA methylation changes. Furthermore, we identified several putative tumor suppressor genes (e.g., DLEU7) and oncogenes (e.g., DUSP4). While ∼70% of identified gene promoters gaining methylation were marked by bivalent histone marks (H3K4me3/H3K27me3) in embryonic stem cells, ∼70% of those losing methylation were marked by H3K4me3. Finally, we established a pure FLC DNA methylation signature and validated it in an independent dataset. Our analysis reveals a distinct epigenetic signature of pure FLC as compared to nc-HCC, with DNA methylation changes occurring in the vicinity of liver developmental genes. These data suggest new options for targeting FLC based on cancer epigenome aberrations.
Introduction
Fibrolamellar hepatocellular carcinoma (FLC) is a rare sub-type of primary liver carcinomas, often arising in adolescents and young adults, without any identified risk factor or underlying cirrhosis.Citation1 The gold standard of diagnosis relies on Edmondson pathological triad.Citation2 However, there is poor reproducibility among experts for histological diagnosis of primary liver tumors with fibrous stroma in non-cirrhotic patients, including FLC.Citation3 Reasons may be related to tumor heterogeneity and the existence of morphological variants of FLC within classical hepatocellular carcinoma (HCC).Citation1
In 2012, we proposed to classify FLC in 2 entities: pure-FLC (p-FLC) and mixed-FLC (m-FLC).Citation4 We showed that p-FLC usually occurs in young patients (<30 years), which often present lymph node metastasis at the time of diagnosis and frequently experience extra-hepatic recurrences. Conversely, m-FLC occurs in older patients and frequently involves the liver as the primary site of disease recurrence.Citation4 However, the morphological distinction between those entities is of great interest to individualize patient treatments, biomarkers are needed to distinguish p-FLC from m-FLC and other HCC arising in non-cirrhotic liver (nc-HCC).Citation4 Using transcriptomic profiling of a large set of p-FLC, m-FLC and HCC arising in non-cirrhotic liver, we and others recently showed that p-FLC harbor a unique signature characterized by the strong expression of specific neuroendocrine genes (i.e., PCSK1, DNER, CALCA and NTS).Citation5-7
Meanwhile, a recurrent DNAJB1-PRKACA chimeric transcript has been identified in a data set of 15 FLC cases (100%) suggesting that this genetic alteration contributes to tumor pathogenesis.Citation8 However, whether this translocation is pathognomonic for the diagnosis remains unclear. Indeed, in the Cornella et al. study, which reported on 77 FLC, the presence of fusion transcript was found in 79% of cases.Citation5 Conversely, in the Graham et al. study, rearrangements of the PRKACA locus were seen in all 19 FLC cases (100%).Citation9
Beside the specificity of DNAJB1-PRKACA transcript in support of diagnosis of FLC, the role of epigenetics alterations in shaping FLC identity and distinguishing p-FLC from other HCC subtypes remains limited. As DNA methylation is a defining trait of cellular identity in mammalian cells and as most dynamic regulations of normal development occur in CG sites distal to transcription start sites,Citation10 we thus decided to investigate genome-wide DNA methylation changes in p-FLC as compared to nc-HCC and normal livers.
Historically, rarity of FLC hampers its comprehensive genomic and epigenetic characterization. Furthermore, the majority of series reporting to date genomic and epigenomic features of FLC involved limited number of cases and were contradictory.Citation11-13 Recently, the development of genome-wide sequencing of DNA methylation illuminate our understanding of the plasticity of DNA methylation during different physiological process as well as differentiation of embryonic stem cells and cancer.Citation10,14,Citation15 For instance, during the differentiation of embryonic stem cells into fibroblasts, DNA methylation changes have been shown to occur in majority outside of core promoters, in partially methylated domains (PMDs), which represent large hypomethylated regions covering almost 40% of our genome.Citation14 However, little is known about dynamic changes of PMDs following liver carcinogenesis in general, and FLC in particular.
To clarify the situation, we thus decided to analyze global DNA methylation in a cohort of patients resected for FLC. In addition, we performed the first next-generation sequencing of DNA methylation in p-FLC and nc-HCC and reported specific DNA methylation signature of p-FLC.
Methods
Patients and samples
We analyzed a subset of a previously reported cohort encompassing 22 p-FLC and 6 m-FLC from patients which underwent surgical resection between January 1, 1987 and December 31, 2007 at 2 French referral centers (Beaujon University Hospital and Bicêtre hospital) (Table S1).Citation4 Furthermore, 10 nc-HCC and 13 adjacent normal livers were also obtained as control. p-FLC and m-FLC were reviewed by 2 expert pathologists (VP and MF), as previously described.Citation16 All tumor samples were de-identified, collected as the CIT (Cartes d'Identité des tumeurs) cohort, stored and used with the informed consent from the patients or their parents. For the 5 pediatric p-FLC, 2 areas of the primary tumor have been collected (Table S1). The transcriptomic signature for 39 liver samples with available RNA has been previously reported (Table S1).Citation6 Those include a total of 29 primary tumors (17 p-FLCs, 5 m-FLCs and 7 nc-HCC) and 10 tumor-adjacent normal livers.Citation6 Raw data regarding the gene expression of those cases have been used to correlate DNA methylation with gene expression changes. All patients had curative liver resection. Tumor recurrence was based on typical CT and MRI features or histological confirmation.
Fusion transcript detection and RT-PCR
RNA was available for 19 p-FLCs (corresponding to 17 patients), 5 m-FLCs, 7 nc-HCC and 10 normal livers. The presence of the DNAJB1-PRKACA recurrent fusion transcripts was searched in those cases by RT-PCR followed by Sanger sequencing as previously reported by Honeyman et al.Citation8 RT-PCR analysis to validate SMARCA4 and RXRA expression were done using Taqman gene expression assays Hs00231324_m1 and Hs01067640_m1, respectively. Briefly, RNA was converted to cDNA using High Capacity RNA-to-cDNA Kit (Applied Biosystems). RT-PCR was then performed using TaqMan® Universal Master Mix II (Applied Biosystems) according to manufacturer instructions. Eukaryotic 18S rRNA endogenous was used as control.
Digital Restriction Enzyme Analysis of Methylation (DREAM) method
Next-generation sequencing of DNA methylation was performed for 4 p-FLC, 1 m-FLC, 2 nc-HCCs and 2 normal livers (), as previously described.Citation17 Briefly, genomic DNA (5 μg) was sequentially digested with FastDigest SmaI endonuclease (Fermentas) and XmaI endonuclease (NEB). The digested DNA was purified and the 3′ recessed ends of the DNA created by XmaI digestion were filled in with 3′-dA tails. This was followed by deep sequencing on an Illumina Genome Analyzer II. Sequencing reads were mapped to SmaI sites in the human genome (hg18), and signatures corresponding to methylated and unmethylated CpGs were listed for each SmaI site. Methylation frequencies for individual were then calculated. The methylation ratio of each individual SmaI sites was calculated as the number of tags starting with CCGGG divided by the total number of tags mapped to a given SmaI site.Citation17
Table 1. List of cases assessed by DREAM for DNA methylation
Analysis of DNA methylation changes
We used at least 10 sequencing reads to analyze methylation levels at individual SmaI sites and analyzed promoter gain and loss of DNA methylation. Promoters were defined as regions between −2000 base pair (bp) from transcription start site (TSS) to +2000 bp from TSS for each Refseq transcript. Overall, using those criteria, 10896 common CG sites in promoter CG islands were covered in all samples processed. If we restrict the criteria to CG sites between −1000 and +1000 from TSS, 9750 CG sites were covered by DREAM. To calculate promoter methylation, we averaged the methylation level of all CpG sites located between −2000 bp and +2000 bp from transcription start site.
Spearman correlation of DNA methylation of CG sites in the 2 normal livers was performed using at least 10 tags coverage per CG sites, and after excluding CG sites located on X and Y chromosomes.
Based on technical replicate experiments, and with a least 10-fold coverage, DREAM can detect differences in methylation greater than 15% with a false positive rate below 0.03 (Fig. S1A).Citation17 Thus, the cut-off of 15% methylation was used to analyze CG sites that are differentially methylated between p-FLC group from one side and nc-HCC and normal liver group on the other side.
Unsupervised clustering of DNA methylation was performed using Array-Track according to the default setting, using the most variable probes.
Statistical analysis was performed using GraphPad Prism 5.0. P-values <0.05 were considered statistically significant.
Pyrosequencing
Methylation of LINE-1 was assessed as previously described.Citation18 Tumors with LINE-1 hypomethylation were defined as those with LINE-1 methylation level inferior to the mean overall level of the whole p-FLC population minus one standard error of mean (SEM).
Methylation of selected genes was performed by quantitative bisulfite pyrosequencing on the training set (n = 4) and then in an independent set of 23 p-FLC (corresponding to 19 patients), 5 m-FLC and 7 HCC arising in non-cirrhotic liver, using the protocol described previously.Citation19 The list of all primers used in this study is provided in Table S2.
Results
DNAJB1-PRKACA is specific for the diagnosis of pure-FLC with endocrine signature
We firstly assessed the presence of the oncogenic DNAJB1-PRKACA fusion transcript in all samples for which RNA was available. Overall, 14 out of 17 (82.3%) primary p-FLC showed presence of the DNAJB1-PRKACA oncogenic fusion transcript as compared to none of m-FLC, nc-HCC and tumor-adjacent normal livers. Of note, the 3 p-FLC cases which were negative for DNAJB1-PRKACA were previously considered as outliers in our p-FLC transcriptomic classification.Citation6 Clinically, those were related to oldest patients of our cohort. Biologically, those cases did not display endocrine signature defined by the overexpression of PCSK1, DNER, CALCA and NTS genes.Citation6 We thus conclude that DNAJB1-PRKACA is specific for the diagnosis of p-FLC.
LINE-1 methylation predicts patient outcome
We then asked whether LINE-1 methylation, a surrogate marker of global DNA methylation, was associated with clinicopathological features of patients with resected p-FLC (n = 20). Average LINE-1 methylation in p-FLC (65.31% ± 1.49%) was overall slightly different from normal liver (69.27% ± 0.53%) (P = 0.046) (). Six out of the 20 cases of p-FLC showed LINE-1 hypomethylation (). Analysis of clinicopathological features of those tumors showed that even though they did not differ in term of AJCC stage from tumors without LINE-1 hypomethylation (P = 0.2), they were of bigger size (P = 0.001), showed more micro-satellites nodules (P = 0.0007) and had tendency to present with vascular invasion (P = 0.08) and as multiple tumors (P = 0.09) (Table S3). Interestingly, patients with tumors harboring low LINE-1 methylation had poor recurrence-free survival (RFS) and overall survival (OS) as compared to others (). Thus, we conclude that p-FLC with global hypomethylation may be more aggressive and share molecular alterations distinguishing them from others.
Figure 1. (A) Distribution of LINE-1 methylation levels in pure fibrolamellar carcinoma (p-FLC) and normal adjacent liver. Each dot represents the methylation level of individual samples. Horizontal lines represent the mean methylation levels for each group. (B) Kaplan-Meier curves for recurrence-free survival in low LINE-1 methylation group (n = 6) versus intermediate and high LINE-1 methylation group (n = 14). (C) Kaplan-Meier curves for overall survival in low LINE-1 methylation group (n = 6) vs. intermediate and high LINE-1 methylation group (n = 14).
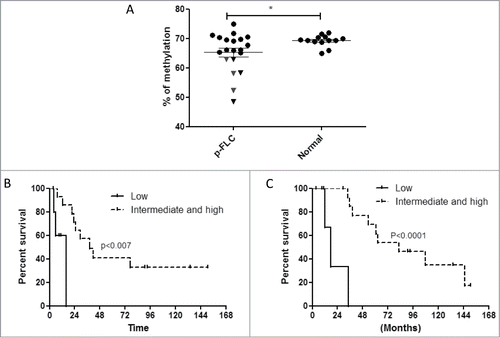
Identification of putative tumor suppressor genes
To identify putative tumor suppressor genes (TSG), we selected 4 p-FLC including 2 cases with low LINE-1 methylation (FLC-2 and FLC-110), and 2 cases with minimal change in LINE-1 methylation (FLC-107 and FLC-133). Furthermore, as a control we used 1 nc-HCC (HCC-29) and 1 m-FLC (HCC-13) with dramatic LINE-1 hypomethylation as compared to normal liver (methylation levels of 36% and 41%, respectively); in addition, we also performed DNA methylation analysis of one nc-HCC (HCC-32) with sub-normal LINE-1 methylation (methylation level of 61%).
Using DREAM, we've been able to generate quantitative analysis of DNA methylation of selected CG sites with an average of 27,788,966 tags obtained per sample (). Overall, using a minimum threshold of 3 tags/ site (median: 29-233 tags/site), we obtained quantitative methylation results for 53,335 CG sites (). Using a minimum threshold of 10 tags at least per site, we covered 28,549 CG sites with a median coverage ranging from 87 to 647 tags per site, allowing us to analyze DNA methylation changes at deep resolution. DNA methylation levels of the 2 normal livers were almost identical suggesting no epigenetic alterations within normal favoring p-FLC development (Spearman correlation = 0.95) (P < 0.0001) ().
Figure 2. (A) Correlation of DNA methylation % within CG sites detected by DREAM in 2 normal liver samples. Minimum coverage 10+ reads. Spearman r = 0.95, p < 0.0001. (B) Bar graphs of the number of genes gaining DNA methylation in each pure fibrolamellar carcinoma (p-FLC) and non-cirrhotic hepatocellular carcinoma (HCC) sample. (C) Bar graphs of the number of genes losing DNA methylation in each p-FLC and HCC sample. (D) Unsupervised clustering analysis using differentially methylated CG sites located in CG islands (CGI). (E) Unsupervised clustering analysis using differentially methylated CG sites located outside CGI.
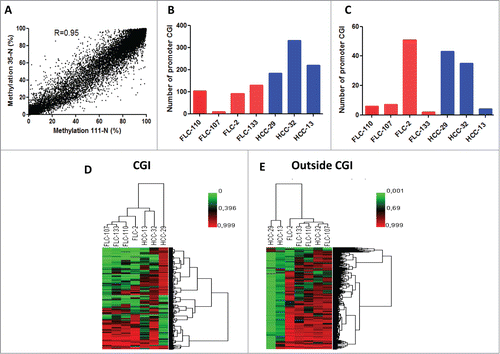
Because hypermethylation of promoters located in CG islands (CGI) is a common feature of cancer that likely leads to suppression of TSG, we thus sought to identify a list of promoters located in CGI which gain DNA methylation in p-FLC as compared to normal. Methylation gain was defined as genes with increase of methylation ≥15% in cancer as compared to less than 5% in normal livers. Using those criteria, we identified 252 genes out of 4503 promoter CGI which gained DNA methylation in p-FLC (Table S4). The number of genes varied between 10 to 130 genes per sample (). Of note, 50 genes gained DNA methylation in 3 out of the 4 p-FLC (Fig. S2). Out of those, we validated the 2 candidate genes DLEU7 and ZNF709 by pyrosequencing (PSQ). Interestingly, DLEU7 has been previously shown to be a TSG in leukemia.Citation20 Gene Ontology (GO) using DAVID pathway analysisCitation21 revealed that genes gaining DNA methylation are enriched for embryonic morphogenesis (P = 8.27E-12), regulation of RNA metabolic process (P = 1.22E-08) and embryonic organ morphogenesis (P = 4.50E-08) (Table S5). We then decided to investigate the genes that gain methylation and get repressed (FC≤1.5 as compared to normal), using our previously reported transcriptomic data of the same dataset.Citation6 Overall, 8 candidate genes met those criteria (ADRA1A, CYP26A1, ITGA4, ZSCAN18, CHL1, OLFM1, GOLSYN and SSTR1); Interestingly, ADRA1A was the unique gene which was found to be methylated in 2 out of the 4 p-FLCs.
Furthermore, we matched this 252-genes list with the list of TSG extracted from TSGene database, a websource for all known TSG.Citation22 As a result, we identified 14 genes as putative TSG in p-FLC; out of those, SOX11 methylation occurred in 3 samples, and ZIC1, IRX1 and GAS1 occurred in 2 samples.
On the other hand, 538 promoters gained DNA methylation in nc-HCC and the number of genes varied between 184–331 genes per sample ().
Identification of putative oncogenes
While the potential role of DNA methylation is established for silencing TSG, and demethylation for activation of oncogenes, we sought to analyze genes that lost DNA methylation in p-FLC. Overall, 56 promoters lost DNA methylation in p-FLC as compared to 47 genes in nc-HCC (). Seven genes out of those lost DNA methylation in at least 2 samples (CACNA1D, ZNF710, FAM163, MAP4K2, BDH1, IGF2 and DUSP4) (Fig. S3) (Table S6). Furthermore, 5 genes gained gene expression concomitantly (CACNA1D, DUSP4, ASPHD1, PLP2 and GPC4); interestingly, the expression of CACNA1D and DUSP4 was increased in 2 out of 4 p-FLC concomitantly with their demethylation (Fig. S4). IGF2 and DUSP4 methylation were validated by PSQ.
Integration of DNA methylation and histone modifications
Regarding the interplay between DNA methylation and histone modifications, we thus decided to match the list of genes gaining DNA methylation with histone modification marks obtained from embryonic stem cells (ES). Strikingly, the majority of them (162/252; 64.3%) were bivalent genes, 179 genes (71.03%) were marked by H3K27me3 and 67 (26.6%) marked by H3K4me3 (Fig. S5A).
Conversely, when we matched the list of genes that lost DNA methylation, 14 (25%) genes were marked by H3K27me3, 38 (67.9%) genes were marked by H3K4me3 and 13 (23.2%) were bivalent (Fig. S5B).
Unsupervised clustering of CG sites in CG islands distinguishes p-FLC from nc-HCC
We then asked if p-FLCs display a unique methylome as compared to classical HCC. To answer this question, we performed unsupervised hierarchical clustering using the 10% most variant probes of CG sites in islands (CGI). Clustering analysis revealed that p-FLC harbor a unique methylation signature as compared to HCC (). This was not the case when unsupervised clustering was performed using CG sites located outside CGI (). Indeed, this later analysis showed 2 clusters. The first comprises the majority of p-FLC and includes the HCC-32 case, while the second cluster contains 2 severely hypomethylated HCC (m-FLC-13 and HCC-29). Of note, those 2 cases displayed beside patterns of dramatic DNA hypomethylation in CG sites outside CGI, a concomitant gain of DNA methylation in CG sites located in CGI (Fig. S6A–B). These data are in contrast with the 4 p-FLC and HCC-29 cases showing dismal DNA demethylation outside CGI, except for p-FLC-2 (Fig. S6C–F).
To further understand the difference of global DNA methylation between the 2 clusters obtained using CG sites outside CGI, we analyzed methylation level of repetitive elements and found statistically different methylation of all repetitive elements including SINE-1, LINE-1, centromeric satellites and LTR between the 2 groups (Fig. S7A). We validated these data by pyrosequencing for LINE-1 methylation (Fig. S7B). We thus conclude that DNA methylation of CG sites in CGI allows better to distinguish p-FLC from other HCC, rather than CG sites outside CGI.
Partially methylated domains are the most prone regions for DNA methylation changes
As we observed gain of DNA methylation predominantly in CGI, in association with global hypomethylation outside CGI, we thus investigated whether any particular genomic features may correlate with DNA methylation changes during FLC carcinogenesis. To do so, we firstly focused on CG sites which gained DNA methylation in cancer. An arbitrary cut-off of 20% methylation difference was chosen, because of the excellent FDR of our method using this threshold.Citation17 Interestingly, although gain of DNA methylation was higher in CG sites located in CGI as compared to those located outside CGI, CG sites with some level of methylation in normal (≥20 %) were more likely to gain methylation in cancer (). Conversely, loss of DNA methylation did not differ whether CG sites where highly methylated (≥70 %) or partially methylated (<70 %) ().
Figure 3. (A) Box-plots of percentage of CG sites covered by DREAM method and gaining DNA methylation, whether located in promoters or outside promoters according to their methylation levels in normal livers. Green is related to CG sites with methylation level < 20% in normal liver. Red is related to CG sites with methylation level ≥ 20% in normal liver. (B) Box-plots of percentage of CG sites covered by DREAM method and losing DNA methylation, whether located in promoters or outside promoters according to their methylation levels in normal livers. Green is related to CG sites with methylation level < 70% in normal liver. Red is related to CG sites with methylation level ≥ 70 % in normal liver. (C) Box-plots of percentage of CG sites gaining DNA methylation, according to different genomic regions. (D) Box-plots of percentage of CG sites losing DNA methylation, according to different genomic regions. Abbreviations: PMD: partially methylated domains; LAD: lamina associated domains. For statistical analysis: ns: non significant; *P-value < 0.05; **P-value < 0.001; ***P-value < 0.0001.
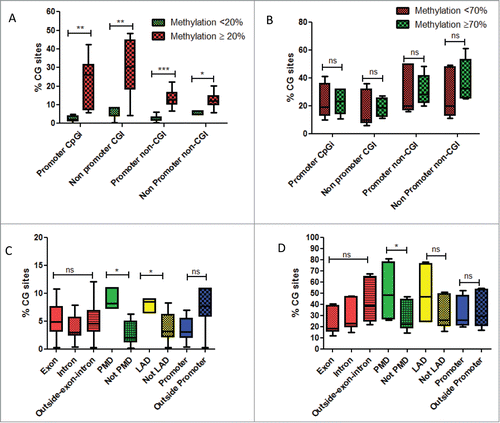
We then asked whether there are some specific CG sites located in CGI that are more likely to gain DNA methylation as compared to normal. PMD and Lamina-Associated Domains (LAD) stand out as more prone to DNA methylation gains (). It is interesting here to mention that we did not observe DNA methylation changes in CG sites whether located in promoters, exon, intron or outside gene bodies (). Likewise, we observed a global hypomethylation of CG sites located within PMDs (). We conclude that PMD regions are more prone to DNA methylation changes. Interestingly, genes located within PMDs are lowly expressed in normal livers even though some of them were unmethylated (Fig. S8A–B).
Identification of DNA methylation signature specific for pure-FLC
We then decided to establish an epigenetic signature of p-FLC as compared to other HCC and normal liver. For that, we selected CG sites which are differentially methylated between p-FLC from one side and normal liver and nc-HCC from the other side. Using stringent criteria (P < 0.05, and methylation difference ≥15 % or ≤−15%), we identified 1.26% (360/28549) CG sites with a minimum coverage of 10 tags. Out of those, only 32 CG sites mapped to promoter CGI including 29 genes as ZNF703, TPM4, IGF2, MAP4K2, DUSP4 and ARID5A (Table S7). Thus, the majority of DNA methylation changes occurred outside promoter CGI, mainly in regions distal to transcription start sites as gene bodies (n = 154) or outside genes (n = 112).
Using more stringiest criteria for promoter definition (either in CGI or outside) (± 1000 bp from TSS), there were only 0.32% (38/11574) differentially methylated CG sites in promoters as compared to ∼1.9% (322/16973) of CG sites located outside promoters. As the majority of DNA methylation changes occurred outside promoters, we used GREAT, a software that assigns biological meaning to a set of non-coding genomic regions using the annotations of the nearby genes.Citation23 GREAT analysis revealed that differentially methylated CG sites are located in genomic regions enriched for genes involved in liver (P = 4.44E-7) and hepatobiliary development (P = 4.44E-7) (Table S8). Enrichment for liver development was related to the following 12 genes: ASS1, BDH1, CCND1, CEBPA, CEBPG, GATA6, HES1, NOTCH1, RXRA, SMARCA4, SOX9 and VWF.
We then asked whether there are gene expression differences of those liver developmental genes between p-FLC and normal liver. Using our previous micro-arrays data, we identified that the 2 genes RXRA and SMARCA4 were differentially expressed between p-FLC and normal livers (). Using RT-PCR, we validate overexpression of SMARCA4 (P = 0.003) in p-FLC; however, the decrease of RXRA expression in p-FLC was at the limit of significance (P = 0.05) (not shown). Importantly, those genes belonged to a group of 13 upstream regulators identified by Ingenuity Pathway Analysis (IPA) as governing differentially expressed genes between p-FLC and normal livers () (Table S9).
Figure 4. (A–B) Box-plots for expression levels of SMARCA4 and RXRA in pure fibrolamellar carcinoma and normal adjacent livers using our previously published microarray data related to 17 p-FLC and 10 normal livers cases. (C–D) Network of genes coordinated by SMARCA4 and RXRA according to Ingenuity Pathway Analysis. Red: upregulated; green: down-regulated.
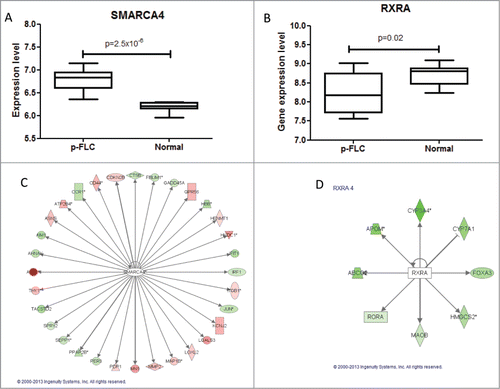
Establishment and independent validation of p-FLC signature
We selected 4 of the top differentially methylated CG sites (ATP2B4, ZNF703 and TPM4) differentiating p-FLC from HCC and normal liver and validated them by pyrosequencing. We found extremely high correlation of pyrosequencing with DREAM results (Spearman R = 0.92, P < 0.0001) (not shown). We then assessed DNA methylation of those differentially methylated regions in an independent data set comprising 23 p-FLC, 8 nc-HCC and 5 m-FLC. Hierarchical unsupervised clustering reveals 2 epi-clusters, the first containing all but one p-FLC (C1) and the other containing m-FLC and nc-HCC (C2) (). Of note, the 3 p-FLC classified as p-FLC by 2 pathologists and found as outlier in our previous transcriptomic classification were located within C2 epi-cluster suggesting that they have been misclassified. Only, one p-FLC case was found within C2 cluster but no RNA was available in this case to check for presence of fusion transcript.
Figure 5. Unsupervised clustering for DNA methylation using 4 sets of differentially methylated genes. Note that all but one pure fibrolamellar hepatocellular carcinoma (FLC) belong to cluster C1, while mixed fibrolamellar carcinoma and hepatocellular carcinoma in non-cirrhotic liver belong to cluster C2. For the only pure FLC which clustered within C2 cases, no RNA was available to check for the presence of fusion transcript.
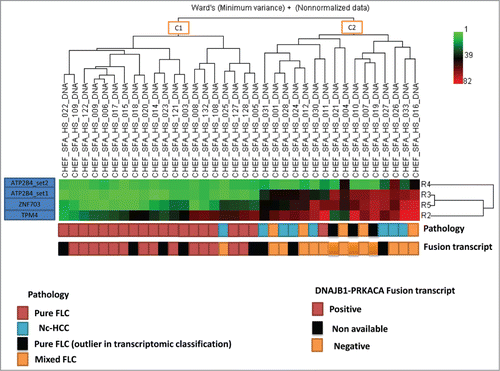
Validation of identified putative TSG and oncogenes
We then sought to examine the methylation levels of selected genes gaining DNA methylation in the training set, in a validation set encompassing p-FLC, m-FLC and nc-HCC. We thus selected 2 genes that lost promoter DNA methylation in 2 p-FLC cases and become expressed (IGF2 and DUSP4) (not shown). Furthermore, the 2 additional genes DLEU7 and ZNF709 were chosen because they were ranked among the top genes associated with gain of promoter DNA methylation levels. Of note, DLEU7 has been previously reported to play a role as a tumor suppressor gene in leukemia and thus we wanted to investigate its methylation level herein.Citation20 Methylated genes were defined as those with methylation level more than 20% in cancer, as compared to less than 5% methylation level in normal livers. Overall, 11/20 p-FLC gain methylation of DLEU7 and 16/20 p-FLC showed gain methylation of ZNF709 (). Finally, 18 and 14 out of 20 p-FLC lost IGF2 and DUSP4 methylation, respectively ().
Figure 6. (A) Distribution of DLEU7 methylation levels in the validation set of pure fibrolamellar carcinoma (p-FLC), mixed FLC (m-FLC), non-cirrhotic hepatocellular carcinoma (nc-HCC) and normal adjacent liver. Each dot represents the methylation level of individual samples. Horizontal lines represent the mean methylation levels for each group. (B) Distribution of ZNF709 methylation levels in p-FLC, m-FLC, nc-HCC and normal adjacent liver. (C) Distribution of DUSP4 methylation levels in p-FLC, m-FLC, nc-HCC and normal adjacent liver. (D) Distribution of IGF2 methylation levels in p-FLC, m-FLC, nc-HCC and normal adjacent liver.
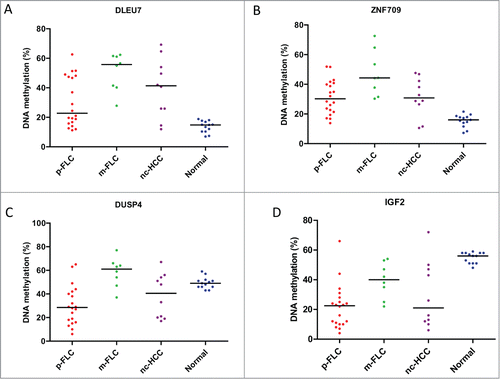
Discussion
To our knowledge, this study represents the first application of next-generation sequencing to deciphering genome-wide DNA methylation alterations in FLC. Our analysis unravels the epigenetic signature of p-FLC as compared to classical HCC and showed that FLC methylation changes primarily occur within partially methylated domains. Furthermore, we discovered a unique DNA methylation signature of pFLC which can help distinguishing p-FLC from other HCC with high sensitivity and specificity.
In contrast to previous studies performed in p-FLCS, which are locus-specific studies,Citation12,13 our study identified in an unbiased way the landscape of frequently methylated genes in p-FLC. Those genes were in majority involved in embryonic morphogenesis and regulation of RNA metabolic process. Consistent with previous reports, genes that gained DNA methylation in p-FLC belong to a “DNA hypermethylation module”.Citation24 Indeed, this module corresponds to almost 75% of hypermethylated genes marked by the polycomb in the context of bivalent chromatin in both embryonic stem cells and adult stem/progenitor cells.Citation24 Interestingly, we also found that genes losing DNA methylation were, in contrast, enriched for H3K4me3 in embryonic stem cells, highly suggesting that cancer cells may recapitulate the gene expression of selected genes in embryonic development. This may provide treatment options for patients with p-FLC, through targeting the Polycomb repressive complex.
One of the largest series of DNA methylation analysis reported by Tränkenschuh et al. on 15 FLC cases revealed distinct gene-specific hypermethylation in FLC and absence of significant global hypomethylation.Citation12 Conversely, Vivekanandan et al. showed that FLC displays low levels of methylation as compared to HCC that arose in the background of viral cirrhosis.Citation13 Herein, consistent with Tränkenschuh et al. report, we found a DNA methylation signature unique to p-FLC as compared to other HCC subtypes. In contrast, we observed that almost one third of p-FLC displayed global hypomethylation consistent with their clinical aggressiveness, challenging the notion that this disease is homogeneous at the epigenetic level.
The quantitative nature of the results obtained through deep resolution allows us to establish and validate a DNA methylation signature which may be used in addition to the DNAJB1-PRKACA oncogenic fusion transcript in better classifying p-FLC. Of note, 3 of pFLCs which we reported as outliers in our previous transcriptomic classification of p-FLC turned out to not harbor the oncogenic fusion transcript and were thus misclassified by 2 pathologists. Interestingly, our DNA methylation signature was consistent for those cases as they turned out to cluster within the C2 cluster, which does not contain p-FLCs. We thus conclude, in accordance with Graham et al., that DNAJB1-PRKACA is really specific for the diagnosis of p-FLC.Citation9 This may clarify the data recently reported by Cornella et al., where 21% of FLC did not show oncogenic fusion transcript.
In our pFLC signature, 4 markers related to 3 genes (ATP2B4, ZNF703 and TPM4) were identified in the training dataset. In the independent data set, those markers turned out to have a high sensitivity and specificity in the diagnosis of pFLC. When we focused on the cases positive for the presence of the oncogenic fusion transcript, our signature displayed 100% specificity in diagnosing p-FLC. Thus, DNA methylation may be used in routine in diagnosing p-FLC if validated by others.
Another topic that deserves to be discussed is the fact that the majority of DNA methylation changes observed between pFLC from one side and classical HCC and normal livers from the other sides occurred outside gene promoters. Furthermore, those were related to regions located in the vicinity of liver development genes (i.e., SMARCA4, RXRA). This is consistent with the recent methylome data across many human tissue types showing a dynamic DNA methylation regulation for only 21.8% of autosomal CG sites, most of which are distal to transcription start sites.Citation10 We thus speculate that those differentially methylated regions may co-localize with liver regulatory elements, such as enhancers which allow shaping of distinct lineage-specific regulation.
Our study has several limitations. First, our next-generation sequencing involved a handful of cases, although it is the first of its kind in the literature. Second, we identified several genes regulated by DNA methylation; however, functional validation is lacking mainly due to the absence of cell lines available. Despite its limitation, our study represents the first unbiased analysis of the landscape of DNA methylation in pFLC as compared to nc-HCC. This allowed us to identify putative novel tumor suppressor genes and oncogenes that may be involved in FLC carcinogenesis or progression. Functional analyses are furthermore needed in the future to establish the role of those genes in cancer initiation, invasion and proliferation. Finally, we established and validated a DNA methylation signature which may be used in distinguishing pure from mixed FLC, in addition to the oncogenic fusion transcript.
Disclosure of Potential Conflicts of Interest
No potential conflict of interest was disclosed.
Author Contributions
Study concept and design, interpretation, manuscript writing: GG Malouf, V Paradis, T Tahara, J Yamazaki, R Mouawad, D Khayat, H Long, Y Lu, S Liang, C Guettier, M Fabre, N Raynal, J Jelinek, E Raymond, Issa JP. Data Analysis: GG Malouf, T Tahara, J Yamazaki, H Long, R Mouawad, Y Lu, S Liang, N Raynal, J Jelinek. Obtained Funding: E Raymond, JP Issa. Acquisition of Data: GG Malouf, V Paradis, M Fabre, C Guettier T Tahara, J Yamazaki, H Long, Y Lu, S Liang, N Raynal, R Mouawad, J Jelinek. Provision of Study Materials or Patients: V Paradis, M Fabre, L Brugières, E Raymond. Final Approval of the Manuscript: All authors.
1076955_Supplemental_Material.zip
Download Zip (7.4 MB)Supplemental Material
Supplemental data for this article can be accessed on the publisher's website.
Funding
This study has been supported in part by Cartes d'Identité des Tumeurs (CIT) program from the Ligue Nationale Contre le Cancer, Foundation Nelia et Amadeo Barletta (F.N.A.B.), Association pour la Recherche et l'Enseignement en Cancérologie (A.A.R.E.C.) and AVEC foundation.
References
- Torbenson M. Fibrolamellar Carcinoma: 2012 Update. Scientifica 2012; 2012:743790; PMID:24278737
- Edmondson HA. Differential diagnosis of tumors and tumor-like lesions of the liver in infancy and childhood. AMA J Dis Child 1956; 91(2):168-86
- Malouf G, Falissard B, Azoulay D, Callea F, Ferrell LD, Goodman ZD, Hayashi Y, Hsu HC, Hubscher SG, Kojiro M, et al. Is histological diagnosis of primary liver carcinomas with fibrous stroma reproducible among experts? J Clin Pathol 2009; 62:519-524; PMID:19155239; http://dx.doi.org/10.1136/jcp.2008.062620
- Malouf GG, Brugieres L, Le Deley MC, Faivre S, Fabre M, Paradis V, Aerts I, Le Tourneau C, Dreyer C, Branchereau S, et al. Pure and mixed fibrolamellar hepatocellular carcinomas differ in natural history and prognosis after complete surgical resection. Cancer 2012; 118:4981-90; PMID:22415897; http://dx.doi.org/10.1002/cncr.27520
- Cornella H, Alsinet C, Sayols S, Zhang Z, Hao K, Cabellos L, Hoshida Y, Villanueva A, Thung S, Ward SC, et al. Unique genomic profile of fibrolamellar hepatocellular carcinoma. 2013 2015, 148:806-818 e810; PMID:25557953
- Malouf GG, Job S, Paradis V, Fabre M, Brugieres L, Saintigny P, Vescovo L, Belghiti J, Branchereau S, Faivre S, et al. Transcriptional profiling of pure fibrolamellar hepatocellular carcinoma reveals an endocrine signature. Hepatology 2014; 59:2228-37; PMID:24443104; http://dx.doi.org/10.1002/hep.27018
- Xu L, Hazard FK, Zmoos AF, Jahchan N, Chaib H, Garfin PM, Rangaswami A, Snyder MP, Sage J. Genomic analysis of fibrolamellar hepatocellular carcinoma. Hum Mol Genet 2014, 24:50-63; PMID:25122662; http://dx.doi.org/10.1093/hmg/ddu418
- Honeyman JN, Simon EP, Robine N, Chiaroni-Clarke R, Darcy DG, Lim, II, Gleason CE, Murphy JM, Rosenberg BR, Teegan L, et al. Detection of a recurrent DNAJB1-PRKACA chimeric transcript in fibrolamellar hepatocellular carcinoma. Science 2014; 343(6174):1010-4; PMID:24578576
- Graham RP, Jin L, Knutson DL, Kloft-Nelson SM, Greipp PT, Waldburger N, Roessler S, Longerich T, Roberts LR, Oliveira AM, et al. DNAJB1-PRKACA is specific for fibrolamellar carcinoma. Mod Pathol 2015; 28(6):822-9; PMID:25698061
- Ziller MJ, Gu H, Muller F, Donaghey J, Tsai LT, Kohlbacher O, De Jager PL, Rosen ED, Bennett DA, Bernstein BE, et al. Charting a dynamic DNA methylation landscape of the human genome. Nature 2013, 500:477-481; PMID:23925113; http://dx.doi.org/10.1038/nature12433
- Sirivatanauksorn Y, Sirivatanauksorn V, Lemoine NR, Williamson RC, Davidson BR. Genomic homogeneity in fibrolamellar carcinomas. Gut 2001, 49:82-86; PMID:11413114; http://dx.doi.org/10.1136/gut.49.1.82
- Trankenschuh W, Puls F, Christgen M, Albat C, Heim A, Poczkaj J, Fleming P, Kreipe H, Lehmann U. Frequent and distinct aberrations of DNA methylation patterns in fibrolamellar carcinoma of the liver. PLoS One 2010, 5:e13688; PMID:21060828; http://dx.doi.org/10.1371/journal.pone.0013688
- Vivekanandan P, Torbenson M. Epigenetic instability is rare in fibrolamellar carcinomas but common in viral-associated hepatocellular carcinomas. Mod Pathol 2008, 21:670-675; PMID:18264082; http://dx.doi.org/10.1038/modpathol.2008.32
- Lister R, Pelizzola M, Dowen RH, Hawkins RD, Hon G, Tonti-Filippini J, Nery JR, Lee L, Ye Z, Ngo QM, et al. Human DNA methylomes at base resolution show widespread epigenomic differences. Nature 2009, 462:315-22; PMID:19829295; http://dx.doi.org/10.1038/nature08514
- Hansen KD, Timp W, Bravo HC, Sabunciyan S, Langmead B, McDonald OG, Wen B, Wu H, Liu Y, Diep D, et al. Increased methylation variation in epigenetic domains across cancer types. Nat Genet 2011, 43:768-75; PMID:21706001; http://dx.doi.org/10.1038/ng.865
- Malouf GG, Brugières L, Ledeley MC, Faivre S, Fabre M, Paradis V, Aerts I, Le Tourneau C, Dreyer C, Branchereau S, et al. Pure and Mixed Fibrolamellar Hepatocellular Carcinomas Differ in Natural History and Prognosis after Complete Surgical Resection. Cancer 2012; 118(20):4981-90.
- Jelinek J, Liang S, Lu Y, He R, Ramagli LS, Shpall EJ, Estecio MR, Issa JP. Conserved DNA methylation patterns in healthy blood cells and extensive changes in leukemia measured by a new quantitative technique. Epigenetics 2012, 7:1368-78; PMID:23075513; http://dx.doi.org/10.4161/epi.22552
- Figueiredo JC, Grau MV, Wallace K, Levine AJ, Shen L, Hamdan R, Chen X, Bresalier RS, McKeown-Eyssen G, Haile RW, et al. Global DNA hypomethylation (LINE-1) in the normal colon and lifestyle characteristics and dietary and genetic factors. Cancer Epidemiol Biomarkers Prev 2009, 18:1041-9; PMID:19336559; http://dx.doi.org/10.1158/1055-9965.EPI-08-0926
- Colella S, Shen L, Baggerly KA, Issa JP, Krahe R. Sensitive and quantitative universal Pyrosequencing methylation analysis of CpG sites. Biotechniques 2003, 35:146-50; PMID:12866414
- Hammarsund M, Corcoran MM, Wilson W, Zhu C, Einhorn S, Sangfelt O, Grander D. Characterization of a novel B-CLL candidate gene-DLEU7-located in the 13q14 tumor suppressor locus. FEBS Lett 2004, 556:75-80; PMID:14706829; http://dx.doi.org/10.1016/S0014-5793(03)01371-1
- Huang da W, Sherman BT, Lempicki RA. Systematic and integrative analysis of large gene lists using DAVID bioinformatics resources. Nat Protoc 2009; 4(1):44-57; PMID:19131956.
- Zhao M, Sun J, Zhao Z. TSGene: a web resource for tumor suppressor genes. Nucleic Acids Res 2013, 41:D970-976; PMID:23066107; http://dx.doi.org/10.1093/nar/gks937
- McLean CY, Bristor D, Hiller M, Clarke SL, Schaar BT, Lowe CB, Wenger AM, Bejerano G. GREAT improves functional interpretation of cis-regulatory regions. Nat Biotechnol 2010, 28:495-501; PMID:20436461; http://dx.doi.org/10.1038/nbt.1630
- Easwaran H, Johnstone SE, Van Neste L, Ohm J, Mosbruger T, Wang Q, Aryee MJ, Joyce P, Ahuja N, Weisenberger D, et al. A DNA hypermethylation module for the stem/progenitor cell signature of cancer. Genome Res 2012, 22:837-49; PMID:22391556; http://dx.doi.org/10.1101/gr.131169.111