ABSTRACT
Chronic obstructive pulmonary disease (COPD) is a smoking-related disease characterized by genetic and phenotypic heterogeneity. Although association studies have identified multiple genomic regions with replicated associations to COPD, genetic variation only partially explains the susceptibility to lung disease, and suggests the relevance of epigenetic investigations. We performed genome-wide DNA methylation profiling in homogenized lung tissue samples from 46 control subjects with normal lung function and 114 subjects with COPD, all former smokers. The differentially methylated loci were integrated with previous genome-wide association study results. The top 535 differentially methylated sites, filtered for a minimum mean methylation difference of 5% between cases and controls, were enriched for CpG shelves and shores. Pathway analysis revealed enrichment for transcription factors. The top differentially methylated sites from the intersection with previous GWAS were in CHRM1, GLT1D1, and C10orf11; sorted by GWAS P-value, the top sites included FRMD4A, THSD4, and C10orf11. Epigenetic association studies complement genetic association studies to identify genes potentially involved in COPD pathogenesis. Enrichment for genes implicated in asthma and lung function and for transcription factors suggests the potential pathogenic relevance of genes identified through differential methylation and the intersection with a broader range of GWAS associations.
Introduction
Chronic obstructive pulmonary disease (COPD) is a smoking-related disease characterized by progressive airflow obstruction, genetic and phenotypic heterogeneity. Although genetic studies have identified multiple genomic regions with replicated associations with COPD, genetic variation only partially explains susceptibility to lung disease. Loci associated with complex disease traits are enriched in regulatory regions with epigenetic annotationsCitation1 and may highlight mechanisms relevant to complex traits. Interrogation of the epigenome may therefore uncover molecular targets that are perturbed by smoking yet only meet a suggestive threshold for relevance to lung disease through genetic association analysis. These targets may be among previously identified sub-genome-wide significant loci.Citation2,3 Given the growing burden of COPD worldwide, it is imperative to identify molecular characteristics that may provide insights into COPD susceptibility and severity.
Several studies have identified DNA methylation sites associated with COPD (Supplemental Table S1). Many of these have focused on whole blood; however, buccal, sputum, and lung tissue samples have also been investigated. DNA methylation has been suggested to be under genetic control in normal lung tissue,Citation4 and predictive of gene expression in COPD.Citation5 In previous studies of the small-airway epithelium, genome-wide DNA methylation associations with smoking exposureCitation6 and with COPD statusCitation7 have identified key genes and pathways exhibiting epigenetic disruption. Genetic association studies have identified loci at genome-wide significance; genes nearest to these loci (GWAS genes) include IREB2 in the 15q25 locus (CHRNA3/5, IREB2), HHIP in the 4q31 locus, and FAM13A in the 4q22 locus.Citation8-10 Recently, RIN3, MMP12, and TGFB2 were identified as likely COPD genesCitation11 and GWAS of CT emphysemaCitation12 identified DLC1 and AGER. Information regarding DNA methylation in the regions surrounding these loci is limited, particularly in lung tissue from subjects with COPD; in normal lung tissue,Citation4 2 CpG sites (cg24631222 and cg04882995) were found to be significantly associated with lung cancer GWAS SNPs in the 15q25.1 locus (CHRNA5, CHRNA3, CHRNB4). Integration of epigenetic and genetic association studies has the potential to highlight targets of interest related to COPD pathogenesis,Citation1,2,13 which involve both genetic and environmental factors.
We hypothesized that examination of DNA methylation would reveal genes involved in the etiology and pathogenesis of COPD. Genome-wide DNA methylation profiling in lung tissue identified differentially methylated loci associated with COPD affection status. These findings in lung tissues from severe COPD and ex-smoking control subjects highlighted new genes to consider for COPD pathogenesis and provided epigenetic annotation to GWAS candidates, through integration with significant and sub-genome-wide significant COPD GWAS results, lending priority to genes for future investigation.
Results
DNA methylation data were available in lung tissue for 114 severe COPD cases [mean forced expiratory volume in 1 sec (FEV1) 26.3% predicted] and 46 controls with normal spirometry (). There were no significant differences between cases and controls by sex, age, or race. On average, the cases had higher cumulative pack-years of cigarettes smoked and quit smoking on average fewer years in the past (P-value < 0.0001).
Table 1. Study subjects.
Differential methylation
Adjusted for covariates including age, sex, race, and pack-years of smoking, there were 2,456 differentially methylated sites associated with COPD case-control status (5% FDR, no filter for mean methylation difference, Manhattan and volcano plots in ). Filtering the data using a minimum difference in methylation between cases and controls (β-diff) of 5% yielded 535 associations ( and Supplemental Table S2). The counts of the results with each island location annotation were examined (Supplemental Fig. S1); not all CpG sites have an island related annotation. Significant enrichment in the shore and shelf regions was observed (hypergeometric test P-value < 0.0001). Nominally significant associations between the methylation and gene expression levels were observed for 2 of the 7 genes in the top (β-diff > 5%) results (PITPNM1 and HNF1B) (Supplemental Figs. S2-S8, P-value < 0.05). Eleven of the 535 differentially methylated sites demonstrated a mean absolute difference between cases and controls of at least 10% (). Six of these are annotated to genes (), with the top finding (cg06289725) annotated to M1 muscarinic acetylcholine receptor (CHRM1; FDR q-value = 0.03, β-diff = 10%); 5 of these associations did not annotate to genes (e.g., cg01595951, Supplemental Fig. S9). Associations between methylation and gene expression levels (P-value < 0.05) were observed for all 6 of the annotated genes with a 10% difference between cases and controls, which include CHRM1, deltex 1, E3 ubiquitin ligase (DTX1), PACSIN1, FOXK1, SHANK2, and FOXP2 (Supplemental Figs. S10-S15). Of note, total pack-years of cigarette smoking and time since quitting smoking were not statistically significant predictors of lung tissue methylation (at 5% FDR).
Figure 1. Differential methylation results. (A) Manhattan plot of the results from analysis of differential methylation by COPD affection status. The blue line represents the threshold for an FDR of 5%. (B) Volcano plot of these results (FDR < 5% and >5% β-diff in blue). Sites with FDR < 5% and β-diff < 5% are shown (cg10800464, cg11635101:MAD1L1, cg07068735:RAB8B, cg00622552:ODF3L1, and cg20802051:AK3L1).
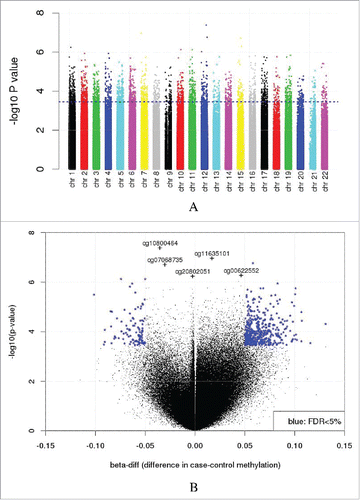
Figure 2. Case and control methylation levels for the top results. Case-control methylation plots (using beanplot R package) for the CpG sites with gene annotations that have at least a 10% β-diff (regression coefficient for affection status). The P-values are adjusted for multiple testing.
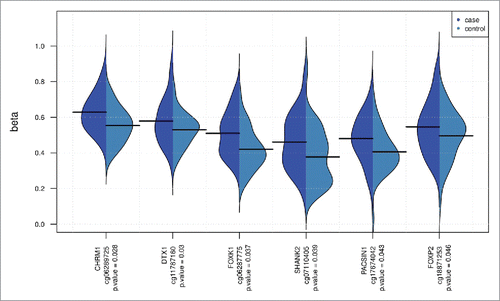
Table 2. Top 10 CpG sites associated with COPD above 5% β-diff threshold, sorted by P-value.
Table 3. CpG sites associated with COPD above 10% β-diff threshold, sorted by P-value.
To further examine the differential methylation results associated with COPD, pathway analyses were performed. The GO analysis for the top differential methylation results (5% FDR and β-diff > 5%) revealed significant enrichment for known transcription factor activity (FDR q-value = 0.006) in the molecular function analysis (37 of the 332 unique gene annotations recognized by WebGestalt, Supplemental Table S3). Significant enrichments related to biological processes were not observed. The top enriched pathways in the ConsensusPathDB analysis were ‘EGFR1’ and ‘Wnt signaling’ (FDR q-value < 0.05).
Hypothesizing that genes harboring multiple differentially methylated sites (same gene annotation for multiple CpG sites) may provide cumulative support for epigenetic associations, we identified multi-site genes using the 1,216 gene-annotated CpG sites that were differentially methylated by at least 1% (at 5% FDR). A hypergeometric test was applied to determine enrichment of sites for each gene and the results were sorted by P-value (). Top multi-site genes include phosphatidylinositol transfer protein, membrane associated 1 (PITPNM1), which is nominally differentially expressed in the lung by COPD affection status (); the 6 significant sites (out of the 17 assayed) span a 1,847 base region. Endothelial cell-specific molecule 1 (ESM1) is a gene also differentially expressed in the lung (); the four significant sites (out of 8 assayed) span 164 bases. For these multi-site genes there is a consistent difference in methylation between cases and controls across the clustered CpG sites ( and Supplemental Fig. S16).
Figure 3. Case and control methylation and expression levels for 2 multi-site genes. Multi-site results for ESM1 and PITPNM1. Plots of COPD case-control methylation for CpG sites with 5% FDR and β-diff > 1%; ESM1 (A), PITPNM1 (B). Corresponding gene expression plots for these genes (case-control differential expression P-values are unadjusted); ESM1 (C), PITPNM1 (D).
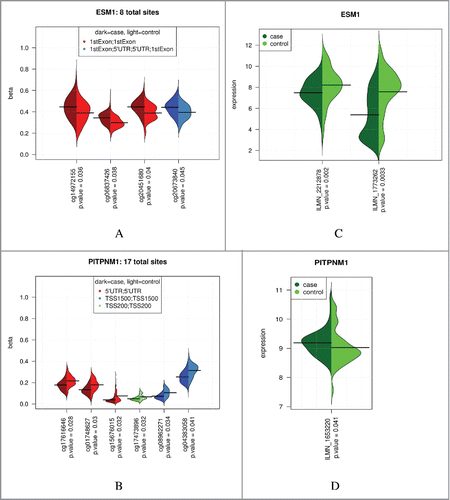
Table 4. Genes most enriched (hypergeometric test P <0.25) with differentially methylated CpG sites that are associated with COPD affection status (multi-site genes).
Integration with GWAS
We integrated the genome-wide significant loci identified in previous COPD genome-wide association studies,Citation8,9,11,12 with the differential methylation results after filtering for minimal differences in methylation (β-diff) (3,260 sites, β-diff > 5% and 44,662 sites; β-diff > 1%). We observed nominal (FDR < 10%) evidence of differential methylation in the threshold COPD GWAS genes FAM13A, HHIP, DLC1, TGFB2, and RIN3 (Supplemental Tables S4 and S5). Examining the GWASCitation11 loci (P-value < 1x10-5) within a 100 kb window surrounding each β-diff > 1% and FDR < 5% site, we observed a site (cg27554782) annotated to CHRNB4 with nearby GWAS SNPs annotated to CHRNA3, CHRNA5, and CHRNB4, within the 15q25 locus.
We integrated the GWASCitation11 results at a P-value < 0.01 with the significant differential methylation results (5% FDR and β-diff > 5%) by gene symbol annotation (, Supplemental Table S6). Of the 344 unique gene annotations for these 535 differential methylation results, 142 were found among the 6,425 genes in these sub-genome-wide significant loci that were also represented on the HumanMethylation450 BeadChip array. The top results were the highest ranked findings with at least 5% difference in methylation between cases and controls— CpG site cg25823837, annotated to glycosyltransferase 1 domain containing 1 (GLT1D1; FDR q-value = 0.017, β-diff = 5.8%) and cg08754149, annotated to chromosome 10 open reading frame 11 (C10orf11; FDR q-value = 0.028, β-diff = −7.5%). When sorted by GWAS P-value, the top sites from this intersection were cg12656653, in FERM domain containing 4A (FRMD4A), and cg17882867, in thrombospondin type 1 domain containing 4 (THSD4)(Supplemental Table S7); cg08754149 in C10orf11 was also among these top ranked results. Seventeen of the 37 transcription factors identified in the GO analysis of differential methylation were among the genes in this methylation-GWAS intersection; 2 of these 17 transcription factors are robustly differentially methylated sites (FOXK1, FOXP2)(). Lastly, 7 of the genes in the GWAS intersection are multi-site genes, including ESM1 (, ), CHRM1 (), and PTCHD4 ().
Table 5. Intersection by gene annotation of the sub-genome-wide significant (P-value < 0.01) COPD GWAS results and the differential methylation results (5% FDR and β-diff > 5%); top ten results sorted by differential methylation P-value.
Discussion
The susceptibility to, and severity of, COPD is highly variable among smokers. For this study, the short-term effects of smoking were avoided by selecting a cohort of former smokers. Genetic variation has been associated with COPD; however, much of the variation in COPD susceptibility remains unexplained. Through genome-wide DNA methylation analysis and integration of differential methylation results with sub-threshold GWAS results, we sought to identify new COPD genes and provide functional annotation to genes identified via genome-wide association. The results of a combined genetic-epigenetic analysis may highlight genes modulated by both environmental and genetic factors, and lend priority to genes that do not meet strict statistical thresholds for genome-wide association.
Statistically significant associations (FDR < 5%) for differential methylation between COPD cases and controls identified in this study capture mean differences that range from less than one percent to a maximum of approximately 13 percent, and were distributed throughout the genome. DNA methylation studies in complex diseases do not capture the same broad range of variation as cancer studies; however, our approach was to focus on associations with at least a 5% difference in mean methylation between cases and controls, thus revealing sites more likely to be biologically meaningful. The application of a 5% filter for methylation difference revealed 535 significant sites enriched for CpG island shore and shelf annotations. Prior studies have suggested that the shores and shelves are regulatory regions.Citation2,14,15
Associations with gene expression were more robust for CpGs with at least a 10% mean difference in methylation between cases and controls, when compared with the results after applying a 5% difference threshold, further suggesting that selection for larger ranges of differential methylation in complex diseases may help to reveal biologically relevant methylation marks. Among the 5% and 10% filtered results, several genes of interest emerged: CHRM1, DTX1, GLT1D1, and C10orf11. M1 muscarinic acetylcholine receptor (CHRM1) has been implicated in bronchoconstriction of the airways and a small candidate gene study noted association of CHRM1 to asthma.Citation16 In addition, CHRM1 has been implicated in association with nicotine dependence.Citation17 CHRM1 presents as an interesting gene, not only because of significance in our study (FDR q-value = 0.03, β-diff = 0.10), but also given the putative role of other muscarinic receptor genes in COPD.Citation18 Deltex 1, E3 ubiquitin ligase (DTX1) may play a role in COPD through its involvement in natural killer T cell development and its function in notch signaling.Citation19 More compelling may be the location of the differential methylation in the gene body of DTX1 and within a CpG island, particularly given the positively correlated association between the site methylation level and gene expression. From a previous pharmacogenetics GWAS,Citation20 a locus in glycosyltransferase 1 domain containing 1 (GLT1D1) was found to be associated with reduced responses to leukotriene modifiers in the treatment of asthma. The CpG site annotated to GLT1D1 (cg25823837) is located 123,920 bases upstream from this locus (rs517020). Chromosome 10 open reading frame 11 (C10orf11) was previously found to be associated with lung function.Citation21 Observing these differential methylation results as a whole, there is suggestive evidence of involvement of differential methylation in genes with prior implications in respiratory disease and phenotypes directly related to COPD. Lastly, a pathway analysis revealed enrichment for transcription factor activity among the GO molecular function results. Among the top differentially methylated results, 3 genes are transcription factors: HNF1B, FOXK1, and FOXP2. With respect to the ConsensusPathDB results, EGFR1 signaling has been implicated in chronic bronchitisCitation22 and WNT signaling is involved in the pathogenesis of lung development, COPD,Citation23 and asthma.Citation24
Differential methylation in multiple sites across a defined genomic region may reveal more biological relevance than single CpG associations, and in contrast to previous methods, such as bump hunting, that are agnostic to genomic annotation,Citation25,26 we defined regions using multi-site associations for annotated genes. Among these multi-site genes, 3 are of particular interest: CHRM1, as it was the top result among 10% β-diff results; ESM1, a gene mainly expressed in the endothelial cells in human lung and kidney tissuesCitation27; and PITPNM1, a gene found within a linkage disequilibrium block surrounding the susceptibility gene identified in a previous association study of childhood asthma.Citation28 The ESM1 result provides a compelling demonstration of COPD-related gene regulation through hypermethylation in the cases, and concomitant reduction in gene expression. Although the ESM1 sites fall within the first exon for a majority of the transcripts, and this location (downstream of the transcription start site) would predict a positive correlation between gene expression and methylation,Citation29 other transcripts are also annotated to the 5′ UTR, where anti-correlation could be expected.Citation14 The regulatory effects of DNA methylation are still not fully understood.Citation1,15 Given the regulatory nature (5′ UTR and transcription start site region) of the sites annotated to PITPNM1, the anti-correlation observed (increased expression with hypomethylation in cases) follows the more traditional paradigm.
Leveraging prior statistically significant COPD GWAS findings, we performed an intersection of the differential methylation results with genes from genome-wide significant GWAS loci. This yielded 3 sites annotated to 2 of the GWAS genes at a 10% FDR (DLC1 and TGFB2);Citation11,12 no differentially methylated CpG sites were identified at a 5% FDR. Using more liberal criteria of 1% difference in methylation revealed 17 sites annotated to 5 of the GWAS genes significant at a 10% FDR (FAM13A, HHIP, DLC1, TGFB2, RIN3). Intersecting the differentially methylated sites with the sub-genome-wide significant GWAS results yielded 142 overlapping genes, with the top 2 being higher ranking differential methylation sites, annotated to GLT1D1 and C10orf11. The fact that 17 of the 37 transcription factors identified in the differential methylation results were found among the genes in this intersection highlights the regulatory implications of intersecting genetic and methylation associations. Two of these 17 transcription factors are robustly differentially methylated and differentially expressed between cases and controls: FOXK1 and FOXP2. The GWAS intersection also included the multi-site genes ESM1 and CHRM1, and CHRM1 contains a top associated methylation site. The top sites from this intersection with the lowest P-values from the GWAS were in FRMD4A and THSD4. FRMD4A is a gene involved in regulation of epithelial cell polarizationCitation30 and a genetic variant in FRMD4A was found to be associated with nicotine dependence.Citation31 CpG sites in this gene were found to have methylation levels associated with prenatal smoke exposureCitation32 and were differentially methylated by smoking status in buccal mucosa.Citation33 Variants in the THSD4 locus have been found to be associated with lung functionCitation34 and, in particular, lung function in severe COPD.Citation35
This study revealed differentially methylated sites annotated to COPD GWAS candidate genes and genes with previously identified links to lung disease and treatment response, as well as others in physical proximity with known GWAS susceptibility loci. These sites have strong support in this study. Their methylation differences between cases and controls were larger than usually reported for complex disease studies, and multi-site methylation signals also support their relevance. The integration with prior GWAS results has highlighted several genes, including CHRM1 and ESM1 with their GWAS and multi-site results, DLC1 given its significance in prior emphysema GWAS, and C10orf11 and GLT1D1, due to their single-site ranking and GWAS associations.
Some limitations of this study involve cellular heterogeneity inherent in homogenized lung tissue. Future studies should focus on follow-up of these findings in single lung cell types. Integration with genotyping data will elucidate the association between genetic variants and DNA methylation. Additionally, although we have observed correlation between DNA methylation and gene expression for the most robustly differentially methylated sites, we have not performed functional validation of these findings. Epigenome editingCitation36-38 is becoming a viable approach for validation of DNA methylation-expression correlations with refinements in laboratory methods including CRISPR-Cas9.Citation39 Application of these technologies to epigenetics research will accelerate the pace of discovery as well as provide a path to epigenetic therapeutics, and is a future direction for this work. Lastly, our study focused on severe COPD and may not be generalizable to milder COPD, treatment effects, or additional COPD phenotypes, including emphysema and airways disease.
Epigenetic studies complement genetic association studies to identify COPD pathogenesis genes. Enrichment for genes implicated in asthma and lung function as well as in transcription factors suggest the potential functional relevance of genes identified through differential methylation and the intersection with a broader range of sub-threshold GWAS associations. Epigenetic associations may highlight new pathogenesis pathways and targets for treatment and primary prevention of COPD.
Methods
Lung tissue samples were collected from subjects undergoing thoracic surgery for lung transplantation, lung volume reduction surgery, or lung nodule resection at 3 medical centers: Brigham and Women's Hospital (Boston, MA), St. Elizabeth's Hospital (Boston, MA), and Temple University Hospital (Philadelphia, PA). Institutional Review Board approval was obtained at the 3 centers and subjects provided written informed consent. All subjects were former smokers who quit smoking at least one month prior to surgery. Distant normal tissue was used from nodule resection samples. Phenotypes obtained from the medical records included demographics, anthropometrics, smoking history, and pulmonary function testing. Severe COPD subjects were defined by GOLD grade 3–4 spirometry.Citation40 Control smokers had normal spirometry [FEV1 ≥ 80% predicted and ratio of FEV1 to forced vital capacity (FEV1/FVC) ≥ 0.7].
DNA methylation
Lung tissue samples were snap frozen and stored at −80°C. For each of the samples, DNA was extracted and 1 μg of DNA was bisulfite-converted using the EZ DNA Methylation Gold Kit (Zymo Research, Irvine, CA). The genome-wide methylation levels of the DNA samples were assayed using the Infinium HumanMethylation450 BeadChip array from Illumina (San Diego, CA). Additional details are provided in an online data supplement. In total, 485,512 genome-wide CpG sites were interrogated for each sample. Data import and pre-processing were performed using R and the Bioconductor packages minfi (version 1.14.0).Citation41 Detection P-values were examined for probes with a ‘cg’ prefix ('rs' and ‘ch’ probes were excluded) and only for probes mapped to autosomal chromosomes. Quality control metrics include median detection P-values across all samples less than 0.05 for each probe, and fewer than 25% of detection P-values > 0.05 for each probe. Probes not meeting these thresholds were excluded, as were cross-reactive probes.Citation42 Probes for CpG sites located within zero to one base of a known SNP (dbSNP 141 GRCh37.p13) were excluded from analysis. Multidimensional scaling (MDS) plots were produced using data from probes mapped to only the X chromosome in order to highlight any sex mismatch issues. A summary of the quality control process is provided in Supplemental Table S8, where the final number of probes for analysis was 349,826 and 160 of 171 subjects were retained. Background adjustment was performed on the cleaned data via the normal-exponential using out-of-band (noob) background correction method with dye-bias equalization,Citation43 followed by BMIQ (wateRmelon version 1.8.0) within array normalization.Citation44 The final percent methylation values (β value with a range 0.0 to 1.0) were calculated using: β = meth / (meth + unmeth + offset), where the offset had the default Illumina value of 100 and meth and unmeth are the fluorescence intensity values at methylated versus unmethylated sites respectively. As a final pre-analysis cleaning step for this study, lower-quality values in the β matrix (detection P-value > 1x10-9) were excluded. Manufacturer annotation of assay site locations with respect to CpG islands and genes were used.Citation45 Gene symbols were updated to the approved names, as of July 2015, using data from the HUGO Gene Nomenclature Committee database (http://www.genenames.org).
Differential methylation
Differential methylation analyses were performed for each site using R with the Bioconductor package limma (version 3.24.12).Citation46 Models for COPD were adjusted for age, sex, race, pack-years of smoking, and plate number (categorical variable). We also investigated pack-years of smoking and time since quitting smoking for association with methylation in models adjusted for age, sex, race, plate number, and pack-years (for time since quitting model only), with and without adjustments for COPD. A false discovery rate (FDR) of 5% was applied to the results. Given that statistically significant sites may have limited biological meaning for small methylation differences, we examined the data with a focus on mean percent differences in methylation between cases and controls (β-diff) of at least 5%; however, we considered results with at least 1% difference when examining genes harboring multiple differentially methylated marks in the same gene region. We performed pathway analysis using ConsensusPathDBCitation47 and Gene Ontology (GO) analysis using WebGestalt.Citation48 Enrichment results with less than 5% FDR were considered significant.
Integration with prior Genome-wide Association Study (GWAS) results
All differential methylation results for CpG sites annotated to 8 genes found in genome-wide significant loci from previous COPD and emphysema GWASCitation8,9,11,12 were extracted and filtered at β-diff thresholds of 1% and 5% to create 2 subsets of results. The P-values for all results in these 2 subsets were examined to assess the overall significance of the associations involving CpG sites annotated to these GWAS genes.
To integrate with sub-threshold COPD GWAS, the results from a recent COPD case-control GWASCitation11 were extracted at a P-value threshold of 0.01. The total number of GWAS markers meeting this threshold was 95,545, resulting in 12,718 unique gene symbols, with 6,425 of these symbols among the site annotations for the HumanMethylation450 BeadChip array. Annotations for the GWAS results were obtained from the Ensembl database (GRCh37) using the R package biomaRt.Citation49 A list of these genes was extracted that were also found among the CpG annotations for the significant differential methylation results (FDR < 5% and β-diff > 5%). The top differential methylation result with a CpG annotated to a gene in this list was paired with the top GWAS result having the same gene symbol annotation.
Lung tissue gene expression profiling
RNA was extracted from the same lung tissue samples using the AllPrep kit (Qiagen, Valencia, CA). RNA quality was assessed on a BioAnalyzer (Agilent, Santa Clara, CA). Gene expression profiling was performed using HumanHT-12 BeadChips (Illumina, San Diego, CA) and has been reported separately.Citation50 Quality control was performed to identify outliers and samples with questionable or low-quality levels. This process yielded 151 samples for analysis (SupplementalTable S9). There were 32,831 probes retained, after filtering for low variance and percentage of high detection P-values. These data were background corrected, log2-transformed (to stabilize the varianceCitation51) and quantile-normalized using the R Bioconductor package lumi.Citation52 Of the 151 samples, 147 (107 cases and 40 controls) intersected with the 160 samples in the final DNA methylation dataset. Associations of methylation with gene expression were tested using linear models adjusted for age, sex, race, pack-years of smoking, the methylation plate number, and a gene expression batch variable.
Disclosure of potential conflicts of interest
Drs. Morrow, DeMeo, Cho, Pinto-Plata, Celli, Marchetti, Criner, Bueno, Glass, Quackenbush, and Choi report no competing interests related to this manuscript. Dr. Washko has been a consultant for GSK, Genentech, Emmes, PulmonX. Dr. Hersh has been a consultant for CSL Behring and Mylan. Dr. Silverman has received honoraria and consulting fees from Merck, grant support and consulting fees from GlaxoSmithKline, and honoraria from Novartis.
KEPI_A_1226451_supplemental_data.zip
Download Zip (4.1 MB)Acknowledgments
We thank Drs. Amund Gulsvik, Per Bakke, Augusto Litonjua, Pantel Vokonas, Ruth Tal-Singer, and the GenKOLS, NETT/NAS, ECLIPSE, and COPDGene studies for use of their GWAS meta-analysis data.
Funding
NIH grants P01 HL105339, R01 HL111759, P01 HL114501. The COPDGene study (R01 HL089856 and R01 HL089897). (NCT00608764) was funded by the National Institutes of Health is also sup- 460 ported by the COPD Foundation through contributions made to an Industry Advisory Board comprised of AstraZeneca, Boehringer Ingelheim, Novartis, Pfizer, Siemens, GSK and Sunovion. The National Emphysema Treatment Trial was supported by the NHLBI N01HR76101, N01HR76102, N01HR76103, N01HR76104, N01HR76105, N01HR76106, N01HR76107, N01HR76108, 465 N01HR76109, N01HR76110, N01HR76111, N01HR76112, N01HR76113, N01HR76114, N01HR76115, N01HR76116, N01HR76118 and N01HR76119, the Centers for Medicare and Medicaid Services and the Agency for Healthcare Research and Quality. The Normative Aging Study is supported by the Cooperative Studies Program/ERIC of the US 470 Department of Veterans Affairs and is a component of the Massachusetts Veterans Epidemiology Research and Information Center (MAVERIC). The Norway GenKOLS study (Genetics of Chronic Obstructive Lung Disease, GSK code RES11080), the ECLIPSE study (NCT00292552; GSK code SCO104960), and the ICGN study were funded by GlaxoSmithKline.
References
- Kundaje A, Meuleman W, Ernst J, Bilenky M, Yen A, Heravi-Moussavi A, Kheradpour P, Zhang Z, Wang J, Ziller MJ, et al. Integrative analysis of 111 reference human epigenomes. Nature 2015; 518:317-30; PMID:25693563; http://dx.doi.org/10.1038/nature14248
- Feinberg AP. Epigenomics reveals a functional genome anatomy and a new approach to common disease. Nat Biotechnol 2010; 28:1049-1052; PMID:20944596; http://dx.doi.org/10.1038/nbt1010-1049
- Olsson AH, Volkov P, Bacos K, Dayeh T, Hall E, Nilsson EA, Ladenvall C, Rönn T, Ling C. Genome-Wide Associations between Genetic and Epigenetic Variation Influence mRNA Expression and Insulin Secretion in Human Pancreatic Islets. PLoS Genet 2014; 10:e1004735; PMID:25375650; http://dx.doi.org/10.1371/journal.pgen.1004735
- Shi J, Marconett CN, Duan J, Hyland PL, Li P, Wang Z, Wheeler W, Zhou B, Campan M, Lee DS, et al. Characterizing the genetic basis of methylome diversity in histologically normal human lung tissue. Nat Commun 2014 Feb 27; 5:3365; PMID:24572595; http://dx.doi.org/10.1038/ncomms4365
- Yoo S, Takikawa S, Geraghty P, Argmann C, Campbell J, Lin L, Huang T, Tu Z, Feronjy R, Spira A, et al. Integrative Analysis of DNA Methylation and Gene Expression Data Identifies EPAS1 as a Key Regulator of COPD. PLoS Genet 2015; 11:e1004898; PMID:25569234; http://dx.doi.org/10.1371/journal.pgen.1004898
- Buro-Auriemma LJ, Salit J, Hackett NR, Walters MS, Strulovici-Barel Y, Staudt MR, Fuller J, Mahmoud M, Stevenson CS, Hilton H, et al. Cigarette smoking induces small airway epithelial epigenetic changes with corresponding modulation of gene expression. Hum Mol Genet 2013; 22:4726-38; PMID:23842454; http://dx.doi.org/10.1093/hmg/ddt326
- Vucic EA, Chari R, Thu KL, Wilson IM, Cotton AM, Kennett JY, Zhang M, Lonergan KM, Steiling K, Brown CJ, et al. DNA Methylation Is Globally Disrupted and Associated with Expression Changes in Chronic Obstructive Pulmonary Disease Small Airways. Am J Respir Cell Mol Biol 2013; 50:912-22; PMID:24298892; http://dx.doi.org/10.1165/rcmb.2013-0304OC
- Cho MH, Boutaoui N, Klanderman BJ, Sylvia JS, Ziniti JP, Hersh CP, DeMeo DL, Hunninghake GM, Litonjua AA, Sparrow D, et al. Variants in FAM13A are associated with chronic obstructive pulmonary disease. Nat Genet 2010; 42:200-2; PMID:20173748; http://dx.doi.org/10.1038/ng.535
- Cho MH, Castaldi PJ, Wan ES, Siedlinski M, Hersh CP, Demeo DL, Himes BE, Sylvia JS, Klanderman BJ, Ziniti JP, et al. A genome-wide association study of COPD identifies a susceptibility locus on chromosome 19q13. Hum Mol Genet 2012; 21:947-57; PMID:22080838; http://dx.doi.org/10.1093/hmg/ddr524
- Pillai SG, Ge D, Zhu G, Kong X, Shianna KV, Need AC, Feng S, Hersh CP, Bakke P, Gulsvik A, et al. A genome-wide association study in chronic obstructive pulmonary disease (COPD): identification of two major susceptibility loci. PLoS Genet 2009; 5:e1000421; PMID:19300482; http://dx.doi.org/10.1371/journal.pgen.1000421
- Cho MH, McDonald M-LN, Zhou X, Mattheisen M, Castaldi PJ, Hersh CP, Demeo DL, Sylvia JS, Ziniti J, Laird NM, et al. Risk loci for chronic obstructive pulmonary disease: a genome-wide association study and meta-analysis. Lancet Respir Med 2014; 2:214-25; PMID:24621683; http://dx.doi.org/10.1016/S2213-2600(14)70002-5
- Cho MH, Castaldi PJ, Hersh CP, Hobbs BD, Barr RG, Tal-Singer R, Bakke P, Gulsvik A, San José Estépar R, Van Beek EJR, et al. A Genome-Wide Association Study of Emphysema and Airway Quantitative Imaging Phenotypes. Am J Respir Crit Care Med 2015; 192:559-69; PMID:26030696; http://dx.doi.org/10.1164/rccm.201501-0148OC
- Bjornsson H. An integrated epigenetic and genetic approach to common human disease. Trends Genet 2004; 20:350-8; PMID:15262407; http://dx.doi.org/10.1016/j.tig.2004.06.009
- van Eijk KR, de Jong S, Boks MPM, Langeveld T, Colas F, Veldink JH, de Kovel CGF, Janson E, Strengman E, Langfelder P, et al. Genetic analysis of DNA methylation and gene expression levels in whole blood of healthy human subjects. BMC Genomics 2012; 13:636-636; PMID:23157493; http://dx.doi.org/10.1186/1471-2164-13-636
- Jones PA. Functions of DNA methylation: islands, start sites, gene bodies and beyond. Nat Rev Genet 2012; 13:484-92; PMID:22641018; http://dx.doi.org/10.1038/nrg3230
- Maeda Y, Hizawa N, Jinushi E, Honda A, Takahashi D, Fukui Y, Konno S, Shimizu T, Shimizu H, Yamaguchi E, et al. Polymorphisms in the Muscarinic Receptor 1 Gene Confer Susceptibility to Asthma in Japanese Subjects. Am J Respir Crit Care Med 2006; 174:1119-24; PMID:16931638; http://dx.doi.org/10.1164/rccm.200601-081OC
- Lou X-Y, Ma JZ, Payne TJ, Beuten J, Crew KM, Li MD. Gene-based analysis suggests association of the nicotinic acetylcholine receptor β1 subunit (CHRNB1) and M1 muscarinic acetylcholine receptor (CHRM1) with vulnerability for nicotine dependence. Hum Genet 2006; 120:381-9; PMID:16874522; http://dx.doi.org/10.1007/s00439-006-0229-7
- Cherubini E, Esposito MC, Scozzi D, Terzo F, Osman GA, Mariotta S, Mancini R, Bruno P, Ricci A. Genetic Polymorphism of CHRM2 in COPD: Clinical Significance and Therapeutic Implications. J Cell Physiol 2016 Aug; 231(8):1745-51; Epub 2016 Jan 15. PMID:26633752; http://dx.doi.org/10.1002/jcp.25277
- Müller MR, Rao A. NFAT, immunity and cancer: a transcription factor comes of age. Nat Rev Immunol 2010; 10:645-56; PMID:20725108; http://dx.doi.org/10.1038/nri2818
- Dahlin A, Litonjua A, Irvin CG, Peters SP, Lima JJ, Kubo M, Tamari M, Tantisira KG. Genome-wide association study of leukotriene modifier response in asthma. Pharmacogenomics J 2016 Apr; 16(2):151-7; Epub 2015 Jun 2. PMID:26031901; http://dx.doi.org/10.1038/tpj.2015.34
- Artigas MS, Loth DW, Wain LV, Gharib SA, Obeidat M'en, Tang W, Zhai G, Zhao JH, Smith AV, Huffman JE, et al. Genome-wide association and large-scale follow up identifies 16 new loci influencing lung function. Nat Genet 2011; 43:1082-90; PMID:21946350; http://dx.doi.org/10.1038/ng.941
- Marinaş AE, Ciurea P, Mărgăritescu C, Cotoi OS. Expression of Epidermal Growth Factor (EGF) and its receptors (EGFR1 and EGFR2) in chronic bronchitis. Rom J Morphol Embryol 2012; 53(4):957-66; PMID:23303019
- Heijink IH, de Bruin HG, van den Berge M, Bennink LJC, Brandenburg SM, Gosens R, van Oosterhout AJ, Postma DS. Role of aberrant WNT signalling in the airway epithelial response to cigarette smoke in chronic obstructive pulmonary disease. Thorax 2013; 68:709-16; PMID:23370438; http://dx.doi.org/10.1136/thoraxjnl-2012-201667
- Sharma S, Tantisira K, Carey V, Murphy AJ, Lasky-Su J, Celedón JC, Lazarus R, Klanderman B, Rogers A, Soto-Quirós M, et al. A Role for Wnt Signaling Genes in the Pathogenesis of Impaired Lung Function in Asthma. Am J Respir Crit Care Med 2010; 181:328-36; PMID:19926868; http://dx.doi.org/10.1164/rccm.200907-1009OC
- Jaffe AE, Murakami P, Lee H, Leek JT, Fallin MD, Feinberg AP, Irizarry RA. Bump hunting to identify differentially methylated regions in epigenetic epidemiology studies. Int J Epidemiol 2012; 41:200-9; PMID:22422453; http://dx.doi.org/10.1093/ije/dyr238
- Peters TJ, Buckley MJ, Statham AL, Pidsley R, Samaras K, Lord RV, Clark SJ, Molloy PL. De novo identification of differentially methylated regions in the human genome. Epigenetics Chromatin 2015; 8:1-16; PMID:25621012; http://dx.doi.org/10.1186/1756-8935-8-1
- Lassalle P, Molet S, Janin A, Van der Heyden J, Tavernier J, Fiers W, Devos R, Tonnel A-B. ESM-1 is a novel human endothelial cell-specific molecule expressed in lung and regulated by cytokines. J Biol Chem 1996; 271:20458-20464; PMID:8702785; http://dx.doi.org/10.1074/jbc.271.34.20458
- Kamada F, Mashimo Y, Inoue H, Shao C, Hirota T, Doi S, Kameda M, Fujiwara H, Fujita K, Enomoto T, et al. The GSTP1 Gene Is a Susceptibility Gene for Childhood Asthma and the GSTM1 Gene Is a Modifier of the GSTP1 Gene. Int Arch Allergy Immunol 2007; 144:275-86; PMID:17643058; http://dx.doi.org/10.1159/000106316
- Bell JT, Pai Aa, Pickrell JK, Gaffney DJ, Pique-Regi R, Degner JF, Gilad Y, Pritchard JK. DNA methylation patterns associate with genetic and gene expression variation in HapMap cell lines. Genome Biol 2011; 12:R10-R10; PMID:21251332; http://dx.doi.org/10.1186/gb-2011-12-1-r10
- Ikenouchi J, Umeda M. FRMD4A regulates epithelial polarity by connecting Arf6 activation with the PAR complex. Proc Natl Acad Sci 2010; 107:748-53; PMID:20080746; http://dx.doi.org/10.1073/pnas.0908423107
- Yoon D, Kim Y-J, Cui W-Y, Van der Vaart A, Cho YS, Lee J-Y, Ma JZ, Payne TJ, Li MD, Park T. Large-scale genome-wide association study of Asian population reveals genetic factors in FRMD4A and other loci influencing smoking initiation and nicotine dependence. Hum Genet 2012; 131:1009-21; PMID:22006218; http://dx.doi.org/10.1007/s00439-011-1102-x
- Breton CV, Siegmund KD, Joubert BR, Wang X, Qui W, Carey V, Nystad W, Håberg SE, Ober C, Nicolae D, et al. Prenatal Tobacco Smoke Exposure Is Associated with Childhood DNA CpG Methylation. PLoS ONE 2014; 9:e99716; PMID:24964093; http://dx.doi.org/10.1371/journal.pone.0099716
- Wan ES, Qiu W, Carey VJ, Morrow J, Bacherman H, Foreman MG, Hokanson JE, Bowler RP, Crapo JD, DeMeo DL. Smoking-Associated Site-Specific Differential Methylation in Buccal Mucosa in the COPDGene Study. Am J Respir Cell Mol Biol 2015; 53:246-54; PMID:25517428; http://dx.doi.org/10.1165/rcmb.2014-0103OC
- Repapi E, Sayers I, Wain LV, Burton PR, Johnson T, Obeidat M'en, Zhao JH, Ramasamy A, Zhai G, Vitart V, et al. Genome-wide association study identifies five loci associated with lung function. Nat Genet 2010; 42:36-44; PMID:20010834; http://dx.doi.org/10.1038/ng.501
- Hardin M, Zielinski J, Wan ES, Hersh CP, Castaldi PJ, Schwinder E, Hawrylkiewicz I, Sliwinski P, Cho MH, Silverman EK. CHRNA3/5, IREB2, and ADCY2 Are Associated with Severe Chronic Obstructive Pulmonary Disease in Poland. Am J Respir Cell Mol Biol 2012; 47:203-8; PMID:22461431; http://dx.doi.org/10.1165/rcmb.2012-0011OC
- Bernstein DL, Le Lay JE, Ruano EG, Kaestner KH. TALE-mediated epigenetic suppression of CDKN2A increases replication in human fibroblasts. J Clin Invest 2015; 125:1998-2006; PMID:25866970; http://dx.doi.org/10.1172/JCI77321
- Nunna S, Reinhardt R, Ragozin S, Jeltsch A. Targeted Methylation of the Epithelial Cell Adhesion Molecule (EpCAM) Promoter to Silence Its Expression in Ovarian Cancer Cells. PLoS ONE 2014; 9:e87703; PMID:24489952; http://dx.doi.org/10.1371/journal.pone.0087703
- Rivenbark AG, Stolzenburg S, Beltran AS, Yuan X, Rots MG, Strahl BD, Blancafort P. Epigenetic reprogramming of cancer cells via targeted DNA methylation. Epigenetics 2012; 7:350-60; PMID:22419067; http://dx.doi.org/10.4161/epi.19507
- Vojta A, Dobrinić P, Tadić V, Bočkor L, Korać P, Julg B, Klasić M, Zoldoš V. Repurposing the CRISPR-Cas9 system for targeted DNA methylation. Nucleic Acids Res 2016; 44:5615-28; PMID:26969735; http://dx.doi.org/10.1093/nar/gkw159
- Vestbo J, Hurd SS, Agustí AG, Jones PW, Vogelmeier C, Anzueto A, Barnes PJ, Fabbri LM, Martinez FJ, Nishimura M, et al. Global strategy for the diagnosis, management, and prevention of chronic obstructive pulmonary disease: GOLD executive summary. Am J Respir Crit Care Med 2013; 187:347-65; PMID:22878278; http://dx.doi.org/10.1164/rccm.201204-0596PP
- Hansen KD, Ayree M, Irizarry RA. minfi: Analyze Illumina's 450k methylation arrays (v. 1.5.1). 2012; http://bioconductor.org/packages/release/bioc/html/minfi.html
- Chen Y, Lemire M, Choufani S, Butcher DT, Grafodatskaya D, Zanke BW, Gallinger S, Hudson TJ, Weksberg R. Discovery of cross-reactive probes and polymorphic CpGs in the Illumina Infinium HumanMethylation450 microarray. Epigenetics 2013; 8:203-9; PMID:23314698; http://dx.doi.org/10.4161/epi.23470
- Triche TJ, Weisenberger DJ, Van Den Berg D, Laird PW, Siegmund KD. Low-level processing of Illumina Infinium DNA Methylation BeadArrays. Nucleic Acids Res 2013; 41:e90-e90; PMID:23476028; http://dx.doi.org/10.1093/nar/gkt090
- Teschendorff AE, Marabita F, Lechner M, Bartlett T, Tegner J, Gomez-Cabrero D, Beck S. A beta-mixture quantile normalization method for correcting probe design bias in Illumina Infinium 450 k DNA methylation data. Bioinformatics 2013; 29:189-96; PMID:23175756; http://dx.doi.org/10.1093/bioinformatics/bts680
- Bibikova M, Barnes B, Tsan C, Ho V, Klotzle B, Le JM, Delano D, Zhang L, Schroth GP, Gunderson KL, et al. High density DNA methylation array with single CpG site resolution. Genomics 2011; 98:288-95; PMID:21839163; http://dx.doi.org/10.1016/j.ygeno.2011.07.007
- Smyth GK. Limma: linear models for microarray data. In: Gentleman R, Carey V, Dudoit S, Irizarry R, Huber W, editors. Bioinformatics and Computational Biology Solutions Using {R} and Bioconductor. New York: Springer; 2005. page 397-420; http://dx.doi.org/10.1007/0-387-29362-0
- Kamburov A, Stelzl U, Lehrach H, Herwig R. The ConsensusPathDB interaction database: 2013 update. Nucleic Acids Res 2013; 41:D793-800; PMID:23143270; http://dx.doi.org/10.1093/nar/gks1055
- Wang J, Duncan D, Shi Z, Zhang B. WEB-based GEne SeT AnaLysis Toolkit (WebGestalt): update 2013. Nucleic Acids Res 2013; 41:W77-83; PMID:23703215; http://dx.doi.org/10.1093/nar/gkt439
- Durinck S, Moreau Y, Kasprzyk A, Davis S, De Moor B, Brazma A, Huber W. BioMart and Bioconductor: a powerful link between biological databases and microarray data analysis. Bioinformatics 2005; 21:3439-40; PMID:16082012; http://dx.doi.org/10.1093/bioinformatics/bti525
- Morrow J, Qiu W, DeMeo DL, Houston I, Pinto-Plata VM, Celli BR, Marchetti N, Criner GJ, Bueno R, Washko GR, et al. Network Analysis of Gene Expression in Severe COPD Lung Tissue Samples [abstract]. Am J Respir Crit Care Med 2015; 191:A1253; (published conference abstract from: A30. BIG DATA: HARVESTING FRUITS FROM COPD AND LUNG CANCER. May 1, 2015, A1253-A1253)
- Lin SM, Du P, Huber W, Kibbe WA. Model-based variance-stabilizing transformation for Illumina microarray data. Nucleic Acids Res 2008; 36:e11-e11; PMID:18178591; http://dx.doi.org/10.1093/nar/gkm1075
- Du P, Kibbe WA, Lin SM. lumi: a pipeline for processing Illumina microarray. Bioinformatics 2008; 24:1547-8; PMID:18467348; http://dx.doi.org/10.1093/bioinformatics/btn224