ABSTRACT
Epigenome-wide association studies of prenatal exposure to different environmental factors are becoming increasingly common. These studies are usually performed in umbilical cord blood. Since blood comprises multiple cell types with specific DNA methylation patterns, confounding caused by cellular heterogeneity is a major concern. This can be adjusted for using reference data consisting of DNA methylation signatures in cell types isolated from blood. However, the most commonly used reference data set is based on blood samples from adult males and is not representative of the cell type composition in neonatal cord blood. The aim of this study was to generate a reference data set from cord blood to enable correct adjustment of the cell type composition in samples collected at birth. The purity of the isolated cell types was very high for all samples (>97.1%), and clustering analyses showed distinct grouping of the cell types according to hematopoietic lineage. We explored whether this cord blood and the adult peripheral blood reference data sets impact the estimation of cell type composition in cord blood samples from an independent birth cohort (MoBa, n = 1092). This revealed significant differences for all cell types. Importantly, comparison of the cell type estimates against matched cell counts both in the cord blood reference samples (n = 11) and in another independent birth cohort (Generation R, n = 195), demonstrated moderate to high correlation of the data. This is the first cord blood reference data set with a comprehensive examination of the downstream application of the data through validation of estimated cell types against matched cell counts.
Introduction
Epigenome-wide association studies (EWAS) are widely used to investigate the association between DNA methylation variation and a range of phenotypes. This is frequently done in whole blood, which is easy to obtain and readily available for EWAS in established cohorts. Blood consists of a mixture of functionally and developmentally distinct cell types in varying proportions. Different cell types and tissues display different DNA methylation profiles, and blood contains subpopulations of many cell types.Citation1-4 DNA methylation differences between blood samples are strongly influenced both by cellular heterogeneity (i.e., DNA methylation profiles of constituent cell types and their proportions) and the direct or indirect effect associated with the phenotype of interest. Consequently, cell type composition effects will ultimately confound the phenotype associations if not taken into account.
There are different strategies to overcome the problem of cell type heterogeneity in blood samples. The best method is to isolate single cell types and study specific DNA methylation patterns. However, this is laborious and expensive, and is not possible in established cohorts because it requires fresh blood samples. An alternative approach, proposed by Houseman et al.,Citation5 is to use a reference data set consisting of cell type specific DNA methylation signatures. These signatures can be used as surrogates for the distribution of white blood cells, from which one can infer cell type proportions. Specifically, differentially methylated CpGs between cell types are used as markers to identify cell types and to estimate the cell type composition. The estimated cell type percentages can then be included as covariates in the statistical model used to compare DNA methylation in the study groups (e.g., limmaCitation6). For tissues in which the cell type composition is poorly characterized (e.g., placenta, saliva, adipose, or tumor tissue), reference-free methods have been developed to adjust for differences in cell type composition.Citation7,8 However, some of these methods are limited by the potential risk of diminishing important phenotypic variation.Citation9
Cord blood, obtained from the umbilical cord at birth, represents neonatal blood and differs from adult peripheral blood in many aspects. These two blood sources represent very different physiological states and are characterized by different cytokine profiles,Citation10 cell type compositionsCitation11 and levels of immune cell maturity.Citation12-16 There are distinct cell type specific differences in DNA methylationCitation17,18 and gene expressionCitation19,20 between cord blood and peripheral blood.
A reference data set consisting of cell type specific DNA methylation signatures from peripheral whole blood isolated from 6 adult menCitation21 has frequently been used for the deconvolution of both peripheral and cord blood samples.Citation22-25 While this is considered an established method to correct for differences in cell type composition in adult peripheral blood,Citation26 this male adult reference data is not representative of cord blood.Citation27
In parallel to the present study, 2 analogous data sets consisting of cell type specific DNA methylation signatures have become available.Citation28,29 However, these studies did not benchmark their cell type proportions estimates against detailed cell counts. Therefore, the aims of this study were to: 1) characterize cell type composition in cord blood and generate a reference data set for deconvolution of cord blood samples, 2) explore the impact of using cord blood vs. adult peripheral blood reference data sets for estimation of cell type composition in cord blood samples, and 3) validate the estimated proportions by comparing them to detailed cell counts in the reference cord blood samples and in an independent birth cohort.
Results
General description of the data
Eleven fresh cord blood samples collected from neonates delivered at term (37–41 weeks, 6 girls and 5 boys) at Oslo University Hospital were included in the study. Within 12 h of collection of each sample, the following cell types were isolated from the cord blood mononuclear cells (CBMCs) using fluorescence-activated cell sorting (FACS): CD4+ and CD8+ T-lymphocytes, CD19+ B-cells, CD56+ natural killer (NK) cells, CD14+ monocytes and granulocytes (Supplementary Fig. S1). All sorted cells were reanalyzed by FACS to determine purity. Overall, the average purity of cell type populations from all samples was very high (>97.1%, Supplementary Table S1). This is crucial for the application of this data for deconvolution of whole blood samples.
Quantification of cells was performed using 2 approaches: automated 5-part differential cell counting (5-part diff count) and FACS. The 5-part diff count in cord blood was carried out for 9 out of the 11 samples. The 5-part diff counts (i.e., lymphocytes, monocytes, neutrophils, basophils, and eosinophils) are given as relative proportions in Supplementary Table S2. Generally, there was little inter-individual variation in the cell type proportions. Cord blood also contains nucleated red blood cells (NRBCs) and immature granulocytes (IGs), which can represent a substantial proportion of the total nucleated cells in cord blood. The absolute NRBC and IG counts are also given in Supplementary Table S2. The absolute NRBC counts were used to correct the relative proportions of white blood cells.
Distinct cell type specific DNA methylation in cord blood reflects haematopoietic lineage
Genome-wide DNA methylation was measured in all 6 cell types and in cord whole blood from each sample (n = 77) using the Infinium HumanMethylation450 (450K) BeadChip. In addition, technical replicates (n = 7) were included to measure technical variation. This data set is available as an R package (FlowSorted.CordBloodNorway.450K) in Bioconductor and can be specified in the estimateCellCounts function in Minfi. Stringent quality control and probe filtering procedures were applied to minimize technical variation (see Materials and Methods), which resulted in a final data set consisting of 398,133 probes.
Principal component analysis (PCA) showed distinct clustering of the cell types (), reflecting different DNA methylation profiles. Cell types were significantly associated with the first 4 principal components (Kruskal-Wallis test; P = 4.1 × 10−15, P = 1.1 × 10−14, P = 1.2 × 10−13, and P = 1.2 × 10−11, respectively), which explained 75.1% of the total variance (Supplementary Fig. S2). Overall, there were few inter-individual cell type specific differences in DNA methylation, demonstrating consistency in the data and high purity of the isolated cell types. There were no detectable cell type-specific differences between sexes after removal of probes on the sex chromosomes. Furthermore, the technical replicates of cord blood and CD4+ cells (n = 6 and 1, respectively) clustered within their respective cell type groups indicating no batch effects related to the BeadChip or sentrix position (). Not surprisingly, the cell types clustered according to the haematopoietic lineage (i.e., lymphoid or myeloid cells) and/or functional characteristics. The lymphoid cells (CD4+ and CD8+ T-lymphocytes and NK-cells) and the myeloid cells (monocytes and granulocytes) were separated in the PCA. However, the B-cells separated from the other lymphoid-derived cells and were strongly associated with the second principal component.
Figure 1. PCA scatterplot of cell type specific DNA methylation in cord blood. PCA from DNA methylation measurements at 398 133 probes in 6 cell types and cord whole blood isolated from 11 samples (n = 77). The two first principal components are plotted with the proportion of variance explained by each component indicated next to the axis labels. The plot clearly shows distinct clustering of the different cell types and most of the variance in DNA methylation can be attributed to the different cell types. No detectable technical variation (bisulfite conversion and BeadChip) was measured by the technical replicates (n = 7).
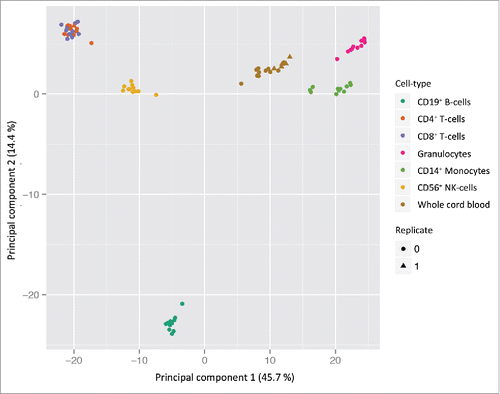
Significant differences in estimated cell type proportions using cord blood and peripheral blood reference data
Next, we explored whether cord blood and adult peripheral bloodCitation21 reference data sets had an impact on the estimation of cell type composition in cord blood samples. These analyses were performed in a large set of cord blood samples (n = 1092) selected from the Norwegian Mother and Child study (MoBa),Citation30-32 permitting robust evaluation of the estimated proportions generated from the 2 reference data sets. Overall, the estimated cell type proportions based on the 2 reference data sets were significantly different for all cell types (Mann-Whitney test, P-values: CD4+ < 2.2 × 10−16, CD8+ < 2.2 × 10−16, NK-cells < 2.2 × 10−16, B-cells < 2.2 × 10−16, monocytes < 2.2 × 10−16 and granulocytes = 1.5 × 10−14). The mean differences between the estimated proportions varied among the cell types, with monocytes and granulocytes showing the greatest mean differences (). Furthermore, the mean differences in the estimated proportions using the cord blood reference data set were greater for CD8+ and CD4+ T-cells, NK-cells, and monocytes.
Figure 2. Estimated cell type proportions in cord blood samples. Box plots of the estimated cell type proportions in cord blood samples selected from an independent birth cohort (MoBa, n = 1092). The blue boxes represent the estimates generated by the present cord blood reference data set and the green boxes represent the estimates generated by the adult reference data set. The boxes signify the upper and lower quartiles, and a black line within the box of each data set denotes the median.
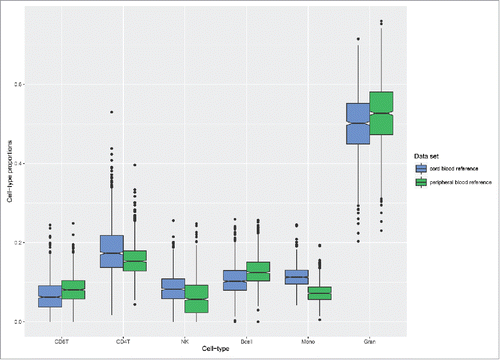
Benchmarking of the estimated cell type proportions to matched cell counts
It is essential to test the ability of the constrained projection model by Houseman et al.Citation5 to accurately predict cell type proportions in cord blood samples. In order to investigate this in detail, we performed 2 sets of analyses and compared the estimated cell type proportions to matched cell counts.
First, we evaluated the present cord blood reference data set and compared the estimated cell type composition in the 11 cord blood samples that were included to matched cell counts. For 9 out of the 11 samples, automated 5-part diff counts were available. As this method only generated counts for lymphocytes, monocytes and granulocytes, the estimated proportions produced by the model were collapsed accordingly to enable comparison (i.e., the estimates from CD4+, CD8+, B-cells, and NK-cells were merged into one lymphocyte category). Overall, there was high consistency between the cell counts and estimates for the 3 cell type categories with small differences in the mean proportions () and high within-sample correlation (Pearson r = 0.93, 0.77, and 0.97 for lymphocytes, monocytes, and granulocytes, respectively). The model seemed to slightly overestimate the lymphocytes and monocytes, while underestimating the granulocytes.
Figure 3. Comparison of estimated cell types and matched cell counts. A) Scatter plots of estimated cell type proportions and matched cell counts in the reference cord blood samples from which 5-part diff counts were available (n = 9). The estimated cell type proportions of CD4+, CD8+, NK, and B-cells were collapsed to one lymphocyte category to enable comparison with the cell count data. B) Scatter plots of estimates and matched cell counts in an individual birth cohort (Generation R, n = 195). Smoothening lines represents the linear model.
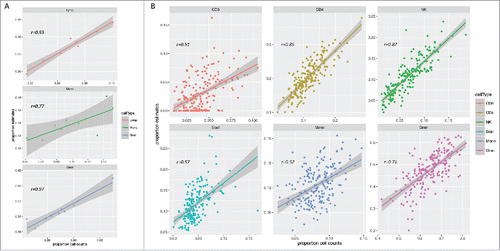
Although the model predicted the cell type composition with high accuracy in the reference cord blood samples, it is also essential to replicate this in an independent cohort to assess the reproducibility of the reference data across birth cohorts. To test this we applied the prediction model to samples selected from the Generation R studyCitation33,34 (n = 195), from which cord blood 450K data and matched detailed cell counts were available for the 6 cell types. The results from these analyses revealed moderate to high correlation between estimates and cell counts (Pearson r: CD8+ = 0.51, CD4+ = 0.85, NK = 0.87, B-cells = 0.57, monocytes = 0.52 and granulocytes = 0.71, ). However, discrepancies in these data were greater compared to the reference cord blood samples, but in agreement with a previously published validation study on the equivalent adult peripheral blood reference set.Citation26 In general, the model produced precise estimates of the CD8+ cells, whereas the remaining cell types were moderately correlated moderately overestimated, except the granulocytes, which were underestimated (Supplementary Fig. S3). The generated estimates were consistent across the 2 birth cohorts tested (MoBa and Generation R).
Discussion
We have generated a cord blood reference data set consisting of cell type specific DNA methylation signatures using the Infinium 450K BeadChip, which can be applied to the deconvolution of cord blood samples in EWAS. Cord blood was collected from term neonates delivered by healthy women. Cell types were isolated from the samples using FACS, which is considered the gold standard for the identification and isolation of cell populations within heterogeneous cell samples. Fluorescent labeling and FACS was optimized to ensure accurate separation of cells, which resulted in very pure cell fractions. This is crucial for correct downstream deconvolution of cord blood samples and prediction of cell type proportions with a high degree of sensitivity and specificity obtained from the constrained projection algorithm.Citation5
PCA revealed distinct clustering of cell types with low inter-individual variance. The cell types clustered according to hematopoietic lineage, providing biological evidence of a robust data set. The B-cells separated from the other lymphoid-derived cells by the second principal component. This is in agreement with similar studies in cord bloodCitation28 and adult peripheral blood. Citation21 B- and T-cells have different functions in the immune system, but also different developmental origins (T-cells from the thymus and B-cells from the bone marrow).Citation35 These 2 factors likely explain some of the variation.
Cord blood also contains nucleated red blood cells (NRBCs) in addition to white blood cells. NRBCs, precursors of red blood cells, are normally only found in fetuses and neonates. These cells contain a nucleus and can represent a substantial proportion of the total nucleated cells in cord blood. Whereas the 2 analogous cord blood data setsCitation28,29 also contain sorted NRBCs, this was not included in the present study except for quantification. de Goede et al.Citation28 reported cross-contamination of isolated cell types by NRBCs in their data set due to physical interactions. Although we cannot exclude the possibility of cross-contamination in our sorted cells, we consider this to be a negligible source of variation for the downstream application of the data since this potentially represents only a tiny fraction of the sorted cells. To our knowledge, there is very limited information about the DNA methylation signature of these cells besides the basic description given based on the small number of samples included in the 2 studies (n = 5 and 4, respectively).Citation28,29 In these studies, the global DNA methylation deviated from the normally observed bimodal distribution and displayed intermediate DNA methylation. In addition, NRBCs also showed considerably more inter-individual variability than the other cell types in both studies. Whether this is caused by variable purity of the sorted cells is not known since purity measurements are unavailable. However, NRBCs are known to rapidly decline from the bloodstream almost immediately after birthCitation36, which might affect the composition of these cells (i.e., inter-individual differences in function and maturation) depending on the time of separation and DNA extraction. Consequently, the estimation of NRBCs based on the few samples in these reference data sets should be interpreted with caution. In fact, the NRBC estimates generated by the model in the MoBa samples we tested were notably higher than the normal reference values in cord blood reported in different birth cohorts.Citation37-39 Finally, the estimated proportion of NRBCs in the cord blood samples in our study based on the 2 other data setsCitation28,29 were higher than the cell counts (Supplementary Fig. S4). Future projects based on a larger data set of NRBCs is needed in order to fully reveal the potential influence of variations in NRBCs on differences in cord blood DNA methylation analysis.
Cord blood and adult peripheral blood reflect very different physiological states and comprise cells with different morphology, maturity, and functions. In general, the immune systems at birth and during adult life are very different.Citation40 The innate responses are most prominent early in life and the adaptive immune system has not gained the experience necessary for optimal memory responses.Citation35 Studies have also identified DNA methylation differences between cord blood and peripheral blood samples in childhood.Citation17,41,42 Although this probably also reflects age-associated DNA methylation and differences in cell type composition, it suggests that adult reference data sets are not suitable surrogates for cord blood. Comparisons of the estimated cell types in cord blood using both the present cord blood and adult peripheral blood reference data sets revealed significant differences for all cell types. This is in agreement with 2 recent studies demonstrating poor correlation of cell type estimates using adult reference data and comparable cell counts in cord bloodCitation27 and moderate prediction of isolated cord blood cell types.Citation29
In line with the discussion above, it is essential to do a comprehensive examination of the constrained projection model to accurately predict cell type proportions in cord blood samples. A validation of the prediction model in the reference cord blood samples showed high correlation of estimates and matched cell counts. In theory, a high correlation is expected since the estimates are generated based on cell types sorted from these samples. Nevertheless, this is an important test and the results serve as a control and validation of both the reference data and the prediction model.
We tested our reference data in an independent cohort (the Generation R study), from which both detailed cell counts and cord blood 450K data were available. These analyses revealed moderate to high correlation of the estimates and cell counts. In general, the estimates generated by the model were higher than the cell counts for all cell types, except for granulocytes. This is in agreement with a previous validation of an adult reference data set.Citation26 The observed bias in the estimates could reflect differences in other variables, such as maternal age, gestational age, and ethnicity between the reference and validation cohorts. In addition, any difference in the fluorescent labeling of cells and performance of the conjugated antibodies between the 2 platforms would contribute to the observed differences. This is particularly true for the NK-cells, which are defined differently in the reference data set and the validation cohort. Specifically, NK-cells in the reference data set are not depleted for CD3, and thus include a fraction of CD3+CD56+ (NKT-cells). Further, the NK-cells in the validation cohort are depleted for NKT-cells, but defined as being CD16+ and/or CD56+. Most of the CD16+ cells are also CD56+, but some are not. Unfortunately, it is not possible to unravel the impact these differences will have on the results presented here.
Our reference data only allowed prediction of the 6 cell types included, which accounted for approximately 90% of the white blood cells in the validation cohort. In addition, NRBCs also contribute to cord blood DNA methylation, but NRBC counts were not available for these samples. The prediction algorithm assumes that the observed DNA methylation values in the cord blood samples are a mixture of the cell types in the reference data set. From a statistical point of view, it is difficult to infer how the algorithm deals with the missing cell types. This will depend on the cell type specific DNA methylation at the positions differentiating the reference data, which might be different from global levels. Hence, if the missing cell types are most similar to cell types other than granulocytes this will result in underestimation of granulocytes. In addition, granulocytes are a heterogeneous population possessing functional and phenotypic heterogeneity, which likely involves differences in DNA methylation.Citation16 It is not possible to dissect how the different granulocyte subsets contribute to the global DNA methylation in these samples and whether or not the reference samples are capturing the variation between individuals in the validation cohort. Furthermore, the cell types in the reference data set were isolated from CBMCs to remove red blood cells, which otherwise would have interfered with the FACS. Isolation of CBMCs involves a density gradient centrifugation step that also depletes the majority of granulocytes, as these typically sediment on top of the red blood cells. In theory, this results in a biased depletion of the granulocyte subsets. Unfortunately, it is not possible to explore if there are residual granulocytes in the CBMC fraction in our data, or any of the equivalent reference data sets. However, the generated estimates were consistent across the 2 birth cohorts tested.
The present cord data set is based on 6 major cell types sorted by FACS. Although this technology yielded high purity of the sorted cell types, this is only highly accurate for the specific cell type surface markers used in this study. Hence, deconvolution of cord blood samples is limited by the selected surface markers. However, variations in minor cell subpopulations, which could be associated with a phenotypeCitation43 are not accounted for. The complete cell type composition in whole blood (cord and peripheral) is currently unknown, and future single-cell analysis will be required to specifically exhaust the potential variability in blood cells.
In summary, we have developed a reference data set of cell type specific DNA methylation signatures in cord blood, which can be applied for deconvolution of cord blood samples in EWAS. Cord blood is different from adult peripheral blood, and comparison of the estimates generated by the present cord blood reference data set and an adult reference data set revealed significant differences for all the cell types measured. Importantly, this data set has been tested by comparing cell type estimates against matched cell counts both in the cord blood reference samples and in a validation cohort. This is the first cord blood reference data set with a comprehensive examination of the downstream application of the data through validation of estimated cell types against matched cell counts.
Material and methods
Recruitment of cord blood samples
We recruited 11 healthy pregnant women with uncomplicated pregnancies at the ABC (Alternative Birth Care) unit at Oslo University Hospital after informed written consent. Exclusion criteria were pregnancy-related complications (e.g., preeclampsia, diabetes, BMI > 35) or chronic disease (e.g., cancer, autoimmune), smoking during pregnancy and medication intake. After delivery (5 male and 6 female), fresh umbilical cord blood samples were collected into EDTA tubes (10 ml) by puncture of the umbilical cord vein. The cord blood samples were analyzed within 12 h. Only cord blood from term deliveries was included (delivery gestational week 37; gestational age was determined by ultrasound second trimester screening). The Regional Committee for Medical and Health Research Ethics of South-Eastern Norway approved the study.
Cell preparation, immunological staining, and flow cytometric sorting
The cord blood samples were diluted in PBS pH 7.4 (Gibco, Life Technologies) with 2% Fetal Bovine Serum (Life Technologies, USA) filtered with a sterile 70 µm cup-type filter (Beckman Coulter, BC) and subjected to Lymphoprep (Stem Cell Technologies, Norway) density-gradient centrifugation within 12 h after collection. The cells were then spun down at 300 g for 10 min and resuspended in 500 ml of medium (RPMI) containing 50 μl human FcR blocking agent (Miltenyi Biotechnologies, Germany) and incubated on ice for 20-30 min. The number of cells was estimated on a Sysmex K4500 by adding 10 μl of the cell suspension to 190 μl 5% FCS RPMI. The number of stained cells for sorting ranged from 22-240 million. All untreated umbilical cord blood was analyzed with a Sysmex Lavender Top Management System for 5-part differentiation. The cells were incubated for 30 min with monoclonal conjugated antibodies (the concentrations are presented in Supplementary Table S3). Prior to sorting, the antibodies were titrated to find the optimal antibody ratio. For optimization of flow cytometric sorting, fluorescence-minus-one (FMO) was carried out for all populations, except granulocytes. Erythrocytes were removed by incubation of the cell suspension for 45 min on ice with 10 ml of 1x BD Lysing solution (BD Biosciences, Belgium) and further excluded in a SSC vs. CD45 dot plot. The cells were washed by pelleting at 300 g/10 min and carefully resuspended in 1 ml of 5% FCS RPMI. The cells were kept on ice sheltered from light until sorting on a BD FACS Aria. In order to minimize loss of cells, instrument compensations were carried out using antibody labeled Anti-Mouse Ig, κ coated beads (BD CompBeads, BD Biosciences, Norway). The samples were kept at 4°C and cells were sorted into cold polypropylene tubes (VWR, Norway) to prevent adherence. The following populations were sorted: granulocytes, CD56+ NK-cells, CD19+ B-cells, CD8+ and CD4+ T- lymphocytes, and CD14+ monocytes. Sorted cells were re-analyzed and the purity calculated based on CD marker against total number of events in the MNC and granulocyte gate in the SSC-a vs. CD45 Chrome Orange-A dot (Supplementary Table S1). Of note, the CD56+ NK-cells were not depleted for CD3 and likely contain a small fraction of CD3+CD56+ (NKT-cells). The sorted populations were centrifuged at 500 g at 4°C for 10 min. The pellets were resuspended in 180 µl supernatant and lysed with 20 μl Proteinase K solution (Qiagen, Germany) and 200 μl Buffer AL (Lysis buffer, Qiagen, Germany) and stored at −80°C.
Immunophenotyping of white blood cell subsets in the samples selected from the Generation R study is described elsewhere.Citation44
DNA extraction and bisulphite conversion
DNA from sorted cell types and cord blood was extracted using the Blood and Cell culture DNA mini kit (QIAGEN). Bisulphite conversion of DNA was completed using the EZ-96 DNA Methylation-Gold Kit (Zymo Research, USA) according to the manufacturer's instructions. All the samples (n = 84 in total) were converted on the same plate, thereby minimizing potential batch effects related to bisulphite conversion.
DNA methylation analysis
DNA methylation was assessed using the Infinium HumanMethylation450 BeadChip according to manufacturer's instructions (Illumina, USA). In line with the main downstream application of the data (i.e., deconvolution of cord blood samples), the sorted cell types and cord blood samples were always hybridized on the same BeadChip to minimize potential batch effects within cell types. Technical replicates were also included to allow measurement of technical variation between BeadChips and sentrix position. To test this, whole blood and CD4+ cells isolated from one sample were replicated on all BeadChips.
Data processing and general description of cell type specific DNA methylation
All analyses were carried out using the R programming language (http://www.r-project.org/). iDat files were preprocessed in MinfiCitation45 and normalized using SWAN.Citation46 Stringent quality control and probe filtering procedures were applied to minimize technical variation. The data were filtered to remove probes with detection P-values > 0.01 in any sample, those mapping to the X and Y chromosomes, and cross-reactive probes.Citation47 This resulted in a total data set consisting of 398,133 probes.
Principal component analysis was performed to examine the data set for strong signals in the DNA methylation values that were related to any of the measured traits. A non-parametric Kruskal-Wallis test was used to test for association of the principal components to the respective traits.
Deconvolution of cord blood samples and validation of the estimated cell type composition
Two study populations were used for testing and validation of the cord blood reference data set: The Norwegian Mother and Child Cohort Study (MoBa) and the Generation R study. MoBa is a prospective population-based pregnancy cohort study conducted by the Norwegian Institute of Public Health.Citation31 Participants were recruited from all over Norway from 1999-2008, and 40.6% of delivering women in this period consented to participation. The cohort now includes 114,500 children, 95,200 mothers and 75,200 fathers. Blood samples were obtained from both parents at recruitment and from mothers and children (umbilical cord) at birth.Citation48 The Generation R Study is a population-based prospective cohort study from fetal life onwards in Rotterdam, the Netherlands, which has been previously described in detail.Citation34 In total, n = 9,778 mothers with a delivery date from April 2002 until January 2006 were enrolled in the study. The study has been approved by the Medical Ethical Committee of the Erasmus MC, University Medical Center Rotterdam, and written consent was obtained for all participants. All children were born between April 2002 and January 2006. The cohort is being followed up until young adulthood. Epigenome-wide DNA methylation was measured using the Infinium HumanMethylation450 BeadChip according to manufacturer's instructions, on DNA extracted from cord blood samples from 969 children of European ancestry. Detailed cell counts were available in 195 of these.
Deconvolution of cord blood samples and the contribution of each cell type were estimated using the method of Houseman et al.Citation5 implemented in the estimateCellCount function in the Minfi R package.Citation45 We chose to increase the number of nProbes in the estimateCellCount function from 50 to 250 since this resulted in a slightly better correlation of the generated estimates and cell counts. Deconvolution of cord blood samples was also done using the adult reference dataCitation21 set in the FlowSorted.Blood.450K R package as a comparison. A Mann-Whitney U test was used to compare mean estimates per cell type in cord blood samples selected from the Norwegian Mother and Child cohort (MoBa, n = 1092), which were generated based on the present cord blood and the adult reference data sets.
Validation of the present reference data set was done by comparing of the estimated cell type proportions against matched cell counts in both the reference cord blood samples (n = 11) and in cord blood samples selected from the Generation R study (n = 195). Correlation of the cell type estimates and matched cell counts was calculated using the Pearson correlation test.
Disclosure of potential conflicts of interest
No potential conflicts of interest were disclosed.
KEPI_A_1214782_supplementary_data.zip
Download Zip (226.3 KB)Acknowledgments
This work was supported by the Norwegian Institute of Public Health, Southern and Eastern Norway Regional Health Authority, and The Norwegian Research Council. We thank the women who donated cord blood to the reference data set and the midwives at the ABC clinic at Oslo University Hospital for the recruitment and collection of the cord blood samples. We thank Martin Hammerø for excellent assistance in the laboratory. Kasper Hansen contributed to the development and submission of the R package FlowSorted.CordBloodNorway.450K to Bioconductor. The Infinium HumanMethylation450 BeadChips were processed at the Norwegian Microarray Consortium (Oslo University hospital, Radium). The Norwegian Mother and Child Cohort Study is supported by the Norwegian Ministry of Health and the Ministry of Education and Research, NIH/NIEHS (contract no N01-ES-75558), NIH/NINDS (grant no.1 UO1 NS 047537-01 and grant no.2 UO1 NS 047537-06A1). The present study is also supported by the Norwegian Research Council/Human Biobanks and Health (grant no. 221097). We are grateful to all the families in Norway who are participating in this on-going MoBa cohort study. The Generation R Study is conducted by the Erasmus Medical Center in close collaboration with the School of Law and Faculty of Social Sciences of the Erasmus University Rotterdam, the Municipal Health Service Rotterdam area, Rotterdam, the Rotterdam Homecare Foundation, Rotterdam and the Stichting Trombosedienst & Artsenlaboratorium Rijnmond (STAR-MDC), Rotterdam. We gratefully acknowledge the contribution of children and parents, general practitioners, hospitals, midwives and pharmacies in Rotterdam. The study protocol was approved by the Medical Ethical Committee of the Erasmus Medical Center, Rotterdam. Written informed consent was obtained for all participants. The generation and management of the Illumina 450K methylation array data (EWAS data) for the Generation R Study was executed by the Human Genotyping Facility of the Genetic Laboratory of the Department of Internal Medicine, Erasmus MC, the Netherlands. The EWAS data was funded by a grant to VWJ from the Netherlands Genomics Initiative (NGI)/Netherlands Organization for Scientific Research (NWO) Netherlands Consortium for Healthy Aging (NCHA; project nr. 050-060-810), by funds from the Genetic Laboratory of the Department of Internal Medicine, Erasmus MC. We thank Mr. Michael Verbiest, Ms. Mila Jhamai, Ms. Sarah Higgins, Mr. Marijn Verkerk and Dr. Lisette Stolk for their help in creating the EWAS database. The Generation R Study is made possible by financial support from the Erasmus Medical Center, Rotterdam, the Erasmus University Rotterdam and the Netherlands Organization for Health Research and Development. V.W.J. received a grant from the Netherlands Organization for Health Research and Development (VIDI 016.136.361) and a Consolidator Grant from the European Research Council (ERC-2014-CoG-64916). J.F.F. has received funding from the European Union's Horizon 2020 research and innovation program under grant agreement No 633595 (DynaHEALTH).
References
- Lister R, Pelizzola M, Dowen RH, Hawkins RD, Hon G, Tonti-Filippini J, Nery JR, Lee L, Ye Z, Ngo Q-M, et al. Human DNA methylomes at base resolution show widespread epigenomic differences. Nature 2009; 462:315-22; PMID:19829295; http://dx.doi.org/10.1038/nature08514
- Ji H, Ehrlich LIR, Seita J, Murakami P, Doi A, Lindau P, Lee H, Aryee MJ, Irizarry RA, Kim K, et al. Comprehensive methylome map of lineage commitment from haematopoietic progenitors. Nature 2010; 467:338-42; PMID:20720541; http://dx.doi.org/10.1038/nature09367
- Liu H, Liu X, Zhang S, Lv J, Li S, Shang S, Jia S, Wei Y, Wang F, Su J, et al. Systematic identification and annotation of human methylation marks based on bisulfite sequencing methylomes reveals distinct roles of cell type-specific hypomethylation in the regulation of cell identity genes. Nucleic Acids Res 2015; 44:75-94; PMID:26635396; http://dx.doi.org/10.1093/nar/gkv1332
- Consortium EP, I D, Guigó Serra R, Birney E. An integrated encyclopedia of DNA elements in the human genome. Nature 2012; 489:57-74; PMID:22955616; http://dx.doi.org/10.1038/nature11247
- Houseman E, Accomando WP, Koestler DC, Christensen BC, Marsit CJ, Nelson HH, Wiencke JK, Kelsey KT. DNA methylation arrays as surrogate measures of cell mixture distribution. BMC Bioinformatics 2012; 13:86; PMID:22568884; http://dx.doi.org/10.1186/1471-2105-13-86
- Smyth GK. Linear models and empirical bayes methods for assessing differential expression in microarray experiments. Stat Appl Genet Mol Biol 2004; 3: Article3-25; PMID:16646809; http://dx.doi.org/10.2202/1544-6115.1027
- Zou J, Lippert C, Heckerman D, Aryee M, Listgarten J. Epigenome-wide association studies without the need for cell type composition. Nat Meth 2014; 11:309-11; PMID:24464286; http://dx.doi.org/10.1038/nmeth.2815
- Houseman EA, Molitor J, Marsit CJ. Reference-free cell mixture adjustments in analysis of DNA methylation data. Bioinformatics 2014; 30:1431-9; PMID:24451622; http://dx.doi.org/10.1093/bioinformatics/btu029
- McGregor K, Bernatsky S, Colmegna I, Hudson M, Pastinen T, Labbe A, Greenwood CMT. An evaluation of methods correcting for cell type heterogeneity in DNA methylation studies. Genome Biol 2016; 17:1; PMID:26753840; http://dx.doi.org/10.1186/s13059-016-0935-y
- Nitsche A, Zhang M, Clauss T, Siegert W, Brune K, Pahl A. Cytokine profiles of cord and adult blood leukocytes: differences in expression are due to differences in expression and activation of transcription factors. BMC Immunol 2007; 8:18; PMID:17764543; http://dx.doi.org/10.1186/1471-2172-8-18
- Chirumbolo S, Ortolani R, Veneri D, Raffaelli R, Peroni D, Pigozzi R, Colombatti M, Vella A. Lymphocyte phenotypic subsets in umbilical cord blood compared to peripheral blood from related mothers. Cytometry B Clin Cytom 2011; 80B:248-53; PMID:21692178; http://dx.doi.org/10.1002/cyto.b.20588
- Elahi S, Ertelt JM, Kinder JM, Jiang TT, Zhang X, Xin L, Chaturvedi V, Strong BS, Qualls JE, Steinbrecher KA, et al. Immunosuppressive CD71+ erythroid cells compromise neonatal host defence against infection. Nature 2013; 504:158-62; PMID:24196717; http://dx.doi.org/10.1038/nature12675
- Verneris MR, Miller JS. The phenotypic and functional characteristics of umbilical cord blood and peripheral blood natural killer cells. Br J Haematol 2009; 147:185-91; PMID:19796267; http://dx.doi.org/10.1111/j.1365-2141.2009.07768.x
- López MC, Palmer BE, Lawrence DA. Phenotypic differences between cord blood and adult peripheral blood. Cytometry B Clin Cytometry 2009; 76B:37-46; PMID:18642326; http://dx.doi.org/10.1002/cyto.b.20441
- Ssemaganda A, Kindinger L, Bergin P, Nielsen L, Mpendo J, Ssetaala A, Kiwanuka N, Munder M, Teoh TG, Kropf P, et al. Characterization of neutrophil subsets in healthy human pregnancies. PLoS One 2014; 9:e85696; PMID:24551035; http://dx.doi.org/10.1371/journal.pone.0085696
- Scapini P, Cassatella MA. Social networking of human neutrophils within the immune system. Blood 2014; 124:710-9; PMID:24923297; http://dx.doi.org/10.1182/blood-2014-03-453217
- Martino DJ, Tulic MK, Gordon L, Hodder M, Richman TR, Metcalfe J, Prescott SL, Saffery R. Evidence for age-related and individual-specific changes in DNA methylation profile of mononuclear cells during early immune development in humans. Epigenetics 2014; 6:1085-94; PMID:21814035; http://dx.doi.org/10.4161/epi.6.9.16401
- Jacoby M, Gohrbandt S, Clausse V, Brons NH, Muller CP. Interindividual variability and co-regulation of DNA methylation differ among blood cell populations. Epigenetics 2014; 7:1421-34; PMID:23151460; http://dx.doi.org/10.4161/epi.22845
- Merkerova M, Vasikova A, Bruchova H, Libalova H, Topinka J, Balascak I, Sram RJ, Brdicka R. Differential gene expression in umbilical cord blood and maternal peripheral blood. Eur J Haematol 2009; 83:183-90; PMID:19500137; http://dx.doi.org/10.1111/j.1600-0609.2009.01281.x
- Jiang H, van de Ven C, Baxi L, Satwani P, Cairo MS. Differential gene expression signatures of adult peripheral blood vs cord blood monocyte-derived immature and mature dendritic cells. Exp Hematol 2009; 37:1201-15; PMID:19647780; http://dx.doi.org/10.1016/j.exphem.2009.07.010
- Reinius LE, Acevedo N, Joerink M, Pershagen G, Dahlén S-E, Greco D, Söderhäll C, Scheynius A, Kere J. Differential DNA methylation in purified human blood cells: implications for cell lineage and studies on disease susceptibility. PLoS One 2012; 7:e41361; PMID:22848472; http://dx.doi.org/10.1371/journal.pone.0041361
- Koestler DC, Marsit CJ, Christensen BC, Accomando W, Langevin SM, Houseman EA, Nelson HH, Karagas MR, Wiencke JK, Kelsey KT. Peripheral blood immune cell methylation profiles are associated with nonhematopoietic cancers. Cancer Epidemiol Biomarkers Prev 2012; 21:1293-302; PMID:22714737; http://dx.doi.org/10.1158/1055-9965.EPI-12-0361
- Liu Y, Aryee MJ, Padyukov L, Fallin MD, Hesselberg E, Runarsson A, Reinius L, Acevedo N, Taub M, Ronninger M, et al. Epigenome-wide association data implicate DNA methylation as an intermediary of genetic risk in rheumatoid arthritis. Nat Biotechnol 2013; 31:142-7; PMID:23334450; http://dx.doi.org/10.1038/nbt.2487
- Yuan T, Jiao Y, de Jong S, Ophoff RA, Beck S, Teschendorff AE. An integrative multi-scale analysis of the dynamic DNA Methylation Landscape in Aging. PLoS Genet 2015; 11:e1004996; PMID:25692570; http://dx.doi.org/10.1371/journal.pgen.1004996
- Langevin SM, Houseman EA, Accomando WP, Koestler DC, Christensen BC, Nelson HH, Karagas MR, Marsit CJ, Wiencke JK, Kelsey KT. Leukocyte-adjusted epigenome-wide association studies of blood from solid tumor patients. Epigenetics 2014; 9:884-95; PMID:24671036; http://dx.doi.org/10.4161/epi.28575
- Koestler DC, Christensen BC, Karagas MR, Marsit CJ, Langevin SM, Kelsey KT, Wiencke JK, Houseman EA. Blood-based profiles of DNA methylation predict the underlying distribution of cell types: A validation analysis. Epigenetics 2013; 8:816-26; PMID:23903776; http://dx.doi.org/10.4161/epi.25430
- Yousefi P, Huen K, Quach H, Motwani G, Hubbard A, Eskenazi B, Holland N. Estimation of blood cellular heterogeneity in newborns and children for epigenome-wide association studies. Environ Mol Mutagen 2015; 56:751-8; PMID:26332589; http://dx.doi.org/10.1002/em.21966
- de Goede OM, Razzaghian HR, Price EM, Jones MJ, Kobor MS, Robinson WP, Lavoie PM. Nucleated red blood cells impact DNA methylation and expression analyses of cord blood hematopoietic cells. Clin Epigenetics 2015; 7:95; PMID:26366232; http://dx.doi.org/10.1186/s13148-015-0129-6
- Bakulski KM, Feinberg JI, Andrews SV, Yang J, Brown S, McKenney S, Witter F, Walston J, Feinberg AP, Fallin MD. DNA methylation of cord blood cell types: Applications for mixed cell birth studies. Epigenetics 2016; 28:1-8
- Magnus P, Irgens LM, Haug K, Nystad W, Skjærven R, Stoltenberg C, MoBa Study Group. Cohort profile: the Norwegian Mother and Child Cohort Study (MoBa). Int J Epidemiol 2006; 35:1146-50; PMID:16926217; http://dx.doi.org/10.1093/ije/dyl170
- Magnus P, Birke C, Vejrup K, Haugan A, Alsaker E, Daltveit AK, Handal M, Haugen M, Høiseth G, Knudsen GP, et al. Cohort Profile Update: The norwegian mother and child cohort study (MoBa). Int J Epidemiol 2016; 45:382-8; PMID:27063603; http://dx.doi.org/10.1093/ije/dyw029
- Rønningen KS, Paltiel L, Meltzer HM, Nordhagen R, Lie KK, Hovengen R, Haugen M, Nystad W, Magnus P, Hoppin JA. The biobank of the Norwegian mother and child cohort Study: A resource for the next 100 years. Eur J Epidemiol 2006; 21:619-25; PMID:17031521; http://dx.doi.org/10.1007/s10654-006-9041-x
- Kruithof CJ, Kooijman MN, van Duijn CM, Franco OH, de Jongste JC, Klaver CCW, Mackenbach JP, Moll HA, Raat H, Rings EHHM, et al. The Generation R Study: Biobank update 2015. Eur J Epidemiol 2014; 29:911-27; PMID:25527369; http://dx.doi.org/10.1007/s10654-014-9980-6
- Jaddoe VWV, van Duijn CM, Franco OH, van der Heijden AJ, van IIzendoorn MH, de Jongste JC, van der Lugt A, Mackenbach JP, Moll HA, Raat H, et al. The Generation R Study: design and cohort update 2012. - PubMed - NCBI. Eur J Epidemiol 2012; 27:739-56; PMID:23086283; http://dx.doi.org/10.1007/s10654-012-9735-1
- Basha S, Surendran N, Pichichero M. Immune responses in neonates. Expert Rev Clin Immunol 2014; 10:1171-84; PMID:25088080; http://dx.doi.org/10.1586/1744666X.2014.942288
- Hermansen M. Nucleated red blood cells in the fetus and newborn. Arch Dis Child Fetal Neonatal Ed 2001; 84:F211-5; PMID:11320052; http://dx.doi.org/10.1136/fn.84.3.F211
- Glasser L, Sutton N, Schmeling M, Machan JT. A comprehensive study of umbilical cord blood cell developmental changes and reference ranges by gestation, gender and mode of delivery. J Perinatol 2015; 35:469-75; PMID:25634517; http://dx.doi.org/10.1038/jp.2014.241
- Suman FR, Raj RSS, Priyathersini N, Rajendran R, Rajendran R, Ramadoss U. Biological reference interval for hematological profile of umbilical cord blood: A study conducted at a tertiary care centre in South India. J Clin Diagn Res 2015; 9:SC07-9; PMID:26557584
- Chang Y-H, Yang S-H, Wang T-F, Lin T-Y, Yang K-L, Chen S-H. complete blood count reference values of cord blood in taiwan and the influence of gender and delivery route on them. Pediatr Neonatol 2011; 52:155-60; PMID:21703558; http://dx.doi.org/10.1016/j.pedneo.2011.03.007
- Comans-Bitter WM, de Groot R, van den Beemd R, Neijens HJ, Hop WCJ, Groeneveld K, Hooijkaas H, Van Dongen JJM. Immunophenotyping of blood lymphocytes in childhoodReference values for lymphocyte subpopulations. J Pediatr 1997; 130:388-93; PMID:9063413; http://dx.doi.org/10.1016/S0022-3476(97)70200-2
- Herbstman JB, Wang S, Perera FP, Lederman SA, Vishnevetsky J, Rundle AG, Hoepner LA, Qu L, Tang D. Predictors and consequences of global DNA methylation in cord blood and at three years. PLoS One 2013; 8:e72824; PMID:24023780; http://dx.doi.org/10.1371/journal.pone.0072824
- Martino D, Loke Y, Gordon L, Ollikainen M, Cruickshank MN, Saffery R, Craig JM. Longitudinal, genome-scale analysis of DNA methylation in twins from birth to 18 months of age reveals rapid epigenetic change in early life and pair-specific effects of discordance. Genome Biol 2013; 14:R42; PMID:23697701; http://dx.doi.org/10.1186/gb-2013-14-5-r42
- Bauer M, Linsel G, Fink B, Offenberg K, Hahn AM, Sack U, Knaack H, Eszlinger M, Herberth G. A varying T cell subtype explains apparent tobacco smoking induced single CpG hypomethylation in whole blood. Clin Epigenetics 2015; 7:1; PMID:25628764; http://dx.doi.org/10.1186/s13148-015-0113-1
- van den Heuvel D, Jansen MAE, Dik WA, Bouallouch-Charif H, Zhao D, van Kester KAM, Smits-te Nijenhuis MAW, Kolijn-Couwenberg MJ, Jaddoe VWV, Arens R, et al. Cytomegalovirus- and epstein-barr virus–induced T-cell expansions in young children do not impair naive T-cell populations or vaccination responses: the Generation R study. J Infect Dis 2015; 213:233-42; PMID:26142434; http://dx.doi.org/10.1093/infdis/jiv369
- Aryee MJ, Jaffe AE, Corrada-Bravo H, Ladd-Acosta C, Feinberg AP, Hansen KD, Irizarry RA. Minfi: a flexible and comprehensive Bioconductor package for the analysis of Infinium DNA methylation microarrays. Bioinformatics 2014; 30:1363-9; PMID:24478339; http://dx.doi.org/10.1093/bioinformatics/btu049
- Maksimovic J, Gordon L, Oshlack A. SWAN: Subset-quantile within array normalization for Illumina Infinium HumanMethylation450 BeadChips. Genome Biol 2012; 13:R44; PMID:22703947; http://dx.doi.org/10.1186/gb-2012-13-6-r44
- Chen Y-A, Lemire M, Choufani S, Butcher DT, Grafodatskaya D, Zanke BW, Gallinger S, Hudson TJ, Weksberg R. Discovery of cross-reactive probes and polymorphic CpGs in the Illumina Infinium HumanMethylation450 microarray. Epigenetics 2014; 8:203-9; PMID:23314698; http://dx.doi.org/10.4161/epi.23470
- Paltiel L, Anita H, Skjerden T, Harbak K, Bækken S. The biobank of the norwegian mother and child cohort study–present status. Norsk Epidemiologi 2014; 24:29-35; http://dx.doi.org/10.5324/nje.v24i1-2.1755