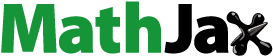
ABSTRACT
Epigenetic control of human immunodeficiency virus-1 (HIV-1) genes is critical for viral integration and latency. However, epigenetic changes in the HIV-1-infected host genome have not been well characterized. Here, we report the first large-scale epigenome-wide association study of DNA methylation for HIV-1 infection. We recruited HIV-infected (n = 261) and uninfected (n = 117) patients from the Veteran Aging Cohort Study (VACS) and all samples were profiled for 485,521 CpG sites in DNA extracted from the blood. After adjusting for cell type and clinical confounders, we identified 20 epigenome-wide significant CpGs for HIV-1 infection. Importantly, 2 CpGs in the promoter of the NLR family, CARD domain containing gene 5 (NLRC5), a key regulator of major histocompatibility complex class I gene expression, showed significantly lower methylation in HIV-infected subjects than in uninfected subjects (cg07839457: t = −6.03, Pnominal = 4.96 × 10−9; cg16411857: t = −7.63, Pnominal = 3.07 × 10−13). Hypomethylation of these 2 CpGs was replicated in an independent sample (GSE67705: cg07839457: t = −4.44, Pnominal = 1.61 × 10−5; cg16411857: t = −5.90; P = 1.99 × 10−8). Methylation of these 2 CpGs in NLRC5 was negatively correlated with viral load in the 2 HIV-infected samples (cg07839457: P = 1.8 × 10−4; cg16411857: P = 0.03 in the VACS; and cg07839457: P = 0.04; cg164111857: P = 0.01 in GSE53840). Our findings demonstrate that differential DNA methylation is associated with HIV infection and suggest the involvement of a novel host gene, NLRC5, in HIV pathogenesis.
Introduction
Epigenetic mechanisms are involved in human immunodeficiency virus-1 (HIV-1) integration into the host genomeCitation1-3 and viral latency.Citation4-6 Epigenetic modifications regulate gene transcription and expression without changing the DNA sequence. Histone deacetylation has been shown to be important for the quiescence of HIV gene expression in HIV-1-infected T cells.Citation7,8 In contrast, DNA hypermethylation is associated with viral latency, likely by inhibiting transcriptional initiation of the integrated provirus and inhibiting viral post-integration reactivation from latency.Citation7,9 Therefore, epigenetic reprogramming regulates the virus life cycle at multiple stages and modulates the interaction between the viral and host genomes. However, epigenetic characterization of the host genome in HIV-infected vs. uninfected individuals has not been thoroughly conducted.
Epigenetic control specifically targets genes involved in cell cycle progression, senescence, survival, inflammation, and immunity. The hallmark of HIV infection is the depletion of peripheral CD4+ T cells, which compromises host defense. HIV-1 infection may initiate or influence epigenetic processes in host cells and manipulate host genome epigenetic reprogramming.Citation10 For example, HIV-1 increases DNA methyltransferase expression in HIV-1-infected CD4+ T cells, which is responsible for de novo methylation.Citation11,12 DNA methylation of host genes in response to HIV-1 potentially impacts HIV-1 transcription and replication. Therefore, characterizing DNA methylation in the host genome may provide insight into the pathogenesis of HIV infection.
Candidate gene/region studies of DNA methylation associated with HIV infection have shown that CpG sites in the promoter of FOXP3 were significantly less methylated in HIV-infected patients than in uninfected patients in colonic mucosa and blood samples.Citation13 A previous study reported that CpGs in the IL2 promoter were completely methylated in naïve CD4+ T cells and significantly demethylated in memory CD4+ T cells during chronic HIV infection,Citation14 indicating that alterations in DNA methylation occur in different subpopulations of CD4+ T cells during HIV infection. Interestingly, a recent study revealed hypomethylation in the region encoding the human leukocyte antigen locus in chronic HIV-infected patients.Citation15 These findings indicate that methylation should be examined at the epigenome level.
Epigenome-wide analysis of DNA methylation is a robust and unbiased approach for investigating the association of DNA methylation with complex disease phenotypes.Citation16-18 However, the epigenome-wide DNA methylation patterns in HIV-1 infection are largely unknown. Recently, a study reported 4,679 differentially methylated regions in the genomes of a single monozygotic twin pair in which one sibling was HIV-infected, while the other was HIV-uninfected.Citation19 This study found that 2 genes, IGFBP6 and SATB2, were hypermethylated in the HIV-infected individual compared to the uninfected individual in 8 patients. This study used a small sample size, and thus studies in larger samples are necessary.
The goal of this study was to identify differentially methylated CpGs in the host genome between HIV-infected and uninfected subjects and to determine whether HIV-associated methylation sites are correlated with viral load (VL). Here, we report an epigenome-wide association study (EWAS) of HIV-infected and uninfected individuals selected from a well-established HIV cohort, the Veterans Aging Cohort Study (VACS). We replicated the biologically meaningful findings in an independent publicly available sample (GSE67705, http://www.ncbi.nlm.nih.gov/geo/query/acc.cgi?acc=GSE67705). We also examined the relationship between HIV-associated CpG methylation and VL in HIV-infected patients from the VACS sample and an independent publically available sample (GSE67751, http://www.ncbi.nlm.nih.gov/geo/query/acc.cgi?acc=GSE67751).
Results
EWAS revealed significant differential methylation CpG sites between HIV-1-infected and uninfected patients
To correct for technical bias and biological confounders, we adapted a recently developed comprehensive pipeline for EWAS to detect CpG sites related to HIV infection.Citation20 We first examined whether cell type composition was associated with methylation effects in HIV infection. Using a well-established method,Citation21,22 we estimated the cell type proportion in each sample. We compared the EWAS results from models with and without cell type correction. shows the volcano plots with the P-value and coefficient at a CpG site between HIV-infected and uninfected patients without and with correction for cell type composition. A model without cell type correction revealed 405 differential CpG sites associated with HIV infection (). The quantile–quantile plot showed significant inflation (Supplementary Fig S1). An EWAS corrected for cell type proportion identified 20 significant CpG sites associated with HIV infection (). After correcting for cell type composition, we found that none of the 20 HIV-associated CpG sites were correlated with any of the 6 cell types (P > 0.05) (Supplementary Fig. S2). These results suggest that cell type strongly affects EWAS results for HIV infection and that it is essential to correct for cell types in EWAS analysis. We then examined whether demographic, clinical, and batch variables confounded the EWAS analysis. Using control probe intensities designed for the Illumina Infinium HumanMethylation450 Beadchip (450K array), we detected multiple confounders in addition to cell types, including batch, age, sex, smoking, and cell type (Supplementary Fig. S3A). After adjusting for these confounding factors, no correlation between residual methylation and confounders, except smoking status, was observed (Supplementary Fig. S3B), further suggesting that our analytic strategy sufficiently corrected for methylation signals from different cell types and other confounders. Batch showed no confounding effects in our analytic pipeline. Smoking status was adjusted in the model.
Figure 1. Effect of cell composition on DNA methylation. Volcano plots show the coefficient at a CpG site vs. -log10(P-value). Blue circles indicate hypomethylated CpG sites, while red circles indicate hypermethylated CpG sites. (A) Volcano plot showing association results without correction for cell types. (B) Volcano plot with correction for 6 cell types in the blood.
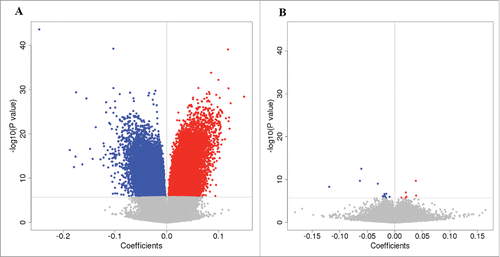
As shown in , 20 CpG sites reached epigenome-wide significance for HIV infection (false discovery rate, FDR q < 0.05). Significant CpGs, relationship with gene region, and effect sizes are presented in . Consistent with previous findings, global methylation in HIV-infected individuals was lower than in uninfected individuals. Among the 20 significant CpG sites, 14 CpGs were hypomethylated, while 6 CpGs were hypermethylated in the HIV-infected samples. Eighteen of 20 CpGs were methylated at an intermediate level (0.2 < β < 0.8). Methylation at 14 significant CpG sites differed by more than 5% (5–25%) between HIV-infected and uninfected subjects. The average methylation at each significant CpG in these 14 sites in HIV-infected and uninfected groups is shown in . The absolute fold-changes in these 14 CpGs in HIV-infected and uninfected groups were 1.1–2.0, reflecting moderate to large effects of methylation alteration in HIV-infected individuals relative to uninfected subjects (). Notably, CpGs located in islands presented larger fold-changes between HIV-infected and uninfected groups compared to CpGs near islands.
Figure 2. Epigenome-wide association analysis of 437,722 CpG sites in 378 samples. A. Manhattan plot showing chromosome location of 22 autosomes (X-axis) and –log10(P-value) for each CpG (Y-axis). Blue line indicates the threshold for significance (FDR q < 0.05). Red line indicates the significance level after Bonferroni correction (Padj < 0.05). Two CpG sites in the promoter of NLRC5 on chromosome 16 reached epigenome-wide significance (cg07839457: P = 4.96E-09; cg16411857: P = 2.01E-10). B. Quantile-quantile plot showing observed vs. expected –log10(P-value) for association at all CpG sites (λ = 1.17). A regression model was applied for EWAS, adjusted for batch, cell types, and other clinical confounding factors.
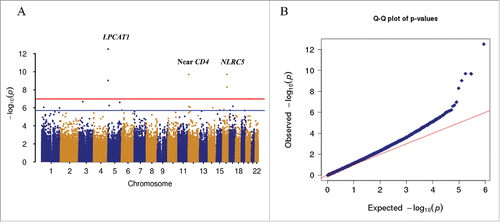
Figure 3. Epigenome-wide significant differences at 14 CpG sites. A. Box plots of methylation β values for 14 significant CpG sites with average methylation difference greater than 5% between HIV-infected and uninfected individuals. B. Log2 value of fold-changes for 14 significant CpG sites in HIV-infected vs. HIV-uninfected patients.
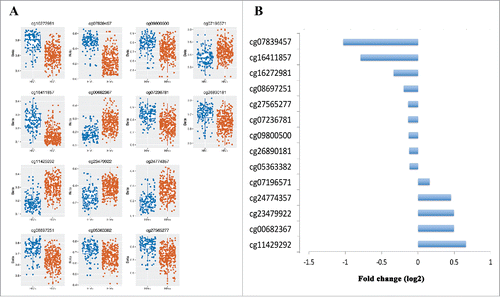
Table 1. Epigenome-wide association analysis identified 20 CpG sites for HIV infection in the Veteran Aging Cohort Study.
Five of 20 CpGs located in 3 genes remained significant after more stringent Bonferroni correction (Pnominal < 10−7, Bonferroni P < 0.05) (cg16272981, cg16411857, cg11429292, cg08697251, cg07839457). Among these 3 genes, a novel gene for HIV infection, NLR family, CARD domain containing gene 5 (NLRC5), showed the largest effect size on methylation alteration and biological relevant to HIV infection. NLRC5 was recently identified as a major histocompatibility complex (MHC) class I transcriptional activator through genome-wide gene expression profiling of human cell lines expressing either wild type or mutant NLRC5.Citation23 We found that 2 CpGs in the promoter of NLRC5, cg07839457 and cg16411857, showed lower methylation levels in HIV-infected subjects compared to uninfected subjects (cg07839457: t = −6.03, Pnominal = 4.96 × 10−9 and cg16411857: t = −6.59, Pnominal = 2.01 × 10−10) (). The distribution of methylation levels in these 2 CpGs was significantly different between the HIV-infected and uninfected groups (). The methylation fold-changes between HIV-infected and uninfected subjects for cg07839457 and cg16411857 were 0.49 and 0.58, respectively. These two CpGs were located approximately 350 bp apart in the TSS1500 within a CpG island (cg16411857) or surrounding a CpG island (cg07839457) in NLRC5. Histone modification H3K27ac was enriched in this region. Interestingly, multiple transcription factors, such as TEF-1, AP-2rep, and MAX, are located within this region.Citation24-26 These data suggest that methylation alterations at these 2 CpGs may be involved in dysregulation of NLRC5 expression in HIV infection. However, the relationship between NLRC5 expression and HIV infection has not been investigated.
Figure 4. DNA methylation in NLRC5 were associated with HIV infection in 2 independent data sets. A. Regional Manhattan plot of DNA methylation association analysis with HIV infection on chromosome 16, gene structure of NLRC5, and methylation level of 21 CpG sites in NLRC5 between HIV-infected and uninfected groups in the Veteran Aging Cohort Study (VACS). B. Methylation distributions of cg07839457 and cg16411857 in HIV-infected patients and uninfected patients in the VACS sample. C. Methylation level of 21 CpG sites in NLRC5 between HIV-infected and uninfected groups in the public dataset GSE66705. D. Methylation distributions of cg07839457 and cg16411857 in HIV-infected patients and uninfected patients in GSE66705.
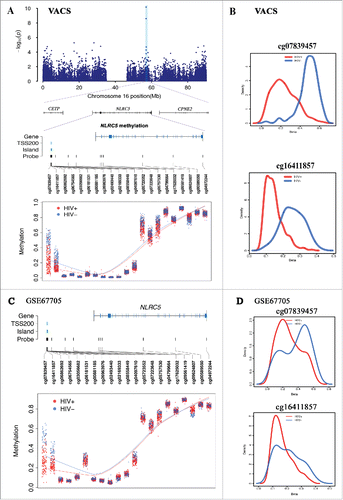
Another significant CpG site, cg11429292, which is 10 kb upstream of the CD4 gene, was hypermethylated in HIV-infected patients compared to uninfected patients (t = 6.58, Pnominal = 2.04 × 10−10). CD4 encodes the primary receptor for HIV-1 and regulates T helper cell function. Two highly significant CpGs (cg16272981 and cg08697251) in LPCAT1 were differentially methylated between HIV-infected and uninfected subjects, but their relationship with HIV infection is unknown.
A number of other differential CpG sites reached an FDR q < 0.05, but were not retained after more stringent Bonferroni correction (). These CpGs may be biologically relevant, as they were located on genes involved in HIV-1 pathogenesis. For example, we observed reduced DNA methylation of cg07236781 in the 1st Exon of RUNX3 (t = −5.02, FDR q = 0.03). RUNX3 is a member of the RUNX family and is a critical transcriptional regulator of CD4+ T cells. The RUNX protein complex may bind to the HIV LTR and play a role in viral latency.Citation27 Another interesting differential methylated CpG site, cg07196571 in the promoter of sorting nexin 22 SNX22 (SNX22), may be involved in HIV-1 pathogenesis. Methylation of CpG in the promoter of SNX22 showed 4% greater methylation in HIV-infected individuals than uninfected individuals in the VACS sample. A previous study showed that knockdown of SNX22 significantly increased CD4 expression in HIV-1-infected T cells, suggesting that SNX22 is involved in HIV-mediated CD4 downregulation.Citation28
Previously reported CpG sites in candidate genes, IL2, IGFBP6, and SATB2, of HIV infection showed nominal significance, but were not retained after statistical correction. The CpG cg25065535, located in TSS1500 of IL2, was hypermethyalted in HIV-infected individuals compared to uninfected indiviudals (t = 2.78, Pnominal = 0.006), which agrees with the results of a previous study.Citation14 We also detected hypermethylation of IGFBP6 (cg04791129: t = 2.13, Pnominal = 0.03) and SATB2 (cg05358815: t = 2.93, Pnominal = 0.003) in HIV-infected indiviudals compared to uninfected individuals, which is also consistent with the previous study.Citation19 None of these 2 CpG sites reached epigenome-wide significance.
Replication of methylation alterations in NLRC5 in HIV infection (GSE67705)
Because the methylation alterations in the promoter of NLRC5 showed large differences between HIV-infected and uninfected samples, we sought to replicate these findings in an independent sample. This dataset included 142 HIV-infected and 44 uninfected samples from 2 different cohorts. Using a similar analytic pipeline, we found significant differential methylation of the same 2 CpGs in the promoter of NLRC5 in this sample (cg07839457: t = −4.44, Pnominal = 1.61 × 10−5; cg16411857: t = −5.90; P = 1.99 × 10−8). Site cg07839457 showed 15% lower methylation and cg16411857 showed 13% lower methylation in the HIV-infected samples compared to the HIV-uninfected samples (), which are consistent with the findings for NLRC5 in the VACS sample. The results for 21 CpGs of NLRC5 in the VACS sample and GSE67705 sample are shown in Supplemental . These findings further support the important role of NLRC5 in HIV pathogenesis.
The other 3 significant CpG sites identified in the VACS samples showed no significant association with HIV infection in the replication sample GSE67705. The top CpG site in LPCAT1, cg16272981, showed nominal significance (t = −2.4, P = 0.02). CpG sites associated with HIV infection in the VACS sample, cg11429292 and cg00682367, did not reach nominal significance levele (P > 0.05).
Methylation of NLRC5 was correlated with HIV-1 viral load
Among the 20 HIV-associated CpGs, 3 CpGs showed a significant association with VL in the VACS samples, including 2 CpG sites in NLRC5 (cg07839457: P = 1.80 × 10−4; cg16411857: P = 0.03 × 10−6). shows the correlation of these 2 CpGs with unsuppressed VL (>75) in the VACS samples. Methylation of significant CpG sites in LPCAT1 was not correlated with VL. These results suggest that alterations in DNA methylation, particularly in the promoters of NLRC5, are also involved in the control of HIV-1 replication in the blood. In an independent public data set from the Multicenter AIDS Cohort Study (MACS; GSE53840), methylation of cg07839457 and cg16411857 on NLRC5 was negatively correlated with viral load in HIV-infected samples (P = 0.04 for cg07839457 and P = 0.01 for cg16411857), supporting the findings for the VACS samples. shows the correlations of these 2 CpGs with VL in the unsupressed samples. Despite the limited sample size, these results indicate that methylation changes in NLRC5 play a role in HIV-1 replication.
Figure 5. Correlation between methylation levels of cg07839457 and cg16411857 in NLRC5 and HIV-1 viral load in the 2 independent samples. Negative correlation with HIV-1 viral load in HIV-infected individuals (VL > 75) (r2 = 0.13, P = 1.8 × 10−4 for cg07839457 and r2 = 0.04, P = 0.03 for cg16411857) in the VACS (A) and in an independent publicly available sample (GSE53840) (VL > 75) (r2 = 0.18, P = 0.04 for cg07839457 and r2 = 0.15, P = 0.01 for cg16411857) (B).
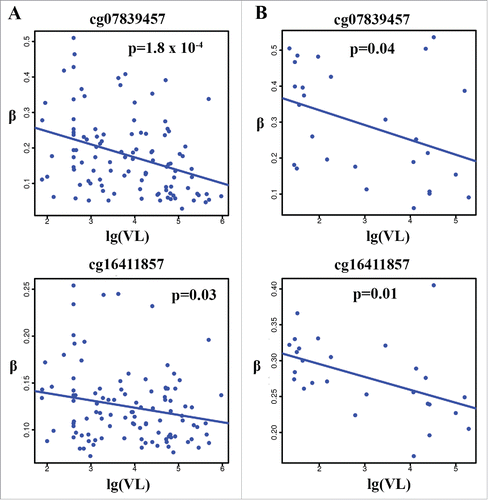
Discussion
HIV-1 infection is known to increase DNA methyltransferase activity in CD4+ T cells in vitro.Citation11,12 However, this hypothesis has not been evaluated on an epigenome-wide scale in humans. Here, we report the epigenome-wide characterization of DNA methylation for HIV infection. Using an EWAS approach, we identified 20 CpGs that were differentially methylated between HIV-infected and uninfected samples. Specifically, hypomethylation of 2 CpGs (cg07839457 and cg16411857) in the promoter of NLRC5 was associated with HIV infection. The associations of these 2 CpG sites in NLRC5 with HIV infection were replicated in an independent sample (GSE67705). Furthermore, methylation of these 2 CpGs in NLRC5 was negatively correlated with VL, which was also replicated in an independent cohort (MACS). Although differential DNA methylation of the candidate genes, IL2, IGFBP6, and SATB2, did not reach epigenome-wide significance, we found similar trends in methylation alteration as previous reports.Citation14,19 Our findings suggest that alterations in DNA methylation are associated with the host genomic response to HIV-1 infection and support a role for NLRC5 in HIV-1 pathogenesis.
We observed significant alterations in DNA methylation in the HIV-infected host epigenome. Most of the significant CpGs showed lower methylation levels in HIV-infected subjects compared to uninfected subjects. Most significant CpGs showed intermediate levels of methylation, while a small number of CpGs showed extremely low or high methylation. The CpGs that were significantly associated with HIV infection were near or within genes known to be involved in immune activation, which is central to the pathophysiology associated with chronic viral infection. These findings support that mild to moderate methylation of these genes may have large impacts on the regulation of immune and inflammatory functions.Citation29 Although the mechanism of how HIV-1 impacts the host methylome is unknown, methylation alteration may be an epigenetic consequence of the integration of HIV-1 DNA into the infected host genome. This interpretation is based on the results of a study showing that foreign DNA fragment insertion can have important functional consequences that are beyond the site of foreign DNA insertion.Citation30 The effects of foreign DNA on the host genome were previously observed in studies in which tumor viral DNA such as adenovirus type 12 was inserted and remarkably altered methylation; such epigenetic genome-wide consequences play an important role in oncogenesis.Citation31 Recently, insertion of a 5.6-kb plasmid in the human genome altered methylation in 3,791 of 480,000 CpG sites examined compared to non-transgenomic clones, suggesting epigenome destabilization of foreign DNA in human cells.Citation32 Therefore, it is reasonable to speculate that HIV-1 DNA insertion into the host genome can lead de novo methylation and decrease host epigenome stability. Future studies examining the epigenetic effects of HIV DNA insertion in HIV-infected cells will provide insight into methylation alteration in the HIV-infected genome observed in this study.
Among the significant HIV-associated CpG sites, the most interesting CpG sites were within the promoter of NLRC5. Both CpG sites were moderately under-methylated in HIV-infected subjects compared to uninfected subjects in 2 independent samples. NLR proteins constitute a protein family associated with the adaptive cellular immune response and play an important role in host recognition and the defense against pathogens.Citation33-35 NLRC5 is located on chromosome 16 and is expressed in a wide variety of cell types and tissues.Citation36 NLRC5 was recently identified as a specific transactivator of MHC class I genes and was found to interact with major class II transactivators with a similar MHC promoter-bound factorCitation36-39 Precisely how NLRC5 is involved in HIV-1 infection remains unknown. However, a recent study demonstrated that hypermethylation of the promoter of NLRC5 downregulated NLRC5 expression in cancer cells.Citation40 The expression of NLRC5 and MHC class I genes was highly correlated with human cancers. Interestingly, methylation of NLRC5 was also inversely associated with MHC class I gene expression.Citation40 These results suggest that NLRC5 is a target for cancer immune evasion. Our findings of the hypomethylation of 2 CpGs in HIV-infected subjects suggests that upregulation of NLRC5 expression enhances the transcription of MHC class I genes, which involves the mechanisms of host defense in HIV infected genome.
Additionally, methylation of NLRC5 was inversely correlated with HIV-1 viral load in the VACS cohort, which was validated in an independent cohort, suggesting that NLRC5 is involved in viral replication. This gene also plays a role in the cytokine response and antiviral immunity by inhibiting nuclear factor (NF)-κB activation and negatively regulating type I interferon (IFN) signaling pathways.Citation41,42 NLRC5 negatively regulates the NF-κB and type I IFN signaling pathways and NF-κB and IFN upregulate HIV-1 replication.Citation41 The methylation alterations in NLRC5 may be associated HIV replication via the upregulation of NF-κB and interferon pathways. However, the cause-consequence relationship between methylation of NLRC5 and HIV infection is unknown. Hypomethylation of NLRC5 may result from chronic HIV infection, but further studies are needed to clarify this.
The top significant signals in the EWAS were from LPCAT1, a newly identified protein coding gene on chromosome 5p15.33.Citation43 Two CpG sites (cg16272981 and cg08697251) in the gene body of LPACT1 were significantly hypomethylated in HIV-infected subjects compared to uninfected controls. However, these 2 CpG sites were not associated with HIV infection in the replication sample and neither of the 2 CpG sites was associated with VL. A previous study suggested that LPCAT1 is involved in the regulation of inflammatory lipids.Citation44 The role of LPACT1 in HIV infection is unknown. Our findings suggest that the role of LPACT1 in HIV disease should be further investigated.
We found nominally significant differential methylation in IL2, IGFBP6, and SATB2 between HIV-infected and uninfected groups. A previous in vitro study reported the hypermethylation of IL2 in CD4+ T cells from HIV-infected non-controllers than in CD4+ T cells from uninfected individuals.Citation14 We observed the same trend in hypermethylation for HIV infection in our patient-based sample. Similarly, we observed hypermethylation of IGFBP6 and SATB2 for HIV infection, which is consistent with the results of a previous twin study that included one HIV-infected twin and one uninfected twin.Citation19 These genes did not reach epigenome-wide significance, which may be because of the greater heterogeneity in our patient-based sample compared to laboratory-controlled studies and the twin sample.
One limitation of EWAS is cell type heterogeneity in the blood samples, as epigenetic changes are correlated with cellular differentiation into specific lineages.Citation45 Cell compositions significantly differ between HIV-infected and uninfected subjects and can be particularly problematic in an EWAS. However, isolation of a specific cell type is often not feasible when evaluating large clinical samples because the total cell numbers are low, particularly in cryopreserved specimens. Our results showed that the statistical approach for estimating and correcting the cell composition can substantially reduce the confounding effects of cell type differences and improve the signals associated with the underlying pathophysiology. This approach has been applied in EWAS of other complex diseases.Citation17,46,47 Notably, despite the more conservative and comprehensive analytic approach used for EWAS, the results still showed slight inflation, suggesting that other unknown confounding factors were not completely corrected. However, the findings for NRLC5 in 2 independent samples indicate that this gene is associated with HIV infection rather than a confounding effect.
A limitation of this study was the inability to differentiate between methylation signals involved in HIV-1-induced pathophysiological processes and cell type-specific methylation. Here, we treated cell type as a confounding factor to limit the generation of spurious signals. However, a reduced CD4+ T cell count is a clinical marker of HIV progression. The adjusted cell composition may suppress HIV-related methylation signals, which may be also methylation signatures of CD4+ T cells.Citation48 This may explain why known genes involved in HIV pathogenesis, such as CCR5, did not reach epigenome-wide significance. Future independent studies including longitudinal studies of patients starting antiretroviral therapy are necessary to replicate these findings. We were also unable to examine the functional roles of the identified methylation sites in HIV infection. Future studies investigating the regulatory mechanism of methylation alteration in the identified genes will provide additional insight into the epigenetic mechanisms.
The study had several strengths: 1) This is the first EWAS with sufficient power to characterize altered DNA methylation patterns of the host genome associated with HIV disease; 2) Biologically meaningful methylation of NLRC5 with large effect sizes was replicated in an independent sample; 3) Methylation in NLRC5 was associated with HIV VL in 2 independent samples. The differential methylation signals observed highlight the potential of EWAS to explore the pathophysiology of HIV disease in humans and is an important step toward improving the understanding of the mechanisms involved in host epigenetic regulation of HIV infection.
Materials and methods
Sample description
The study was approved by the Human Research Protection Program of Yale University and Institutional Research Board committee at the Connecticut Veteran Healthcare System, West Haven Campus. All subjects in the VACS sample were informed of the study and each subject provided written consent for participation.
VACS dataset for EWAS
All subjects were participants in the VACS, an observational, prospective longitudinal study of HIV-infected and age, race/ethnicity, sex, and site-matched uninfected veterans in care at 8 US. Department of Veterans Affairs medical centers across the US.Citation49 Total white blood cell counts and CD4+ and CD8+ T cell subsets were enumerated at time of peripheral blood sample collection. HIV VL was measured per standard of care by PCR as copies per milliliter. We selected 261 HIV-infected and 117 uninfected patients for this study. Genomic DNA was extracted from whole blood using a standard method. Demographic and clinical characteristics are presented in .
Table 2. Demographic and clinical characteristics of HIV-infected and HIV-uninfected patients from the Veteran Aging Cohort Study.
EWAS replication data set (GSE67705)
A dataset from the study “Methylome-wide analysis of chronic HIV infected patients and healthy controls” (GSE67705) (http://www.ncbi.nlm.nih.gov/geo/query/acc.cgi?acc=GSE67705) was used as a replication sample. A total of 186 samples (HIV-infected: 142; HIV-uninfected: 44) were available for analysis. HIV-infected samples were obtained from the CNS HIV Antiretroviral Therapy Effects Research (CHARTER) study (http://www.charterresource.ucsd.edu/). An additional 35 HIV-infected samples were included in the GSE67705. All subjects were male, of European descent, and 25 to 65 y old. HIV-infected subjects were on cART and VL was controlled at less than 20 copies/mL. Because of the limited sample size, this data set was used to replicate the significant CpG sites related to HIV infection identified in the VACS sample.
Multicenter AIDS cohort study (MACS, GSE53840)
This dataset was obtained from a public database (http://www.ncbi.nlm.nih.gov/geo/query/acc.cgi?acc=GSE53840). This cohort included 109 HIV-infected men with a mean age of 52 years (31–68 years). Average CD4+ and CD8+ T cell percentages were 30% (2–54%) and 46% (19–79%), respectively. Non-detectable VL was defined as a VL lower than 10 copies/mL as reported in the database. Genomic DNA was extracted from peripheral blood mononuclear cells. HIV-uninfected subjects were not included in this cohort.Citation50 Thus, this data set was used as a validation sample to examine the correlations between HIV-associated significant CpG sites and VL identified from the VACS.
DNA methylation profiling, cell type estimation, and batch effects in the VACS sample
DNA was extracted from whole-blood samples using PaxGene collection tubes (QIAGEN, Hilden, Germany) and FlexiGene DAN extraction kits (QIAGEN). Genomic DNA methylation profiling was conducted at the Yale Center for Genomic Analysis (https://medicine.yale.edu/keck/ycga/) using the Illumina Infinium HumanMethylation450 Beadchip (450K; Illumina, San Diego, CA, USA). To reduce batch effects, all samples were randomly placed on each array. The same scientist (G Wang at the Yale Center for Genomic Analysis) who was blinded to the HIV status conducted the microarray experiment. Quality control and probe normalization were performed as previously described by Lehne et al.Citation20 Detailed information regarding this procedure is presented in the supplementary material.
Using an algorithm developed by Houseman et al.,Citation48,54 we applied the 600 probes from the 450K array, which included the signature methylation sites of 6 cell types (CD4+ T cells, CD8+ T cells, NK T cells, B cells, monocytes, and granulocytes) in the blood,Citation48 and estimated the cell composition in each sample using the minfi R package. To validate the accuracy of the estimated cell composition, we compared the correlation between the methylation-estimated and clinical laboratory reports of CD4+ and CD8+ T cell percentage in HIV-infected samples. The methylation-estimated CD4+ and CD8+ percentages were highly correlated with the laboratory measurements (r = 0.76, P < 0.0001 for CD4+, r = 0.77, P < 0.0001 for CD8+) (Supplementary Fig. S4A and B). As expected, the percentage of CD4+ T cells was significantly lower (t = −12.1, P = 1.03 × 10−25) and the percentage of CD8+ T cells was significantly higher (t = 16.6, P = 1.38 × 10−45) in HIV-infected patients than in HIV-uninfected patients (Supplementary Fig. S4C). Therefore, the 6 methylation-estimated cell type proportions were adjusted as covariates in the subsequent EWAS analytic model.
To further detect whether batch and other clinical variables confounded HIV infection in our sample, we estimated the correlation between each potential confounder and the first 30 principal components of control probes designed for the 450K array (Fig. S3A). We then performed a linear regression to adjust significant confounders. Fig. S3B showed no significant correlation between principal components on residual methylation and confounders except smoking status, which was covariate in the final regression model. Only male samples were analyzed to avoid sex bias.
Statistical analysis
To adjust for significant global confounding factors in the VACS sample as described by Lehne et al.,Citation20 we conducted 2 regression analyses to determine the associations between methylome-wide CpGs and HIV infection. The following steps were performed to correct for global covariations that may confound specific methylation in HIV infection.
We first estimated residual β using regression model (Equation1(1)
(1) ):
(1)
(1)
We then performed a second PCA on the resulting regression residual β values and regressed out the first 5 PCs to further control for confounders in the regression model (Equation2(2)
(2) ).
(2)
(2)
Significance was set at a false discovery error (FDR) < 0.05. To further confirm the correction of global confounders, we performed Pearson correlation analysis between the first 30 PCs on residual methylation from model (1) and batch, demographic, clinical, and 6 cell type confounders.
To validate our analytic pipeline, we performed an EWAS for age because age-associated DNA methylation has been well established.Citation51,52 EWAS revealed 5,332 CpG sites for age in the VACS sample. The top significant aging-related CpG site was cg16867657on ELOVL2 (Supplementary Fig. S5); this gene is well known to be related to aging.Citation53,54 Other aging-related genes involved in differential methylation, such as FHL2 and KLF14, were replicated among the most significant CpG sites in our sample.Citation51 Supplemental Table 2 lists the top 50 significant CpG sites associated with age in our sample. These results validated our data collection, processing, normalization, and analysis.
`To examine whether HIV-associated CpG methylation identified by EWAS is correlated with HIV-1 viral replication, we performed linear regression for significant CpG sites derived from model (2) with VL, adjusted for age, sex, and race. We excluded HIV-1 suppressors with VL <75 in the VACS sample. The relationship between methylation at significant CpG sites and HIV-1 load identified in the VACS cohort was confirmed in an independent cohort from GSE53840 as described above.
Data availability
Demographic, clinical variables, and methylation for the VACS sample were submitted to the GEO dataset (GSE77696).
Disclosure of potential conflicts of interest
No potential conflicts of interest were disclosed.
Author contributions
Xinyu Zhang was responsible for bioinformatics data processing and statistical analysis. Amy Justice provided DNA samples and clinical data and contributed to the interpretation of results and manuscript preparation. Zuoheng Wang was involved in statistical modeling and data analysis. Hongyu Zhao contributed to the analytic strategy and manuscript preparation. Ying Hu was involved in establishing the bioinformatics pipeline. Guilin Wang conducted the microarray-based DNA methylation assay. Eric Johnson contributed to manuscript preparation. Richard Sutton interpreted the results and assisted with manuscript preparation. Brinda Emu contributed to data interpretation and manuscript preparation. John Krystal contributed to data interpretation and manuscript preparation. Ke Xu was responsible for the study deign, study protocol, sample preparation, data analysis, interpretation, and manuscript preparation.
KEPI_A_1221569_supplemental_data.zip
Download Zip (6.8 MB)Acknowledgments
The authors appreciate the support of the Veteran Aging Study Cohort and Yale Center of Genomic Analysis.
Funding
The work was funded by the National Institute on Drug Abuse [R03 DA039745, K12 DA000167, R01 DA038632, R01 DA042691]; National Institute on Alcoholism and Alcohol Abuse [R21AA022870]; and National Center for Post-traumatic Stress Disorder.
References
- Ay E, Banati F, Mezei M, Bakos A, Niller HH, Buzas K, Minarovits J. Epigenetics of HIV infection: promising research areas and implications for therapy. AIDS Rev 2013; 15(3):181-8; PMID:24002202
- Paschos K, Allday MJ. Epigenetic reprogramming of host genes in viral and microbial pathogenesis. Trends Microbiol 2010; 18(10):439-47; PMID:20724161; http://dx.doi.org/10.1016/j.tim.2010.07.003
- Harbers K, Schnieke A, Stuhlmann H, Jahner D, Jaenisch R. DNA methylation and gene expression: endogenous retroviral genome becomes infectious after molecular cloning. Proc Natl Acad Sci U S A 1981; 78(12):7609-13; PMID:6950402; http://dx.doi.org/10.1073/pnas.78.12.7609
- Kumar A, Darcis G, Van Lint C, Herbein G. Epigenetic control of HIV-1 post integration latency: implications for therapy. Clin Epigenetics 2015; 7(1):103; PMID:26405463; http://dx.doi.org/10.1186/s13148-015-0137-6
- Park J, Lim CH, Ham S, Kim SS, Choi BS, Roh TY. Genome-wide analysis of histone modifications in latently HIV-1 infected T cells. AIDS 2014; 28(12):1719-28; PMID:24762674; http://dx.doi.org/10.1097/QAD.0000000000000309
- Bednarik DP, Cook JA, Pitha PM. Inactivation of the HIV LTR by DNA CpG methylation: evidence for a role in latency. EMBO J 1990; 9(4):1157-64; PMID:2323336
- Kauder SE, Bosque A, Lindqvist A, Planelles V, Verdin E. Epigenetic regulation of HIV-1 latency by cytosine methylation. PLoS Pathog 2009; 5(6):e1000495; PMID:19557157; http://dx.doi.org/10.1371/journal.ppat.1000495
- Tripathy MK, Abbas W, Herbein G. Epigenetic regulation of HIV-1 transcription. Epigenomics 2011; 3(4):487-502; PMID:22126207; http://dx.doi.org/10.2217/epi.11.61
- Blazkova J, Trejbalova K, Gondois-Rey F, Halfon P, Philibert P, Guiguen A, Verdin E, Olive D, Van Lint C, Hejnar J, et al. CpG methylation controls reactivation of HIV from latency. PLoS Pathog 2009; 5(8):e1000554; PMID:19696893; http://dx.doi.org/10.1371/journal.ppat.1000554
- Deeks SG, Walker BD. Human immunodeficiency virus controllers: mechanisms of durable virus control in the absence of antiretroviral therapy. Immunity 2007; 27(3):406-16; PMID:17892849; http://dx.doi.org/10.1016/j.immuni.2007.08.010
- Mikovits JA, Young HA, Vertino P, Issa JP, Pitha PM, Turcoski-Corrales S, Taub DD, Petrow CL, Baylin SB, Ruscetti FW. Infection with human immunodeficiency virus type 1 upregulates DNA methyltransferase, resulting in de novo methylation of the gamma interferon (IFN-gamma) promoter and subsequent downregulation of IFN-gamma production. Mol Cell Biol 1998; 18(9):5166-77; PMID:9710601; http://dx.doi.org/10.1128/MCB.18.9.5166
- Fang JY, Mikovits JA, Bagni R, Petrow-Sadowski CL, Ruscetti FW. Infection of lymphoid cells by integration-defective human immunodeficiency virus type 1 increases de novo methylation. J Virol 2001; 75(20):9753-61; PMID:11559808; http://dx.doi.org/10.1128/JVI.75.20.9753-9761.2001
- Pion M, Jaramillo-Ruiz D, Martinez A, Munoz-Fernandez MA, Correa-Rocha R. HIV infection of human regulatory T cells downregulates Foxp3 expression by increasing DNMT3b levels and DNA methylation in the FOXP3 gene. AIDS 2013; 27(13):2019-29; PMID:24201117; http://dx.doi.org/10.1097/QAD.0b013e32836253fd
- Nakayama-Hosoya K, Ishida T, Youngblood B, Nakamura H, Hosoya N, Koga M, Koibuchi T, Iwamoto A, Kawana-Tachikawa A. Epigenetic repression of interleukin 2 expression in senescent CD4+ T cells during chronic HIV type 1 infection. J Infect Dis 2015; 211(1):28-39; PMID:25001463; http://dx.doi.org/10.1093/infdis/jiu376
- Gross AM, Jaeger PA, Kreisberg JF, Licon K, Jepsen KL, Khosroheidari M, Morsey BM, Swindells S, Shen H, Ng CT, et al. Methylome-wide analysis of chronic HIV infection reveals five-year increase in biological age and epigenetic targeting of HLA. Mol Cell 2016; 62(2):157-68; PMID:27105112; http://dx.doi.org/10.1016/j.molcel.2016.03.019
- De Jager PL, Srivastava G, Lunnon K, Burgess J, Schalkwyk LC, Yu L, Eaton ML, Keenan BT, Ernst J, McCabe C, et al. Alzheimer's disease: early alterations in brain DNA methylation at ANK1, BIN1, RHBDF2 and other loci. Nat Neurosci 2014; 17(9):1156-63; PMID:25129075; http://dx.doi.org/10.1038/nn.3786
- Liang L, Willis-Owen SA, Laprise C, Wong KC, Davies GA, Hudson TJ, Binia A, Hopkin JM, Yang IV, Grundberg E, et al. An epigenome-wide association study of total serum immunoglobulin E concentration. Nature 2015; 520(7549):670-4; PMID:25707804; http://dx.doi.org/10.1038/nature14125
- Paul DS, Beck S. Advances in epigenome-wide association studies for common diseases. Trends Mol Med 2014; 20(10):541-3; PMID:25092140; http://dx.doi.org/10.1016/j.molmed.2014.07.002
- Zhang Y, Li SK, Yi Yang K, Liu M, Lee N, Tang X, Wang H, Liu L, Chen Z, Zhang C, et al. Whole genome methylation array reveals the down-regulation of IGFBP6 and SATB2 by HIV-1. Sci Rep 2015; 5:10806; PMID:26039376; http://dx.doi.org/10.1038/srep10806
- Lehne B, Drong AW, Loh M, Zhang W, Scott WR, Tan ST, Afzal U, Scott J, Jarvelin MR, Elliott P, et al. A coherent approach for analysis of the Illumina HumanMethylation450 BeadChip improves data quality and performance in epigenome-wide association studies. Genome Biol 2015; 16:37; PMID:25853392; http://dx.doi.org/10.1186/s13059-015-0600-x
- Houseman EA, Accomando WP, Koestler DC, Christensen BC, Marsit CJ, Nelson HH, Wiencke JK, Kelsey KT. DNA methylation arrays as surrogate measures of cell mixture distribution. BMC Bioinformatics 2012; 13:86; PMID:22568884; http://dx.doi.org/10.1186/1471-2105-13-86
- Jaffe AE, Irizarry RA. Accounting for cellular heterogeneity is critical in epigenome-wide association studies. Genome Biol 2014; 15(2):R31; PMID:24495553; http://dx.doi.org/10.1186/gb-2014-15-2-r31
- Meissner TB, Li A, Biswas A, Lee KH, Liu YJ, Bayir E, Iliopoulos D, van den Elsen PJ, Kobayashi KS. NLR family member NLRC5 is a transcriptional regulator of MHC class I genes. Proc Natl Acad Sci U S A 2010; 107(31):13794-9; PMID:20639463; http://dx.doi.org/10.1073/pnas.1008684107
- Anbanandam A, Albarado DC, Nguyen CT, Halder G, Gao X, Veeraraghavan S. Insights into transcription enhancer factor 1 (TEF-1) activity from the solution structure of the TEA domain. Proc Natl Acad Sci U S A 2006; 103(46):17225-30; PMID:17085591; http://dx.doi.org/10.1073/pnas.0607171103
- Roth C, Schuierer M, Gunther K, Buettner R. Genomic structure and DNA binding properties of the human zinc finger transcriptional repressor AP-2rep (KLF12). Genomics 2000; 63(3):384-90; PMID:10704285; http://dx.doi.org/10.1006/geno.1999.6084
- Hurlin PJ, Ayer DE, Grandori C, Eisenman RN. The Max transcription factor network: involvement of Mad in differentiation and an approach to identification of target genes. Cold Spring Harb Symp Quant Biol 1994; 59:109-16; PMID:7587059; http://dx.doi.org/10.1101/SQB.1994.059.01.014
- Klase Z, Yedavalli VS, Houzet L, Perkins M, Maldarelli F, Brenchley J, Strebel K, Liu P, Jeang KT. Activation of HIV-1 from latent infection via synergy of RUNX1 inhibitor Ro5-3335 and SAHA. PLoS Pathog 2014; 10(3):e1003997; PMID:24651404; http://dx.doi.org/10.1371/journal.ppat.1003997
- Landi A, Vermeire J, Iannucci V, Vanderstraeten H, Naessens E, Bentahir M, Verhasselt B. Genome-wide shRNA screening identifies host factors involved in early endocytic events for HIV-1-induced CD4 down-regulation. Retrovirology 2014; 11:118; PMID:25496667; http://dx.doi.org/10.1186/s12977-014-0118-4
- Mehta S, Jeffrey KL. Beyond receptors and signaling: epigenetic factors in the regulation of innate immunity. Immunol Cell Biol 2015; 93(3):233-44; PMID:25559622; http://dx.doi.org/10.1038/icb.2014.101
- Doerfler W. Beware of manipulations on the genome: epigenetic destabilization through (foreign) DNA insertions. Epigenomics 2016; 8(5):587-91; PMID:26997469; http://dx.doi.org/10.2217/epi-2016-0019
- Doerfler W. Impact of foreign DNA integration on tumor biology and on evolution via epigenetic alterations. Epigenomics 2012; 4(1):41-9; PMID:22332657; http://dx.doi.org/10.2217/epi.11.111
- Weber S, Hofmann A, Herms S, Hoffmann P, Doerfler W. Destabilization of the human epigenome: consequences of foreign DNA insertions. Epigenomics 2015; 7(5):745-55; PMID:26088384; http://dx.doi.org/10.2217/epi.15.40
- Kobayashi KS, van den Elsen PJ. NLRC5: a key regulator of MHC class I-dependent immune responses. Nat Rev Immunol 2012; 12(12):813-820; PMID:23175229; http://dx.doi.org/10.1038/nri3339
- Lamkanfi M, Kanneganti TD. Regulation of immune pathways by the NOD-like receptor NLRC5. Immunobiology 2012; 217(1):13-6; PMID:22024701; http://dx.doi.org/10.1016/j.imbio.2011.08.011
- Yao Y, Wang Y, Chen F, Huang Y, Zhu S, Leng Q, Wang H, Shi Y, Qian Y. NLRC5 regulates MHC class I antigen presentation in host defense against intracellular pathogens. Cell Res 2012; 22(5):836-47; PMID:22491475; http://dx.doi.org/10.1038/cr.2012.56
- Staehli F, Ludigs K, Heinz LX, Seguin-Estevez Q, Ferrero I, Braun M, Schroder K, Rebsamen M, Tardivel A, Mattmann C, et al. NLRC5 deficiency selectively impairs MHC class I- dependent lymphocyte killing by cytotoxic T cells. J Immunol 2012; 188(8):3820-8; PMID:22412192; http://dx.doi.org/10.4049/jimmunol.1102671
- Neerincx A, Rodriguez GM, Steimle V, Kufer TA. NLRC5 controls basal MHC class I gene expression in an MHC enhanceosome-dependent manner. J Immunol 2012; 188(10):4940-50; PMID:22490867; http://dx.doi.org/10.4049/jimmunol.1103136
- Biswas A, Meissner TB, Kawai T, Kobayashi KS. Cutting edge: impaired MHC class I expression in mice deficient for Nlrc5/class I transactivator. J Immunol 2012; 189(2):516-20; PMID:22711889; http://dx.doi.org/10.4049/jimmunol.1200064
- Meissner TB, Liu YJ, Lee KH, Li A, Biswas A, van Eggermond MC, van den Elsen PJ, Kobayashi KS. NLRC5 cooperates with the RFX transcription factor complex to induce MHC class I gene expression. J Immunol 2012; 188(10):4951-8; PMID:22490869; http://dx.doi.org/10.4049/jimmunol.1103160
- Yoshihama S, Roszik J, Downs I, Meissner TB, Vijayan S, Chapuy B, Sidiq T, Shipp MA, Lizee GA, Kobayashi KS. NLRC5/MHC class I transactivator is a target for immune evasion in cancer. Proc Natl Acad Sci U S A 2016; 113(21):5999-6004; PMID:27162338; http://dx.doi.org/10.1073/pnas.1602069113
- Cui J, Zhu L, Xia X, Wang HY, Legras X, Hong J, Ji J, Shen P, Zheng S, Chen ZJ, et al. NLRC5 negatively regulates the NF-kappaB and type I interferon signaling pathways. Cell 2010; 141(3):483-96; PMID:20434986; http://dx.doi.org/10.1016/j.cell.2010.03.040
- Tong Y, Cui J, Li Q, Zou J, Wang HY, Wang RF. Enhanced TLR-induced NF-kappaB signaling and type I interferon responses in NLRC5 deficient mice. Cell Res 2012; 22(5):822-35; PMID:22473004; http://dx.doi.org/10.1038/cr.2012.53
- Soupene E, Kuypers FA. Phosphatidylcholine formation by LPCAT1 is regulated by Ca(2+) and the redox status of the cell. BMC Biochem 2012; 13:8; PMID:22676268; http://dx.doi.org/10.1186/1471-2091-13-8
- Harayama T, Shindou H, Ogasawara R, Suwabe A, Shimizu T. Identification of a novel noninflammatory biosynthetic pathway of platelet-activating factor. J Biol Chem 2008; 283(17):11097-106; PMID:18285344; http://dx.doi.org/10.1074/jbc.M708909200
- Verma M. Epigenetic regulation of HIV, AIDS, and AIDS-related malignancies. Methods Mol Biol 2015; 1238:381-403; PMID:25421672; http://dx.doi.org/10.1007/978-1-4939-1804-1_21
- Liu Y, Aryee MJ, Padyukov L, Fallin MD, Hesselberg E, Runarsson A, Reinius L, Acevedo N, Taub M, Ronninger M, et al. Epigenome-wide association data implicate DNA methylation as an intermediary of genetic risk in rheumatoid arthritis. Nat Biotechnol 2013; 31(2):142-7; PMID:23334450; http://dx.doi.org/10.1038/nbt.2487
- Langevin SM, Houseman EA, Accomando WP, Koestler DC, Christensen BC, Nelson HH, Karagas MR, Marsit CJ, Wiencke JK, Kelsey KT. Leukocyte-adjusted epigenome-wide association studies of blood from solid tumor patients. Epigenetics 2014; 9(6):884-95; PMID:24671036; http://dx.doi.org/10.4161/epi.28575
- Nelson G, Jia L, Elloumi F, Binns E, Goedert JJ, Limou S, Winkler C. Adjusting infinium methylation profiles to suppress signals from varying cell proportion. Am Soc Hum Genet 2015; PMID:26404086
- Fultz SL, Skanderson M, Mole LA, Gandhi N, Bryant K, Crystal S, Justice AC. Development and verification of a “virtual” cohort using the National VA Health Information System. Med Care 2006; 44(8 Suppl 2):S25-30; PMID:16849965; http://dx.doi.org/10.1097/01.mlr.0000223670.00890.74
- Horvath S, Levine AJ. HIV-1 infection accelerates age according to the epigenetic clock. J Infect Dis 2015; 212(10):1563-73; PMID:25969563; http://dx.doi.org/10.1093/infdis/jiv277
- Reynolds LM, Taylor JR, Ding J, Lohman K, Johnson C, Siscovick D, Burke G, Post W, Shea S, Jacobs DR, Jr, et al. Age-related variations in the methylome associated with gene expression in human monocytes and T cells. Nat Commun 2014; 5:5366; PMID:25404168; http://dx.doi.org/10.1038/ncomms6366
- Bacalini MG, Boattini A, Gentilini D, Giampieri E, Pirazzini C, Giuliani C, Fontanesi E, Remondini D, Capri M, Del Rio A, et al. A meta-analysis on age-associated changes in blood DNA methylation: results from an original analysis pipeline for Infinium 450k data. Aging (Albany NY) 2015; 7(2):97-109; PMID:25701668; http://dx.doi.org/10.18632/aging.100718
- Garagnani P, Bacalini MG, Pirazzini C, Gori D, Giuliani C, Mari D, Di Blasio AM, Gentilini D, Vitale G, Collino S, et al. Methylation of ELOVL2 gene as a new epigenetic marker of age. Aging Cell 2012; 11(6):1132-4; PMID:23061750; http://dx.doi.org/10.1111/acel.12005
- Bekaert B, Kamalandua A, Zapico SC, Van de Voorde W, Decorte R. Improved age determination of blood and teeth samples using a selected set of DNA methylation markers. Epigenetics 2015; 10(10):922-30; PMID:26280308; http://dx.doi.org/10.1080/15592294.2015.1080413