Abstract
Breast cancer is more common in European Americans (EAs) than in African Americans (AAs) but mortality from breast cancer is higher among AAs. While there are racial differences in DNA methylation and gene expression in breast tumors, little is known whether such racial differences exist in breast tissues of healthy women. Genome-wide DNA methylation and gene expression profiling was performed in histologically normal breast tissues of healthy women. Linear regression models were used to identify differentially-methylated CpG sites (CpGs) between EAs (n = 61) and AAs (n = 22). Correlations for methylation and expression were assessed. Biological functions of the differentially-methylated genes were assigned using the Ingenuity Pathway Analysis. Among 485 differentially-methylated CpGs by race, 203 were hypermethylated in EAs, and 282 were hypermethylated in AAs. Promoter-related differentially-methylated CpGs were more frequently hypermethylated in EAs (52%) than AAs (27%) while gene body and intergenic CpGs were more frequently hypermethylated in AAs. The differentially-methylated CpGs were enriched for cancer-associated genes with roles in cell death and survival, cellular development, and cell-to-cell signaling. In a separate analysis for correlation in EAs and AAs, different patterns of correlation were found between EAs and AAs. The correlated genes showed different biological networks between EAs and AAs; networks were connected by Ubiquitin C. To our knowledge, this is the first comprehensive genome-wide study to identify differences in methylation and gene expression between EAs and AAs in breast tissues from healthy women. These findings may provide further insights regarding the contribution of epigenetic differences to racial disparities in breast cancer.
Abbreviations
5hmC | = | 5-hydroxymethylcytosine |
AAs | = | African Americans |
bps | = | base pairs |
CpGs | = | CpG sites |
DM | = | differentially-methylated |
EAs | = | European Americans |
HM450 | = | HumanMethylation450 BeadChips |
PCA | = | Principal Component Analysis |
QCs | = | quality controls |
TCGA | = | The Cancer Genome Atlas |
TSS | = | transcription start site |
TSG | = | tumor suppressor genes |
UBC | = | Ubiquitin C |
UTR | = | untranslated regions |
Introduction
Breast cancer is a complex and heterogeneous disease at the molecular level, characterized by complex patterns of genetic and epigenetic alterations. In the United States, breast cancer incidence rates are higher in European-Americans (EAs) relative to African-Americans (AAs) Citation1; however, AAs are at greater risk of aggressive phenotypes and higher breast cancer mortality (http://www.cancer.gov/about-nci/organization/crchd/cancer-health-disparities-fact-sheet) . There are documented differences in molecular markers in tumors for EAs compared to AAs, possibly due to differences in breast cancer etiology between races.Citation2
Epigenetic markers of DNA methylation are among such important differences in tumors for EAs and AAs.Citation2,3 Hypermethylation of DNA in promoter regions of tumor suppressor genes (TSG) such as p16, ER-α, PR, BRCA1, GSTP1, TIMP-4, and CDH1 is known to be a major event in breast carcinogenesis.Citation4 Several breast cancer risk factors,Citation5-8 including race,Citation2,9 have been reported to increase the risk of altered DNA methylation in breast tumors.
Hypermethylation is hypothesized to be an early event that occurs in healthy women many years before clinically detectable breast cancer Citation10; however, little is known about what factors increase the risk of these events. There are data that suggest that low breast folate and alcohol consumption are associated with p16 gene hypermethylation Citation11; genetic variation in genes for one-carbon metabolism may affect global methylation in normal tissues from healthy individuals.Citation12 To date, however, few studies have examined differential methylation by race in histologically normal tissues.Citation13-15 Among them, analyses have been limited to global methylation and to blood specimens.Citation13,14 In one study to examine genome-wide methylation differences by race, Adkins et al. reported 3,623 loci in DNA significant differentially methylated between AAs and EAs at birth using umbilical cord blood.Citation15 However, to our knowledge, no study has comprehensively examined differences in methylation by race of genome-wide DNA methylation in normal breast tissues.
Similar to DNA methylation, differential gene expression in breast tumors is well-studied. Several researchers have reported differential expression in breast tumors compared to adjacent normal tissues, and gene expression profiling has been used to classify molecular subtypes of disease.Citation16 Several studies have reported associations between gene expression and breast cancer risk factors in both tumor and normal breast tissues.Citation17-20 Authors reported differences in gene expression by age,Citation17 body mass index,Citation18 parity,Citation19 and breast density.Citation20 There is less known about factors affecting gene expression in normal tissues from healthy individuals. In particular, little is known about associations of gene expression with race in breast tissues of women without breast cancer.
The study of normal tissues is distinguished from breast tumor analysis because it provides insights into breast cancer mechanisms unperturbed by major genomic changes and early breast carcinogenesis. Here, we aimed to assess differences by race for genome-wide DNA methylation and gene expression in healthy women with no prior history of breast cancer. Although there are studies of gene methylation and expression in breast cancer, ours is the first study to provide comprehensive genome-wide analysis in histologically-confirmed normal breast tissues.
Materials and Methods
Study subjects and tissue collection
Detailed study methods for subject accrual have been described elsewhere.Citation10,21 Briefly, women who underwent reduction mammoplasty at Georgetown University Medical Center (Washington, DC), the University of Maryland (College Park, MD), the Washington Hospital Center (Washington, DC) and the Center for Plastic Surgery (Buffalo, NY) between 1997 and 2009 were included in this study. All women in the study gave written informed consent and the Institutional Review Boards at all the participating institutions approved this study. Recorded data by personal interview included demographics, lifestyle, reproductive, and family medical history, diet, medication use, and other exposures. Race was determined by self-report. Breast tissues were surgically removed, evaluated and determined to be free from gross and microscopic histopathological abnormalities by a certified pathologist. Tissues were blunt-dissected to remove adipose tissue, part of the tissue being rapidly frozen in Liquid Nitrogen and part of it being submersed in RNA later® (Ambion, Inc., Austin, Texas) and stored at −80°C.
Methylation analysis
DNA was extracted from dissected frozen fresh breast tissue using a MasterPure™ DNA purification kit (Epicenter, Madison, WI) according to the manufacturer's specifications. Following bisulfite treatment of DNA using the EZ DNA Methylation kit (Zymo Research, Irvine, CA), the bisulfite-converted DNA was hybridized onto the HumanMethylation450 BeadChips (Illumina, San Diego, CA) (HM450), following the Illumina Infinium HD Methylation protocol in the Genomics Core Facility at Roswell Park Cancer Institute (Buffalo, NY).Citation2 Briefly, this consisted of a whole genome amplification step followed by enzymatic end-point fragmentation, precipitation and resuspension. The Illumina iScan SQ scanner was used to create images of the single arrays and the intensities of the images were extracted using GenomeStudio (v.2011.1) Methylation module (v.1.9.0) software. About 20% of samples (16/83) were duplicated as internal quality controls (QCs). The scatter plot with the linear regression line of multiple QC experiments showed a highly significant correlation (r=0.99) between duplicate signal intensities in the arrays.
Probe quality of Infinium HumanMethylation450 BeadChip
Raw intensity data as IDAT files were imported into Partek Genomics Suite™ 6.6 (Partek Inc., St. Louis, MO). The data was normalized using the Subset-quantile Within Array Normalization (SWAN).Citation22 Any probes with detection P > 0.05 were filtered out before further analysis (n = 4,242). Sex-chromosome-linked probes (n = 11,650) were also removed. We further excluded probes including SNPs in the target CpG (n = 115,996) or within 10 base pairs (bps) of the target CpGs (n = 145,116) based on NCBI dbSNP build 138 to avoid any potential effect of SNPs. In addition, problematic probes (non-specific probes) described in 2 recent studies to hybridize to multiple genomic locations by in silico analysis were excluded Citation23,24 in order to remove potential bias measurements of DNA methylation. GRCh37/hg19 (Human Genome version 19) was used as reference genome for this study. The final dataset contained 247,456 CpGs across 83 samples (61 EAs and 22 AAs).
Locus-by-locus analysis to identify differential methylation between AAs and EAs
Partek Genomics Suite™ 6.6 (Partek) was used for the statistical analysis of differential methylation and visualization unless otherwise specified. β-values ranging from 0 (100% unmethylated) to 1 (100% methylated) were determined for of the methylated probe intensity and total probe intensities. For the statistical modeling purpose, β values were converted to M-values by logit-transformation (log2 ratio of methylated probe intensity and unmethylated probe intensity). It is because β-values have severe heteroscedasticity for highly methylated or unmethylated CpG sites.Citation25 For identification of patterns in DNA methylation, unsupervised analysis including unsupervised hierarchical clustering Citation26 and Principal Component Analysis (PCA) Citation27 were performed. For heatmaps, the Euclidian distance between the 2 groups of samples (EAs and AAs) was calculated by the average linkage.
To characterize the methylation patterns, the DM CpGs were divided by functional roles according to their genomic locations such as promoter: within 1500 bps of a transcription start site (TSS) (TSS1500); within 200 bps of a TSS (TSS200); 5' untranslated regions (5'UTR); first exon (1stExon); body (non-promoter); 3'UTR (non-promoter); and intergenic regions.Citation28
Human transcriptome array
Total RNA was extracted directly from frozen tissue stored in RNAlater (Ambion) using 1.5 mm Triple-Pure Zirconium Beads (Benchmark Scientific, Edison, NJ) and RNeasy Plus Mini Kit (Qiagen, Valencia, CA), according to the manufacturer's protocol. The gene expression microarray was performed on GeneChip® Human Transcriptome Array 2.0 (Affymetrix Inc., Santa Clara, CA) by the Nucleic Acid Shared Resource at The Ohio State University. Because some samples did not pass quality checks, we were only able to analyze 75 samples (56 EAs and 19 AAs) among the 83 samples which were also analyzed by HM450. The arrays were scanned on an Affymetrix GeneChip scanner 3000 using the AGCC Software (Affymetrix GeneChip® Command Console®). The CEL files generated by the arrays were imported into the Affymetrix Expression Console® Software (version 1.3.1) for log2 transformation and quantile normalization. 10 percent of samples were duplicated as QCs. The scatter plot and linear regression of multiple QC experiments showed a highly significant correlation (r = 0.99) between duplicate signal intensities in the arrays.
Correlation analysis between methylation and gene expression
Spearman correlation coefficients were used to examine the association between the DM CpGs (M-value) from the methylation array and matched gene expression (log2 transformed intensity) based on genomic location of probes provided by annotation files from the HM450 and Affymetrix using Partek Genomics Suite™ 6.6 (Partek). We first matched the exact gene names for statistically significant 485 DM CpGs (FDR q < 0.05) from the HM450 (Illumina) and mRNA expression (Affymetrix) arrays. The CpGs located up to 1500 bps upstream of the gene as well as gene body and 3'UTR were included in the analysis. Spearman correlation P < 0.05 were considered statistically significant.
Statistical methods
Tests for independence comparing participants' characteristics and race were tested using chi-square or the Mann-Whitney rank test for categorical and continuous variables, respectively.
A generalized linear regression model was used to identify the DM CpGs by race after adjusting for BMI and age; these three variables, but not smoking status, alcohol use, age at menarche, age at first live birth contributed to the variance in data (Data not shown). Multiple testing correction was performed using the Benjamini and Hochberg False Discovery Rate (FDR) with significantly differential methylation defined at corrected P < 0.05 which is corresponding to raw P < 1.35 × 10−4. Differential methylation linked to CpG islands (UCSC genome browser Citation29), shore (0-2 kb from CpG island), or shelves (2-4 kb from CpG island) by functional location between EAs and AAs: We defined hypermethylated CpGs as the average methylation levels if the CpGs of EAs were higher than AAs, respectively and vice versa.
Welch's t-test was performed to test differential gene expression between EAs and AAs. A P < 0.05 was considered statistically significant.
To examine if differences by race in correlations existed between methylation and gene expression, interaction analysis after adjusting for age and BMI was performed using JMP® v10 (JMP, Cary, NC). A cross-product term for race − CpG methylation was included in adjusted correlation models.
Ingenuity pathway analysis
The genes corresponding to promoter CpGs among the significant DM CpGs were analyzed for their potential biological implications using Ingenuity Pathway Analysis (IPA). Also, the DM genes that were significantly correlated between methylation and gene expression were separately analyzed for networks. The genes were sorted based on reported or suggested biological and molecular functions and diseases in IPA. The identified genes were mapped to networks available in the IPA and then ranked by score. The score [score= −log10(P-value)] computed by IPA is according to the probability of the set of identified genes and a list of biological functions stored in the IPA knowledge base (IPKB). A score >3 represents a corresponding P <0.0001 that a gene network was not generated by chance alone.
Validation of differentially-methylated CpGs by Pyrosequencing
Validation of HM450 results was performed using Pyrosequencing of the specific CpGs. Bisulfite conversion was performed using EZ DNA Methylation Gold Kit (Zymo research, Orange, CA) according to the manufacturer's instructions. Two primer sets were designed to amplify each locus interrogated on the array for each target. Primer sequences can be found in Table S1. PCR was performed in 20 μl volume reactions containing 2 μl (about 20-25 ng) of converted DNA, 0.2 μM primers, and AmpliTaq Gold PCR kit (Applied Biosystems, Branchburg, NJ). PCR amplicons were processed for Pyrosequencing analysis according to the manufacturer's standard protocol (Qiagen, Valencia, CA) using a PyroMark MD system (Qiagen) with Pyro Q-CpG 1.0.9 software (Qiagen) for CpG methylation quantification. A total of 80 EAs and 40 AAs samples were used for validation, which include (1) 57 EAs and 22 AAs fresh frozen breast tissues that were analyzed by HM450 (defined as technical validation) and (2) 23 EAs and 18 AAs fresh frozen breast tissues that were not analyzed by HM450 (defined as biological validation).
Results
Participants' characteristics
Characteristics of study participants are shown in . Biospecimens from 83 (61 EAs and 22 AAs) and 41 (23 EAs and 18 AAs) study participants were available for HM450 and Pyrosequencing validation of differentially-methylated CpGs, respectively. Among biospecimens included in HM450 analysis, the mean age for EAs and AAs was 40.7 (SD ±13.3) and 34.4 (SD ±7.5), respectively. Among biospecimens included in Pyrosequencing, the median age for EAs and AAs was 37.5 (SD ±14.9) and 37.61 (SD ±8.9), respectively. Between EAs and AAs, BMI (P =1.04x10−6) differed most significantly for HM450. Alcohol status (P = 0.01) was significantly different between the groups for the Pyrosequencing validation set.
Table 1. Characteristics of study participants.
Identification of differentially-methylated CpGs by race
There were 485 CpGs differentially-methylated (DM) between EAs and AAs at a FDR q <0.05. The magnitude of differential methylation levels (delta-β, x-axis) against raw P values (y-axis) are shown in a Volcano plot for all 247,456 CpGs (). Among the 485 DM CpGs, 11% (52/485) had ≥10% methylation differences between the races (Delta-β values ≥|0.1|), while the majority (89%) of the DM CpGs presented relatively small differences (Delta-β values ≤|0.1|) (). The Manhattan plot in indicates that the DM CpGs are spread across all autosomes. Of the 485 DM CpGs, 348 DM CpGs (71.8%) were linked to 182 specific genes (on average 1.9 CpGs/gene). Among these genes, 30 had more than >1 DM CpG and 10 had ≥3 DM CpGs (Table S2, yellow marked): C3orf24 (6 CpGs in chromosome 3); C6orf27 (3 CpGs in chromosome 6); DIP2C (3 CpGs in chromosome 1); HSP90AA1 (3 CpGs in chromosome 1); KCNK15 (3 CpGs in chromosome 20); OPCML (3 CpGs in chromosome 11); PACS2 (3 CpGs in chromosome 14); PM20D1 (3 CpGs in chromosome 1); RNF219 (3 CpGs in chromosome 13); and SEPT8 (8 CpGs in chromosome 5). C3orf24 and SEPT8 are indicated in Figure 1B.
Figure 1. Differentially-methylated CpGs among 247,456 CpGs. (A) A volcano plot showing differential methylation [delta-β (average β-values of EAs)-(average β-values of AAs)] (x-axis) with corresponding −log10P-value (y-axis) by race after adjusting for age and BMI. Red dots (+ figures on x-axis) indicate hypermethylated CpGs in EAs compared to AAs while blue dots (- figures on x-axis) represent hypermethylated CpGs in AAs relative to EAs. (B) The significance −log10P-value of the associations by chromosomes is shown in in a Manhattan plot. CpGs for C3orf24 (6CpGs) in chromosome 3 and SEPT8 (8 CpGs) in chromosome 5 are marked as red boxes. The genome-wide significance level of 1.35x10−4 is indicated by the horizontal line in both Volcano (A) and Manhattan plots (B). Unsupervised analysis for entire 247,456 CpGs (C and D) and 485 differentially-methylated CpGs (E and F) are shown. (C and E) Principal component analysis (PCA) of the methylation data are plotted using the first 3 principal components. Data from EAs are shown in blue and data from AAs are in red. (D and F) Unsupervised hierarchical clustering of β-values for CpGs (rows) in 83 samples (columns). Blue and red blocks on the top of the maps represent 61 EAs and 22 AAs. While purple for the CpGs represents hypermethylation and green for CpGs represent hypomethylation.
![Figure 1. Differentially-methylated CpGs among 247,456 CpGs. (A) A volcano plot showing differential methylation [delta-β (average β-values of EAs)-(average β-values of AAs)] (x-axis) with corresponding −log10P-value (y-axis) by race after adjusting for age and BMI. Red dots (+ figures on x-axis) indicate hypermethylated CpGs in EAs compared to AAs while blue dots (- figures on x-axis) represent hypermethylated CpGs in AAs relative to EAs. (B) The significance −log10P-value of the associations by chromosomes is shown in Figure. 1B in a Manhattan plot. CpGs for C3orf24 (6CpGs) in chromosome 3 and SEPT8 (8 CpGs) in chromosome 5 are marked as red boxes. The genome-wide significance level of 1.35x10−4 is indicated by the horizontal line in both Volcano (A) and Manhattan plots (B). Unsupervised analysis for entire 247,456 CpGs (C and D) and 485 differentially-methylated CpGs (E and F) are shown. (C and E) Principal component analysis (PCA) of the methylation data are plotted using the first 3 principal components. Data from EAs are shown in blue and data from AAs are in red. (D and F) Unsupervised hierarchical clustering of β-values for CpGs (rows) in 83 samples (columns). Blue and red blocks on the top of the maps represent 61 EAs and 22 AAs. While purple for the CpGs represents hypermethylation and green for CpGs represent hypomethylation.](/cms/asset/e471b2af-fea4-41f4-bb7e-e3f4cc028ae4/kepi_a_1121362_f0001_oc.gif)
To determine methylation signal clustering of the samples by race, we performed unsupervised analysis including Principal Component Analysis (PCA) () and hierarchical clustering (). Both PCA and the heatmap resulting from hierarchical clustering analysis showed clear and distinct methylation patterns between EAs and AAs using the 485 DM CpGs identified at FDR q <0.05 () compared to the results using the entire CpGs analyzed (). The first component of variance for the autosomal DM CpGs, accounting for 25.3% of the overall variation in methylation, distinguished samples based on race (). In order to investigate effects of potential confounding factors () in the methylation levels of 485 DM CpGs, PCA was used. The PCA showed that the separation between EAs and AAs using the 485 DM CpGs were not associated with breast cancer risk factors including age at menarche, age at first live birth, alcohol consumption history, breast biopsies, family history of breast cancer, menopausal status, and smoking status (Fig. S1). A full list of the 485 DM CpGs including detailed information such as Probe ID (Target ID), β-values, P values, and genomic location is provided in Table S2.
Characterization of Differentially-Methylated CpGs
Of the 485 DM CpGs identified after correction for multiple comparisons, 203 (42%) of DM CpGs were hypermethylated in EAs compared to AAs and 282 (58%) were hypermethylated in AAs relative to EAs (). Among the hypermethylated CpGs in EAs, 48% were localized in the promoter regions that correspond to regions TSS1500, TSS200, 5'UTR, and 1stExon (, left side). In contrast, the hypermethylated DM CpGs in AAs were mostly localized to gene body (39%), followed by 31% in Intergenic and 27% in promoter regions (, right side). Regardless of functional locations, methylation levels were up to 3.6-fold higher (56%-207% higher β-values) in AAs (range of β-values: 0.58-0.78) compared to the hypermethylated ones in EAs (range of β-values: 0.16-0.56) (). Similarly, in the heatmap in , rows were more consistently purple, corresponding to higher methylation among AAs compared to EAs.
Figure 2. Landscape of differentially methylated CpGs. (A) The percentages of hypermethylated in EAs compared to AAs (Left) and in AAs compared to EAs (Right) are shown. The functional promoters CpGs are separated by a bold line. (B) The methylation levels of hypermethylated CpGs are shown by functional location including TSS1500, TSS200, 5'UTR, 1stExon, Body, and 3'UTR and intergenic in EAs (blue bar) and AAs (red bar).
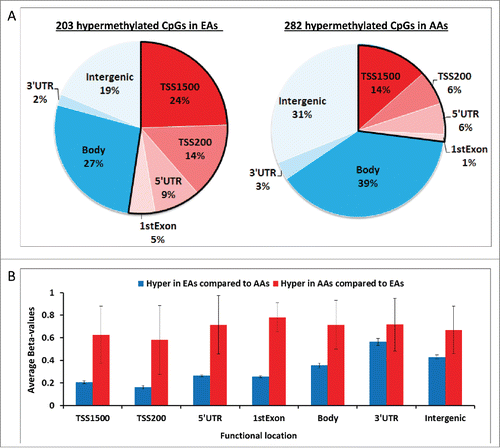
Figure 3. Differential methylation levels stratified by location of interrogated CpGs. Average methylation levels at CpGs within CpG islands and surrounding regions are shown in promoters (A), non-promoters (B), and intergenic regions (C). Differences between EAs and AAs were tested using Unpaired t test with Welch's t-test (**** P<0.0001, *** 0.001 to 0.001, ** 0.001 to 0.01, * 0.01 to 0.05, NS not significant).
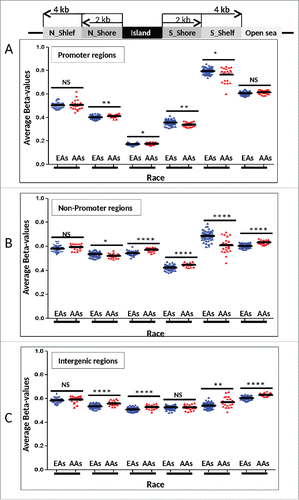
There were 341 (70%) DM CpGs that were linked to CpG islands and surrounding areas as follows, namely N_Shelf (4 kb upstream of CpG island; n = 21), N_Shore (0–2 kb upstream of the CpG islands; n = 72), CpG island (n=160), S_Shore (0–2 kb downstream of the CpG islands; n = 74), S_Shelf (2–4 kb downstream of the CpG islands; n = 14), and Open sea (more than 4kb away from the CpG island; n = 144) locations. They were further divided into 3 categories by functional roles according to their genomic locations such as promoters (n = 177 CpGs; ), non-promoters (n = 193; ), and intergenic regions (n = 137; ). A larger variation was observed in promoters () followed by non-promoters () and relatively consistent methylation levels were found in intergenic regions () in both EAs and AAs. Overall, similar methylation levels were observed between promoters and non-promoters related DM CpGs in both EAs and AAs. However, significantly lower methylation levels were observed in both EAs (67% lower) and AAs (69% lower) among promoter CpG islands compared to non-promoter ones (P <0.0001) (). The DM CpGs in S_Shelf tended to have higher methylation levels with larger variation across the samples in both EAs and AAs, especially in non-promoter regions ().
Figure 4. The top network of genes correlated with methylation in EAs only (A) or AAs only (B). Genes encoding for molecules in red are positively correlated while those in green are negatively correlated between methylation and gene expression. In both networks, the proteins are connected through Ubiquitin C (UBC). The nodal relationships indicated in solid lines denote direct while those in dashed denote indirect interactions. Different shapes represent the functional classes of proteins. The length of a line reflects published evidence supporting the node-to-node relationship concerned. The shapes of each node represent the molecule classes can be found in http://ingenuity.force.com/ipa/IPATutorials?id=kA250000000TN2wCAG.
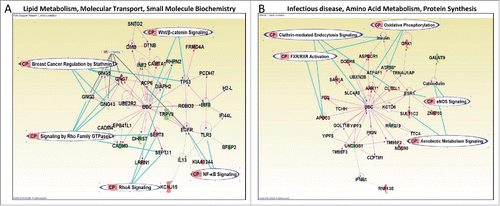
Correlation with DM CpGs and Their Gene Expression Among 485 DM CpGs
The Spearman correlation for each DM CpG and transcript pair was analyzed in EAs and AAs separately. The full list of correlation result is given in Table S3. In EAs and AAs, respectively, 24 pairs (24 CpGs and 22 matched genes) and 26 pairs (26 CpGs and 22 matched genes) were significantly correlated (positively or negatively) between methylation and gene expression at P <0.05. None of the pairs was found in both AAs and EAs (Table S3). While a majority (71%) of the 24 pairs from EAs were negatively correlated, a high proportion of positive correlations (73%) were found among the 26 pairs in AAs (Table S3). The patterns of correlation for 2 genes (DPEP3 and GNG7) found in EAs were significantly different from ones in AAs at P interaction <0.05 (). Among 26 significantly correlated pairs in AAs, the correlation patterns of 9 pairs (ANK1, APOC3, CARS2, FLG, GPX1, PLA2G4C, and RHBDL1) were significantly different from those in EAs at Interaction P <0.05 ( and Fig. S2). The correlation pattern of ANK1 was the most significantly different between the groups (Interaction P = 0.0066).
Table 2. A list of CpGs that are significantly correlated with gene expression levels in AAs or EAs and interaction P-value.
Among the significantly correlated DM genes between gene expression and methylation, 2 genes, GPX1 (Fig. S3, Upper right) and PLA2G4C (Fig. S3, Lower right), were also significantly differentially expressed between the EAs and AAs at P <0.05.
Potential biological roles of DM CpGs
To investigate the possible biological implications of the DM CpGs by race, we focused on 177 of the 485 DM CpGs DM CpGs that were located in promoter regions. These CpGs were co-localized in 181 unique genes (one CpG can reside in more than one transcript variants of the same gene or different genes). IPA revealed enrichment for genes (75%, 136/181) involved in cancer as follows for the top 3 networks (Table S4): (1) Cell Death and Survival, Embryonic Development, Organismal Development (Score = 47); (2) Cellular Compromise, Cell-To-Cell Signaling and Interaction, Hematological System Development and Function (Score = 40); and (3) Cellular Development, Hematological System Development and Function, Hematopoiesis (Score = 34). We also performed IPA on the subset of the genes whose expression levels were significantly correlated with methylation levels in EAs or AAs separately to identify the biological difference or similarity between groups. The top network of functional associations was “Lipid Metabolism, Molecular Transport, Small Molecule Biochemistry” and “Infectious disease, Amino Acid Metabolism, Protein Synthesis” () for the genes that were significantly correlated with methylation levels in EAs and AAs, respectively. Interestingly, 2 networks were connected through Ubiquitin C (UBC) () although the genes involved are different between the networks. The “Lipid Metabolism, Molecular Transport, Small Molecule Biochemistry” network in EAs were characterized by genes that play in Signaling by Rho Family GTPase, Breast cancer regulation by Stathmin1, RhoA Signaling, Wnt/β-catenin Signaling, and NF-kB Signaling (). In contrast, genes involved in Clathrin-mediated endocytosis Signaling, Oxidative phosphorylation, Xenobiotic Metabolism Signaling, eNOS Signaling, and FXR/RXR Activation are essential for the “Infectious disease, Amino Acid Metabolism, Protein Synthesis” network in AAs ().
Technical and biological validation using pyrosequencing
Fifteen DM CpGs were selected based on their involvement in breast cancer or other cancers in general for validation using 12 Pyrosequencing assays. This included 14 DM CpGs harbored by 11 genes (ANK1, ATP5B, CARS2, ESYT3, HIPK2, OPCML, PM20D1, PTH1R, RNF135, TRPV3, and ZNF710) and one (cg06486129) in an intergenic region. For technical validation (57 EAs and 22 AAs subjects), a very strong positive correlation was found (Spearman correlation r=0.96, P <1.0x10−45), showing that all DM CpGs were technically validated (). The highest concordance between the 2 methods (r = 0.89, P = 1.36x10−11) was observed for cg07167872 (PM20D1), which had the biggest difference in methylation level between EAs (average β-values: 0.37) and AAs (average β-values: 0.61) in HM450 (Table S2). Six out of 14 CpGs (CARS2, HIPK2, PTH1R, RNF135, TRPV3, and ZNF710) were also biologically validated in 41 samples (23 EAs and 18 AAs) to be differentially-methylated by race at P <0.05 (Table S5). All 14 DM CpGs were significantly different between EAs and AAs in pooled samples (80 EAs and 40 AA) that included the initial sample set (57 EAs and 22 AAs) and the independent sample set (23 EAs and 18 AAs) (Table S5).
Figure 5. Validation results of 15 differentially-methylated CpGs by Pyrosequencing. Gene name, TargetID (probe ID) by Illumina, correlation efficient (r) and p-value are presented. β-values for each individual samples are presented by different colored dots by different assays. The x-axis and y-axis indicates the β-value from the Illumina HumanMethylation450 BeadChip and % of methylation by Pyrosequencing, respectively.
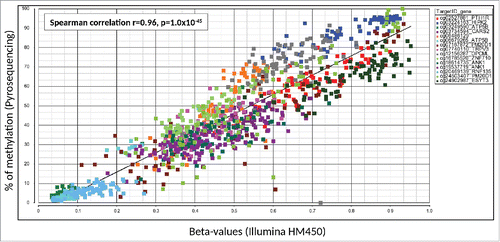
Discussion
DNA methylation has been shown to be a molecular epigenetic marker that differs in breast tumors among EAs and AAs.Citation2,3 There is little information about when the differences occur in the carcinogenic process. In this study of women who have never had cancer and who underwent breast reduction surgery, we found genome-wide differential methylation between EAs and AAs. There were a total of 485 differentially methylated loci after correction for multiple testing. Promoter-related hypermethylated DM CpGs were about twice as frequent among EAs relative to AAs in promoters while DM CpGs among AAs were more likely to be in the gene body or in intergenic regions. We also found different correlation patterns between methylation levels of DM CpGs and gene expression by race. Gene network analysis showed that CpGs that were hypermethylated in AA or EA shared biological pathways although the genes involved in 2 networks were different. To our knowledge, the present study is the first to comprehensively examine genome-wide differential DNA methylation between EAs and AAs in histologically normal breast tissues.
Racial differences in breast cancer incidence and mortality among US women are well documented. EAs have the highest incidence rate while AAs have poorer survival rates.Citation1 This disparity in breast cancer is believed to be a complex combination of breast cancer risk factors including environment, socioeconomic status, and genetics.Citation12 Previous studies have shown biological differences of DNA methylation in breast cancers by races,Citation3 suggesting the impact of race on molecular pathways in cancers. However, these studies have been limited by a focus on tumor suppressor genes (e.g., CDH13, HIN-1, TWIST1, CCND2, and RASSF1A). Recently, Ambrosone et al. reported genome-wide differential methylation between EAs and AAs among women diagnosed with breast cancer (ER-negative cancer in particular), suggesting methylation may provide, a least in part, a biologic basis for the observed racial disparity.Citation2 It remains unclear if such differences are present in normal breast tissues; such differences could be potentially useful to understand epigenetic predisposition that influences racial disparities for the disease.
In the current study, 485 CpGs were identified as differentially-methylated between EAs and AAs. By detailed characterization of the 485 DM CpGs, the highest frequency of hypermethylated CpGs were observed in promoter regions and gene body in EAs and AAs, respectively, suggesting that these DM CpGs may possibly play different roles in gene regulation. Similarly, there was approximately twice the frequency of hypermethylated CpGs in promoter regions in EAs (22%) compared to AAs (12%) in ER negative breast tumors.Citation2
Understanding how differences in DNA methylation relate to gene expression is important. However, it can be challenging to understand given the complex association of DNA methylation with gene expression. For examples, dense intragenic DNA methylation alters chromatin structure that reduces the efficiency of Polymerase II elongation,Citation30 the rate of transcriptional elongation regulating alternative splicing Citation31; transcription influenced by DNA methylation via chromatin remodeling Citation32; and methylation of gene bodies lead alternative splicing and disease-causing mutations.Citation33
In this study, 10% of the DM CpGs were significantly correlated with the gene expression. This figure is similar to previous genome-wide studies in primary fibroblast samples (8.9%),Citation34 ductal carcinoma in situ (DCIS) of the breast (10.5%) Citation35 and considerably higher than other studies in breast tumors (The Cancer Genome Atlas (TCGA) breast cancer data set) (3.3%) (35). Taken together, these data suggest that associations of methylation to gene expression vary by tissue type. The observed 10% of the CpGs correlated with expression suggests that the expression is likely affected by other biological mechanisms such as other epigenetic factors (e.g., noncoding RNAs and histone modification) and DNA sequence variation.
Biological networks of the DM genes showed different top networks involved for correlated genes in EAs and AAs, but both networks were connected through Ubiquitin C, suggesting there may be common biological roles of the DM CpGs in breast tissues between EAs and AAs. Ubiquitin is a small regulatory protein with multiple important functions including roles in protein degradation, DNA repair, cell cycle regulation, kinase modification, and cell signaling pathways.Citation36 A ubiquitin-proteasome pathway involving ubiquitin in breast cancer was emphasized in the regulation of proteins stability including breast cancer suppressor such as BRCA1-BARD1, ErbB2/HER2/Neu.Citation37 Recently, an ubiquitin gene network was suggested as a potential prognostic biomarker for multiple breast cancer subsets.Citation38 Additional studies are needed to clarify whether the different gene networks for correlated genes by race impacts breast cancer disparity, and if this difference is somehow associated with Ubiquitin C.
We also searched the literature for the 23 most relevant DM CpGs at a significance level surpassing Bonferroni correction for all tested probes (P <1.66×10−7) to investigate if the DM CpGs may have important roles in cancer development. About 26% of the DM CpGs related genes have been studied in cancer (including breast cancer) and include one oncogene (TNK2) and 4 TSGs (AHRR, OPCML, PACS2, and HIPK2). TNK2, an oncogenic kinase, has been shown to enhance migration and invasion of breast cancers Citation39 and promote the growth of tamoxifen-resistant breast cancer.Citation40 The lost or reduced expression of AHRR has been observed in multiple human malignant tissues including breast cancers, and knockdown of this gene in in vitro experiments conferred resistance to apoptotic signals, enhanced motility and angiogenic potential. OPCML gene was found to be regulated by hypermethylation in breast cancer.Citation41,42 PACS2 and HIPK2 may play important roles in the DNA damage signaling by modulation of SIRT1-mediated deacetylation of p53 and co-localization and phosphorylation of p53.Citation43 Thus, it is conceivable, with the data reported herein, that these genes may influence racial disparities in breast cancer etiology and prognosis.
This study had several strengths. It is the first to profile methylation differences between EAs and AAs in normal breast tissues, before morphological changes occur during carcinogenesis. While studies of breast cancer patients, and their tumor and adjacent morphologically normal tissues indicate a field effect, it may be that those field effects represent a very early carcinogenic process. Separately, in order to profile the methylation differences by race, we excluded probes containing single nucleotide polymorphisms or weak binding. Understanding factors related to those early changes may provide insight into the early carcinogenic process. Additionally, we were able to validate methylation with gene expression and propose plausible biological implications of our finding.
This study also had some limitations. First, we were limited by the small sample size (and a low number of AAs women in particular) limited a statistical power; there may be additional DM CpGs between EAs and AAs that we were not able to identify. In addition, our participants underwent elective reduction mammoplasty, so our findings may not be generalizable to other women. However, this limitation was tempered by multivariable adjustment analysis for age and BMI. Another is a methodological limitation that bisulfite treatment-based HM450 is unable to distinguish between 5mC and 5-hydroxymethylcytosine (5hmC), an important emerging site for methylation.Citation44 A final limitation is that we have not yet linked the identified DM with known other breast cancer risk factors, available from epidemiological questionnaires.
In summary, we report for the first time a comprehensive analysis of genome-wide differential methylation between EAs and AAs in histologically normal breast tissues from cancer-free women. Despite limitations in sample size, we observed significantly different methylation levels coupled with gene expression between the races. Our findings indicate that racial differences in DNA methylation exist among healthy women and that those methylation differences are correlated with gene expression at some levels, suggesting a contribution to racial disparities in breast cancer. Confirmation of these findings is warranted as well as future studies to investigate if differential methylation by race is associated with other breast risk factors.
Disclosure of Potential Conflicts of Interest
No potential conflicts of interest were disclosed
Supplemental Material
Supplemental data for this article can be accessed on the publisher's website.
KEPI_A_1121362_supplementary_information.zip
Download Zip (1.1 MB)Acknowledgments
This work was supported by a grant, DOD BC022346. We thank the Genomics Core Facility at Roswell Park Cancer Institute (Buffalo, NY) and Nucleic Acid Shared Resource at The Ohio State University (Columbus, OH) for performing Illumina HumanMethylation450 Beadchip and GeneChip® Human Transcriptome Array 2.0, respectively.
References
- Siegel RL, Miller KD, Jemal A. Cancer statistics, 2015. CA 2015; 65:5-29; PMID:25559415
- Ambrosone CB, Young AC, Sucheston LE, Wang D, Yan L, Liu S, Tang L, Hu Q, Freudenheim JL, Shields PG, et al. Genome-wide methylation patterns provide insight into differences in breast tumor biology between American women of African and European ancestry. Oncotarget 2014; 5:237-48; PMID:24368439; http://dx.doi.org/10.18632/oncotarget.1599
- Wang S, Dorsey TH, Terunuma A, Kittles RA, Ambs S, Kwabi-Addo B. Relationship between tumor DNA methylation status and patient characteristics in African-American and European-American women with breast cancer. PloS One 2012; 7:e37928; PMID:22701537; http://dx.doi.org/10.1371/journal.pone.0037928
- Das PM, Singal R. DNA methylation and cancer. J Clin Oncol 2004; 22:4632-42; PMID:15542813; http://dx.doi.org/10.1200/JCO.2004.07.151
- Christensen BC, Kelsey KT, Zheng S, Houseman EA, Marsit CJ, Wrensch MR, Wiemels JL, Nelson HH, Karagas MR, Kushi LH, et al. Breast cancer DNA methylation profiles are associated with tumor size and alcohol and folate intake. PLoS Genet 2010; 6:e1001043; PMID:20686660; http://dx.doi.org/10.1371/journal.pgen.1001043
- Johnson KC, Koestler DC, Cheng C, Christensen BC. Age-related DNA methylation in normal breast tissue and its relationship with invasive breast tumor methylation. Epigenetics 2014; 9:268-75; http://dx.doi.org/10.4161/epi.27015
- Tao MH, Marian C, Shields PG, Nie J, McCann SE, Millen A, Ambrosone C, Hutson A, Edge SB, Krishnan SS, et al. Alcohol consumption in relation to aberrant DNA methylation in breast tumors. Alcohol 2011; 45:689-99; PMID:21168302; http://dx.doi.org/10.1016/j.alcohol.2010.11.006
- Tao MH, Marian C, Nie J, Ambrosone C, Krishnan SS, Edge SB, Trevisan M, Shields PG, Freudenheim JL. Body mass and DNA promoter methylation in breast tumors in the Western New York Exposures and Breast Cancer Study. Am J Clin Nutri 2011; 94:831-8; PMID:21775555; http://dx.doi.org/10.3945/ajcn.110.009365
- Conway K, Edmiston SN, Tse CK, Bryant C, Kuan PF, Hair BY, Parrish EA, May R, Swift-Scanlan T. Racial variation in breast tumor promoter methylation in the Carolina Breast Cancer Study. Cancer Epidemiology Biomarkers Prevention 2015; 24:921-30; http://dx.doi.org/10.1158/1055-9965.EPI-14-1228
- Dumitrescu RG, Marian C, Krishnan SS, Spear SL, Kallakury BV, Perry DJ, Convit JR, Seillier-Moiseiwitsch F, Yang Y, Freudenheim JL, et al. Familial and racial determinants of tumour suppressor genes promoter hypermethylation in breast tissues from healthy women. J Cell Mol Med 2010; 14:1468-75; PMID:19799643; http://dx.doi.org/10.1111/j.1582-4934.2009.00924.x
- Llanos AA, Dumitrescu RG, Brasky TM, Liu Z, Mason JB, Marian C, Makambi KH, Spear SL, Kallakury BV, Freudenheim JL, et al. Relationships among folate, alcohol consumption, gene variants in one-carbon metabolism and p16INK4a methylation and expression in healthy breast tissues. Carcinogenesis 2015; 36:60-7; PMID:25344837; http://dx.doi.org/10.1093/carcin/bgu219
- Llanos AA, Marian C, Brasky TM, Dumitrescu RG, Liu Z, Mason JB, Makambi KH, Spear SL, Kallakury BV, Freudenheim JL, et al. Associations between genetic variation in one-carbon metabolism and LINE-1 DNA methylation in histologically normal breast tissues. Epigenetics 2015; 10:727-35; http://dx.doi.org/10.1080/15592294.2015.1062205
- Zhang FF, Cardarelli R, Carroll J, Fulda KG, Kaur M, Gonzalez K, Vishwanatha JK, Santella RM, Morabia A. Significant differences in global genomic DNA methylation by gender and race/ethnicity in peripheral blood. Epigenetics 2011; 6:623-9; http://dx.doi.org/10.4161/epi.6.5.15335
- Terry MB, Ferris JS, Pilsner R, Flom JD, Tehranifar P, Santella RM, Gamble MV, Susser E. Genomic DNA methylation among women in a multiethnic New York City birth cohort. Cancer Epidemiology Biomarkers Prevention 2008; 17:2306-10; http://dx.doi.org/10.1158/1055-9965.EPI-08-0312
- Adkins RM, Krushkal J, Tylavsky FA, Thomas F. Racial differences in gene-specific DNA methylation levels are present at birth. Birth Defects Res Clin Mol Teratology 2011; 91:728-36; PMID:21308978; http://dx.doi.org/10.1002/bdra.20770
- Sotiriou C, Pusztai L. Gene-expression signatures in breast cancer. N Engl J Med 2009; 360:790-800; PMID:19228622; http://dx.doi.org/10.1056/NEJMra0801289
- Pirone JR, D'Arcy M, Stewart DA, Hines WC, Johnson M, Gould MN, Yaswen P, Jerry DJ, Smith Schneider S, Troester MA. Age-associated gene expression in normal breast tissue mirrors qualitative age-at-incidence patterns for breast cancer. Cancer Epidemiology Biomarkers Prevention 2012; 21:1735-44; http://dx.doi.org/10.1158/1055-9965.EPI-12-0451
- Sun X, Casbas-Hernandez P, Bigelow C, Makowski L, Joseph Jerry D, Smith Schneider S, Troester MA. Normal breast tissue of obese women is enriched for macrophage markers and macrophage-associated gene expression. Breast Cancer Res Treatment 2012; 131:1003-12; PMID:22002519; http://dx.doi.org/10.1007/s10549-011-1789-3
- Rotunno M, Sun X, Figueroa J, Sherman ME, Garcia-Closas M, Meltzer P, Williams T, Schneider SS, Jerry DJ, Yang XR, et al. Parity-related molecular signatures and breast cancer subtypes by estrogen receptor status. Breast cancer Res 2014; 16:R74; PMID:25005139; http://dx.doi.org/10.1186/bcr3689
- Sun X, Gierach GL, Sandhu R, Williams T, Midkiff BR, Lissowska J, Wesolowska E, Boyd NF, Johnson NB, Figueroa JD, et al. Relationship of mammographic density and gene expression: analysis of normal breast tissue surrounding breast cancer. Clin Cancer Res 2013; 19:4972-82; PMID:23918601; http://dx.doi.org/10.1158/1078-0432.CCR-13-0029
- Llanos AA, Brasky TM, Dumitrescu RG, Marian C, Makambi KH, Kallakury BV, Spear SL, Perry DJ, Convit RJ, Platek ME, et al. Plasma IGF-1 and IGFBP-3 may be imprecise surrogates for breast concentrations: an analysis of healthy women. Breast Cancer Res Treatment 2013; 138:571-9; PMID:23456194; http://dx.doi.org/10.1007/s10549-013-2452-y
- Maksimovic J, Gordon L, Oshlack A. SWAN: Subset-quantile within array normalization for illumina infinium HumanMethylation450 BeadChips. Genome Biol 2012; 13:R44; PMID:22703947; http://dx.doi.org/10.1186/gb-2012-13-6-r44
- Price ME, Cotton AM, Lam LL, Farre P, Emberly E, Brown CJ, Robinson WP, Kobor MS. Additional annotation enhances potential for biologically-relevant analysis of the Illumina Infinium HumanMethylation450 BeadChip array. Epigenetics Chromatin 2013; 6:4; PMID:23452981; http://dx.doi.org/10.1186/1756-8935-6-4
- Chen YA, Lemire M, Choufani S, Butcher DT, Grafodatskaya D, Zanke BW, Gallinger S, Hudson TJ, Weksberg R. Discovery of cross-reactive probes and polymorphic CpGs in the Illumina Infinium HumanMethylation450 microarray. Epigenetics 2013; 8:203-9; http://dx.doi.org/10.4161/epi.23470
- Du P, Zhang X, Huang CC, Jafari N, Kibbe WA, Hou L, Lin SM. Comparison of Beta-value and M-value methods for quantifying methylation levels by microarray analysis. BMC Bioinformatics 2010; 11:587; PMID:21118553; http://dx.doi.org/10.1186/1471-2105-11-587
- Quackenbush J. Computational analysis of microarray data. Nat Rev Genetics 2001; 2:418-27; PMID:11389458; http://dx.doi.org/10.1038/35076576
- Williams HAaLJ. Principal component analysis. Wiley Interdisciplinary Rev 2010; 2:433-59; http://dx.doi.org/10.1002/wics.101
- Bibikova M, Barnes B, Tsan C, Ho V, Klotzle B, Le JM, Delano D, Zhang L, Schroth GP, Gunderson KL, et al. High density DNA methylation array with single CpG site resolution. Genomics 2011; 98:288-95; PMID:21839163; http://dx.doi.org/10.1016/j.ygeno.2011.07.007
- Bock C, Walter J, Paulsen M, Lengauer T. CpG island mapping by epigenome prediction. PLoS Computational Biol 2007; 3:e110; PMID:17559301; http://dx.doi.org/10.1371/journal.pcbi.0030110
- Lorincz MC, Dickerson DR, Schmitt M, Groudine M. Intragenic DNA methylation alters chromatin structure and elongation efficiency in mammalian cells. Nat Struct Mol Biol 2004; 11:1068-75; PMID:15467727; http://dx.doi.org/10.1038/nsmb840
- Kornblihtt AR. Chromatin, transcript elongation and alternative splicing. Nat Struct Mol Biol 2006; 13:5-7; PMID:16395314; http://dx.doi.org/10.1038/nsmb0106-5
- Suzuki MM, Bird A. DNA methylation landscapes: provocative insights from epigenomics. Nat Rev Genetics 2008; 9:465-76; PMID:18463664; http://dx.doi.org/10.1038/nrg2341
- Jones PA. Functions of DNA methylation: islands, start sites, gene bodies and beyond. Nat Rev Genetics 2012; 13:484-92; PMID:22641018; http://dx.doi.org/10.1038/nrg3230
- Wagner JR, Busche S, Ge B, Kwan T, Pastinen T, Blanchette M. The relationship between DNA methylation, genetic and expression inter-individual variation in untransformed human fibroblasts. Genome Biol 2014; 15:R37; PMID:24555846; http://dx.doi.org/10.1186/gb-2014-15-2-r37
- Fleischer T, Frigessi A, Johnson KC, Edvardsen H, Touleimat N, Klajic J, Riis ML, Haakensen VD, Warnberg F, Naume B, et al. Genome-wide DNA methylation profiles in progression to in situ and invasive carcinoma of the breast with impact on gene transcription and prognosis. Genome Biol 2014; 15:435; PMID:25146004
- Lucia Radici MB, Rita Crinelli, Mauro Magnani Ubiquitin C gene: Structure, function, and transcriptional regulation Adv Biosci Biotechnol 2013; 4:1057-62; http://dx.doi.org/10.4236/abb.2013.412141
- Ohta T, Fukuda M. Ubiquitin and breast cancer. Oncogene 2004; 23:2079-88; PMID:15021895; http://dx.doi.org/10.1038/sj.onc.1207371
- Xiang Y, Zhang J, Huang K. Mining the tissue-tissue gene co-expression network for tumor microenvironment study and biomarker prediction. BMC Genomics 2013; 14 Suppl 5:S4; PMID:24564578; http://dx.doi.org/10.1186/1471-2164-14-S5-S4
- Howlin J, Rosenkvist J, Andersson T. TNK2 preserves epidermal growth factor receptor expression on the cell surface and enhances migration and invasion of human breast cancer cells. Breast Cancer Res 2008; 10:R36; PMID:18435854; http://dx.doi.org/10.1186/bcr2087
- Mahajan K, Mahajan NP. ACK1/TNK2 tyrosine kinase: molecular signaling and evolving role in cancers. Oncogene 2014; 34(32):4162-7
- Sellar GC, Watt KP, Rabiasz GJ, Stronach EA, Li L, Miller EP, Massie CE, Miller J, Contreras-Moreira B, Scott D, et al. OPCML at 11q25 is epigenetically inactivated and has tumor-suppressor function in epithelial ovarian cancer. Nat Genetics 2003; 34:337-43; PMID:12819783; http://dx.doi.org/10.1038/ng1183
- Cui Y, Ying Y, van Hasselt A, Ng KM, Yu J, Zhang Q, Jin J, Liu D, Rhim JS, Rha SY, et al. OPCML is a broad tumor suppressor for multiple carcinomas and lymphomas with frequently epigenetic inactivation. PloS One 2008; 3:e2990; PMID:18714356; http://dx.doi.org/10.1371/journal.pone.0002990
- D'Orazi G, Rinaldo C, Soddu S. Updates on HIPK2: a resourceful oncosuppressor for clearing cancer. J Exp Clin Cancer Res 2012; 31:63; http://dx.doi.org/10.1186/1756-9966-31-63
- Lian CG, Xu Y, Ceol C, Wu F, Larson A, Dresser K, Xu W, Tan L, Hu Y, Zhan Q, et al. Loss of 5-hydroxymethylcytosine is an epigenetic hallmark of melanoma. Cell 2012; 150:1135-46; PMID:22980977; http://dx.doi.org/10.1016/j.cell.2012.07.033