ABSTRACT
Differential intragenic methylation in social insects has been hailed as a prime mover of environmentally driven organismal plasticity and even as evidence for genomic imprinting. However, very little experimental work has been done to test these ideas and to prove the validity of such claims. Here we analyze in detail differentially methylated obligatory epialleles of a conserved gene encoding lysosomal α-mannosidase (AmLAM) in the honeybee. We combined genotyping of progenies derived from colonies founded by single drone inseminated queens, ultra-deep allele-specific bisulfite DNA sequencing, and gene expression to reveal how sequence variants, DNA methylation, and transcription interrelate. We show that both methylated and non-methylated states of AmLAM follow Mendelian inheritance patterns and are strongly influenced by polymorphic changes in DNA. Increased methylation of a given allele correlates with higher levels of context-dependent AmLAM expression and appears to affect the transcription of an antisense long noncoding RNA. No evidence of allelic imbalance or imprinting involved in this process has been found. Our data suggest that by generating alternate methylation states that affect gene expression, sequence variants provide organisms with a high level of epigenetic flexibility that can be used to select appropriate responses in various contexts. This study represents the first effort to integrate DNA sequence variants, gene expression, and methylation in a social insect to advance our understanding of their relationships in the context of causality.
Introduction
The transcriptional events that lead to the development of a multicellular organism result from interactions between the underlying genetic sequence and an array of epigenomic marks that are modulated, in part, by environmental signals. Central to these processes are a variety of epigenetic pathways contributing to phenotypic plasticity and environmental adaptation.Citation1-3 One of the key challenges in understanding what drives phenotypic variation lies in determining the extent to which the nucleotide sequence influences epigenetic states. Instances where cis- or trans-acting genetic polymorphisms influence an epigenetic state, known as an ‘obligatory epiallele’, can have clear transcriptional effects.Citation4 Recent work has shown that genetic variation is associated with altered chromatin states Citation5,6 and differential DNA methylation, Citation7 which appear to modify gene expression primarily through altered transcription factor binding.Citation7,8 This occurs in a highly context-dependent manner. Consequently, defining the exact mechanisms whereby a given epiallele can influence transcription and, subsequently, phenotype remains a challenge.
Obligatory methylated epialleles are known to play an important role in driving phenotype across a number of eukaryotes. In contrast to pure epialleles that are stochastically methylated, differential methylation at obligatory epialleles is driven by changes in the DNA sequence.Citation4,9,10 In mammals these are best known to occur in cases of allele-specific methylation (ASM) where, primarily, cis-acting sequences influence methylation at a locus.Citation11-14 This phenomenon is distinct from genomic imprinting, where differential DNA methylation occurs not as a function of the underlying sequence, but depending on whether the locus is paternally or maternally derived.Citation15,16 In both cases, such ASM can have important phenotypic consequences, with differential DNA methylation controlling gene expression directly, and being implicated in pathologies such as cancer.Citation17,18
In the invertebrate Apis mellifera, differential DNA methylation has been implicated in modulating both phenotypic and behavioral plasticity.Citation19-23 Evidence for such roles is largely correlative with little mechanistic understanding of how DNA methylation can influence active transcription and alternative splicing. Consequently, it is not clear what determines whether methylation is causative of gene expression changes versus merely being an indicator of transcriptional status, and through which pathways this process is being coordinated.
While parent-of-origin effects have been hinted for a number of traits in this organism,Citation24-27 there is currently no evidence that differentially methylated regions (DMRs) are associated with genomic imprinting. Given the extensive differential methylation that occurs in A. mellifera, and its association with complex traits, we wanted to investigate whether DMRs are associated with the underlying genetic sequence, the paternal or maternal inheritance of an allele, or whether they are primarily influenced by environmental cues.
To achieve this aim we performed a genome-wide analysis of several sequenced methylomes and identified a DMR encoding lysosomal α-mannosidase (AmLAM) that appeared to correlate with DNA sequence variants. To confirm the existence of AmLAM epialleles, we established several colonies with single drone inseminated queens (henceforth referred to as SDI hives) and performed ultra-deep next-generation bisulfite sequencing (BS-seq) of AmLAM amplicons to determine DNA methylation levels across individuals within each SDI hive. We uncovered 2 distinct patterns of methylation for AmLAM: a highly methylated state linked to a single allele, LAMC+CT; and a non-methylated state, linked to 3 identified alleles, LAMC+GA, LAMCΔGA, and LAMT+GT. These methylation states follow Mendelian inheritance patterns, confirming that a number of obligatory LAM epialleles exist within the honeybee population. Further analyses showed that this differential gene body methylation alters AmLAM expression in a context-dependent manner, and leads to the expression of an uncharacterized antisense long noncoding RNA (lncRNA).
Results
A differentially methylated region in the gene encoding lysosomal α-mannosidase
In the process of comparing genome-wide methylation profiles in the honeybee haploid and diploid embryos we have come across a ∼0.8 kb region, located on Linkage Group 4 (genome assembly 4.5), containing 20 CpGs that are highly methylated (median level ∼65%) only in the diploid sample. We identified this region as exons 16 to 18 of the gene coding for lysosomal α-mannosidase (AmLAM GB44223, ), and noticed that 2 sequence variants correlated with a particular methylation pattern (Fig. S1). One allele, identified by a 12 bp deletion in exon 16 was found to be non-methylated, while a second allele, identified by 2 SNPs separated by a single cytosine residue in intron 17, was always found to be highly methylated. This finding prompted us to perform an in-depth characterization of AmLAM alleles, and investigate whether differentially methylated LAM epialleles exist in the honey bee population and if they are imprinted in a parent-of-origin manner.
Figure 1. Manually annotated AmLAM gene model showing all exons in 2 mutually exclusive transcript variants. The alternatively spliced and methylated exons are shown against orange and gray backdrops respectively. The four polymorphisms used for genotyping are shown in an insert (Y= C or T, K= G or T, W=A or T, DEL=12 bp deletion). The region analyzed by bisulphite sequencing is indicated by the gray line in the insert, with individual CpG sites represented by vertical lines. Those CpG sites affected by sequence variations are shown in red.
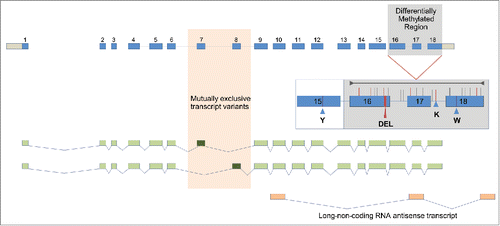
Identification of multiple allelic variants of AmLAM
AmLAM encodes an ancient and highly conserved protein (Fig. S2) that has been implicated in essential metabolic functions in eukaryotes, namely in the maturation and degradation of glycoprotein-linked oligosaccharides.Citation28,29 Its critical role is underscored by the symptoms of a debilitating disease affecting many organs and tissues in mammals including humans that is caused by mutations in the LAM gene.Citation30 In honeybees the LAM locus is 7,264 bp long and encodes 18 exons that are alternatively spliced yielding 2 mutually exclusive transcript variants with either exon 7 or 8 being transcribed (). This splicing pattern was conserved in Drosophila.Citation23,31,32 At present it is not clear if the 2, only marginally different protein isoforms, have distinct catalytic activities, but they appear to be always co-expressed. AmLAM is ubiquitous, but shows markedly variable levels throughout development and in various tissues. Not surprisingly, AmLAM is highly expressed in the larval digestive system, where there is approximately 150 times greater expression than in larval fat body or head (Fig. S3). Moderate to high levels of expression have also been detected in other tissues and organs, including adult brains, ovaries, and testes (Fig. S3).
Figure 2. Methylation levels of the region spanning exon 16 and exon 18 of the αLAM gene. To follow the inheritance of methylation across a generation a single male drone was mated with a queen; haploid drones develop from unfertilized eggs laid by the queen, and diploid workers/queens develop from fertilized eggs. BS-sequencing was performed on individuals collected from 3 hives (#1-3). (A). Methylation density of the 4 AmLAM alleles analyzed: LAMC+CT, LAMC+GA, LAMCΔGA, and LAMT+GT across 2 amplicons, ex1617 and ex1718. It should be noted that no sequence variation was present in the ex1617 amplicon that allowed for the discrimination between the LAMC+CT and LAMT+GT alleles, and as such individuals from hive #2 were excluded from the methylation density analysis for ex1617. (B). Schematic of the most frequent patterns of methylation linked to the LAMC+CT and LAMCΔGA alleles found in hive #1. (C). Pedigree of hive #1 showing the percentage frequency that one of the 25 CpG sites in the analyzed region was found to be methylated, 0% indicating that the CpG was never found to be methylated, 100% indicating that the CpG was always found to be methylated. BS-sequencing was performed on the founding drone and queen, and their progeny at multiple stages of development (48 h larva, 96 h larva, and pupal stages). To account for potential tissue-specific methylation effects, 2 tissue types were analyzed in adult and pupal stages, the brain/head and thorax; larvae were processed whole. In cases in which 2 tissue types were analyzed, brain/head results are indicated in the top panel, thorax in the bottom panel. Note that in individuals of the LAMC+CT/ LAMC+CT genotype individual alleles could not be distinguished from one another, the percentage frequency result has been duplicated to indicate ploidy.
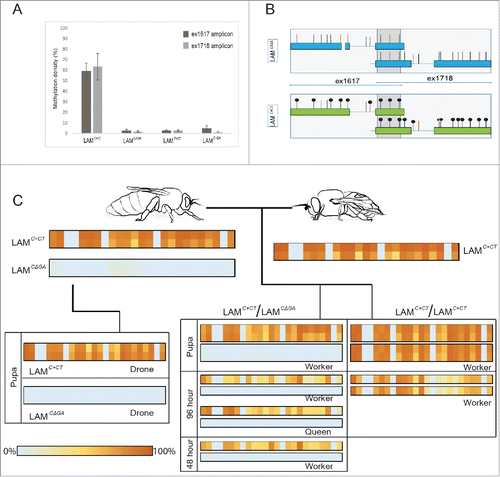
To identify allelic variants of AmLAM, we limited genetic variation in the analyzed population and utilized honeybee colonies founded by single drone inseminated queens (SDI hives). By a combination of PCR-based genotyping and direct ultra-deep amplicon sequencing, we identified 6 allelic variants of LAM in the honeybee population. Across the analyzed region, 16 sequence variations were identified (Table S1); these include 3 missense mutations and 6 sequence variations that result in either the introduction or removal of a CpG motif. The 12-bp in-frame deletion in exon 16 was only identified in one allele, the LAMCΔGA allele, and is predicted to result in the truncation of the LAM D-peptide (; Fig. S2). Of the 6 alleles, only one, the LAMC+CT allele, contains the 2 SNPs found to be linked to a high level of methylation. We selected SDI hives that contained these 2 alleles, along with 2 others, LAMC+GA and LAMT+GT, to further analyze the allele-methylation relationship (Fig. S4).
Next generation BS-sequencing of amplicons identifies novel obligatory AmLAM epialleles
We have employed ultra-deep bisulfite sequencing of amplicons using Illumina MiSeq. In contrast to low-depth (<50x) genome-wide methylation profiles that are assembled from short <100-bp reads representing combined methylomes of many different cell types, the MiSeq approach allows for analyzing longer sequence lengths of 600 bp and at a depth of several hundred thousand reads per amplicon. The resolving power of this approach is sufficient to visualize all condition-specific methylation patterns that may be associated with dozens of distinct cell types, even if methylation levels in certain cell types are very low.Citation33
To determine the level of methylation of each allele, we performed sequencing of 2 LAM amplicons, ex1617 (spanning exons 16 to 17) and ex1718 (spanning exons 17 to 18), which yielded an average coverage of 106,611 and 95,985 reads, respectively. We determined the methylation density across both amplicons for individuals sampled from 3 SDI hives (called 1, 2, and 3) that contained different combinations of the LAMC+GA, LAMCΔGA, LAMT+GT, and LAMC+CT alleles (Fig. S4); total methylation density was determined by averaging the methylation density across both amplicons. For each SDI hive the methylation density was determined for the founding queen and drone and their progeny at multiple stages of development; this allowed for a cross-generational analysis and for potential developmental or tissue specific effects on the methylation density to be identified.
We found that the LAMC+CT allele, identified in SDI hives 1 and 2, displayed a mean total methylation density of 61.4 ± 10.3% (, panel A) with no significant difference in the total methylation density of LAMC+CT found between the SDI hives [t(2)=4.30, P=0.60, by a 2-sample t-test]. This high level of methylation is in contrast with the LAMCΔGA, LAMC+GA, and LAMT+GT alleles, which lacked methylation entirely, or displayed very low levels of methylation. Analysis of the region revealed that the LAMCΔGA allele, present in SDI hives 1 and 3, was found to have a mean total methylation density of only 2.1 ± 1.2%; the LAMC+GA allele, present only in SDI hive 3, 2.9 ± 1.9%, and LAMT+GT, present in SDI hives 2 and 3, 2.8 ± 1.0%. No significant difference in the methylation levels were found between these 3 alleles [F(2,19)=1.07, P=0.36, by one-way ANOVA). In all cases, the majority of the reads displayed no methylation; for instance, 67.6-94.5% of all reads of the LAMCΔGA allele in SDI hive 1 lacked methylation completely, while the LAMC+CT allele within the same hive rarely displayed this lack of methylation; 12.9-52.7% of all reads exhibited methylation at 20 out of the 22 CpGs present in the region analyzed ( panel B).
Further analysis of the methylation patterns of the LAMC+CT alleles showed that although there was no significant difference in the total methylation density between SDI hives 1 and 2, there were, within the region covered by ex1617 (), major differences at CpGs 4 and 9: CpG 4 was unmethylated in the allele originating from SDI hive 1 founding drone and CpG 9 was unmethylated in the allele originating from SDI hive 2 founding queen. Sanger sequencing of both alleles did not reveal any additional, closely linked sequence variation that could explain this phenomenon.
Figure 3. Differential methylation of the LAMC+CT allele. (A). Methylation patterns for founding individuals from SDI hives #1 and 2. (B). All methylation patterns covered by Illumina Miseq; “Frequency” denotes the pattern sorting direction (i.e., most frequent patterns at top).
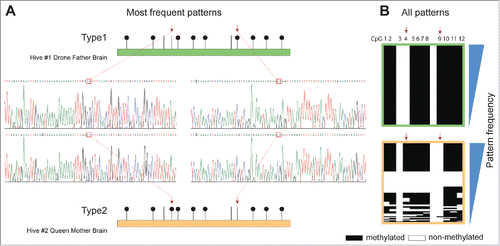
By performing in-depth, cross-generational analysis of SDI hive 1, we were able to test whether additional factors, such as developmental stage, tissue-type, or caste, influenced methylation of the AmLAM alleles. We analyzed the overall level of methylation at each of the 25 CpGs present in the sequenced region. The patterns of methylation linked to each allele followed Mendelian inheritance patterns and remained relatively stable between developmental stages and tissue type ( panel C).
To ascertain whether tissue-specific effects influenced the total methylation density of the LAM alleles, we conducted a 2-sample t-test for both alleles in SDI hive 1. No significant effect of tissue-type was found on the LAMCΔGA allele, t(4)=2.78, P=0.64. However, we found that there was a significantly higher total methylation density of the LAMC+CT allele, t(10)=2.23, P=0.004, in head/brain (M=70.6%) samples than in thorax (M=62.6%).
We tested age related effects on the methylation density by comparing SDI hive 1 adults, pupae, 96 h larvae, and a 48 h larval individual. A one-way ANOVA was conducted that showed a significant effect of age on the total methylation density of the LAMC+CT allele [F(3,13)=17.8, P<0.0001]. Contrasts revealed a significant difference between the pupal (M=65.2%) and 96 h (M=47.3%) stages of development, t(6)=2.50, P=0.001, as well as between adult (M=70.1%) and 96 h (47.3%) stages of development, t(7)=2.36, P<0.001. These age related effects were not found for the LAMCΔGA allele, with no significant effects of age on total methylation density found by one-way ANOVA [F(3,7)=2.1, P=0.19].
We conducted a one-way ANOVA to test whether caste influenced the total methylation density of either alleles present in SDI hive 1; no significant effect of caste was found on the LAMC+CT allele [F(2,14)=1.65, P=0.23], nor was there an effect on the LAMCΔGA allele [F(2,8)=1.34, P=0.32], indicating that caste does not affect the methylation density of the LAM alleles.
AmLAM epialleles influence expression in a context-dependent manner, and methylation is associated with the expression of a novel lncRNA
Next, we investigated whether the identified allele specific methylation was associated with changes in AmLAM expression across multiple developmental stages utilizing quantitative PCR. The DNA methylation status of each individual was inferred based on genotype, with 3 categories analyzed: diploid individuals without methylation, diploid individuals with one methylated allele, and diploid individuals with both alleles methylated.
Across SDI hives 1 and 2, no significant difference between these 3 categories was found in the abdomen of pupae [F(2,21)= 0.71, P=0.51, by one-way ANOVA], or in the thoraces of pupae [F(2,10)=0.63, P=0.55, by one-way ANOVA]. In 48 h whole larvae, however, LAM expression was found to increase with methylation; a one-way ANOVA showed a significant difference in relative expression [F(2,8)= 8.88, P=0.009], with contrasts revealing a significantly higher level of expression in individuals with both alleles methylated (M=9.9) when compared to individuals without methylation (M=6.3) [t(5)= 2.57, P=0.001, by 2-sample t-test](). Further analysis of individuals from SDI hive 1 showed that no significant difference in AmLAM expression was apparent between genotypes for either brain [t(2)= 4.30, P=0.79, by a 2-sample t-test] or stomach [t(3)= 3.18, P=0.29, by a 2-sample t-test].
Figure 4. Relative expression of AmLAM and an antiLAM lncRNA transcript at multiple stages of development. Transcript levels were measured via quantitative PCR and normalized against CAM and TBP transcript levels; normalized results from CAM and TBP were averaged. Expression of both transcripts in 48-h larvae, and pupal thorax and abdomen relative to a single pupal abdomen sample are shown. The level of significance is indicated by: *P <0.05; **P<0.01; ***P<0.001; **** P<0.0001. The methylation status of an allele is indicated by “–” (non-methylated) or “+” (methylated).
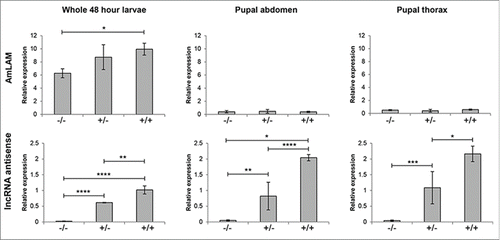
Given that ASM is frequently associated with transcriptional changes such as the allelic-specific or monoallelic expression typical of genomic imprinting, we went on to test the possibility of allelic imbalance through the quantitation of the LAM alleles. In SDI hive 1 we quantitated the LAMC+CT (methylated) and LAMCΔGA (non-methylated) alleles in heterozygous individuals and in SDI hive 3 we quantitated the LAMT+CT (non-methylated) and LAMCΔGA (non-methylated) alleles, also in heterozygous individuals. We tested pupal stages, and did not find a significant difference in expression between these alleles in SDI hive 1 [t(23)= 2.06, P=0.24, by 2-sample t-test] or in SDI hive 3 [t(4)=2.78, P=0.30, by 2-sample t-test], and in all cases the allelic ratio did not deviate significantly from 1:1 (with a 1:1 ratio representing equal expression of both alleles) (Fig. S5).
Interestingly, analysis of a long RNA-coding antisense transcript shows that there is a strong correlation between the expression of this transcript and methylation across multiple stages of development. We found a significant effect of methylation on the relative expression of this lncRNA transcript in whole 48 h larvae [F(2,8)=111.2, P<0.0001, by one-way ANOVA], in the abdomen of pupae [F(2,10)=17.4, P<0.001, by one-way ANOVA], and in the thoraces of pupae [F(2,10)=14.5, P=0.001, by one-way ANOVA]( ), but did not find a significance difference for either brain [t(3)=3.18, P=0.10, by 2-sample t-test] or stomach [t(4)=2.78, P=0.10, by 2-sample t-test].
Although there is no apparent correlation between distinct methylation states of the AmLAM locus and its splicing pattern (not shown), we cannot exclude the possibility that subtle differences in the relative proportion of the 2 spliced variants exist in certain situations and that such events may be influenced by methylation of exons 16-18 and local chromatin conformation. However, the conservation of this splicing pattern in Drosophila, which lost DNA methylation enzymology, implies that this particular epigenomic modification is not necessary to produce both isoforms (gene CG6206 in in ref 23).
Discussion
Our analyses of multiple obligatory epialleles of the honeybee LAM gene lend support to the emerging view that differentially methylated regions, associated with guiding phenotypes in a context-dependent manner, are in part driven by the underlying DNA sequence. Using colonies founded by single drone inseminated queens (SDI hives) to follow the inheritance of the methylation patterns linked to each allele cross-generationally, we have confirmed the allele-methylation relationship. Across all SDI hives we found clear linkage between a given allele and either the methylated or non-methylated state, with the methylation patterns following Mendelian inheritance patterns. The level of methylation was found to be relatively stable across tissue types and developmental stages, with only subtle variations observed for age and tissue type. In view of our findings, it is likely that these variations reflect different programming conditions associated with specific tissues or developmental stages. However, they also may result from stochastic fluctuations in the level of DNA methylation at a given CpG site as shown recently in mammalian cells.Citation34
Evidence has highlighted the correlation between intragenic DNA methylation and active transcription, in both honeybees and mammals.Citation35,36 Our findings here support this view, with an increase in methylation of AmLAM correlated with an increase in expression. This effect, however, was not found across all contexts, but only in earlier stages of development when AmLAM activity is at its peak, highlighting that such alterations to transcription occur in a highly context-dependent manner. One possibility is that exonic methylation together with trans-acting factors operates as an enhancing system that amplifies the transcript's levels in those situations when more LAM is required. More detailed examination of specific tissue and even cell types may reveal more situations whereby expression of AmLAM is modulated by methylation.
Interestingly, one allele, LAMC+CT, appears to occur in 2 methylated states (differential methylation occurring at CpGs 4 and 9). At present we have not identified any additional underlying genetic polymorphisms that could explain these differences. It is possible that an unknown trans-acting factor is influencing the differential methylation in this region. Given that in 2 SDI hives this is maintained cross-generationally seems specific rather than stochastic. Another possibility is that this pattern originated in a previous generation by stochastic de novo methylation in the grandparents and was observed as stable in the 2 generations analyzed in our study. However, if methylation density at a given region rather than differences at individual CpGs influences downstream transcriptional events,Citation37,38 the differential methylation of the LAMC+CT allele may not necessarily be phenotypically relevant.
The correlation between a novel lncRNA antisense transcript produced from the AmLAM locus and methylation is also of interest. Again, this appears to be context-dependent, with more significant differences found during larval development and pupal stages. Given that these stages are associated with rapid growth, anatomical changes, and the switching of key developmental pathways, it is possible this lncRNA modulates expression of additional regulatory factors. At present, we can only speculate on the function of this lncRNA. Although future experiments may shed more light on the functional importance of this gene, lncRNAs represent a special challenge in experimental studies even in more advanced mammalian systems.Citation39 This is best illustrated by many distinct models attempting to explain lncRNA molecular mechanisms.Citation40
DNA methylation in A. mellifera plays an important role in generating phenotypic outcomes.Citation19,41 Methylation in this organism occurs intragenically, where it is associated with active transcription and alternative splicing and appears to be largely involved in modulating the transcription of highly conserved ‘housekeeping’ genes whose products provide the essential cellular processes, in particular energy and metabolic flux.Citation20,42 Such constitutively expressed genes supply essential activities without which cells cannot properly function. In spite of their perpetual activation, the products of 'housekeeping' genes might be required at different levels throughout development,Citation43 or under changing environmental conditions. Indeed, evidence suggests that even the most stable transcripts are sensitive to both biotic and abiotic external influences.Citation44,45 Our data provide evidence that context-dependent levels of 'housekeeping' transcripts and their products can be modulated by differential methylation that could either intensify or weaken the transcriptional efficiency of constitutively expressed genes. Higher levels of AmLAM can be advantageous in various situations requiring an increased metabolic and energy flux, such as accelerated larval growth, or building the adult structures from imaginal discs. Also, given a relatively high activity of AmLAM in the mucus gland of male reproductive apparatus (not shown), these epialleles could play a role in sperm competition, an important part of sexual selection in honeybees and other social insects whose queens mate with multiple males.Citation46 In a broader context, the coexistence of differentially methylated conditionally-expressed epialleles in the honeybee population could be highly advantageous for a self-organizing system as a mechanism generating stochastic phenotypic variation from which the colony can select most appropriate responses in changing environments.Citation47,48 A general idea emerging from this and similar studies is that epigenomic dynamics is not only influenced by polymorphic changes in DNA, but also that sequence variants may be necessary to ensure the high level of flexibility of the epigenetic code.
Several authors have argued that genomic imprinting via differential methylation of genomic loci has to occur in haplo-diploid social insects as a mechanism of genomic competition within a society with high level of relatedness.Citation49,50 Our data, while not excluding parental imprinting in the honeybees, explicitly show that differential methylation per se cannot be used to support such notions. Instead, meticulous genetic and molecular analyses of parent-specific expression of epialleles have to be conducted in various contexts.
The extent to which sequence variants in the honeybee are associated with obligatory epialleles affecting both methylation and expression on the whole genome scale is not known. We have found at least 220,000 SNPs in the honeybee genome that have the potential to modify genomic methylation either by removing or adding the CpG target (NCBI SNP database: ftp://ftp.ncbi.nlm.nih.gov/snp/organisms/bee_7460). Given the relatively small number of methylated CpGs in the honeybee and the multiple-male mating behavior of queens in this species, the impact of DNA polymorphism on epigenetically influenced phenotypes could be substantial. Importantly, our findings suggest that the currently available methylomes,Citation20,51 which are processed via BS-map filtering that eliminates most SNP-generated CpGs as sequencing errors, need to be carefully reanalyzed to improve their applicability in epigenetic studies. Only fully annotated methylomes can be used to evaluate the physiological and evolutionary potential of epialleles generated by sequence polymorphism.
To date, only a small number of studies investigated the contribution of a genetic component to variable DNA methylation and gene expression, as well as genome-level differences in gene expression linked to DNA methylation. In a recent effort, Wagner et al.Citation36 have shown that although genetic and methylation variation jointly explain 31% of gene expression variation in their fibroblast samples, the underlying mechanisms appear multifaceted and diverse, with a close interplay with other epigenetic marks. Importantly, their results show that in addition to positive correlation similar to our findings, intragenic DNA methylation can also be negatively correlated with gene expression, raising a possibility that various contexts may influence the actual directionality. They reasoned that further studies on inter-individual variation in histone marks and chromatin accessibility, ideally in an allele-specific manner, may bring the framework necessary to the interpretation of sequence and methylation variation. In this context, our data suggest that rapid progress in the understanding of the genotype-methylome-transcriptome relationship can be achieved using relevant, easily accessible systems with measurable phenotypic endpoints. Studies in organisms with smaller, sparsely methylated genomes, such as the honeybee, reduce the molecular complexity to manageable components. Our studies in the honeybee suggest that further analyses of genes like AmLAM may help us not only overcome some general bottlenecks in this field regarding the interdependence of genetic and epigenetic factors and their coordination via specific pathways, but also assign causality to DNA methylation above the levels of genes and proteins, especially with complex phenotypes.
Methods
Biological material
SDI hives were established as described in Lockett et al. Citation21 using artificially inseminated queens from Dewar Apiaries (Queensland). Drone fathers were killed and preserved in 95% ethanol before shipment. All collected specimens were snap frozen in liquid nitrogen and stored at −80°C. Handling and dissections were done as per our standard protocols.Citation52-54 A total of 10 colonies were initially sampled to identify allelic variants. To analyze the effect of allelic variation on the methylation state we wanted to compare alleles within a hive and between hives; we selected 3 SDI hives where heterozygous individuals were present, and where the allelic variants could be compared in this manner. The total number of individuals analyzed by BS-seq was 21 (10 from hive 1, 5 from hive 2, and 6 from hive 3). For expression analysis, individuals from 3 methylated states were analyzed: 9 without methylation, 33 with one methylated allele, and 16 with both alleles methylated.
DNA and RNA extraction
DNA and RNA isolation were performed as described previously.Citation54-56 Briefly, DNA was purified using the MasterPure DNA purification kit from Epicentre Biotechnologies. For expression analysis RNA was extracted using TRIzol® (Invitrogen), and where DNA was required from the same starting material DNA was extracted from the Trizol interface using a standard phenol-chloroform method. To assess RNA quality 1-2 µL of each RNA sample was denatured with formamide, containing 0.01% SybrGreen and evaluated by agarose gel electrophoresis (1.5% agarose; 20 V/cm).
Sanger sequencing
To perform direct sequencing 20 ng of genomic DNA was amplified with AmLAM specific primers: 5′TGGCAAAGAGA-TAATAACGAGATA3′, and 5′ TCTTATTTAGACGCTTTGG-TAGTA3’. The PCR product was purified utilizing Agencourt® AMPure® XP PCR Purification system (Beckman Coulter). Purified PCR product (100 ng) was sequenced with 9.6 pmol of the above primers, along with the primers 5′CTTCAACTGTTGCTTCGT-CCA3′ and 5′CCACTTACTTGCGAGGTAGCA3′, and sequencing was performed using the Applied Biosystems 3730 capillary sequencer.
Genotyping
AmLAM alleles were determined via a combination of direct sequencing (see above) and genotyping of 3 polymorphisms (, Table S2): pm-1, a 12bp deletion in exon16 (ex16del); pm-2, c.2825 A>T SNP (ex18A>T), and pm-3, c.2241C>T SNP (ex15C>T). For all 3 polymorphisms 20ng of genomic DNA was amplified. For pm-1 PCR products were run into a 2% agarose gel at 8V/cm, and products were sized utilizing a 100 bp DNA ladder (Promega™). For pm-2 and pm-3 PCR products were digested utilizing RsaI or NlaIII as per the manufacturer's protocol (New England Biolabs). The digested PCR product was then run into a 2% agarose gel at 8 V/cm, and sized utilizing a 100 bp DNA ladder (Promega™).
DNA bisulfite conversion and amplicon preparation
Genomic DNA (1.5 µg) was bisulfite converted using the QIAGEN Epitect® Bisulfite Kit, as per the manufacturer's protocol. The converted DNA was amplified via a nested PCR reaction with AmLAM specific primers (see Table S2 for BS-seq specific primers). The PCR products were purified utilizing Agencourt® AMPure® XP PCR Purification system (Beckman Coulter).
NGS library preparation
Libraries were prepared from 500-600 ng of each amplicon utilizing the NEBNext® DNA Library Prep Master Mix for Illumina®, and NEBNext® Multiplex Oligos for Illumina® Index Primers Set1 and Set2 (New England Biolabs). Size selection of adaptor ligated DNA was performed using Agencourt AMPure XP beads (Beckman Coulter), with the bead:DNA ratio of the first bead selection 0.9X, followed by a second bead selection with bead:DNA ratio at 0.2X. Each library was eluted in 30 µL of 0.1X TE, library size confirmed via agarose gel electrophoresis, and diluted to a final concentration of 4 nM.
NGS MiSeq sequencing
Next generation sequencing was performed on Illumina MiSeq instrument using MiSeq Reagent Kit v3 (Illumina) and 600 cycles. PhiX spike was added at 5% concentration as recommended by Illumina for low-diversity libraries.
Bisulfite Sanger sequencing
Purified PCR products were digested with EcoRI and HindIII and cloned into pBluescript KS+ plasmid (Stratagene). Recombinant clones were identified via white-blue selection on X-gal plates and false-positives were excluded after electrophoretic analysis of plasmid sizes in phenol-chloroform bacterial lysates. Plasmids for Sanger sequencing were prepared from 3 ml liquid cultures of recombinants using QIAprep Spin Miniprep Kit (QIAGEN). Sanger sequencing was performed by AGRF Brisbane. Sequencing results were visualized using ChromasPro v.1.11 (Technelysium P/L).
Genome-wide methylation analysis
A custom script was written to extract differential methylation data from whole-genome bisulfite sequencing results. Genomic scaffold coordinates of each methylated cytosine together with methylation level information from each BS-seq sample were combined in a table and sorted on position. Using a window of 500 nucleotides the table was searched for regions containing at least 10 mCpGs with an average difference of not less than 50% between 2 selected samples.
Analysis of BS-seq results
For each individual analyzed, the frequency at which a mCpG occurred was calculated across all reads using custom Python scripts and open-source software. The process comprised of 2 steps. In the first, pairs of reads with the 30 nucleotide sequence starting at position 4 matching exactly the last 30 nucleotides of the primers used for nested amplicon PCR were extracted from FASTQ files, aligned with in silico bisulfite-converted genomic template using MUSCLE,Citation57 overlapping regions (if any) were proportionally truncated and, after removing all aligner-introduced gaps, both reads were combined into one continuous sequence and appended to a separate file for each amplicon and each library/sample. In addition, a quality filter was applied, rejecting all sequences shorter than 90% of the length of the template or containing in excess of 5% gaps; an adjustment was applied in relation to indel-containing SVs/alleles. In the second step, batches of sequences from the “extract” files were re-aligned with the template using MUSCLE (to eliminate any potential positional errors introduced by read indels), the aligned template sequence was used to calculate positional information of all the expected CpGs and SNPs, and the positional data were used to score methylation status (i.e., 0 for T and 1 for C occurring at a CpG position) and extract SV data (i.e., the nucleotide at a SNP position and the read length for indels) for each combined read pair. The data were next appended to a separate table for each amplicon and each library/sample. The final tables were used to calculate and graph allele-specific methylation data using Excel (and PIL scripts for some graph types). Methylation density was calculated as the percentage of methylated CpG motifs found across the AmLAM amplicons.
Expression analysis
AmLAM lncRNA antisense and allele-specific transcripts levels were quantitated via RT-PCR (see Table S2 for quantitative PCR primers). cDNA was synthesized from 2.5 µg of RNA using Maxima reverse transcriptase (Thermo Scientific), as per the manufacturer's protocol and amplified using a SYBR® green I based assay. All RT-PCR experiments were performed utilizing the Applied Biosystems® StepOnePlus™ Real-Time PCR System. Gene expression was normalized against both CAM and TBP, and relative expression calculated utilizing the 2−ΔΔCT method, as previously described.Citation58-60
Disclosure of potential conflicts of interest
No potential conflicts of interest were disclosed.
Supplemental material
Supplemental data for this article can be accessed on the publisher's website.
KEPI_A_1107695_supplementary_material.pdf
Download PDF (3.3 MB)Acknowledgments
We thank 2 anonymous referees for their appreciation of the topic of our study and for their astute comments regarding the content of the study. We also thank Paul Helliwell and Joanna Maleszka for their valuable help with handling and preparations of biological material.
Funding
This work was supported by the National Health and Medical Research Council project grant APP1050593 and the Australian Research Council Discovery grant DP120101803 awarded to RM.
References
- Pikaard CS, Scheid OM. Epigenetic regulation in plants. Csh Perspect Biol 2014; 6:a019315
- Feil R, Fraga MF. Epigenetics and the environment: emerging patterns and implications. Nat Rev Genet 2012; 13:97-109; PMID:22215131
- Law JA, Jacobsen SE. Establishing, maintaining and modifying DNA methylation patterns in plants and animals. Nat Rev Genet 2010; 11:204-20; PMID:20142834; http://dx.doi.org/10.1038/nrg2719
- Richards EJ. Opinion - Inherited epigenetic variation - revisiting soft inheritance. Nat Rev Genet 2006; 7:395-U2; PMID:16534512; http://dx.doi.org/10.1038/nrg1834
- McDaniell R, Lee BK, Song L, Liu Z, Boyle AP, Erdos MR, Scott LJ, Morken MA, Kucera KS, Battenhouse A, et al. Heritable Individual-Specific and Allele-Specific Chromatin Signatures in Humans. Science 2010; 328:235-9; PMID:20299549; http://dx.doi.org/10.1126/science.1184655
- Kasowski M, Kyriazopoulou-Panagiotopoulou S, Grubert F, Zaugg JB, Kundaje A, Liu YL, Boyle AP, Zhang QC, Zakharia F, Spacek DV, et al. Extensive Variation in Chromatin States Across Humans. Science 2013; 342:750-2; PMID:24136358; http://dx.doi.org/10.1126/science.1242510
- Gutierrez-Arcelus M, Lappalainen T, Montgomery SB, Buil A, Ongen H, Yurovsky A, Bryois J, Giger T, Romano L, Planchon A, et al. Passive and active DNA methylation and the interplay with genetic variation in gene regulation. Elife 2013; 2:e00523; PMID:23755361
- Schubeler D. Epigenetic Islands in a Genetic Ocean. Science 2012; 338:756-7; PMID:23139324; http://dx.doi.org/10.1126/science.1227243
- Eichten SR, Briskine R, Song J, Li Q, Swanson-Wagner R, Hermanson PJ, Waters AJ, Starr E, West PT, Tiffin P, et al. Epigenetic and Genetic Influences on DNA Methylation Variation in Maize Populations. Plant Cell 2013; 25:2783-97; PMID:23922207; http://dx.doi.org/10.1105/tpc.113.114793
- Schmitz RJ, He YP, Valdes-Lopez O, Khan SM, Joshi T, Urich MA, Nery JR, Diers B, Xu D, Stacey G, et al. Epigenome-wide inheritance of cytosine methylation variants in a recombinant inbred population. Genome Research 2013; 23:1663-74; PMID:23739894; http://dx.doi.org/10.1101/gr.152538.112
- Kerkel K, Spadola A, Yuan E, Kosek J, Jiang L, Hod E, Li K, Murty VV, Schupf N, Vilain E, et al. Genomic surveys by methylation-sensitive SNP analysis identify sequence-dependent allele-specific DNA methylation. Nat Genet 2008; 40:904-8; PMID:18568024; http://dx.doi.org/10.1038/ng.174
- Schilling E, El Chartouni C, Rehli M. Allele-specific DNA methylation in mouse strains is mainly determined by cis-acting sequences. Genome Research 2009; 19:2028-35; PMID:19687144; http://dx.doi.org/10.1101/gr.095562.109
- Shoemaker R, Deng J, Wang W, Zhang K. Allele-specific methylation is prevalent and is contributed by CpG-SNPs in the human genome. Genome Research 2010; 20:883-9; PMID:20418490; http://dx.doi.org/10.1101/gr.104695.109
- Lo HS, Wang ZN, Hu Y, Yang HH, Gere S, Buetow KH, Lee MP. Allelic variation in gene expression is common in the human genome. Genome Research 2003; 13:1855-62; PMID:12902379; http://dx.doi.org/10.1101/gr.885403
- Wilkins JF. Genomic imprinting and methylation: epigenetic canalization and conflict. Trends Genet 2005; 21:356-65; PMID:15922835; http://dx.doi.org/10.1016/j.tig.2005.04.005
- Ferguson-Smith AC. Genomic imprinting: the emergence of an epigenetic paradigm. Nat Rev Genet 2011; 12:565-75; PMID:21765458; http://dx.doi.org/10.1038/nrg3032
- Bell AC, Felsenfeld G. Methylation of a CTCF-dependent boundary controls imprinted expression of the Igf2 gene. Nature 2000; 405:482-5; PMID:10839546; http://dx.doi.org/10.1038/35013100
- Boumber YA, Kondo Y, Chen X, Shen L, Guo Y, Tellez C, Estecio MRH, Ahmed S, Issa JPJ. An Sp1/Sp3 Binding Polymorphism Confers Methylation Protection. Plos Genet 2008; 4:e1000162; PMID:18725933; http://dx.doi.org/10.1371/journal.pgen.1000162
- Kucharski R, Maleszka J, Foret S, Maleszka R. Nutritional control of reproductive status in honey bees via DNA methylation. Science 2008; 319:1827-30; PMID:18339900; http://dx.doi.org/10.1126/science.1153069
- Foret S, Kucharski R, Pellegrini M, Feng SH, Jacobsen SE, Robinson GE, Maleszka R. DNA methylation dynamics, metabolic fluxes, gene splicing, and alternative phenotypes in honey bees. P Natl Acad Sci USA 2012; 109:4968-73; http://dx.doi.org/10.1073/pnas.1202392109
- Lockett GA, Kucharski R, Maleszka R. DNA methylation changes elicited by social stimuli in the brains of worker honey bees. Genes Brain Behav 2012; 11:235-42; PMID:22098706; http://dx.doi.org/10.1111/j.1601-183X.2011.00751.x
- Herb BR, Wolschin F, Hansen KD, Aryee MJ, Langmead B, Irizarry R, Amdam GV, Feinberg AP. Reversible switching between epigenetic states in honey bee behavioral subcastes. Nature Neuroscience 2012; 15:1371-3; PMID:22983211; http://dx.doi.org/10.1038/nn.3218
- Maleszka R. The social honey bee in biomedical research: realities and expectations. Drug Discovery Today Disease Models 2014; 12:7-13; http://dx.doi.org/10.1016/j.ddmod.2014.06.001
- Guzman-Novoa E, Hunt GJ, Page RE, Jr., Uribe-Rubio JL, Prieto-Merlos D, Becerra-Guzman F. Paternal effects on the defensive behavior of honey bees. J Hered 2005; 96:376-80; PMID:15743904; http://dx.doi.org/10.1093/jhered/esi038
- Unger P, Guzman-novoa E. Maternal effects on the hygienic behavior of Russian x Ontario hybrid honey bees (Apis mellifera L.). J Hered 2010; 101:91-6; PMID:19889722; http://dx.doi.org/10.1093/jhered/esp092
- Oldroyd BP, Allsopp MH, Roth KM, Remnant EJ, Drewell RA, Beekman M. A parent-of-origin effect on honey bee worker ovary size. Proc Biol Sci 2014; 281:20132388; PMID:24285196; http://dx.doi.org/10.1098/rspb.2013.2388
- Kocher SD, Tsuruda JM, Gibson JD, Emore CM, Arechavaleta-Velasco ME, Queller DC, Strassmann JE, Grozinger CM, Gribskov MR, San Miguel P, et al. A Search for Parent-of-Origin Effects on Honey Bee Gene Expression. G3 (Bethesda) 2015; 5:1657-62; PMID:26048562; http://dx.doi.org/full_text
- Liao YF, Lal A, Moremen KW. Cloning, expression, purification, and characterization of the human broad specificity lysosomal acid alpha-mannosidase. J Biol Chem 1996; 271:28348-58; PMID:8910458; http://dx.doi.org/10.1074/jbc.271.45.28348
- Gonzalez DS, Jordan IK. The alpha-mannosidases: Phylogeny and adaptive diversification. Mol Biol Evol 2000; 17:292-300; PMID:10677852; http://dx.doi.org/10.1093/oxfordjournals.molbev.a026309
- Borgwardt L, Lund AM, Dali CI. Alpha-mannosidosis - a review of genetic, clinical findings and options of treatment. Pediatr Endocrinol Rev 2014; 12 Suppl 1:185-91; PMID:25345101
- Hatje K, Kollmar M. Expansion of the mutually exclusive spliced exome in Drosophila. Nat Commun 2013; 4:2460; PMID:24025855; http://dx.doi.org/10.1038/ncomms3460
- Nemcovicova I, Sestak S, Rendic D, Plskova M, Mucha J, Wilson IBH. Characterisation of class I and II alpha-mannosidases from Drosophila melanogaster. Glycoconjugate J 2013; 30:899-909; http://dx.doi.org/10.1007/s10719-013-9495-5
- Kucharski R, Foret S, Maleszka R. EGFR gene methylation is not involved in Royalactin controlled phenotypic polymorphism in honey bees. Sci Rep-Uk 2015; 5:14070; http://dx.doi.org/10.1038/srep14070
- Landan G, Cohen NM, Mukamel Z, Bar A, Molchadsky A, Brosh R, Horn-Saban S, Zalcenstein DA, Goldfinger N, Zundelevich A, et al. Epigenetic polymorphism and the stochastic formation of differentially methylated regions in normal and cancerous tissues. Nat Genet 2012; 44:1207-14; PMID:23064413; http://dx.doi.org/10.1038/ng.2442
- Lyko F, Maleszka R. Insects as innovative models for functional studies of DNA methylation. Trends Genet 2011; 27:127-31; PMID:21288591; http://dx.doi.org/10.1016/j.tig.2011.01.003
- Wagner JR, Busche S, Ge B, Kwan T, Pastinen T, Blanchette M. The relationship between DNA methylation, genetic and expression inter-individual variation in untransformed human fibroblasts. Genome Biol 2014; 15:R37; PMID:24555846; http://dx.doi.org/10.1186/gb-2014-15-2-r37
- Riggs AD, Xiong Z. Methylation and epigenetic fidelity. Proc Natl Acad Sci U S A 2004; 101:4-5; PMID:14695893; http://dx.doi.org/10.1073/pnas.0307781100
- Jeltsch A, Jurkowska RZ. New concepts in DNA methylation. Trends Biochem Sci 2014; 39:310-8; PMID:24947342; http://dx.doi.org/10.1016/j.tibs.2014.05.002
- Bassett AR, Akhtar A, Barlow DP, Bird AP, Brockdorff N, Duboule D, Ephrussi A, Ferguson-Smith AC, Gingeras TR, Haerty W, et al. Considerations when investigating lncRNA function in vivo. Elife 2014; 3:e03058; PMID:25124674; http://dx.doi.org/10.7554/eLife.03058
- Wang KC, Chang HY. Molecular mechanisms of long noncoding RNAs. Mol Cell 2011; 43:904-14; PMID:21925379; http://dx.doi.org/10.1016/j.molcel.2011.08.018
- Maleszka R. Epigenetic integration of environmental and genomic signals in honey bees: the critical interplay of nutritional, brain and reproductive networks. Epigenetics 2008; 3:188-92; PMID:18719401; http://dx.doi.org/10.4161/epi.3.4.6697
- Foret S, Kucharski R, Pittelkow Y, Lockett GA, Maleszka R. Epigenetic regulation of the honey bee transcriptome: unravelling the nature of methylated genes. BMC Genomics 2009; 10:472; PMID:19828049; http://dx.doi.org/10.1186/1471-2164-10-472
- Butte AJ, Dzau VJ, Glueck SB. Further defining housekeeping, or “maintenance,” genes Focus on “A compendium of gene expression in normal human tissues”. Physiol Genomics 2001; 7:95-6; PMID:11773595
- Vandesompele J, De Preter K, Pattyn F, Poppe B, Van Roy N, De Paepe A, Speleman F. Accurate normalization of real-time quantitative RT-PCR data by geometric averaging of multiple internal control genes. Genome Biol 2002; 3:RESEARCH0034; PMID:12184808; http://dx.doi.org/10.1186/gb-2002-3-7-research0034
- Gibson G. The environmental contribution to gene expression profiles. Nat Rev Genet 2008; 9:575-81; PMID:18574472; http://dx.doi.org/10.1038/nrg2383
- den Boer SP, Baer B, Boomsma JJ. Seminal fluid mediates ejaculate competition in social insects. Science 2010; 327:1506-9; PMID:20299595; http://dx.doi.org/10.1126/science.1184709
- Feinberg AP, Irizarry RA. Stochastic epigenetic variation as a driving force of development, evolutionary adaptation, and disease. P Natl Acad Sci USA 2010; 107:1757-64; http://dx.doi.org/10.1073/pnas.0906183107
- Maleszka R, Mason PH, Barron AB. Epigenomics and the concept of degeneracy in biological systems. Brief Funct Genomics 2014; 13:191-202; PMID:24335757; http://dx.doi.org/10.1093/bfgp/elt050
- Queller DC. Theory of genomic imprinting conflict in social insects. Bmc Evol Biol 2003; 3:15; PMID:12871603; http://dx.doi.org/10.1186/1471-2148-3-15
- Drewell RA, Lo N, Oxley PR, Oldroyd BP. Kin conflict in insect societies: a new epigenetic perspective. Trends Ecol Evol 2012; 27:367-73; PMID:22483741; http://dx.doi.org/10.1016/j.tree.2012.02.005
- Lyko F, Foret S, Kucharski R, Wolf S, Falckenhayn C, Maleszka R. The Honey Bee Epigenomes: Differential Methylation of Brain DNA in Queens and Workers. Plos Biol 2010; 8:e1000506; PMID:21072239; http://dx.doi.org/10.1371/journal.pbio.1000506
- Maleszka J, Barron AB, Helliwell PG, Maleszka R. Effect of age, behaviour and social environment on honey bee brain plasticity. J Comp Physiol A Neuroethol Sens Neural Behav Physiol 2009; 195:733-40; PMID:19434412; http://dx.doi.org/10.1007/s00359-009-0449-0
- Dickman MJ, Kucharski R, Maleszka R, Hurd PJ. Extensive histone post-translational modification in honey bees. Insect Biochem Molec 2013; 43:125-37; http://dx.doi.org/10.1016/j.ibmb.2012.11.003
- Wojciechowski M, Rafalski D, Kucharski R, Misztal K, Maleszka J, Bochtler M, Maleszka R. Insights into DNA hydroxymethylation in the honey bee from in-depth analyses of TET dioxygenase. Open Biol 2014; 4:pii: 140110; PMID:25100549; http://dx.doi.org/10.1098/rsob.140110
- Kucharski R, Maleszka R. Arginine kinase is highly expressed in the compound eye of the honey bee, Apis mellifera. Gene 1998; 211:343-9; PMID:9602169; http://dx.doi.org/10.1016/S0378-1119(98)00114-0
- Elsik CG, Worley KC, Bennett AK, Beye M, Camara F, Childers CP, de Graaf DC, Debyser G, Deng JX, Devreese B, et al. Finding the missing honey bee genes: lessons learned from a genome upgrade. BMC Genomics 2014; 15:86; PMID:24479613; http://dx.doi.org/10.1186/1471-2164-15-86
- Edgar RC. MUSCLE: multiple sequence alignment with high accuracy and high throughput. Nucleic Acids Res 2004; 32:1792-7; PMID:15034147; http://dx.doi.org/10.1093/nar/gkh340
- Livak KJ, Schmittgen TD. Analysis of relative gene expression data using real-time quantitative PCR and the 2(T)(-Delta Delta C) method. Methods 2001; 25:402-8; http://dx.doi.org/10.1006/meth.2001.1262
- Lockett GA, Helliwell P, Maleszka R. Involvement of DNA methylation in memory processing in the honey bee. Neuroreport 2010; 21:812-6; PMID:20571459; http://dx.doi.org/10.1097/WNR.0b013e32833ce5be
- Kucharski R, Maleszka R. Microarray and real-time PCR analyses of gene expression in the honey bee brain following caffeine treatment. J Mol Neurosci 2005; 27:269-76; PMID:16280596; http://dx.doi.org/10.1385/JMN:27:3:269