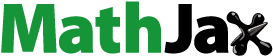
Abstract
Smoking increases the risk of many diseases and could act through changes in DNA methylation patterns. The aims of this study were to determine the association between smoking and DNA methylation throughout the genome at cytosine-phosphate-guanine (CpG) site level and genomic regions. A discovery cross-sectional epigenome-wide association study nested in the follow-up of the REGICOR cohort was designed and included 645 individuals. Blood DNA methylation was assessed using the Illumina HumanMethylation450 BeadChip. Smoking status was self-reported using a standardized questionnaire. We identified 66 differentially methylated CpG sites associated with smoking, located in 38 genes. In most of these CpG sites, we observed a trend among those quitting smoking to recover methylation levels typical of never smokers. A CpG site located in a novel smoking-associated gene (cg06394460 in LNX2) was hypomethylated in current smokers. Moreover, we validated two previously reported CpG sites (cg05886626 in THBS1, and cg24838345 in MTSS1) for their potential relation to atherosclerosis and cancer diseases, using several different approaches: CpG site methylation, gene expression, and plasma protein level determinations. Smoking was also associated with higher THBS1 gene expression but with lower levels of thrombospondin-1 in plasma. Finally, we identified differential methylation regions in 13 genes and in four non-coding RNAs. In summary, this study replicated previous findings and identified and validated a new CpG site located in LNX2 associated with smoking.
Abbreviations
CpG, cytosine-phosphate-guanine; lncRNA, long non-coding RNA; miR, micro RNA; EWAS, epigenome-wide association study; THBS-1, thrombospondin-1; PCR, polymerase chain reaction | = |
Introduction
Smoking is the second leading risk factor for global disease burden worldwide.Citation1 Recent studies estimate that the rate of death from any cause is almost three times higher among current smokers, compared to never smokers.Citation2 The health effects associated with smoking are mediated through a variety of mechanisms that are not fully understood. These include direct DNA damage, vascular dysfunction, inflammation, and platelets functionality, among others.Citation3-8
Changes in DNA methylation could be one of the mechanisms mediating the relationship between smoking and adverse health outcomes. DNA methylation is a heritable but also reversible addition of a methyl group to a nucleotide, and plays an important role in gene and non-coding RNAs transcriptional regulation.Citation9,10 In a cytosine-phosphate-guanine (CpG) dinucleotide context, cytosines are the main substrate for DNA methyltransferase enzymes in mammals, and an association between smoking and DNA methylation at different loci has been reported.Citation11-17 Most of these studies have analyzed individual CpG sites across the genome. Although a single methylated CpG may be linked to gene expression regulation and may affect disease risk, some of the loci reported in the literature to be related to this regulation are genomic regions within a size range of a few hundred to a few thousand bases.Citation18 Evidence of a relation between smoking and DNA methylation at the genomic region level is lacking.
The aim of the present study was to determine the association between smoking and blood DNA methylation in a population-based survey. We aimed at replicating the results obtained in previous studies, and identifying and validating methylation CpG sites associated with smoking exposure. A secondary aim was to identify differentially methylated genomic regions related to smoking.
Results
A total of 645 individuals passed the sample quality controls and were included in the analyses (only 3 were excluded). The main sociodemographic and clinical characteristics of the participants across the defined smoking groups are shown in . Current smokers were younger and a higher proportion were men, compared to never smokers. There were also differences in the lipid profile and physical activity practice across smoking groups. Of the initial 482,421 CpG sites, 427,948 (88.7%) passed the probe quality controls and were analyzed in the discovery phase.
Table 1. Descriptive characteristics of the participants in the discovery phase across smoking groups.
According to the aims of the study and the statistical analysis plan, we present the association between smoking and methylation at both the individual CpG site and region levels.
Association between smoking and methylation at the individual CpG sites
Discovery analysis
We tested the association between smoking and each of the CpG sites separately, comparing the current- and never-smoker groups. The Manhattan plot showing the P-values for the associations at each CpG site and the QQ plot corresponding to the relationship between observed and predicted P-values are included in Figures S1 and S2, respectively. We identified 66 CpG sites in 38 genes that showed differential DNA methylation associated with smoking. At 63 of these sites, current smokers were hypomethylated compared to the never-smoker group (, Table S1, and Fig. S3). A differentially methylated CpG site, cg06394460, was novel and not previously described as associated with smoking. This CpG was located in the ligand of numb-protein X2 gene (LNX2). Among the other 65 CpG sites, we identify two recently reported CpGs located in thrombospondin 1 gene (THBS1, cg05886626) and in metastasis suppressor 1 gene (MTSS1, cg24838345), which could be of interest in arteriosclerosis and cancer, and selected them for further validation. As a sensitivity analysis, we performed the same analyses adjusting for cell count types (Table S1), adjusting for HDL-cholesterol levels as a potential confounderCitation19 (Table S2), stratifying by sex (Table S3), and excluding those participants with previous coronary heart disease (Table S4); the results were consistent.
Table 2. CpG sites differentially methylated in relation to smoking in the discovery cohort. The CpG id, associated gene or transcript, chromosome (chr) location, genomic position, and observed mean β-values (standard deviation) across smoking defined groups are shown.
We observed a linear trend, from current smokers to former and never smokers, of increased (in 63 CpG sites) and decreased (in three CpG sites) methylation across the four smoking groups defined (, , Table S1, Fig. S3). We also observed that methylation at cg05575921, the top hit, had a very high discriminative capacity to identify current smokers vs. never smokers [receiver operating characteristic (ROC) area under the curve = 0.926].
Validation of the CpG sites associated with smoking
Genome-wide array in an independent sample
A basic description of the BAsicMAR validation sample is shown in Table S5. Current smokers showed lower methylation in the three CpG sites of interest, with P-values ranging from 0.011 to 0.004. ().
Table 3. Differences in methylation level in peripheral blood cells, in peripheral blood cells gene expression and in protein plasma levels between current smokers and never smokers in the three new genes identified in the discovery phase. Results are shown as adjusted beta values mean (and standard error)*.
Table 4. Differentially methylated genomic regions in relation to smoking in the discovery cohort. The Ensembl genomic id, chromosome (chr) location, genomic position, strand, associated gene or transcript, and observed mean β-values in current and never smokers are shown.
CpG site-specific pyrosequencing assay
A basic description of the REGICOR validation sample is shown in Table S6. Smoking was also associated with lower methylation in the three CpG sites of interest, with P-values ranging from 0.011 to 5.51x10−11 . ().
Gene expression analysis
A basic description of the PREDIMED validation sample is shown in Table S7. The LNX2 gene was not present in the array and could not be evaluated. The level of THBS1 gene expression was higher among smokers than never smokers; no differences were observed in MTSS1 gene expression.().
THBS1 plasma levels
A basic description of the TALAVERA validation sample is shown in Table S8.
Current smokers showed lower levels of plasma THSB1 compared to never smokers.().
Association between smoking and DNA methylation at the region level
Using an analytical approach based on predefined genomic regions, we identified several differentially methylated regions related to smoking (): i) 7 promoters corresponding to the genes GNG12, ALPPL2, LRRN3, AGAP2-AS1, IGHJ6, IGHJ3P, and IGHJ5; ii) 8 gene bodies, ALPPL2, GPR15, MYO1G, LRRN3, IGHJ3P, CLEC16A, ZNRF1, and F2RL3; iii) 3 CpG islands related to the genes ALPPL2, MYO1G, and PRF1; and iv) 4 non-coding RNAs (lncRNA1447, KIAA0087, miR-802, and Loc728554).
All regions were hypomethylated in smokers, with the exception of those related to the genes MYO1G and PRF1, and the lncRNA1447.
Discussion
In the present study, we replicated differential methylation in CpG sites located in 37 genes previously associated with smoking. Moreover, we discovered and validated a new CpG site (in LNX2) and we also further validated two already known CpG sites (in THSB1 and MTSS1) which could be related with arteriosclerosis and cancer disease, showing lower methylation levels in current smokers compared to never smokers. We selected cg05886626, located at the THSB1 gene (coding for thrombospondin-1), and we observed that smoking was associated with higher THSB1 gene expression levels and, paradoxically, lower concentrations of thrombospondin-1 in plasma. We also report a linear trend in the differentially methylated sites across smoking groups (i.e., current, former, and never smokers), suggesting that this could be a reversible process. Finally, we analyzed the relation between smoking and methylation at a genomic region level and identified differential methylation levels in 13 genes and in regions corresponding to four non-coding RNAs (lncRNA01447, KIAA0087, miR-802, and Loc728554).
The present study replicated findings in a number of CpGs located in 37 genes previously reported to show a differential methylation pattern associated with smoking.Citation12-15,17,20,22 The top hit reported in the present study (cg05575921, P-value 4.11 × 10−84 ) lies in the aryl hydrocarbon receptor repressor gene (AHRR) and replicated the direction and magnitude of the association reported in previous studies.Citation11-16 The differential methylation of this CpG site provides very high discriminative capacity, and therefore could be used as an objective biomarker of active smoking, as has been also reported in a recent study.Citation23 The protein encoded by this gene participates in the aryl hydrocarbon receptor signaling cascade, which mediates dioxin toxicity, and also is involved in regulation of cell growth and differentiation. Tobacco smoke contains more than 3800 constituents, including numerous water-insoluble polycyclic aromatic hydrocarbons that modulate aryl hydrocarbon receptor signaling pathways.Citation24
Our study also replicated the results obtained in the first epigenome-wide association study (EWAS) evaluating the association between DNA methylation in blood and smoking status in adults in a European population.Citation11 The earlier study showed that smoking was associated with lower methylation in the F2RL3 locus [coagulation factor II (thrombin) receptor-like 3]. The product of this gene plays an important role in processes such as platelet activation, intimal hyperplasia, and inflammation. Moreover, F2RL3 methylation levels was associated with mortality in patients with stable coronary heart disease, indicating it is a potential mediator of the impact of smoking.Citation20
The novel contribution of our study is the identification of a new hypomethylated CpG site located in the LNX2 gene that is associated with smoking. Moreover, we provide more knowledge about the relationship between THBS1 and MTSS1 genes and smoking exposition.
The novel hit, LNX2, encodes for the protein ligand of numb-protein X2, an E3 ubiquitin ligase giving specificity to the ubiquitylation process by selectively binding substrates. Recently, its function has emerged as a crucial modulator of T-cell tolerance and immunity. However, substrates, partners, and mechanisms of action remain largely unknown for most E3 ligases. This gene has been previously reported to be associated with adenocarcinoma, epithelial neoplasia and preeclampsia.Citation25 A recent study has also documented that this protein was upregulated during osteoclast differentiation.Citation26
The second interesting hit, THBS1 encodes for thrombospondin-1 (THBS-1), an extracellular matrix, and a calcium-binding protein that regulates cellular adhesion and migration, cytoskeletal organization and cell proliferation, and apoptosis. THBS-1 is present within blood vessels interacting with various proteins to maintain vascular structure and haemostasis.Citation27 Current knowledge related to the effects of THBS-1 on different cellular mechanisms is conflicting and depends on the experimental settings. Some studies have shown a protective action on the vasculature and myocardial healing after a myocardial infarction,Citation28,29 although others observed higher THBS-1 levels in patients with atherosclerotic-related phenotypes.Citation30,31 These higher THBS-1 levels could be a marker for the reparative process during disease progression and not a cause of the disease. A recent meta-analysis of the association between genetic variants in thrombospondin genes and myocardial infarction did not find evidence that the polymorphisms included were associated with myocardial infarction,Citation32 questioning the causal association between this protein and the disease. Independently of the pathogenic role of THBS-1, we report that current smoking was associated with a reduced methylation of the THBS1 gene and with increased gene expression but, paradoxically, with lower THBS-1 plasma levels. This paradoxical relationship between methylation/expression and protein levels could be explained by posttranscriptional regulation of the protein levels or by a complex feedback regulation mechanism.(Fig. S4).
Finally, we identified smoking-associated CpG sites located in the metastasis suppressor 1 gene (MTSS1), which is involved in sonic hedgehog and epidermal growth factor signaling, and also acts as a scaffold protein to regulate cytoskeleton. Downregulation of MTSS1 has been linked to the progression and poor prognosis of various cancers.Citation33 In our study, we could not validate the association between smoking and lower MTSS1 methylation when analyzing gene expression. It is known that DNA methylation affects gene expression, and that this mechanism is usually associated with decreased gene expression.Citation9 Nevertheless, this relationship is complex and not always linear.Citation34–36 Moreover, the sample size included in our gene expression analyses was small. Therefore, the lack of association between smoking and MTSS1 expression could be related to a limited statistical power or to more complex mechanisms regulating the expression of this gene.
For almost all of the 66 CpG sites identified, we observed a time-dependent change in methylation levels when former smokers were included in the analyses. These results are also consistent with the results from other studies.Citation13,22,37 Although it is not possible to evaluate the causal effect because of our study design, these results suggest that the differential methylation patterns related to smoking could be reversible.
Finally, as a secondary aim, we analyzed the association between smoking and differentially methylated regions, identifying several genomic regions; some of them were in new genes not previously identified as associated with smoking (AGAP2-AS1, IGHJ3P, IGHJ5, IGHJ6, CLEC16A, ZNRF1, and PRF1). The protein encoded by the AGAP2-AS1 gene is overexpressed in cancer cells and promotes cancer cell invasion. Some genetic variants in the CLC16A gene have been associated with diabetes mellitus, multiple sclerosis, and rheumatoid arthritis. The protein encoded by the PRF1 gene has structural and functional similarities to complement component 9 (C9). Like C9, this is one of the main cytolytic proteins of cytolytic granules, and it is known to be a key effector molecule for T-cell- and natural killer-cell-mediated cytolysis. Moreover, we identified four non-coding RNAs associated with smoking, one of them (mRNA-801) also recently associated with type 2 diabetes and glucose metabolism.Citation38,39 Further studies in independent samples are warranted to validate and replicate these novel results.
Strengths and limitations
In this study we analyzed one of the largest available series of participants with genome-wide methylation data from a population-based survey. We replicated previous CpG sites already known to be associated with smoking, giving strength and consistency to the body of knowledge available, and we identified a novel site. Furthermore, we validated two additional CpG sites using multiple approaches.
Some limitations of the study also should be considered. First, we did not have an objective measurement of smoking exposure. However, self-reported questionnaires have been shown to be a valid instrument for this purpose.Citation40 Second, we measured DNA methylation in peripheral blood cells. It is known that the methylation levels of some CpGs/regions are tissue-specific,Citation41 and we might have lost some signals by not choosing a tissue where smoking could have had a more direct impact on DNA methylation. However, other authors have suggested that studying methylation patterns of whole blood is a good proxy for the methylation levels from a specific site of action.Citation12,14 Third, the magnitude of the differential methylation between smokers and never smokers in the discovery stage was greater when using the Illumina assay, compared to pyrosequencing, in the validation stage. We found non-significant differences between the REGICOR subsamples included in the discovery and validation stages. These differences do not appear to be related to the clinical profile of the participants and could be explained by technical or methodological differences in the measurements used. Fourth, it would have been preferable to assess gene expression and plasma protein levels in the same population where methylation data were available. The clinical characteristics of the population included in the protein level determinations were similar to that of the discovery stage; however, the group of individuals with gene expression data was older, which could introduce some bias that cannot be controlled. Finally, the design of the study is cross-sectional and we cannot infer causality of the reported association between smoking and DNA methylation levels.
In summary, smoking is associated with significant differential DNA methylation across the genome. In this study, we replicated previous findings and identified and validated a new CpG differentially methylated in smokers in LNX2 gene. Moreover, we confirmed and provided more information about THBS1 and MTSS1 and its relation to smoking. We also identified differential methylation at the genomic region level in several genes and in genomic regions corresponding to four non-coding RNAs (lncRNA01447, KIAA0087, miR-802, and Loc728554). Finally, there was a linear trend between current, former, and never smokers in the levels of methylation, suggesting that this is a reversible process.
Materials and Methods
Study design
We designed a cross-sectional EWAS nested in the Girona Heart Registry (REGICOR, which stands for REgistre GIroni del COR), using follow-up data from a population-based cohort enrolled in 2003–2005 (n = 6352; response rate, 71.5%). The towns that participated in REGICOR represent the urban and rural diversity of Girona Province in Catalonia, Spain.Citation42 During 2009–2013, participants still residing in these towns were invited to participate in a follow-up visit; institutionalized residents were excluded. The response rate was 78.4%. A subsample of those attending their follow-up visit was randomly selected for this study (n = 648). All participants were of European descent. The study was approved by the local ethics committee and conducted according to the principles expressed in the Declaration of Helsinki and relevant legislation in Spain. All participants gave written informed consent prior to their participation.
Smoking status and other covariates
Examinations were performed and questionnaires administered by a team of trained nurses. Standardized questionnaires and methods were used to collect sociodemographic, lifestyle, and other information related to cardiovascular risk factors (Supplementary Methods). Self-reported smoking status was categorized in four groups: current smokers (smoked on average ≥1 cigarette/day at the time of the visit or gave up smoking <1 y before the visit); former smokers, between 1 and 5 y (gave up smoking up to 5 y before the visit); former smokers, more than 5 y; and never smokers.
Array-based DNA methylation analysis with Infinium Methylation 450k
DNA was extracted from whole peripheral blood collected in 10 mL EDTA tubes using a standardized method (Puregen TM; Gentra Systems). DNA quality was checked with Picogreen and DNA methylation was assessed using the Illumina HumanMethylation450 BeadChip (Illumina), following the Illumina Infinium HD Methylation protocol. This array covers 485,577 methylation CpG sites with at least one probe in 99% of the RefSeq genes (21,231 genes).Citation43 The arrays were scanned with the Illumina HiScan SQ scanner. These processes were carried out in 2 laboratories of the Spanish National Genotyping Center: the Center for Genomic Regulation in Barcelona and the Centro Nacional de Investigaciones Oncológicas in Madrid. Two repeated samples were included in all the plates (94 unique samples, 2 repeated samples) to take into account batch effects. We performed sensitivity analysis, normalizing the data by the batch effect, and the results were similar.(Table S9).
Thereafter, an M-value was assigned to each CpG, calculated as the log2 ratio of the intensities of the methylated vs. the unmethylated probe for each individual site according to EquationEquation 1(1)
(1) ,Citation44
(1)
(1) where:
- Mi: intensity of methylated probe
- Ui: intensity of unmethylated probe
- α: constant offset (by default α = 1)
An M-value close to 0 means the CpG site is about half-methylated. Positive M-values mean that there are more methylated than unmethylated cytosines; negative M-values indicate the opposite ratio. Due to its good statistical properties, M-value was the main outcome variable used in our analyses.Citation44,45
For the biological interpretation of the results we also obtained the β-values, or the ratio of the intensities of methylated probe to overall intensities, as shown in the EquationEquation 2(2)
(2) ,Citation44
(2)
(2)
In this case, as suggested by Illumina, the constant offset assigned to α was 100. β-value ranges between 0 (completely unmethylated) and 1 (completely methylated).Citation44,45 We assessed the quality control of the entire process and normalized the data using a standardized pipeline and methods (see Supplementary material).
The gene notation was based on the Illumina manifest or on the information provided by the University of California, Santa Cruz Genome Browser website.Citation46 In those CpGs with no annotated gene, the nearest gene was defined using the same Genome Browser.
Validation of the CpG methylation sites of interest associated with smoking
Genome-wide array in an independent sample
An independent sample from the BAsicMAR study was analyzed, consisting of 377 stroke patients with available genome-wide blood methylation data measured with the same array as the present study and smoking exposure evaluated using the same questionnaire.Citation47
CpG site-specific pyrosequencing assay
An additional independent sample of 622 healthy individuals was randomly selected from the REGICOR study participants. Pyrosequencing assays were designed in order to validate the three CpG sites of interest identified in the discovery phase of our study. When possible, specific primer sequences were designed, using the PyroMark assay design software, version 2.0.01.15 (Table S10), to hybridize with CpG-free sites and ensure methylation-independent amplification. Bisulfite-treated DNA was used as a template for a polymerase chain reaction (PCR), carried out with primers biotinylated to convert the PCR product to single-stranded DNA templates. We used the Vacuum Prep Tool (Biotage) to prepare single-stranded PCR products according to manufacturer instructions. Pyrosequencing reactions and fluorimetric quantification of methylation peaks were conducted in a PyroMark Q24 System, version 2.0.6 (Qiagen). To rule out the presence of technical bias in the quantification of DNA methylation values, internal sequence-specific and bisulfite conversion controls were included and considered in the interpretation of the results.
Gene expression analysis
Genome-wide gene expression data were available from an independent subsample of individuals (n = 22 individuals, 16 never smokers, 6 current smokers) participating in the Prevención Con Dieta Mediterránea (PREDIMED) study.Citation48,49 Whole genome peripheral blood mononuclear cells were assessed by using whole transcriptome microarray (Affymetrix Gene Chip Human Genome U133A 2.0) at the Microarrays Analysis Services, Hospital del Mar Medical Research Institute (IMIM), Barcelona, Spain. Raw data passed quality control and were normalized using robust microarray analysis methodology.Citation50 Intensity signals were standardized and log2 transformed.
Determination of THBS1 by dot-blot
In those genes showing differential CpG methylation patterns and also differential gene expression between current and never smokers, we determined the concentration of the related protein in plasma. Only one protein, THBS1, fulfilled this criterion. These measurements were done in an independent sample (n = 267) from a population-based survey undertaken in another region of Spain (Talavera).Citation51
As previously reported,Citation52 we loaded 10 μg of total plasma protein from each individual onto a nitrocellulose membrane. This membrane was blocked with 5% (w/v) bovine serum albumin, incubated with a monoclonal THBS1 antibody (THBS1 antibody sc-59886, Santa Cruz Biotechnology, Inc.., dilution 1:1000), washed, and then incubated with a peroxidase-conjugated anti-mouse IgG developed with enhancing chemiluminescence reagents (ECL, GE Healthcare).
All the laboratory measurements were performed by personnel blinded to participants’ smoking status.
Statistical analysis
We carried out two different types of analysis in the discovery phase: First, we analyzed the association between DNA methylation at all the individual CpG sites and smoking. We included all individuals and CpG sites that passed quality control. A P-value <1.17 × 10−7 was established to define a difference as statistically significant after Bonferroni correction, we considered the number of valid CpGs as the number of independent tests to be done (0.05/427,948). We analyzed differences in CpG site methylation between 2 groups, current smokers and never smokers, using a multivariate linear regression. In those CpG sites associated with smoking, we also performed a multivariate linear regression to assess differences in DNA methylation across the four pre-defined smoking categories (current smokers, former 1–5 y, former >5 y, never smokers). For all adjusted models, methylation was considered the response variable, binary or 4-category smoking status was the explanatory variable of interest according to the analyses performed, and a pre-defined set of variables (sex, age, batch, and physical activity) were included in the models as adjusting covariates. We performed sensitivity analyses adjusting for this set of variables, including high-density lipoprotein cholesterol and blood cell count estimated by the Houseman Algorithm using the minfi R package.Citation53,54 We also stratified by sex and excluded those participants with previous coronary heart disease.
We calculated the statistical power of the discovery EWAS. Accepting an α risk of 1.7 × 10−7 in a 2-sided test, the sample size we included in our main analysis (n = 107 current smokers and n = 342 never smokers) provided us with 80% power to detect as statistically significant differences greater than or equal to 0.54 standard deviation methylation units in the different CpGs.
Second, we analyzed the association between smoking and DNA methylation at the region level. We used dasen normalized data to remove the non-biological variationCitation55,56 and different types of genomic regions were considered: i) whole gene, defined from the transcription start-point to the transcription end-point according to Ensemble notations; ii) promoter regions, defined as regions between positions 1.5-kb upstream and 0.5-kb downstream of the transcription start-points; iii) CpG islands, defined as regions of DNA >200 bp showing a C+G content >50 % and a ratio of observed vs. expected frequency of CpG dinucleotide ≥0.6.Citation57,58 These regions were inferred from the annotation data of the RnBeads package.Citation55 The association was assessed by linear models implemented in the same package and the models were also adjusted for sex, age, batch, and physical activity. We considered as statistically significant those P-values <0.05 adjusted for the false discovery rate (FDR).
The same statistical methods were used in the validation phase, and DNA methylation validation models were adjusted for age, sex, and batch. The analysis of the differences in gene expression were unadjusted because there were no age and sex differences in PREDIMED study participants, and were assessed using the limma package.Citation59 Age- and sex-adjusted differences in protein level between smokers and never smokers were analyzed using ANCOVA.
A logistic regression model considering smoking as the response variable and methylation at the cg05575921 as the explicative variable was used to assess the discriminative capacity of this CpG. We calculated the ROC area under the curve using the epicalc R package.Citation60
Disclosure of Potential Conflicts of Interest
No potential conflicts of interest were disclosed.
Supplemental Material
Supplemental data for this article can be accessed on the publisher's website.
KEPI_S_1115175.doc
Download MS Word (1.1 MB)Acknowledgment
This work was supported by the following sources: Agència de Gestió Ajuts Universitaris de Recerca (2014 SGR 240); the Spanish Ministry of Economy through the Carlos III Health Institute (ISCIII-FIS-FEDER-ERDF PI11–01801, PI08–1327, PI05–1251, PI05–1297, PI02–0471, FIS99/0013–01, FIS96/0026–01, FIS93/0568, FIS92/0009–05), and the Red de Investigación Cardiovascular (RD12/0042/0013, RD12/0042/0020, RD12/0042/0040 and RD12/0042/0061). The BAsicMAR study was funded by the Spanish Ministry of Economy through the Carlos III Health Institute (ISCIII-FIS-FEDER-ERDF) PI12/01238 and RecerCaixa JJ086116. Sergi Sayols-Baixeras was funded by a contract from Instituto de Salud Carlos III FEDER (IFI14/00007).
References
- Lim SS, Vos T, Flaxman AD, Danaei G, Shibuya K, Adair-Rohani H, Amann M, Anderson HR, Andrews KG, Aryee M, et al. A comparative risk assessment of burden of disease and injury attributable to 67 risk factors and risk factor clusters in 21 regions, 1990-2010: a systematic analysis for the Global Burden of Disease Study 2010. Lancet 2013; 380:2224–60; http://dx.doi.org/10.1016/S0140-6736(12)61766-8
- Carter BD, Abnet CC, Feskanich D, Freedman ND, Hartge P, Lewis CE, Ockene JK, Prentice RL, Speizer FE, Thun MJ, et al. Smoking and mortality: beyond established causes. N Engl J Med 2015; 372:631–40; PMID:25671255; http://dx.doi.org/10.1056/NEJMsa1407211
- Messner B, Bernhard D. Smoking and cardiovascular disease: mechanisms of endothelial dysfunction and early atherogenesis. Arterioscler Thromb Vasc Biol 2014; 34:509–15; PMID:24554606; http://dx.doi.org/10.1161/ATVBAHA.113.300156
- Besaratinia A, Tommasi S. Genotoxicity of tobacco smoke-derived aromatic amines and bladder cancer: current state of knowledge and future research directions. FASEB J 2013; 27:2090–100; PMID:23449930; http://dx.doi.org/10.1096/fj.12-227074
- D’Alessandro A, Boeckelmann I, Hammwhoner M, Goette A. Nicotine, cigarette smoking and cardiac arrhythmia: an overview. Eur J Prev Cardiol 2012; 19:297–305; http://dx.doi.org/10.1177/1741826711411738
- Hecht SS. Lung carcinogenesis by tobacco smoke. Int J Cancer 2012; 131:2724–32; PMID:22945513; http://dx.doi.org/10.1002/ijc.27816
- Takahashi H, Ogata H, Nishigaki R, Broide DH, Karin M. Tobacco smoke promotes lung tumorigenesis by triggering IKKbeta- and JNK1-dependent inflammation. Cancer Cell 2010; 17:89–97; PMID:20129250; http://dx.doi.org/10.1016/j.ccr.2009.12.008
- Huxley R. Risk factors: Smoking and CAD-what's plaque got to do with it? Nat Rev Cardiol 2015; 12:265–6; PMID:25824517; http://dx.doi.org/10.1038/nrcardio.2015.37
- Portela A, Esteller M. Epigenetic modifications and human disease. Nat Biotechnol 2010; 28:1057–68; PMID:20944598; http://dx.doi.org/10.1038/nbt.1685
- Lee K, Park J, Lee K, Richardson LE, Johnson BH, Lee H, Lee J, Kim S, Song KS, Kim YS, et al. nc886 , a non-coding RNA of anti-proliferative role , is suppressed by CpG DNA methylation in human gastric cancer. Oncotarget 2008; 5:3944–55; http://dx.doi.org/10.18632/oncotarget.2047
- Breitling LP, Yang R, Korn B, Burwinkel B, Brenner H. Tobacco-smoking-related differential DNA methylation: 27K discovery and replication. Am J Hum Genet 2011; 88:450–7; PMID:21457905; http://dx.doi.org/10.1016/j.ajhg.2011.03.003
- Joubert BR, Håberg SE, Nilsen RM, Wang X, Vollset SE, Murphy SK, Huang Z, Hoyo C, Midttun Ø, Cupul-Uicab L a., et al. 450K epigenome-wide scan identifies differential DNA methylation in newborns related to maternal smoking during pregnancy. Environ Health Perspect 2012; 120:1425–31; PMID:22851337; http://dx.doi.org/10.1289/ehp.1205412
- Zeilinger S, Kuhnel B, Klopp N, Baurecht H, Kleinschmidt A, Gieger C, Weidinger S, Lattka E, Adamski J, Peters A, et al. Tobacco smoking leads to extensive genome-wide changes in DNA methylation. PLoS One 2013; 8:e63812; PMID:23691101; http://dx.doi.org/10.1371/journal.pone.0063812
- Shenker NS, Polidoro S, van VK, Sacerdote C, Ricceri F, Birrell MA, Belvisi MG, Brown R, Vineis P, Flanagan JM. Epigenome-wide association study in the European Prospective Investigation into Cancer and Nutrition (EPIC-Turin) identifies novel genetic loci associated with smoking. Hum Mol Genet 2013; 22:843–51; PMID:23175441; http://dx.doi.org/10.1093/hmg/dds488
- Besingi W, Johansson Å. Smoke-related DNA methylation changes in the etiology of human disease. Hum Mol Genet 2014; 23:2290–7; PMID:24334605; http://dx.doi.org/10.1093/hmg/ddt621
- Harlid S, Xu Z, Panduri V, Sandler DP, Taylor J A. CpG sites associated with cigarette smoking: Analysis of epigenome-wide data from the sister study. Environ Health Perspect 2014; 122:673–8; PMID:24704585
- Tsaprouni LG, Yang TP, Bell J, Dick KJ, Kanoni S, Nisbet J, Vinuela A, Grundberg E, Nelson CP, Meduri E, et al. Cigarette smoking reduces DNA methylation levels at multiple genomic loci but the effect is partially reversible upon cessation. Epigenetics 2014; 9:1382–96; PMID:25424692; http://dx.doi.org/10.4161/15592294.2014.969637
- Bock C. Analysing and interpreting DNA methylation data. Nat Rev Genet 2012; 13:705–19; PMID:22986265; http://dx.doi.org/10.1038/nrg3273
- Guay SP, Brisson D, Lamarche B, Gaudet D, Bouchard L. Epipolymorphisms within lipoprotein genes contribute independently to plasma lipid levels in familial hypercholesterolemia. Epigenetics 2014; 9:718–29; PMID:24504152; http://dx.doi.org/10.4161/epi.27981
- Breitling LP, Salzmann K, Rothenbacher D, Burwinkel B, Brenner H. Smoking, F2RL3 methylation, and prognosis in stable coronary heart disease. Eur Heart J 2012; 33:2841–8; PMID:22511653; http://dx.doi.org/10.1093/eurheartj/ehs091
- Philibert RA, Beach SR, Lei MK, Brody GH. Changes in DNA methylation at the aryl hydrocarbon receptor repressor may be a new biomarker for smoking. Clin Epigenetics 2013; 5:19; PMID:24120260; http://dx.doi.org/10.1186/1868-7083-5-19
- Guida F, Sandanger TM, Castagne R, Campanella G, Polidoro S, Palli D, Krogh V, Tumino R, Sacerdote C, Panico S, et al. Dynamics of Smoking-Induced Genome-Wide Methylation Changes with Time Since Smoking Cessation. Hum Mol Genet 2015; 24:2349–59; PMID:25556184; http://dx.doi.org/10.1093/hmg/ddu751
- Philibert R, Hollenbeck N, Andersen E, Osborn T, Gerrard M, Gibbons FX, Wang K. A quantitative epigenetic approach for the assessment of cigarette consumption. Front Psychol 2015; 6:1–8; PMID:25688217; http://dx.doi.org/10.3389/fpsyg.2015.00656
- Ono Y, Torii K, Fritsche E, Shintani Y, Nishida E, Nakamura M, Shirakata Y, Haarmann-Stemmann T, Abel J, Krutmann J, et al. Role of the aryl hydrocarbon receptor in tobacco smoke extract-induced matrix metalloproteinase-1 expression. Exp Dermatol 2013; 22:349–53; PMID:23614742; http://dx.doi.org/10.1111/exd.12148
- D’Agostino M, Tornillo G, Caporaso MG, Barone M V, Ghigo E, Bonatti S, Mottola G. Ligand of Numb proteins LNX1p80 and LNX2 interact with the human glycoprotein CD8 and promote its ubiquitylation and endocytosis. J Cell Sci 2011; 124:3545–56; http://dx.doi.org/10.1242/jcs.081224
- Zhou J, Fujiwara T, Ye S, Li X, Zhao H. Ubiquitin E3 Ligase LNX2 is Critical for Osteoclastogenesis In Vitro by Regulating M-CSF/RANKL Signaling and Notch2. Calcif. Tissue Int 2015; 96:465–75; http://dx.doi.org/10.1007/s00223-015-9967-7
- Krishna SM, Golledge J. Review: The role of thrombospondin-1 in cardiovascular health and pathology. Int J Cardiol 2013; 168:692–706; PMID:23664438; http://dx.doi.org/10.1016/j.ijcard.2013.04.139
- Frangogiannis NG, Ren G, Dewald O, Zymek P, Haudek S, Koerting A, Winkelmann K, Michael LH, Lawler J, Entman ML. Critical role of endogenous thrombospondin-1 in preventing expansion of healing myocardial infarcts. Circulation 2005; 111:2935–42; PMID:15927970; http://dx.doi.org/10.1161/CIRCULATIONAHA.104.510354
- Liu A, Mosher DF, Murphy-Ullrich JE, Goldblum SE. The counteradhesive proteins, thrombospondin 1 and SPARC/osteonectin, open the tyrosine phosphorylation-responsive paracellular pathway in pulmonary vascular endothelia. Microvasc Res 2009; 77:13–20; PMID:18952113; http://dx.doi.org/10.1016/j.mvr.2008.08.008
- Choi KY, Kim DB, Kim MJ, Kwon BJ, Chang SY, Jang SW, Cho EJ, Rho TH, Kim JH. Higher Plasma Thrombospondin-1 Levels in Patients With Coronary Artery Disease and Diabetes Mellitus. Korean Circ J 2012; 42:100–6; PMID:22396697; http://dx.doi.org/10.4070/kcj.2012.42.2.100
- Smadja DM, d’Audigier C, Bieche I, Evrard S, Mauge L, Dias JV, Labreuche J, Laurendeau I, Marsac B, Dizier B, et al. Thrombospondin-1 is a plasmatic marker of peripheral arterial disease that modulates endothelial progenitor cell angiogenic properties. Arterioscler Thromb Vasc Biol 2011; 31:551–9; PMID:21148423; http://dx.doi.org/10.1161/ATVBAHA.110.220624
- Koch W, Hoppmann P, De waha A, Schömig A, Kastrati A. Polymorphisms in thrombospondin genes and myocardial infarction: A case-control study and a meta-analysis of available evidence. Hum Mol Genet 2008; 17:1120–6; PMID:18178577; http://dx.doi.org/10.1093/hmg/ddn001
- Lei R, Tang J, Zhuang X, Deng R, Li G, Yu J, Liang Y, Xiao J, Wang HY, Yang Q, et al. Suppression of MIM by microRNA-182 activates RhoA and promotes breast cancer metastasis. Oncogene 2014; 33:1287–96; PMID:23474751; http://dx.doi.org/10.1038/onc.2013.65
- Bell JT, Pai A, Pickrell JK, Gaffney DJ, Pique-Regi R, Degner JF, Gilad Y, Pritchard JK. DNA methylation patterns associate with genetic and gene expression variation in HapMap cell lines. Genome Biol 2011; 12:R10; PMID:21251332; http://dx.doi.org/10.1186/gb-2011-12-1-r10
- Ball MP, Li JB, Gao Y, Lee JH, LeProust EM, Park IH, Xie B, Daley GQ, Church GM. Targeted and genome-scale strategies reveal gene-body methylation signatures in human cells. Nat Biotechnol 2009; 27:361–8; PMID:19329998; http://dx.doi.org/10.1038/nbt.1533
- Lister R, Pelizzola M, Dowen RH, Hawkins RD, Hon G, Tonti-Filippini J, Nery JR, Lee L, Ye Z, Ngo QM, et al. Human DNA methylomes at base resolution show widespread epigenomic differences. Nature 2009; 462:315–22; PMID:19829295; http://dx.doi.org/10.1038/nature08514
- Wan ES, Qiu W, Baccarelli A, Carey VJ, Bacherman H, Rennard SI, Agusti A, Anderson W, Lomas DA, Demeo DL. Cigarette smoking behaviors and time since quitting are associated with differential DNA methylation across the human genome. Hum Mol Genet 2009; 21:3073–82; http://dx.doi.org/10.1093/hmg/dds135
- Higuchi C, Nakatsuka A, Eguchi J, Teshigawara S, Kanzaki M, Katayama A, Yamaguchi S, Takahashi N, Murakami K, Ogawa D, et al. Identification of Circulating miR-101, miR-375 and miR-802 as Biomarkers for Type 2 Diabetes. Metabolism 2015; 64:489–97; PMID:25726255; http://dx.doi.org/10.1016/j.metabol.2014.12.003
- Kornfeld JW, Baitzel C, Konner AC, Nicholls HT, Vogt MC, Herrmanns K, Scheja L, Haumaitre C, Wolf AM, Knippschild U, et al. Obesity-induced overexpression of miR-802 impairs glucose metabolism through silencing of Hnf1b. Nature 2013; 494:111–5; PMID:23389544; http://dx.doi.org/10.1038/nature11793
- Patrick DL, Cheadle A, Thompson DC, Diehr P, Koepsell T, Kinne S. The validity of self-reported smoking: a review and meta-analysis. Am J Public Heal 1994; 84:1086–93; http://dx.doi.org/10.2105/AJPH.84.7.1086
- Lowe R, Slodkowicz G, Goldman N, Rakyan VK. The human blood DNA methylome displays a highly distinctive profile compared with other somatic tissues. Epigenetics 2015; 10:274–81; PMID:25634226; http://dx.doi.org/10.1080/15592294.2014.1003744
- Grau M, Subirana I, Elosua R, Solanas P, Ramos R, Masiá R, Cordón F, Sala J, Juvinyà D, Cerezo C, et al. Trends in cardiovascular risk factor prevalence (1995-2000-2005) in northeastern Spain. Eur J Cardiovasc Prev Rehabil 2007; 14:653–9; PMID:17925624; http://dx.doi.org/10.1097/HJR.0b013e3281764429
- Bibikova M, Barnes B, Tsan C, Ho V, Klotzle B, Le JM, Delano D, Zhang L, Schroth GP, Gunderson KL, et al. High density DNA methylation array with single CpG site resolution. Genomics 2011; 98:288–95; PMID:21839163; http://dx.doi.org/10.1016/j.ygeno.2011.07.007
- Du P, Zhang X, Huang C-C, Jafari N, Kibbe W A, Hou L, Lin SM. Comparison of Beta-value and M-value methods for quantifying methylation levels by microarray analysis. BMC Bioinformatics 2010; 11:587; PMID:21118553; http://dx.doi.org/10.1186/1471-2105-11-587
- Dedeurwaerder S, Defrance M, Bizet M, Calonne E, Bontempi G, Fuks F. A comprehensive overview of Infinium HumanMethylation450 data processing. Brief Bioinform 2014; 15:929–41; PMID:23990268; http://dx.doi.org/10.1093/bib/bbt054
- Rosenbloom KR, Armstrong J, Barber GP, Casper J, Clawson H, Diekhans M, Dreszer TR, Fujita P A, Guruvadoo L, Haeussler M, et al. The UCSC Genome Browser database: 2015 update. Nucleic Acids Res 2015; 43:D670–81; PMID:25428374; http://dx.doi.org/10.1093/nar/gku1177
- Soriano-Tarraga C, Jimenez-Conde J, Giralt-Steinhauer E, Mola M, Ois A, Rodriguez-Campello A, Cuadrado-Godia E, Fernandez-Cadenas I, Carrera C, Montaner J, et al. Global DNA methylation of ischemic stroke subtypes. PLoS One 2014; 9:e96543; PMID:24788121; http://dx.doi.org/10.1371/journal.pone.0096543
- Estruch R, Ros E, Salas-Salvadó J, Covas M-I, Corella D, Arós F, Gómez-Gracia E, Ruiz-Gutiérrez V, Fiol M, Lapetra J, et al. Primary prevention of cardiovascular disease with a Mediterranean diet. N Engl J Med 2013; 368:1279–90; PMID:23432189; http://dx.doi.org/10.1056/NEJMoa1200303
- Castaner O, Corella D, Covas MI, Sorli J V, Subirana I, Flores-Mateo G, Nonell L, Bullo M, de la Torre R, Portoles O, et al. In vivo transcriptomic profile after a Mediterranean diet in high-cardiovascular risk patients: a randomized controlled trial. Am J Clin Nutr 2013; 98:845–53; PMID:23902780; http://dx.doi.org/10.3945/ajcn.113.060582
- Irizarry RA, Hobbs B, Collin F, Beazer-Barclay YD, Antonellis KJ, Scherf U, Speed TP. Exploration, normalization, and summaries of high density oligonucleotide array probe level data. Biostatistics 2003; 4:249–64; PMID:12925520; http://dx.doi.org/10.1093/biostatistics/4.2.249
- Marrugat J, Subirana I, Ramos R, Vila J, Marin-Ibanez A, Guembe MJ, Rigo F, Tormo Diaz MJ, Moreno-Iribas C, Cabre JJ, et al. Derivation and validation of a set of 10-year cardiovascular risk predictive functions in Spain: the FRESCO Study Prev Med 2014; 61:66–74; PMID:24412897; http://dx.doi.org/10.1016/j.ypmed.2013.12.031
- Lopez-Farre AJ, Zamorano-Leon JJ, Segura A, Mateos-Caceres PJ, Modrego J, Rodriguez-Sierra P, Calatrava L, Tamargo J, Macaya C. Plasma desmoplakin I biomarker of vascular recurrence after ischemic stroke. J Neurochem 2012; 121:314–25; PMID:22304020; http://dx.doi.org/10.1111/j.1471-4159.2012.07683.x
- Houseman EA, Accomando WP, Koestler DC, Christensen BC, Marsit CJ, Nelson HH, Wiencke JK, Kelsey KT. DNA methylation arrays as surrogate measures of cell mixture distribution. BMC Bioinformatics 2012; 13:86; PMID:22568884; http://dx.doi.org/10.1186/1471-2105-13-86
- Aryee MJ, Jaffe AE, Corrada-Bravo H, Ladd-Acosta C, Feinberg AP, Hansen KD, Irizarry RA. Minfi: A flexible and comprehensive Bioconductor package for the analysis of Infinium DNA methylation microarrays. Bioinformatics 2014; 30:1363–9; PMID:24478339; http://dx.doi.org/10.1093/bioinformatics/btu049
- Assenov Y, Muller F, Lutsik P, Walter J, Lengauer T, Bock C. Comprehensive Analysis of DNA Methylation Data with RnBeads. Nat. Methods 2014; 11:1138–40
- Zaina S, Heyn H, Carmona FJ, Varol N, Sayols S, Condom E, Ramirez-Ruz J, Gomez A, Goncalves I, Moran S, et al. DNA methylation map of human atherosclerosis. Circ Cardiovasc Genet 2014; 7:692–700; PMID:25091541; http://dx.doi.org/10.1161/CIRCGENETICS.113.000441
- Larsen F, Gundersen G, Lopez R, Prydz H. CpG islands as gene markers in the human genome. Genomics 1992; 13:1095–107; PMID:1505946; http://dx.doi.org/10.1016/0888-7543(92)90024-M
- Gardiner-Garden M, Frommer M. CpG islands in vertebrate genomes. J Mol Biol 1987; 196:261–82; PMID:3656447; http://dx.doi.org/10.1016/0022-2836(87)90689-9
- Ritchie ME, Phipson B, Wu D, Hu Y, Law CW, Shi W, Smyth GK. Limma powers differential expression analyses for RNA-sequencing and microarray studies. Nucleic Acids Res 2015; 43:e47; PMID:25605792; http://dx.doi.org/10.1093/nar/gkv007
- Chongsuvivatwong V. epicalc: Epidemiological calculator. R package version 2.15.1.0. 2012