ABSTRACT
Cord blood is widely used as surrogate tissue in epigenome-wide association studies of prenatal conditions. Cell type composition variation across samples can be an important confounder of epigenome-wide association studies in blood that constitute a mixture of cells. We evaluated a newly developed cord blood reference panel to impute cell type composition from DNA methylation levels, including nucleated red blood cells (nRBCs). We estimated cell type composition from 154 unique cord blood samples with available DNA methylation data as well as direct measurements of nucleated cell types. We observed high correlations between the estimated and measured composition for nRBCs (r = 0.92, R2 = 0.85), lymphocytes (r = 0.77, R2 = 0.58), and granulocytes (r = 0.72, R2 = 0.52), and a moderate correlation for monocytes (r = 0.51, R2 = 0.25) as well as relatively low root mean square errors from the residuals ranging from 1.4 to 5.4%. These results validate the use of the cord blood reference panel and highlight its utility and limitations for epidemiological studies.
Introduction
Cord blood is widely used as a surrogate tissue in epidemiological studies of prenatal, maternal, and environmental conditions, as it is easily accessible at birth. Cord blood DNA methylation is of high interest because it has the potential to reflect early life epigenetic programming events that occur during embryogenesis. The study of DNA methylation in blood or cord blood presents a particular challenge as aggregate DNA methylation measures reflect a mixture of different cell types, primarily leukocytes. To overcome this challenge, statistical methods have been developed to estimate cell type distributions from genome-wide DNA methylation arrays using reference panels from isolated cell types.Citation1 Epigenome-wide association studies in blood have capitalized on this method to infer white blood cell composition using an existing reference panel of isolated leukocytes.Citation2 Although this panel consists of 6 different leukocytes (CD4+ T-cells, CD8+ T-cells, NK-cells, B-cells, monocytes and granulocytes) they were isolated from 6 adult males, which might not accurately represent cell type composition or methylation profiles of nucleated cells found in cord blood.
Recently, a panel of nucleated cell types isolated from cord blood samples of males and female newborns was developed.Citation3 Of particular interest in the cord blood panel is the isolation and characterization of DNA methylation of nucleated red blood cells (nRBCs), commonly present in cord blood but not adult blood.Citation4 However, this panel has not been benchmarked against direct cell count measurements in newborn cord blood. Previous studies of cord blood DNA methylation have used the adult leukocyte DNA methylation reference panel to infer cell type composition, but the performance of the adult reference panel for inferring white blood cell composition of cord blood has shown limited utility.Citation5
In the current report, we evaluated the performance of the cord and adult blood reference panels for estimating cell type distributions and compare them to direct cell count measurements of cord blood leukocytes and nRBCs from a birth cohort of 154 mother-child pairs. We further assessed the performance for predicting cell type composition of all the normalization methods available in the minfi bioconductor package used for the analysis of DNA methylation arrays.Citation6 Lastly, we also evaluated the impact of adjusting for cell estimates using the 2 different panels or the directly measured cell types in an epigenome-wide association of gestational age at birth.
Results
A total of 154 cord blood samples were available for the validation of the estimated cell counts. Mothers were on average 28 y of age at the time of enrollment. About half of newborns were female (55.2%) and almost all were from European descent (99%)(). From the direct clinical measurement of cell types, granulocytes were the most abundant cell type found in cord blood, constituting on average 52.9% [standard deviation (SD) = 7.8] of the nucleated cell types, followed by lymphocytes (mean = 31.3%; SD = 7.2), and monocytes (mean = 10.5%; SD = 2.3). Of the measured cell types in cord blood, nRBCs were the least abundant, constituting on average 5.4% (SD = 3.6) across samples.
Table 1. Sample characteristics for the Gen3G birth cohort used for validation.
We observed moderate to high correlation between the measured and estimated nucleated cell types using the recently published reference cord blood panel.Citation3 Namely, a high level of correlation was observed between measured and estimated nRBCs (r = 0.92, R2 = 0.85), lymphocytes (r = 0.77, R2 = 0.58), and granulocytes (r = 0.72, R2 = 0.52). A moderate correlation was observed for monocytes (r = 0.51, R2 = 0.25). We also observed relatively low root mean square errors (rMSE) of the residuals for nRBCs (rMSE = 1.4%), lymphocytes (rMSE = 4.6%), granulocytes (rMSE = 5.4%), and monocytes (rMSE = 2.0%). Linear regression model prediction summaries are presented in and .
Figure 1. Scatter plots and linear regression line for the comparison of the measured (y-axis) and estimated (x-axis) nucleated cell type composition from the cord blood reference panel.
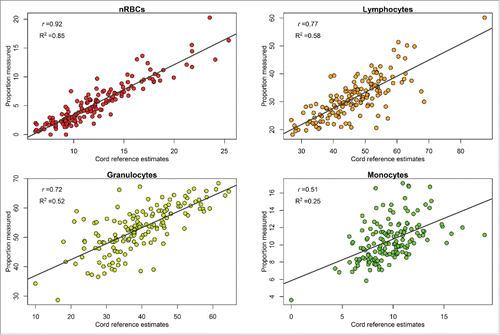
Table 2. Measured and predicted proportions of nucleated cell types using the cord blood reference panel and the adult blood reference panel in the Gen3G birth cohort (n = 154).
Using the adult reference panel to estimate cord blood, leukocyte composition yielded similar results with slightly lower correlations observed for lymphocytes (r = 0.69, R2 = 0.47), granulocytes (r = 0.68, R2 = 0.46), and monocytes (r = 0.44, R2 = 0.19) compared to the estimates obtained from the cord blood reference panel. The rMSE of the residuals were slightly larger when the adult reference panel was used, ranging from 2.1 to 5.7% ().
Although we observed relative high correlation between the measured and estimated nucleated cell types, using either the adult or cord reference panels, the actual predicted distribution of estimated cell types differed, compared to clinically measured values ( and ). Furthermore, different normalization procedures yielded similar results in terms of prediction, but slightly different estimates for the distribution of cell types ( and ). Changing the size of the CpG libraries to include more cell type specific probes (up to 1000 CpGs), selecting both hyper- and hypo-methylated probes, or selecting discriminatory probes based on F-statistic in the cell estimation algorithm did not impact the results (data not shown).
Figure 2. Distributions for the nucleated cell types directly measured in cord blood (red) and the estimates obtained from the cord blood reference panel using the raw DNA methylation data and 5 different normalization methods.
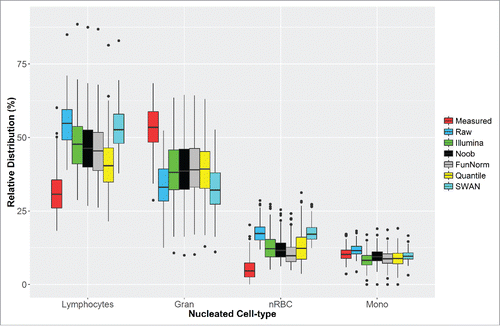
Table 3. Evaluation of normalization methods for the prediction of nucleated cell types in cord blood using the cord blood reference panel, compared to the complete blood count measured cell types in the Gen3G birth cohort (n = 154).
Finally, we performed an epigenome-wide association analysis of gestational age at birth within our cohort to evaluate the impact of model performance after adjusting for cell type composition using the directly measured cell types, the adult reference panel or the cord blood reference panel. After adjusting for child sex and estimated leukocyte composition using the adult reference panel, the genomic inflation factor (λ) was 1.27 in our cohort. The genomic inflation factor was reduced to 1.04 when adjusting for child sex and nucleated cell types, including nRBCs, estimated from the cord blood reference panel. In a similar model adjusted for the directly measured cell types (lymphocytes, monocytes, nRBCs, neutrophils, eosinophils, and basophils) the genomic inflation factor was 1.15. The expected null distribution of P values was better approximated when models were adjusted for the directly measured cell type composition or using the cord blood estimates compared to the adult reference panel ( and supplementary Fig. 1). Using a Bonferroni adjusted threshold for statistical significance (P < 1.02 × 10−7), 11 CpGs were associated with gestational age after adjusting for the directly measured cell type composition, 34 CpGs were associated with gestational age after adjusting for nucleated cells estimated from the cord blood reference panel, and 35 CpGs were found using the adult reference panel adjustment. All of the 11 CpGs found after adjusting for the directly measured cell types were also present and consistent in the direction of association after adjustment of nucleated cells estimated from the cord blood reference panel. However, none of these 11 CpGs reached Bonferroni significance in models adjusted for leukocyte composition using the adult blood reference panel. There was no overlap among the Bonferroni significant CpGs found in models adjusted for cell type composition using the cord or the adult blood reference panels.
Figure 3. Quantile-quantile plot of the log10 (P values) for the epigenome-wide association of gestational age at birth among models adjusted for cell type composition using the adult reference methylation panel, the direct clinically measured cell types, and the cord blood reference methylation panel.
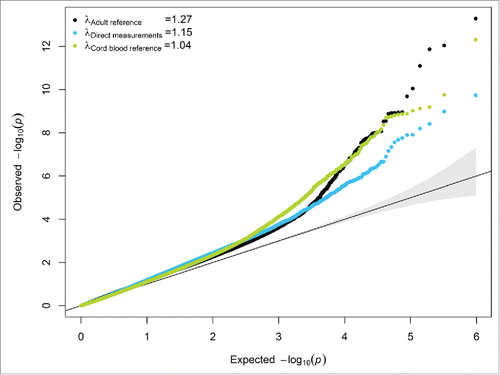
Discussion
The present study evaluates the performance of the newly developed cord blood reference panel for estimating nucleated cell types from DNA methylation data available on commonly used genome-wide arrays. We observed moderate to high degree of co-variation between the estimated and measured cell types in our validation set. We further observed improvement of the genomic inflation factor in the epigenome-wide association analysis for gestational age using the cord blood reference panel to adjust for nucleated cell types in linear regression models. However, the absolute cell type distributions differed considerably between the estimated and measured cell types. The current methodology may be sufficient to estimate relative cell type amounts to control for cell type variation in epidemiological studies, but caution should be used when using the imputed white blood cell composition to make quantitative inferences about immunological parameters in cord blood.
The measured nucleated cell type distribution in our cohort is within previously reported reference ranges for cord blood, suggesting that our results may be generalizable to other cohorts. For example, mean nRBC abundance (nRBCs/100 WBCs) has been previously reported to vary between 4.1–9.2% depending on gestational age and sex of the newborn, and values between 0 to 10% are considered normal.Citation4,7 This is consistent with the mean measured nRBC composition of 5.4% in our study. Granulocytes represented the majority of measured cell types in our sample (mean = 52.9%), consistent with a previous study of 120 healthy newborns where mean cord blood granulocytes (basophils, eosinophils, neutrophils) were reported to be approximately 54.7% of the nucleated cell types.Citation8 Our direct measurements of lymphocytes (mean = 31.3%) and monocytes (mean = 10.5%) were also within agreement with a previous study of 120 healthy newborns were lymphocytes and monocytes were estimated to constitute 35.9 and 8.9% of the nucleated cells in cord blood, respectively.Citation8 The highest correlation and smallest rMSE for the residuals were observed for the comparison of estimated and measured nRBCs. This might be attributed to the fact that discriminatory probes for inferring nRBCs are distinctly hypomethylated (>99 %) compared to all other cell types, highlighting the unique methylation signature of nRBCs.Citation3
Although high correlations between measured and predicted nucleated cell type composition were observed, the current estimation method over-predicts the abundance of lymphocytes and nucleated red blood cells. The estimated proportion of granulocytes was under-predicted. We explored several alternatives to correct for this bias, including multiple normalization processes, selecting more discriminatory probes, and selecting probes based on F-statistic rather than P value. However, none of these approaches adequately corrected for differences in distribution between measured and predicted cell types.
One previous study compared differential cell counts (DCC) in cord blood using manual microscopy and estimated leukocyte composition using the adult reference panel.Citation5 This study found no significant correlations between the manual DCC and the estimated leukocyte composition from the adult reference panel. In contrast, we observed significant moderate correlations between the adult reference panel estimates and the clinically measured leukocyte composition using an automated hematological analyzer. It has been shown that the automated measurement of nucleated cell types using a hematology analyzer is more accurate than the manual DCC when compared to fluorescence activated cell sorting (FACS), considered as the gold standard.Citation9 For example, this study used the same hematological analyzer used for in our validation and reported a root mean square error (RMSE) of 1.46% between FACS and the automated hematological analyzer while the RMSE between the manual DCC and FACS was 2.99%. The accuracy in cell count measurement potentially explains the lack of association between DCC and the adult reference panel estimates previously reported. However, in this previous study utilizing DCC the adult reference panel was reported to overestimate the proportion of lymphocytes and monocytes while underestimating the proportion of granulocytes, consistent with our findings.
A validation study an adult DNA methylation reference panel reported similar correlations for the estimated and measured proportions of lymphocytes (r = 0.61) and monocytes (r = 0.60) as the ones we observed.Citation10 However, this validation study reported higher agreement between measured and estimated cell types for monocytes and lymphocytes among adults. One potential explanation for the differences observed is that the cell type prediction algorithm is based on whole cord blood DNA methylation that might reflect abundance of other cell types not isolated or present in the adult or cord blood reference panels. Other sources of DNA that might not be accurately captured by the automated hematological clinical analyzer include apoptotic cells, haematopoietic stem cells, and cell-free fetal DNA. This is relevant for epidemiological studies as buffy coat, widely used as a source of DNA in birth cohorts, contains haematopoietic and progenitors cells.Citation11 Furthermore, it has been shown that nRBCs can physically interact and adhere to lymphocytes present in the buffy coat.Citation12
nRBCs have been shown to undergo rapid apoptosis with at least half of the nRBCs in maternal blood measured during pregnancy estimated to undergo apoptosis.Citation13 Furthermore, cell free fetal DNA has been detected across different mammals and shown to increase with gestational age in some.Citation14 Another potential source of DNA in cord blood, not included in the available reference panel, are haematopoietic stem cells, which are more abundant in cord blood compared to adult blood or even bone marrow.Citation15 If sufficient amounts of cell-free DNA—from apoptotic nRBC and other sources—or stem cell DNA are present in cord blood, estimates from DNA methylation measurements could yield different cell type distributions. However, we are unable to formally test this hypothesis with our available data.
Furthermore, in the epigenome-wide association of gestational age the lowest genomic inflation factor was observed for the cord blood reference panel even when compared to the direct cell type measurements. This observation supports our hypothesis that additional DNA not originated from the 7 isolated cell types might be source of bias observed between the measured and predicted cell type distributions as the genomic inflation factor is substantially reduced compared to the direct or adult reference panel adjustment. Our aim was to evaluate the impact of cell type adjustment using gestational age as an example, but we did not systematically describe this association. Agreement was observed among the Bonferroni significant CpGs after adjusting for the directly measured cell types and the new cord blood reference panel but there was no agreement for the adult reference panel compared to the other 2 cell type adjustment strategies. Therefore, future studies should evaluate the relationship between DNA methylation, gestational age, and cell type composition.
In conclusion, the moderate to high degree of co-variation between the estimated and measured cell types suggests that the cord blood reference panel cell composition estimates are adequate to control for variations in cell type distribution in epigenome-wide association studies. We also observed an improvement in the genomic inflation factor as well as the distribution of P values when adjusting for the estimated cell types using the cord blood reference panel relative to the adult panel that is commonly used. However, we caution against using the distribution of estimated cell type composition to make inferences about immunological parameters in cord blood. Future studies should develop methodology that quantitatively predicts cell type distribution in cord blood and further evaluate the presence of other sources of DNA in cord blood.
Methods
Study population
Mother and infant pairs were participants in the Genetics of Glucose Regulation in Gestation and Growth (Gen3G), a prospective pre-birth cohort recruited at the Center Hospitalier Universitaire de Sherbrooke (CHUS) in Canada, described in detail previously.Citation16 Expecting mothers were recruited during the first trimester of pregnancy and were eligible for the study if they were 18 y of age or older, had a singleton pregnancy, and were not diagnosed with pre-pregnancy diabetes or diabetes during the first trimester. A total of 154 cord blood samples were available for this analysis with information on both epigenome-wide DNA methylation and direct measures of nucleated white and red blood cells. Study protocols were approved by the CHUS ethics review board and written informed consent was obtained from eligible women.
Measurements of nucleated cells in cord blood
We collected fresh samples of cord blood immediately upon delivery into an EDTA-coated syringe. Samples were transported within an hour of collection to the CHUS hematology lab. We measured absolute cell counts in whole cord blood within an hour and half of sample collection using the XE-5000™ automated hematology system (Sysmex, Canada, Inc.), following the manufacturer's instructions. Briefly, this automated hematology analyzer uses scatter light and fluorescence labeling information to uniquely differentiate cell types.Citation17 This automated analyzer has been shown to perform well compared to other commercial methods or manual microscopy evaluations.Citation18 Data were reported for each sample as absolute counts of nucleated red blood cells (nRBCs), eosinophils, lymphocytes, monocytes, neutrophils, and basophils. The proportion of each cell type was calculated as the count of the cell type divided by the absolute count of all 6 cell types, including nRCBs. We summed the proportion of basophils, eosinophils, and neutrophils to compare to values of granulocytes, as estimated by the cord blood reference panel.
DNA methylation and cell type estimates from minfi
Epigenome-wide DNA methylation measures were performed on cord blood DNA that was extracted from buffy coat and bisulfite converted. Samples were run on the Illumina Infinium HumanMethylation450 BeadChip (Illumina, San Diego, CA). Samples with more than 5% of probes missing were excluded from the analyses; sex-mismatch and duplicate samples were also removed prior to analyses. Samples that passed quality controls were imported into R via the minfi package in Bioconductor.Citation6 The red-green channel set raw data object (rgSet) was used to estimate nucleated cell type distribution using the estimateCellCounts function from minfi. We first estimated cell type composition using the newly developed cord blood reference panel with the default cord blood options, which together processes the experimental and reference methylation data using noob and selects 100 probes per cell type regardless of direction (hyper- or hypo-methylated discriminatory probes can be selected). We also used the adult reference DNA methylation panel to estimate cell type composition using the default processing methods of quantile normalization and selection of 50 hyper- and 50 hypo-methylated discriminatory probes. We further evaluated the impact of modifying the preprocessing methods by using raw (unprocessed) methylation values or 5 different normalization methods available in minfi: Illumina, Noob (cord blood panel default), FunNorm, Quantile, and SWAN. Lastly, we investigated the influence of increasing the number of discriminatory probes to 500 or 1000 CpGs, as well as forcing the cord blood panel to select both hyper- and hypo-methylated probes or selecting discriminatory probes based on F-statistic.
Statistical analysis
We used means and standard deviations (SDs) for numerical variables or counts and proportions for categorical variables to describe demographics of the study sample. To estimate the performance of the prediction for nucleated cell types, we used Person's correlation coefficients (r) and simple linear regression models to estimate the coefficient of determination (R2) as well as the root mean square error (rMSE) of the residuals for each estimated cell type. Estimation performance was evaluated using nucleated cell type composition estimates from the adult or cord reference panels with multiple normalization methods for the DNA methylation data. Scatterplots and boxplots are presented for the estimated and measured proportion of cell types.
To evaluate the impact of adjusting for cell type composition estimated via the adult or cord blood reference panels, an epigenome-wide association study of gestational age at birth was conducted. Namely, we used the limma package to fit linear models of methylation levels for each CpG and gestational age at birth. Models were adjusted for child sex and estimated cell type composition using either the adult reference panel cell estimates, the cord blood reference panel cell estimates, or the directly measured cell type distributions. We compared the effects of cell type adjustment on model performance by calculating the genomic inflation factor (λ) for the 2 different analyses as well as plotting QQ-plots and histograms of the P values for all analyses. All analyses were performed using R 3.3.0 (www.r-project.org/).
Disclosure of potential conflicts of interest
No potential conflicts of interest were disclosed.
KEPI_A_1233091_s02.docx
Download MS Word (364.9 KB)Funding
Fonds de recherche du Québec en santé #20697 (M.-F.H.); Canadian Institute of Health Research #MOP 115071 (M.-F.H.); Diabéte Québec grant (P.P.); ADA Accelerator Award #1-15-ACE-26 (M.-F.H.).
ORCID
Andres Cardenas http://orcid.org/0000-0003-2284-3298
Kelly M. Bakulski http://orcid.org/0000-0002-9605-6337
References
- Houseman EA, Accomando WP, Koestler DC, Christensen BC, Marsit CJ, Nelson HH, Wiencke JK, Kelsey KT. DNA methylation arrays as surrogate measures of cell mixture distribution. BMC Bioinformatics 2012; 13:1; PMID:22214541; http://dx.doi.org/10.1186/1471-2105-13-86
- Reinius LE, Acevedo N, Joerink M, Pershagen G, Dahlén S-E, Greco D, Söderhäll C, Scheynius A, Kere J. Differential DNA methylation in purified human blood cells: implications for cell lineage and studies on disease susceptibility. PloS One 2012; 7:e41361; PMID:22848472; http://dx.doi.org/10.1371/journal.pone.0041361
- Bakulski KM, Feinberg JI, Andrews SV, Yang J, Brown S, McKenney SL, Witter F, Walston J, Feinberg AP, Fallin MD. DNA methylation of cord blood cell types: Applications for mixed cell birth studies. Epigenetics 2016; 11:354-62; PMID:27019159; http://dx.doi.org/10.1080/15592294.2016.1161875
- Hermansen M. Nucleated red blood cells in the fetus and newborn. Arch Dis Child Fetal Neonatal Ed 2001; 84:F211-F5; PMID:11320052; http://dx.doi.org/10.1136/fn.84.3.F211
- Yousefi P, Huen K, Quach H, Motwani G, Hubbard A, Eskenazi B, Holland N. Estimation of blood cellular heterogeneity in newborns and children for epigenome‐wide association studies. Environ Mol Mutagen 2015; 56:751-8; PMID:26332589; http://dx.doi.org/10.1002/em.21966
- Aryee MJ, Jaffe AE, Corrada-Bravo H, Ladd-Acosta C, Feinberg AP, Hansen KD, Irizarry RA. Minfi: a flexible and comprehensive Bioconductor package for the analysis of Infinium DNA methylation microarrays. Bioinformatics 2014; 30:1363-9; PMID:24478339; http://dx.doi.org/10.1093/bioinformatics/btu049
- Glasser L, Sutton N, Schmeling M, Machan J. A comprehensive study of umbilical cord blood cell developmental changes and reference ranges by gestation, gender and mode of delivery. J Perinatol 2015; 35:469-75; PMID:25634517; http://dx.doi.org/10.1038/jp.2014.241
- Suman FR, Raj RSS, Priyathersini N, Rajendran R, Rajendran R, Ramadoss U. Biological reference interval for hematological profile of umbilical cord blood: A study conducted at a tertiary care centre in South India. J Clin Diagn Res 2015; 9:SC07; PMID:26557584; http://dx.doi.org/10.7860/JCDR/2015/14713.6675
- Accomando WP, Wiencke JK, Houseman EA, Nelson HH, Kelsey KT. Quantitative reconstruction of leukocyte subsets using DNA methylation. Genome Biol 2014; 15:1; PMID:24598480; http://dx.doi.org/10.1186/gb-2014-15-3-r50
- Koestler DC, Christensen BC, Karagas MR, Marsit CJ, Langevin SM, Kelsey KT, Wiencke JK, Houseman EA. Blood-based profiles of DNA methylation predict the underlying distribution of cell types: a validation analysis. Epigenetics 2013; 8:816-26; PMID:23903776; http://dx.doi.org/10.4161/epi.25430
- Ademokun J, Chapman C, Dunn J, Lander D, Mair K, Proctor S, Dickinson A. Umbilical cord blood collection and separation for haematopoietic progenitor cell banking. Bone Marrow Transplant 1997; 19:1023-8; PMID:9169647; http://dx.doi.org/10.1038/sj.bmt.1700788
- de Goede OM, Razzaghian HR, Price EM, Jones MJ, Kobor MS, Robinson WP, Lavoie PM. Nucleated red blood cells impact DNA methylation and expression analyses of cord blood hematopoietic cells. Clin Epigenetics 2015; 7:1; PMID:25628764; http://dx.doi.org/10.1186/s13148-015-0129-6
- Sekizawa A, Samura O, Zhen D, Falco V, Farina A, Bianchi DW. Apoptosis in fetal nucleated erythrocytes circulating in maternal blood. Prenat Diagn 2000; 20:886-9; PMID:11113889; http://dx.doi.org/10.1002/1097-0223(200011)20:11<886::AID-PD942>3.0.CO;2-4
- Phillippe M. Cell-free fetal DNA—a trigger for parturition. N Engl J Med 2014; 370:2534-6; PMID:24963574; http://dx.doi.org/10.1056/NEJMcibr1404324
- Kögler G, Sensken S, Airey JA, Trapp T, Müschen M, Feldhahn N, Liedtke S, Sorg RV, Fischer J, Rosenbaum C. A new human somatic stem cell from placental cord blood with intrinsic pluripotent differentiation potential. J Exp Med 2004; 200:123-35; PMID:15263023; http://dx.doi.org/10.1084/jem.20040440
- Guillemette L, Allard C, Lacroix M, Patenaude J, Battista M-C, Doyon M, Moreau J, Ménard J, Bouchard L, Ardilouze J-L. Genetics of Glucose regulation in Gestation and Growth (Gen3G): a prospective prebirth cohort of mother–child pairs in Sherbrooke, Canada. BMJ Open 2016; 6:e010031; PMID:26842272; http://dx.doi.org/10.1136/bmjopen-2015-010031
- Briggs CJ, Linssen J, Longair I, Machin SJ. Improved flagging rates on the Sysmex XE-5000 compared with the XE-2100 reduce the number of manual film reviews and increase laboratory productivity. Am J Clin Pathol 2011; 136:309-16; PMID:21757605; http://dx.doi.org/10.1309/AJCPDLR4KGKAFW4W
- Bruegel M, Nagel D, Funk M, Fuhrmann P, Zander J, Teupser D. Comparison of five automated hematology analyzers in a university hospital setting: Abbott Cell-Dyn Sapphire, Beckman Coulter DxH 800, Siemens Advia 2120i, Sysmex XE-5000, and Sysmex XN-2000. Clin Chem Lab Med 2015; 53:1057-71; PMID:25720071; http://dx.doi.org/10.1515/cclm-2014-0945