ABSTRACT
Hepatocellular carcinoma (HCC) incidence has steadily increased in the US over the past 30 years. Our understanding of epigenetic regulation in HCC is still limited, especially the impact of hepatitis B virus (HBV) or hepatitis C virus (HCV) infection on aberrant DNA methylation. We performed genome-wide DNA methylation profiling in 33 fresh frozen tumor samples, including 10 HBV-HCC, 13 HCV-HCC, and 10 non-infected (NIV-HCC) using the Illumina HumanMethylation450 BeadChip. Gene expression profiling was also performed using the Illumina whole-genome DASL HT Assay. Biological influences and gene networks of the differentially-methylated (DM) CpG loci were predicted using the Ingenuity Pathway Analysis. Genome-wide methylation analysis identified 7, 26, and 98 DM loci between HBV-HCC vs. HCV-HCC, HBV-HCC vs. NIV-HCC, and HCV-HCC vs. NIV-HCC, respectively, at P < 5 × 10−5 for each. Overall, the DM loci were highly enriched for enhancers (48%), promoters (37%), or CpG islands and surrounding regions (37%). Most DM loci were hypermethylated in HCV-HCC compared to HBV-HCC or NIV-HCC. The DM loci were associated with a variety of biological functions including Cell Morphology (HBV-HCC vs. NIV-HCC), Cell Death/ Survival (HBV-HCC vs. NIV-HCC), or Cellular Growth and Proliferation (HCV-HCC vs. NIV-HCC). A subset of the DM loci were correlated (either positively or negatively) with their gene expression or associated with alcohol consumption, BMI, cirrhosis, diabetes, and cigarette smoking. Our findings of differential methylation by viral infection lend insights into the potential effects of viral infection on the epigenetic regulation and further the development and progression of HCC.
Abbreviations
DM | = | differentially-methylated |
HBV | = | hepatitis B virus |
HCC | = | Hepatocellular carcinoma |
HCV | = | hepatitis C virus |
IARC | = | International Agency for Research on Cancer |
IP | = | Ingenuity Pathway Analysis |
NIV | = | non-infected |
shelves | = | 2 kb regions upstream and downstream of the CpG island shores |
shores | = | 2 kb regions upstream and downstream of the CpG islands |
TSG | = | tumor suppressor genes |
TSS | = | transcription start site |
TSS200 | = | 200 bp of a transcription start site |
TSS1500 | = | 1500 bp of a transcription start site |
UBC | = | Ubiquitin C |
Introduction
Liver cancer is the second leading cause of cancer deaths in the world.Citation1 In the US, 39,230 new primary liver cancer cases are expected to be diagnosed in 2016, with approximately 3-fourths being hepatocellular carcinoma (HCC).Citation2 The development of HCC is a complex and multifactorial process involving accumulation of genetic and epigenetic alterations that occur along with the progression of normal liver to chronic hepatitis, cirrhosis and, ultimately, HCC.Citation3,4 The major risk factor for HCC is infection with either hepatitis B virus (HBV) or hepatitis C virus (HCV), the prevalence of which varies from country to country.Citation5 Both HBV and HCV are categorized as human carcinogens by the International Agency for Research on Cancer (IARC).Citation6
Aberrant DNA methylation has been consistently observed in HCC. With the candidate gene approach, studies have found hypermethylation in various tumor suppressor genes (TSG) and hypomethylation in oncogenes in HCC.Citation7-9 Recent genome-wide DNA methylation profiling studies have noted substantial DNA methylation changes in HCC,Citation3,4,10,11 but most of these studies were not designed to specifically identify the changes associated with HBV or HCV infection.
While our prior study showed substantial genome-wide differences in DNA methylation between HCC and adjacent normal tissues,Citation10 we did not examine methylation changes by viral status and other risk factors of HCC. The aim of this study is to evaluate if there are differentially-methylated (DM) loci that are associated with viral infection in HCC and how these differences in epigenetic regulation affect gene expression and relate to biological functions that may influence on HCC development or progression.
Materials and methods
Study enrollment and biospecimen and data collection
This study was approved by the Institutional Review Board of the University of Hawaii at Manoa and all study participants signed a written informed consent. Tumor samples from 33 HCC patients, including 27 from our previous study, Citation10 were analyzed for genome-wide DNA methylation. The 33 samples include 10 non-infected (NIV-HCC), 13 HCV-HCC, and 13 NIV-HCC. HCC was diagnosed histologically by percutaneous biopsy, liver biopsy at the time of surgery, or explanted liver at transplant. Patient information was self-reported and includes age, sex, and ethnicity as identified by the patients, a history of diabetes, a history of cancer besides HCC, previous or current tobacco use, and a family history of cancer. Risk factors for HCC including viral hepatitis, alcohol abuse (defined as greater than 2 drinks/daily for at least 10 years). The presence of other chronic liver diseases was obtained from the medical records. HBsAg, anti-HBc, and anti-HCV serologic status was derived from clinical laboratory testing conducted as part of patients' diagnoses and/or prior medical history. All operative procedures were performed at Hawaii Medical Center-East (2009–2011) by a hepatobiliary/liver transplant surgeon (LW). Final tumor diagnoses and grading of adjacent normal tissue were confirmed by a hepatobiliary pathologist. Adipose tissue was removed through blunt-dissection before the tissue specimen were treated RNA later® (Ambion, Inc., Austin, TX) and stored at −80°C until use.
DNA preparation and genome-wide methylation analysis
DNA was extracted from the tissues using the AllPrep DNA/RNA Mini kit (Qiagen Inc., Valencia, CA). About 500 ng of DNA based on NanoDrop-2000 reading (Thermo Scientific, Wilmington, DE) was bisulfite treated using the EZ DNA Methylation kit (Zymo Research, Irvine, CA) according to the manufacturer's recommendations. The Methylation450 BeadChips (HM450) (Illumina, San Diego, CA) were used to analyze the genome-wide DNA methylation profile across 485,512 CpG loci.Citation9 GenomeStudio (v.2011.1) Methylation module (v.1.9.0) software was used to generate a β-value after normalization and background subtraction with the internal controls. The methylation level at each CpG locus represented by a β-value was calculated according to the normalized probe fluorescence intensity ratio between methylated and unmethylated signals. β-values vary between 0 (fully unmethylated) and 1 (fully methylated). Any probe's fluorescence intensity that was not significantly different from the background (P > 0.05) was removed from further analysis. In addition, probes that were designed for CpG sites on either the X or Y chromosome were excluded from analysis. The remaining CpG sites analyzed in the study were 472,009.
RNA preparation and genome-wide expression analysis
Total RNA was extracted from tissues using the AllPrep DNA/RNA Mini kit (Qiagen Inc., Valencia, CA). The quality of the RNA was checked by running 1 μl of each RNA sample on the Bioanalyzer (Agilent, Santa Clara, CA) using the RNA 6000 Nano chip. RNA (200 ng) was labeled, hybridized onto expression microarrays, and scanned. Briefly, the Illumina Whole Genome DASL (WG-DASL) method uses biotinylated random nonamer and oligo(dT) primers to convert input RNA to cDNA. Next, the biotinylated cDNA is annealed to DASL Assay Pool (DAP) probe groups for extension and ligation followed by PCR amplification with a biotinylated and a fluorophore-labeled universal primer. Last, the single stranded PCR products are eluted and hybridized onto an Illumina HT-12 BeadChip. Due to tissue availability, only a subset of samples (10 HBV-HCC, 9 HCV-HCC, and 9 NIV-HCC) was analyzed by the WG-DASL Assay.
Pyrosequencing
A subset of DM loci (n=6) found by the Methylation450 BeadChip were selected for technical validation in 7 HBV-HCC, 7 HCV-HCC, and 7 NIV-HCC samples. About 25 ng of bisulfite treated DNA was first amplified by PCR in the regions of interest using the PyroMark PCR kit (Qiagen, Valencia, CA) following the suggested protocol. All assays were custom designed using PyroMark Assay Design 2.0. Human Methylated and Non-methylated DNA Set from Zymo Research was used as controls (data not shown). All assay information is described in Supplementary Table 1. A PyroMark Q24 instrument (Qiagen) was used and the sequencing results were analyzed using the PyroMark Q24 software (Qiagen).
Statistical analysis
Distributions of clinical characteristics between HCC participants with different viral infection status were compared using the chi-square and Mann-Whitney rank tests for categorical and continuous variables, respectively.
Methylation β-values were converted to M-values for statistical analysis.Citation12 Partek Genomics Suite (Partek Inc., St. Louis, MO) was used for the analysis. A multivariate ANOVA was performed to identify DM loci by viral infection after adjusting for age and scan date (for batch effect removal) since these variables also contributed to the data variance (data not shown). Raw P-values lower than 5×10−5 were considered statistically significant since no CpGs were significantly different at the Benjamini and Hochberg's false discovery rate (FDR) q < 0.05. Raw P-value < 5×10−5 was corresponding to FDR q < 0.99, q < 0.70, and q < 0.26 in HBV-HCC vs. HCV-HCC, HBV-HCC vs. NIV-HCC, and HCV-HCC vs. NIV-HCC, respectively.
Unsupervised clustering analysis and Principal Component Analysis (PCA) were performed using the Partek Genomics Suite. PCA produced linear combinations of the variables (DM loci) to generate the axes known as principal components, or PCs. In this study, PC1 and PC2 were shown in the results. The Euclidian distance between the groups of samples was calculated with the average linkage.
To identify DM CpG islands, methylation levels (β-value) were averaged based on hg18 CpG islands downloaded from UCSC Genome Browser. This yielded 26,327 CpG islands. The averaged β-values were converted to M-values. A multivariate ANOVA was performed to identify CpG islands by viral infection after adjusting for age and scan date.
DM loci were classified by CpG island or its neighbors [2 kb regions upstream and downstream of the CpG islands (shores) or 2 kb regions upstream and downstream of the CpG island shores (shelves)] and functional promoters [within 1500 bp of a transcription start site (TSS) (TSS1500), within 200 bp of a TSS (TSS200), 5′ untranslated regions (5′UTR), first exon (1stExon)], and other regions (body, 3′UTR, or intergenic). To identify DM regions associated with gene function, loci were first stratified by functional locations described above, plus enhancers and non-enhancers. Next, methylation levels in all loci located each region were averaged. Mann-Whitney rank tests with P < 0.05 was considered statistically significant.
GenomeStudio was used to process the raw data generated by the Illumina WG -DASL Assay, and the processed data were analyzed in Partek Genomics Suite. Probes with detection P-value less than 0.05 were considered valid, and 14,167 genes were reliably measured on the expression assay. For the correlation analysis, Target IDs (Probe IDfrom HM450) of the DM loci were generated to match the UCSC reference genes. Since a CpG site could be localized in more than one gene (co-localization of the genes), a Target ID could be linked to multiple genes. For gene expression, intensities from the Illumina WG -DASL Assay were averaged if there were more than one probe for a gene. Then, the correlation analysis between methylation and gene expression was performed. If there were more than one probe for a gene, the intensities were averaged (gene level analysis). Spearman correlation with P < 0.05 was considered statistically significant when analyzing the relevance of the association of methylation to expression.
DM loci associated with viral infection were further analyzed in all 33 HCC samples for their associations with HCC risk factors, including alcohol consumption, BMI, cirrhosis, diabetes, gender, hyperlipidemia, and smoking. Mann-Whitney rank test was used for the analysis and P < 0.01 were considered statistically significant.
Ingenuity pathway analysis
Ingenuity Pathway Analysis (IPA) (Qiagen, Redwood City, CA) was used to predict gene networks and biological functions. Genes stored in the IPA Ingenuity Knowledge Base (IPKB) were cataloged based on reported or suggested biological and molecular functions as well as disease involvement.Citation13 The identified genes were mapped to networks available in the IPA and then ranked by score. The score (score = −log10[P-value]) computed by IPA is according to the probability of the set of identified genes and a list of biological functions stored in the IPKB. A score greater than 3 represents a corresponding P < 0.0001, indicating that a gene network was not generated by chance alone.Citation14
The cancer genome atlas data (TCGA)
We used level 1 data from 380 liver hepatocellular carcinoma (LHCC) tumors in TCGA, including the idat files from the Methylation450 BeadChip. The data were normalized with internal controls and background subtraction as we did for our study. Among 380 HCC tumors, 115 had information on viral infection including 44 HBV/HCV-HCC, 57 HBV-HCC, and 14 HCV-HCC. The 71 samples (HBV-HCC or HCV-HCC) were used for biological validation of DM loci.
Results
Clinical and pathological characteristics of patients
Characteristics of study participants are shown in . The mean ages at HCC diagnosis for HBV-HCC, HCV-HCC, and NIV-HCC were 62.3 (SD ± 2.2), 64.08 (SD ± 8.5), and 72.0 (SD ± 11.6) years, respectively. The presence of cirrhosis and diabetes differed between HBV-HCC and NIV-HCC at P = 0.02 and P = 0.026, respectively. Age, alcohol consumption, BMI, smoking status, hyperlipidemia, AJCC stage, and race were not significantly different (P > 0.05).
Table 1. Characteristics of study participants.
DM loci in HBV-HCC vs. HCV-HCC
Seven DM loci localized in 6 genes and one intergenic locus (cg03994465) were identified between HBV-HCC and HCV-HCC (, Supplementary Table 3). Both PCA and heatmap showed clear separations by these DM loci (), respectively. The loci were approximately 2.2-fold more methylated in HCV-HCC (average β-value: 0.236) relative to HBV-HCC (average β-value: 0.513) (P = 5.57×10−5) ().
Figure 1. Differentially methylated CpGs between HBV-HCC vs. HCV-HCC. (A) Principal component analysis of the methylation data was plotted using the first 2 principal components (PC1 = 67.2% and PC2 = 9.04%). Each dot represents a sample (red for HBV-HCC and blue for HCV-HCC). (B) Unsupervised hierarchical clustering of β-values for differentially methylated loci. Red and blue blocks on the top of the maps represent HBV-HCC (n = 10) and HCV-HCC (n = 13), respectively. (C) Mean methylation level for all 7 differentially-methylated loci among HBV-HCC (left) and HCV-HCC (right). (D) The top IPA network involving differentially methylated genes. Red and blue genes indicate differentially methylated genes and the connected cancer related genes, respectively. (E) The top associated cellular functions with numbers of differentially-methylated genes.
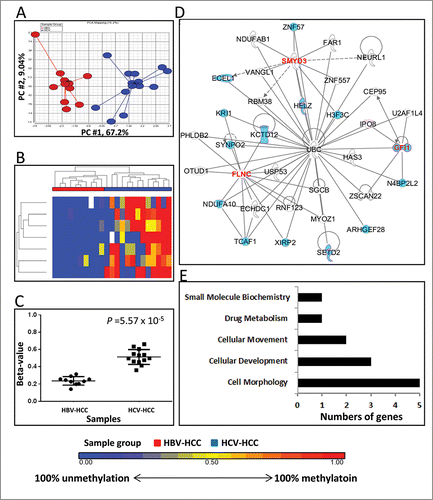
Among 7 DM loci, 5 of those localized in genes (BMP8A, C1orf194, FLNC, GFl1, and KIAA1324) were associated with promoter regions, covering TSS1500, TSS200, 5′UTR, and 1stExon (Supplementary Table 3). Six of the DM loci (85.7%) were located in CpG islands and 4 (57%) in Enhancers (Supplementary Table 3). The DM locus in the BMP8A promoter was in a CpG island, and it had the most significant difference in methylation, Δβ = 0.36 (P = 8.42×10−6) (Supplementary Table 3).
Gene network analysis showed that “Organ Morphology, Skeletal and Muscular System Development and Function, and Connective Tissue Disorder” are the top functions or disease (Score = 8) (). This network was connected with Ubiqutin C (UBC), and 88% of genes in this network were related to cancer development, including HCC (15 HCC related genes; blue colored in ). The top associated cellular function was cell morphology (associated with BMP8A, FLNC, GIF1, KIAA1324, and SMYD3) ().
DM loci in HBV-HCC vs. NIV-HCC
We identified 26 DM loci between HBV-HCC and NIV-HCC (Supplementary Table 4). The two types of HCC showed a clear difference in the methylation pattern of those DM loci (). All except one locus located in the promoter of THBS1 were significantly hypermethylated in HBV-HCC (range of β-value: 0.66–0.80) relative to NIV-HCC (range of β-value: 0.34–0.66) at P = 1.08×10−5 (). Of the 26 DM loci, 14 (54%) were located in Enhancer regions (Supplementary Table 4). Among 25 hypermethylated loci in HBV-HCV compared to NIV-HCC, only one locus (TFAP2A) was located in a CpG Island (Supplementary Table 4); while 8 loci were in the surrounding regions of a CpG island (Shores or Shelves). Of the DM loci, 17 (65%) were associated with 17 unique genes that were involved in the top IPA network of “Cellular Development, Cellular Growth and Proliferation, and Embryonic Development” (Score = 19) (). This network was connected by extracellular regulated kinase (ERK). In this network, 68% of the genes played roles in cancer development including HCC (14 HCC related genes, blue colored in ). The DM loci were enriched for the genes having roles in Cell Death and Survival, including ATF5, CPEB4, CSF2RB, HOXA3, MMP13, NUP62, TFAP2A, and THBS1 ().
Figure 2. Differentially methylated CpGs between HBV-HCC vs. NIV-HCC. (A) Principal component analysis of the methylation data was plotted using the first 2 principal components (PC1 = 68.4% and PC2 = 6.55%). Each dot represents a sample (red for HBV-HCC and green for NIV-HCC). (B) Unsupervised hierarchical clustering of β-values for differentially methylated loci. Red and green blocks on the top of the maps represent HBV-HCC (n = 10) and NIV-HCC (n = 10), respectively. (C) Mean methylation level for all 7 differentially-methylated loci among HBV-HCC (left) and NIV-HCC (right). (D) The top IPA network involving differentially methylated genes. Red and blue genes indicate differentially methylated genes and the connected cancer related genes, respectively. (E) The top associated cellular functions with numbers of differentially-methylated genes.
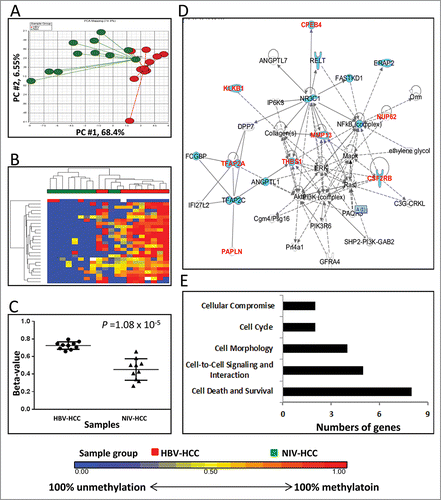
DM loci in HCV-HCC vs. NIV-HCC
Ninety-eight DM loci were identified between HCV-HCC and NIV-HCC (Supplementary Table 5). This number (98 DM) was much higher than that identified between HBV-HCC and NIV-HCC (26 DM) (). The 98 DM loci clearly separated HCV-HCC from NIV-HCC (). All these loci were hypermethylated in HCV-HCC (range of β-value: 0.66–0.78) relative to NIV-HCC (range of β-value: 0.32–0.68) at P = 1.21×10−4 ().
Figure 3. Differentially methylated CpGs between HCV-HCC vs. NIV-HCC. (A) Principal component analysis of the methylation data was plotted using the first 2 principal components (PC1 = 64% and PC2 = 6.65%). Each dot represents a sample (blue for HCV-HCC and green for NIV-HCC). (B) Unsupervised hierarchical clustering of β-values for differentially methylated loci. Blue and green blocks on the top of the maps represent HCV-HCC (n = 13) and NIV-HCC (n = 10), respectively. (C) Mean methylation level for all 7 differentially-methylated loci among HCV-HCC (left) and NIV-HCC (right). (D) The top IPA network involving differentially methylated genes. Red and blue genes indicate differentially methylated genes and connected cancer related genes, respectively. (E) The top associated cellular functions with numbers of differentially-methylated genes.
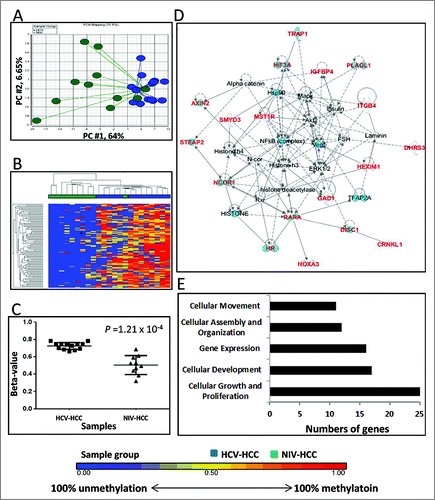
Of the 98 DM loci, 45 (46%) were located in Enhancers, and 34 (35%) were in promoter regions (TSS1500, TSS200, 5′UTR, and 1stExon) (Supplementary Table 5). Sixty-eight of the DM loci (70%) were localized in 60 genes and the rest (30%) were in the intergenic regions (Supplementary Table 5). The DM genes were enriched for transcription regulators (20%, 13/60), including DNAJB6, HEXIM1, HIF3A, HOXA3, HOXA6, HR, NCOR1, PLAGL1, SHOX2, TBX15, TBX4, and TEAD2, and enzymes (17%, 10/60), including CES3, DHRS3, FBXL13, GAD1, SMYD3, ST5, TRAP1, TRIM71, TTLL1, and VARS2. Gene network analysis indicated that the DM genes were involved in “Embryonic Development, Organismal Development, and Cardiovascular Disease” (Score=43) (), and were associated with Cellular Growth and Proliferation (AXIN2, CDHR2, DISC1, DNAJB6, GAD1, HEXIM1, HOXA3, HR, IGFBP4, ITGB4, MST1R, NCOR1, PLAGL1, PRKCDBP, RARA, ROR1, SHOX2, SMYD3, ST5, STEAP2, TEAD2, TFAP2A, TRAP1, TRIM71, and TSPO) ().
Correlation between DM loci and gene expression
Spearman correlation analysis was performed to investigate correlations of the DM loci with expression of their corresponding genes. The analysis showed that 15 DM loci were significantly correlated with gene expression, 60% of which were located in the enhancer regions (). Two highly correlated CpG island DM loci between HBV-HCC and HCV-HCC were in inverse correlation (high methylation and low expression) and were located in the gene body of MFSD7 (r = −0.41, P = 0.031) and promoter of FLNC (r = −0.43, P = 0.022) (). Also, 2 DM loci that were hypermethylated in HBV-HCC compared to NIV-HCC were significantly correlated with gene expression. One was in the S_Shore of the ATF5 promoter and correlated with low expression of the gene (r = −0.64, P = 0.00025). Another located in the 5′UTR (not related to CpG island) of KIAA1199 was also positively correlated with its expression (r = 0.40, P = 0.036) (). In addition, we found 11 DM loci between HCV-HCC and NIV-HCC that were significantly correlated with the expression of 10 paired genes either negatively (CES3, DHRS3, IGFBP4, LRRC17, and SGIP1) or positively (AXIN2, HOXA6, RAB11FIP4, ROR1, and STEAP2) at P < 0.05 ().
Table 2. Correlations of DM loci and gene expression.
DM loci associated with clinical characteristics of HCC
The DM loci from above analyses were further analyzed for their associations with clinical characteristics, including alcohol use, BMI, cirrhosis, diabetes, gender, hyperlipidemia, and smoking in all 33 patients. The most significant results are shown in , and a detailed list of correlations is provided in Supplementary Table 6. The DM loci in MMP13, FMNL3, and MST1R were associated with drinking and smoking habits, and history of cirrhosis, with higher methylation in ever drinkers compared to never drinkers (average β-value 0.95 vs. 0.75), in cirrhosis vs. non-cirrhosis (average β-value 0.81 vs. 0.67), and ever smokers vs. never smokers (average β-value 0.82 vs. 0.72), respectively. A significantly lower methylation in an interegenic locus (enhancer linked region, cg06817454) was found in diabetes relative to non-diabetes (average β-value 0.56 vs. 0.70). Another intergenic locus (cg05412459) located in the S_Shelf was significantly correlated with BMI (r = −0.55, P = 0.0009). None of the DM loci were correlated with gender or hyperlipidemia. A heatmap of methylation levels of all identified DMs by different viral infection as well as clinical characteristics was shown in all 33 samples (). Lower methylation levels of DM loci among NIV-HCC (more consistently blue) were found compared to the ones in HBV-HCC and HCV-HCC (more consistently red). Although overall the unsupervised clustering analysis showed well separated methylation profiles between HBV-HCC and HCV HCC, these groups still had quite similar methylation patterns regardless of clinical characteristics ().
Figure 4. Association between differentially methylated loci by virus infection and other HCC risk factors. Mann-Whitney rank test was used for alcohol consumption (ever vs. never), cirrhosis (yes vs. no), diabetes (yes vs. no), and smoking (yes vs. no) and Spearman correlation was used for BMI (continuous variable).
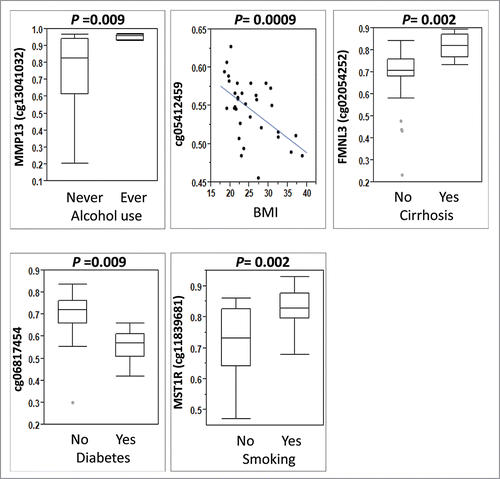
Figure 5. Unsupervised hierarchical clustering of β-values for all differentially methylated loci identified by different viral infection. Unsupervised hierarchical clustering of β-values for DM loci (rows) in 33 HCC samples (columns). Red, blue, green blocks on the top of the maps represent HBV-HCC (n = 10), HCV-HCC (n = 13) and NIV-HCC (n = 10), respectively. The clinical characteristics of the samples including alcohol use, BMI, cirrhosis, diabetes, and smoking were represented as other blocks below sample group.
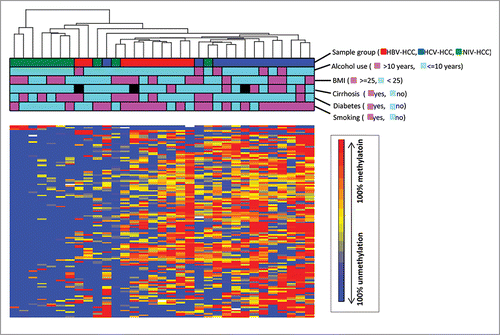
Pyrosequencing analysis
To validate the HM450 results, we selected 6 DM loci for pyrosequencing analysis based on their P-values and availability for assay design. The loci include cg15931894 (CRIM1) and cg02585724 (KIAA1199) identified in HBV-HCC vs. NIV-HCC, and cg00727675 (HOXA3) and cg02149965 (VARS2) identified in HCV-HCC vs. NIV-HCC (Supplementary Table 1). Due to the limitation in assay design for top significant DM loci between HBV-HCC vs. HCV-HCC, we had to select 2 alternate DM loci at raw P-values lower than 1×10−4 for technical validation, cg00201414 (THSD1P) and cg13170600 (Supplementary Table 1). High concordance of methylation levels for all DM loci analyzed was found between the microarray and pyrosequencing analyses (Spearman correlation r = 0.94, P = 6.5 × 10−60) (Supplementary ).
Biological validation of DM loci using TCGA samples
Characteristics of study patients between our study and TCGA are shown in Supplementary Table 2. Most of the patients in TCGA were HBV-HCC (80%) compared to our samples (43%) at P = 0.0007. Race was significantly different for HBV-HCC and HCV-HCC, P = 0.0002 and P = 0.0001, respectively. A majority of the participants in our samples were Asian (78%) while 2 thirds of the patients in TCGA were White (66%). Stages were significantly different for HBV-HCC (P < 0.0001). All HBV-HCC in our samples was the early stage (1 or 2) while 30% of HBV-HCC in TCGAs was the late stage (3 or 4). Age and gender were not different (P > 0.05).
The seven DM loci identified between HBV-HCC and HCV-HCC in UHCC were also analyzed in the TCGA samples (Supplementary ). A locus in BMP8A (cg02575697) was significantly methylated in HCV-HCC compared to HBV-HCC (P = 0.004). A borderline significance was observed for another locus co-localized in KIAA1324 and C1orf194 (cg09820792) (P = 0.06). Although other DM loci did not show statistical difference, we found the expected direction of methylation differences in all loci except 2 loci in an intergenic (cg03994465) and a body of SMYD3 (cg08106377).
Discussion
DNA methylation has been shown as significantly different between HCC and adjacent normal tissue.Citation3,4,10,11 HBV and HCV infection are the 2 main risk factors responsible for HCC development in humans,Citation5 but our understanding of viral infection-associated epigenetic changes in HCC is still limited.Citation15,16 In this study, we investigated genome-wide methylation profiles in HCC in association with viral infection, and found many differentially methylated loci between virally infected and non-infected tumors as well as by different types of infection (HBV vs. HCV). We also discovered that most of the DM loci were in the enhancer regions, CpG islands or gene promoters suggesting a role in the regulation of gene expression (Supplementary Table 7). Indeed, a subset of the DM loci was significantly correlated with the expression of their corresponding genes. We also identified some of the DM loci associated with alcohol use, BMI, cirrhosis, diabetes, and smoking. Biological gene network analysis showed that the majority of the DM genes were related to cancer development including that of HCC and were associated with Cell Morphology, Cell Death/Survival, and Cellular Growth and Proliferation.
HBV and HCV are 2 different types of viruses (DNA vs. RNA), indicating that their molecular mechanisms underlying the liver tumorigenesis may be different.Citation17 HBV may work either through viral DNA insertion into the human genome to activate oncogenes and/or induce genetic instability, or through immune responses to the viral infection which lead to fibrosis and cirrhosis.Citation18,19 HCV, however, is a single-stranded RNA virus the life cycle of which is thought to be predominantly cytoplasmic,Citation20 and the tumorigenic effects may be mediated through the alteration of cell signaling and metabolism as well as via increased immune responses.Citation21
In the current study, we observed more hypermethylated DM loci in HCV-HCC and HBV-HCC as compared to NIV-HCC, particularly in HCV-HCC. This finding is supported by several previous studies that focused on tumor suppressor genes. Deng et al. showed hypermethylation of 15 differentially methylated genes in HCV-HCC relative to HBV-HCC.Citation22 Similarly, Yang et al. found three tumor suppressor genes (APC, p15, SOCS1) to be more frequently methylated in HCV-HCC compared to HBV-HCC or NIV-HCC.Citation23 Moreover, Nishida et al. reported large abundance and higher levels of methylation in HCV-HCC compared to normal liver tissues and NIV-HCC, respectively.Citation24 Thus, evidence supports more prominent methylation changes in HCV-HCC, and our study identified several novel loci with the changes in comparison to HBC-HCC or NIV-HCC.
Altered methylation in regulatory regions such as enhancers or regions surrounding the transcription start sites is known to be important in regulating gene expression.Citation25 Understanding how altered methylation relates to gene regulation can be complex because it has been shown that promoter methylation regulates gene expression while gene body methylation affects alternative splicing, noncoding RNA expression, and disease-causing mutations.Citation26,27 Our studies showed approximately 2.5-fold higher frequency of DM loci in enhancers (46–57%) compared to total loci analyzed in enhancers (21%), P < 0.05 (Supplementary Table 7), indicating potential importance of these DM loci in regulation of distant genes. Indeed, most of the enhancer-related loci were demonstrated to be correlated with the expression of their associated genes in our study. While a majority of the DM loci in HBV-HCC vs. HCV-HCC were located in a CpG island, DM loci identified in HBV-HCC vs. NIV-HCC or in HCV-HCC vs. NIV-HCC were more likely to be in the surrounding regions of a CpG island (<4 kb from CpG island) or not related to CpG island (>4 kb from CpG island), respectively. These findings were consistent with a separate regional analysis of CpG islands based on UCSC Genome Browser to identify DM CpG island by different viral infection (Supplementary Table 8), where 17 DM CpG islands were identified between HBV-HCC vs. HCV-HCC, while 10 or none were found between HCV-HCC vs. NIV-HCC or HBV-HCC vs. NIV-HCC, respectively. This observation indicates that additional studies are needed to clarify whether different regulatory mechanisms exist for these DM loci with regard to their epigenetic regulation and if any, how this difference in regulation contributes to HCC development or progression in viral HCC.
In this study, gene network analyses revealed potential biological differences between HBV-HCC, HCV-HCC, and NIV-HCC. The top network using the DM genes identified in HBV-HCC vs. HCV-HCC was “Cell Morphology, Cell death/Survival, and Cellular Growth and Proliferation,” which was connected with Ubiquitin C (UBC). UBC was first described as a stress responsive gene Citation28 that regulates various functions of a cell (e.g., protein degradation, DNA repair, cell cycle regulation, kinase modification, endocytosis, and regulation of other cell signaling pathways) (http://www.ncbi.nlm.nih.gov/gene/7316). Through an in silico network analysis based on protein-protein interaction, a recent study suggests potentially important roles of the UBC network in the pathogenesis of HCC.Citation29 Another network, “Cellular Development, Cellular Growth and Proliferation, Embryonic Development” was indicated by the DM loci identified in HBV-HCC vs. NIV-HCC. This network was connected by ERK which is a well-known oncogene frequently activated in many cancers including HCC.Citation30-34 Importantly, it has been known that hepatitis Bx, a transactivation protein to stimulate HBV gene expression, promotes angiogenesis by stimulating the ERK expression during HBV infection.Citation17 The last top network, “Embryonic Development, Organismal Development, Cardiovascular Disease,” predicted through the DM loci in HCV-HCC vs. NIV-HCC, was connected by 5 major genes including AKT, ERK1/2, NFkB, VEGF, and Histone H3. First four of these genes have been well studied for their roles in invasion and metastasis and are suggested to be therapeutic targets for HCC.Citation35,36 Histone H3 is involved with the structure of nucleosomes, and is an important protein in epigenetic regulation of gene expression.Citation37 A recent study by Quan et al. suggests that HCV core-induced epigenetic silencing of SFRP1 may lead to the activation of the Wnt signaling pathway and the downregulation of SFRP1 was due to the loss of binding capacity of acetyl-Histone H3 in the SFRP1 promoter,Citation38 indicating an important role of HCV infection in gene regulation through Histone3.
Given that our findings of significant DM loci were based on locus-by-locus analysis, we questioned if there was significant differences in functional regions by viral infection. A separate analysis was performed to test for DM regions based on functional locations including enhancers. In this analysis, methylation levels of CpG sites were averaged by each region (Supplementary ). We found significantly higher methylation in HCV-HCC compared to NIV-HCC only for TSS200 (P < 0.041) and for enhancer (P < 0.038). Although we did not see significant differences for those regions between HBV-HCC and HCV-HCC or between HBV-HCC and NIV-HCC, the methylation levels of TSS200 in HBV-HCC was 3.9% lower than HCV-HCC, but 2.4% higher than NIV-HCC. Similarly, the methylation levels of HCV-HCC in Enhancer were 7% and 4.4% higher than those of HBV-HCC and NIV-HCC, respectively. Taken together, the results of regional analysis were consistent with those of locus-locus analysis, showing more hypermethylation in HCV-HCC and HBV-HCC compared to NIV-HCC.
This study has several features that distinguish it from previous studies. The present study employed a genome-wide approach to examine DNA methylation differences by viral status in HCC. In contrast, many previous studies focused on selected tumor suppressor genes to evaluate the differences between HCV-HCC and HBV-HCC. We identified additional potentially novel DM loci by viral status using the genome-wide approach. In addition, we explored regional analyses by CpG islands, functional location as well as enhancers. We also evaluated the biological implications of the DM loci by assessing their correlation with gene expression and analyzing their regulatory networks. As strength of the study, we were able to validate a subset of DM loci identified between HBV-HCC and HCV-HCC using an independent dataset (TCGA) even though the differences were less significant in TCGA.
It is important to note some limitations of this study. First, the statistical power of our analyses is limited by a relatively small sample size. Therefore, there may be additional DM loci by viral infection that we were unable to identify. Another limitation is that the patients were primarily Asian, and so our finding may not be generalizable to other populations. However, we had fairly equal numbers of HBV-HCC, HCV-HCC, and NIV-HCC, which may provide valuable insight into different epigenetic alterations by viral infection within a population with high incidence of both viral hepatitis and HCC, while many previous studies focused only on HBV-HCC or HCV-HCC depending on the predominant viral incidence in that specific population.
In summary, we report differentially methylated CpG loci by viral infection in HCC using genome-wide methylation profiling. In spite of the limited sample size, we found clear differences in methylation coupled with altered gene expression among HBV-HCC, HCV-HCC, and NIV-HCC, suggesting viral infection to be an important contributor to the epigenetic changes seen in HCC.
Disclosure of potential conflicts of interest
No potential conflicts of interest were disclosed.
KEPI_A_1151586_supplemental_materials.zip
Download Zip (587.4 KB)Acknowledgments
The authors thank the late Mr. Mike Loomis for building and maintaining the clinical database and Mr. Kyle Miyazaki for collecting clinical data. We thank the Pathology Shared Resource for the collection and processing of tumor tissue samples and the Genomics Shared Resource for performing Illumina HumanMethylation450 Beadchip assay at University of Hawaii Cancer Center. We thank Drs. Janos Molnar and Karolina Peplowska for performing the pyrosequencing.
Funding
This work was supported by the following grant: NIH 3P30CA071789-12S6.
Reference
- McGlynn KA, Petrick JL, London WT. Global epidemiology of hepatocellular carcinoma: an emphasis on demographic and regional variability. Clin liver Dis 2015; 19:223-38; PMID:25921660; http://dx.doi.org/10.1016/j.cld.2015.01.001
- Siegel RL, Miller KD, Jemal A. Cancer statistics, 2015. CA Cancer J Clin 2015; 65:5-29; PMID:25559415; http://dx.doi.org/10.3322/caac.21254
- Shitani M, Sasaki S, Akutsu N, Takagi H, Suzuki H, Nojima M, Yamamoto H, Tokino T, Hirata K, Imai K, et al. Genome-wide analysis of DNA methylation identifies novel cancer-related genes in hepatocellular carcinoma. Tumour Biol 2012; 33:1307-17; PMID:22457049; http://dx.doi.org/10.1007/s13277-012-0378-3
- Shen J, Wang S, Zhang YJ, Wu HC, Kibriya MG, Jasmine F, Ahsan H, Wu DP, Siegel AB, Remotti H, et al. Exploring genome-wide DNA methylation profiles altered in hepatocellular carcinoma using Infinium HumanMethylation 450 BeadChips. Epigenetics 2013; 8:34-43; http://dx.doi.org/10.4161/epi.23062
- El-Serag HB. Hepatocellular carcinoma. N Engl J Med 2011; 365:1118-27; PMID:21992124; http://dx.doi.org/10.1056/NEJMra1001683
- Stuver SO, Boschi-Pinto C, Trichopoulos D. Infection with hepatitis B and C viruses, social class and cancer. IARC Sci Publ 1997:319-24; PMID:9353672
- Zhong S, Tang MW, Yeo W, Liu C, Lo YM, Johnson PJ. Silencing of GSTP1 gene by CpG island DNA hypermethylation in HBV-associated hepatocellular carcinomas. Clin Cancer Res 2002; 8:1087-92; PMID:11948118
- Moribe T, Iizuka N, Miura T, Kimura N, Tamatsukuri S, Ishitsuka H, Hamamoto Y, Sakamoto K, Tamesa T, Oka M. Methylation of multiple genes as molecular markers for diagnosis of a small, well-differentiated hepatocellular carcinoma. Int J Cancer J Int du Cancer 2009; 125:388-97; PMID:19384946; http://dx.doi.org/10.1002/ijc.24394
- Tao R, Li J, Xin J, Wu J, Guo J, Zhang L, Jiang L, Zhang W, Yang Z, Li L. Methylation profile of single hepatocytes derived from hepatitis B virus-related hepatocellular carcinoma. PloS one 2011; 6:e19862; PMID:21625442; http://dx.doi.org/10.1371/journal.pone.0019862
- Song MA, Tiirikainen M, Kwee S, Okimoto G, Yu H, Wong LL. Elucidating the landscape of aberrant DNA methylation in hepatocellular carcinoma. PloS one 2013; 8:e55761; PMID:23437062; http://dx.doi.org/10.1371/journal.pone.0055761
- Shen J, Wang S, Zhang YJ, Kappil M, Wu HC, Kibriya MG, Wang Q, Jasmine F, Ahsan H, Lee PH, et al. Genome-wide DNA methylation profiles in hepatocellular carcinoma. Hepatology 2012; 55:1799-808; PMID:22234943; http://dx.doi.org/10.1002/hep.25569
- Du P, Zhang X, Huang CC, Jafari N, Kibbe WA, Hou L, Lin SM. Comparison of Beta-value and M-value methods for quantifying methylation levels by microarray analysis. BMC bioinformatics 2010; 11:587; PMID:21118553; http://dx.doi.org/10.1186/1471-2105-11-587
- Calvano SE, Xiao W, Richards DR, Felciano RM, Baker HV, Cho RJ, Chen RO, Brownstein BH, Cobb JP, Tschoeke SK, et al. A network-based analysis of systemic inflammation in humans. Nature 2005; 437:1032-7; PMID:16136080; http://dx.doi.org/10.1038/nature03985
- Quackenbush J. Computational analysis of microarray data. Nat Rev Genet 2001; 2:418-27; PMID:11389458; http://dx.doi.org/10.1038/35076576
- Zhao Y, Xue F, Sun J, Guo S, Zhang H, Qiu B, Geng J, Gu J, Zhou X, Wang W, et al. Genome-wide methylation profiling of the different stages of hepatitis B virus-related hepatocellular carcinoma development in plasma cell-free DNA reveals potential biomarkers for early detection and high-risk monitoring of hepatocellular carcinoma. Clinical epigenetics 2014; 6:30; PMID:25859288; http://dx.doi.org/10.1186/1868-7083-6-30
- Lambert MP, Paliwal A, Vaissiere T, Chemin I, Zoulim F, Tommasino M, Hainaut P, Sylla B, Scoazec JY, Tost J, et al. Aberrant DNA methylation distinguishes hepatocellular carcinoma associated with HBV and HCV infection and alcohol intake. J Hepatol 2011; 54:705-15; PMID:21146512; http://dx.doi.org/10.1016/j.jhep.2010.07.027
- Arzumanyan A, Reis HM, Feitelson MA. Pathogenic mechanisms in HBV- and HCV-associated hepatocellular carcinoma. Nat Rev Cancer 2013; 13:123-35; PMID:23344543; http://dx.doi.org/10.1038/nrc3449
- Brechot C. Pathogenesis of hepatitis B virus-related hepatocellular carcinoma: old and new paradigms. Gastroenterology 2004; 127:S56-61; PMID:15508104; http://dx.doi.org/10.1053/j.gastro.2004.09.016
- Di Bisceglie AM. Hepatitis B and hepatocellular carcinoma. Hepatology 2009; 49:S56-60; PMID:19399807; http://dx.doi.org/10.1002/hep.22962
- Moradpour D, Penin F, Rice CM. Replication of hepatitis C virus. Nat Rev Microbiol 2007; 5:453-63; PMID:17487147; http://dx.doi.org/10.1038/nrmicro1645
- Bartosch B. Hepatitis B and C viruses and hepatocellular carcinoma. Viruses 2010; 2:1504-9; PMID:21994691; http://dx.doi.org/10.3390/v2081504
- Deng YB, Nagae G, Midorikawa Y, Yagi K, Tsutsumi S, Yamamoto S, Hasegawa K, Kokudo N, Aburatani H, Kaneda A. Identification of genes preferentially methylated in hepatitis C virus-related hepatocellular carcinoma. Cancer science 2010; 101:1501-10; PMID:20345479; http://dx.doi.org/10.1111/j.1349-7006.2010.01549.x
- Yang B, Guo M, Herman JG, Clark DP. Aberrant promoter methylation profiles of tumor suppressor genes in hepatocellular carcinoma. Am J Pathol 2003; 163:1101-7; PMID:12937151; http://dx.doi.org/10.1016/S0002-9440(10)63469-4
- Nishida N, Nagasaka T, Nishimura T, Ikai I, Boland CR, Goel A. Aberrant methylation of multiple tumor suppressor genes in aging liver, chronic hepatitis, and hepatocellular carcinoma. Hepatology 2008; 47:908-18; PMID:18161048; http://dx.doi.org/10.1002/hep.22110
- Aran D, Sabato S, Hellman A. DNA methylation of distal regulatory sites characterizes dysregulation of cancer genes. Gen Biol 2013; 14:R21; PMID:23497655; http://dx.doi.org/10.1186/gb-2013-14-3-r21
- Deaton AM, Bird A. CpG islands and the regulation of transcription. Gen Dev 2011; 25:1010-22; PMID:21576262; http://dx.doi.org/10.1101/gad.2037511
- Jones PA. Functions of DNA methylation: islands, start sites, gene bodies and beyond. Nat Rev Genet 2012; 13:484-92; PMID:22641018; http://dx.doi.org/10.1038/nrg3230
- Lucia Radici MB, Rita Crinelli, Mauro Magnani Ubiquitin C gene: Structure, function, and transcriptional regulation. Adv Biosci Biotechnol 2013; 4:1057-62; http://dx.doi.org/10.4236/abb.2013.412141
- Gui T, Dong X, Li R, Li Y, Wang Z. Identification of hepatocellular carcinoma-related genes with a machine learning and network analysis. J Comput Biol 2015; 22:63-71; PMID:25247452; http://dx.doi.org/10.1089/cmb.2014.0122
- Dhillon AS, Hagan S, Rath O, Kolch W. MAP kinase signalling pathways in cancer. Oncogene 2007; 26:3279-90; PMID:17496922; http://dx.doi.org/10.1038/sj.onc.1210421
- Avila MA, Berasain C, Sangro B, Prieto J. New therapies for hepatocellular carcinoma. Oncogene 2006; 25:3866-84; PMID:16799628; http://dx.doi.org/10.1038/sj.onc.1209550
- Yoshida T, Hisamoto T, Akiba J, Koga H, Nakamura K, Tokunaga Y, Hanada S, Kumemura H, Maeyama M, Harada M, et al. Spreds, inhibitors of the Ras/ERK signal transduction, are dysregulated in human hepatocellular carcinoma and linked to the malignant phenotype of tumors. Oncogene 2006; 25:6056-66; PMID:16652141; http://dx.doi.org/10.1038/sj.onc.1209635
- Tsuboi Y, Ichida T, Sugitani S, Genda T, Inayoshi J, Takamura M, Matsuda Y, Nomoto M, Aoyagi Y. Overexpression of extracellular signal-regulated protein kinase and its correlation with proliferation in human hepatocellular carcinoma. Liver Int 2004; 24:432-6; PMID:15482339; http://dx.doi.org/10.1111/j.1478-3231.2004.0940.x
- Deng R, Zhao X, Qu Y, Chen C, Zhu C, Zhang H, Yuan H, Jin H, Liu X, Wang Y, et al. Shp2 SUMOylation promotes ERK activation and hepatocellular carcinoma development. Oncotarget 2015; 6:9355-69; PMID:25823821; http://dx.doi.org/10.18632/oncotarget.3323
- Wang YH, Dong YY, Wang WM, Xie XY, Wang ZM, Chen RX, Chen J, Gao DM, Cui JF, Ren ZG. Vascular endothelial cells facilitated HCC invasion and metastasis through the Akt and NF-kappaB pathways induced by paracrine cytokines. J Exp Clin Cancer Res 2013; 32:51; http://dx.doi.org/10.1186/1756-9966-32-51
- Steelman LS, Chappell WH, Abrams SL, Kempf RC, Long J, Laidler P, Mijatovic S, Maksimovic-Ivanic D, Stivala F, Mazzarino MC, et al. Roles of the Raf/MEK/ERK and PI3K/PTEN/Akt/mTOR pathways in controlling growth and sensitivity to therapy-implications for cancer and aging. Aging 2011; 3:192-222; PMID:21422497; http://dx.doi.org/10.1371/journal.pone.0019862
- Jang CW, Shibata Y, Starmer J, Yee D, Magnuson T. Histone H3.3 maintains genome integrity during mammalian development. Gen Dev 2015; 29:1377-92; PMID:26159997; http://dx.doi.org/10.1101/gad.264150.115
- Quan H, Zhou F, Nie D, Chen Q, Cai X, Shan X, Zhou Z, Chen K, Huang A, Li S, et al. Hepatitis C virus core protein epigenetically silences SFRP1 and enhances HCC aggressiveness by inducing epithelial-mesenchymal transition. Oncogene 2014; 33:2826-35; PMID:23770846; http://dx.doi.org/10.1038/onc.2013.225