ABSTRACT
Given the skyrocketing costs to develop new drugs, repositioning of approved drugs, such as histone deacetylase (HDAC) inhibitors, may be a promising strategy to develop novel therapies. However, a gap exists in the understanding and advancement of these agents to meaningful translation for which new indications may emerge. To address this, we performed systems-level analyses of 33 independent HDAC inhibitor microarray studies. Based on network analysis, we identified enrichment for pathways implicated in metabolic syndrome and diabetes (insulin receptor signaling, lipid metabolism, immunity and trafficking). Integration with ENCODE ChIP-seq datasets identified suppression of EP300 target genes implicated in diabetes. Experimental validation indicates reversal of diabetes-associated EP300 target genes in primary vascular endothelial cells derived from a diabetic individual following inhibition of HDACs (by SAHA), EP300, or EP300 knockdown. Our computational systems biology approach provides an adaptable framework for the prediction of novel therapeutics for existing disease.
Background
Drug repurposing is a process by which existing, clinically approved drugs are repurposed for different conditions. It offers advantages over traditional drug discovery and development, including being time- and cost-effective. However, in order for drug repurposing to be efficient, we must first have an extensive understanding of the cellular and molecular effects of these drugs.
Potential candidates for drug repurposing are histone deacetylase inhibitors (HDACi), which represent a structurally diverse group of compounds that have emerged as important anticancer therapeutics. Although early work focused almost exclusively on antineoplastic properties, HDACi are now being investigated for a host of non-oncological applications that are driven, at least in part, by their potent anti-inflammatory effects.Citation15 For example, pre-clinical models show that HDAC treatment attenuates cardiac hypertrophy,Citation36,53 while SAHA pre-treatment attenuates cardiac ischemia injury.Citation51 In addition, HDAC inhibitors SAHA and givinostat were shown to prevent the development of type 1 diabetes (T1D) in the non-obese diabetic (NOD) mouse model by modulation of the immune system.Citation8
The initial paradigm was that HDACi activate gene expression by hyperacetylating histones, resulting in a more open chromatin confirmationCitation49 while gene suppression was usually attributed to non-histone effects of HDAC inhibition.Citation4 However, the utilization of genome-wide technologies has revealed more complex interactions with the epigenome. In particular, histone deacetylation at the promoters of suppressed genes, mediated by loss of histone acetyltransferase (HATs) binding, was identified as a key feature of pan-HDAC inhibition.Citation38
HDAC inhibitors include drugs from different chemical families and can target different HDAC classes. Pan-HDAC inhibitors, such as hydroxamic acids suberoylanilide hydroxamic acid (SAHA, Vorinostat, Zolinza™) and trichostatin A (TSA), inhibit the activity of Class I, II, and IV, but not Class III, HDAC enzymes.Citation13,30,31 However, compounds such as romidepsin, largazole, and valproic acid (VPA) selectively inhibit Class I HDAC enzymes and there is currently interest in the further development of class- or isoform-specific compounds.Citation13,22,30,31,52 However, despite the diversity in structure of these drugs, they appear to have overlapping clinical applications. For example, both SAHA and romidepsin are approved for the treatment of CTCL, although they target different HDAC enzymes. However, it remains unclear if this diverse group of drugs regulates common gene expression pathways.
To investigate the effects of diverse HDAC inhibitors on the transcriptome, we performed a comprehensive meta-analysis on gene expression profiles in normal, transformed and malignant cell lines, which we then integrated with extensive network analysis (). While network analysis is currently utilized to develop a better understanding of diseases and their progression, the application of this approach to understanding cellular effects of drugs, known as ‘systems pharmacology’, is less widely used. The integration of systems pharmacology with network-based analysis of disease provides a time- and cost-effective solution to repurposing clinically approved drugs for human diseases.Citation7 By utilizing this approach, we observe highly correlated gene activation and suppression signatures, independent of drug class or cell type, which were grouped into broad pathways, including cell cycle, the immune system, and RNA and protein processing and metabolism. Integrative epigenomic analyses combined with transcription factor binding sites (TFBS) derived from the Encyclopedia of DNA Elements (ENCODE) project (2004) show histone deacetylation is strongly associated with the suppression of EP300 target genes. The integration of multiple datasets identified EP300 target genes were associated with complex diseases that were previously implicated in, but not limited to, cancer, and uncovered novel HDAC inhibition networks associated with the removal of acetylation at gene targets functionally linked with diabetes and vascular disease. Thus, to understand the mechanisms of action, we investigated regulatory patterns of expression using primary human aortic endothelial cells (HAECs) of diabetic and non-diabetic origin. We show reversal of diabetes-associated EP300 target genes in diabetic primary cells by SAHA. Our results suggest the integrative analyses we have used to investigate pharmacological HDAC inhibition show complex epigenetic interactions with EP300 and novel therapeutic links relevant to diabetic vascular pathology.
Figure 1. Broad study overview. The overall design of this study has been summarized in 4 steps. We first performed an extensive meta-analysis of public microarray datasets that involved treatment of diverse human cell types with various pharmacological HDAC inhibitors (Step 1). Network analysis was used to broadly profile the pathways altered by HDAC inhibition (Step 2). Of note, we identify consistent changes to the immune system, cell cycle, and signaling pathways. Transcription factor analysis identified EP300 as a key regulator of genes involved in immunity, lipid metabolism, trafficking and insulin receptor signaling following HDAC inhibition (Step 3), pathways that are dysregulated in diabetic vascular disease. Next, we performed ex vivo validation in a diabetic disease model to explore the therapeutic potential of HDAC inhibitors (Step 4).
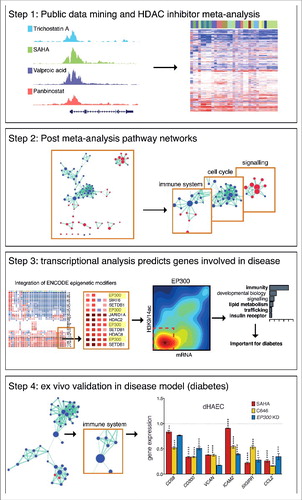
Results
Pharmacological HDAC inhibition segregates gene expression
To determine a representative expression signature, we individually re-analyzed 33 microarray experiments derived from human cells exposed to pharmacological HDAC inhibitors. The studies include isotype specific and pan-HDACi in cancer and primary human cell types. We observed robust expression changes () that include gene activation and suppression (). A heatmap shows strong clustering based on gene expression, irrespective of cell type and drug class (). Spearman's ranks also show strong correlations in gene expression between datasets (), even between structurally unrelated HDAC inhibitors (Figure S1a-d). This initial assessment of the data suggests common gene expression signatures induced by all classes of HDAC inhibitors.
Figure 2. Gene expression changes induced by HDAC inhibition in different human cell types. Differential gene expression for independent human microarray HDACi datasets are summarized. (a) Line plot showing the total number of genes differentially expressed at FDR P values between 0 and 0.05. (b) Pie chart summary of the number of differentially expressed genes (FDR P value <0.05). (c) Heatmap of gene expression changes conferred by HDAC inhibition in human cell types. Each column represents an independent microarray experiment. Blue represents gene activation while red indicates suppression. The intensity of the color correlates with the strength of the P value for any given gene. Columns were normalized by Z-score normalization. (d) Spearman's rank correlation (ρ) of gene expression changes conferred by HDAC inhibitors in different human cell types. Positive correlations are shown in blue while negative correlations are shown in red. A histogram of values and a color key are provided for each heatmap.
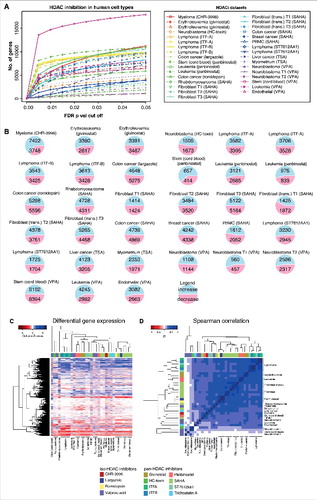
Meta-analysis using Fisher's method for combining P values was used to identify gene expression in response to HDAC inhibition. We identify genes that are either activated or suppressed (Figure S1e) in diverse human cell types. For example, HDAC inhibition increased the expression of Class I (HDAC1, HDAC3), IIA (HDAC5), and Class III (SIRT4, SIRT2) HDACs, while suppressing HATs, including CREBBP and EP300.
In order to determine broad classes of genes consistently regulated by HDAC inhibition, network analysis was performed in combination with Gene Set Enrichment Analysis (GSEA) using REACTOME. We identified common pathways altered by HDAC inhibition and the suppression of genes involved in the cell cycle, immune system, and RNA and protein processing and metabolism (). We observed activation of genes related to signaling and receptor binding (including insulin receptor signaling), telomere packaging, membrane trafficking, and lipid metabolism (). Gene lists were generated from each cluster to define functional gene sets that consistently respond to HDAC inhibition.
Figure 3. Network analysis identifies common pathways altered by HDAC inhibition. Gene set enrichment results for REACTOME pathways altered by HDAC inhibition were visualized using Enrichment Map in Cytoscape (FDR P value <0.05; overlap coefficient cut-off 0.8, combined constant 0.5). Clusters were broadly classified based on biological function. The node colors indicate pathway activation (red) or suppression (blue). Green lines indicate the overlap between two nodes.
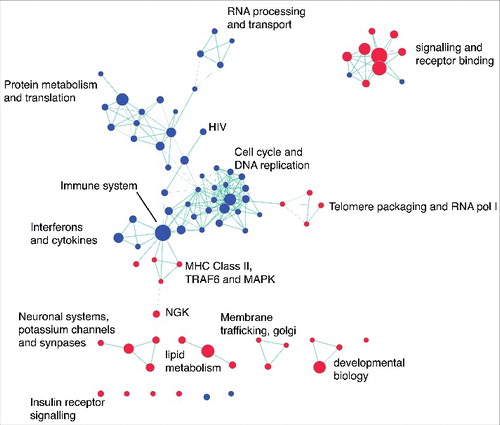
To validate the predictive ability of the meta-analysis, genes identified from the microarray studies were compared to independent RNA-seq and ChIP-seq HDAC inhibitor datasets, obtained from GEO. The datasets include exposure of both cancer and primary cell types to various HDAC inhibitors, including SAHA, TSA, and panbinostat. Using GSEA, we show that top HDAC inhibitor genes derived from the microarray meta-analysis were reproduced in the independent RNA-seq studies (Figure S2a). Next, we compared the gene expression signature from the meta-analysis to histone acetylation data from ChIP-seq. In primary human aortic endothelial cells (HAECs) exposed to SAHA or TSA, genes activated in the meta-analysis were strongly associated with increased histone acetylation, but suppressed genes were only weakly associated with histone deacetylation (Figure S2b). Taken together, these results suggest the expression of genes activated by HDAC inhibition are strongly associated with histone acetylation, whereas gene suppression is likely to be driven by both acetylation dependent and independent mechanisms.
To further explore the role of histone acetylation by HDAC inhibition, we integrated the gene expression (RNA-seq) and histone acetylation (ChIP-seq) data from primary HAECs exposed to SAHA and then intersected this data with the top genes activated or suppressed by HDAC inhibition as determined by the meta-analysis. We confirmed that genes activated in the meta-analysis were indeed activated and acetylated by SAHA in HAECs (, gated box of 426 genes). We also show genes suppressed in the meta-analysis are generally suppressed and deacetylated in HAECs exposed to SAHA (, gated box of 460 genes). Genes in each gated box were classified based on the gene sets defined from the REACTOME network clusters from (). Genes involved in trafficking, neuronal systems, metabolism, and signaling (including insulin receptor signaling) were associated with increased expression and acetylation. In contrast, genes involved in RNA processing, DNA repair, nucleotide metabolism, and the cell cycle were more likely to be suppressed and have reduced promoter acetylation. Genes involved in immunity (including infection and MAPK related pathways), although representing the most number of genes, were equally likely to be increased or decreased by SAHA.
Figure 4. Top genes predicted by meta-analysis are consistent with NGS gene expression and histone acetylation HDACi datasets. RNA-seq and ChIP-seq data from non-diabetic HAECs exposed to SAHA was obtained from GEO (GSE37378). A density plots shows the relationship between gene expression (RNA-seq) and histone acetylation at promoters (ChIP-seq) in response to SAHA in HAECs for the top 1000 (a) activated and (b) suppressed HDACi response genes as defined by the meta-analysis (legend: relative gene density). The gated red boxes highlight genes with (a) increased gene expression and acetylation or (b) decreased gene expression and acetylation by SAHA in HAECs. These genes were then classified based on (c) broad functional classes defined by the network analysis.
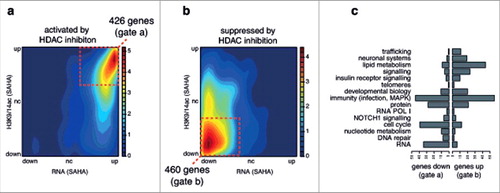
HDAC inhibition suppresses EP300 target genes
Next, to determine pathways regulated by HDAC inhibition for each microarray dataset, we utilized GSEA with the MSigDB gene set database.Citation46 We observe a strong association of regulatory pathways altered by iso- and pan-selective HDAC inhibitors in multiple human cell types. Pathway meta-analysis shows a strong correspondence for activated and suppressed gene sets. Since HDAC inhibition alters co-regulators of gene transcription,Citation38 we integrated transcription factor binding sites (TFBS) using ChIP-seq data sets originating from the ENCODE project.Citation1 We show candidate transcription factors as drivers of gene expression following HDAC inhibition (Figure S2c). Transcription factors were ranked and short-listed by meta-analysis using Fisher's method for combining P values. We identified EZH2, a PRC2 subunit and methyltransferase, as a regulatory component of activated target genes. In contrast, EP300 was strongly associated with gene suppression () and consistent with the notion that SAHA and TSA regulate histone deacetylation of EP300/CREBBP target genes.Citation38
Figure 5. EP300 target genes are associated with gene suppression following HDAC inhibition in human cell types. GSEA ENCODE-TF results associated with gene expression changes induced by HDAC inhibitors in multiple cell lines for (a) gene sets associated with epigenetics modifiers. GSEA was used to compare changes in expression induced by HDAC inhibition in human cell types to microarray gene expression signatures association with the knock down of (b) EP300 (GSE31873) and CREBBP (GSE50588). Blue represents gene sets associated with gene activation while red indicates gene sets associated with gene suppression. The intensity of the color correlates with the NES scores. Pathways with a FDR P value >0.05 are shown in white. (c) The relationship between gene expression (RNA-seq) and histone acetylation (ChIP-seq) for EP300 target genes (from the ENCODE project) are represented as density plots for HAECs exposed to SAHA (legend: relative gene density). (d) EP300 target genes with suppressed expression and reduced acetylation (red box) by SAHA in HAECs were classified based on biological function as defined by the network analysis.
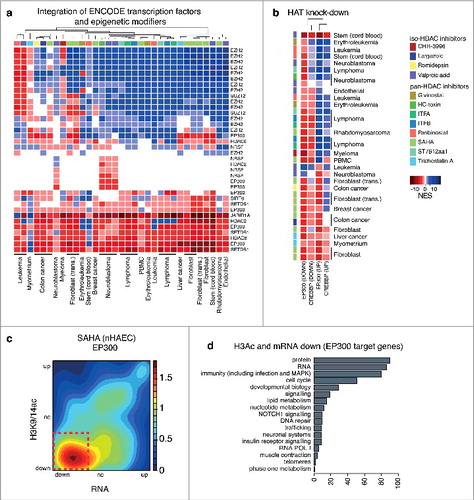
Since EP300 regulates histone acetylation and gene expression, our observation of deacetylation at EP300 target genes was unexpected. Using gene sets derived from re-analyzed gene expression patterns derived from EP300 and CREBBP knockdown (KD) studies obtained from GEO, we used GSEA to show that genes suppressed following EP300 and CREBBP KD are strongly correlated with genes suppressed by pharmacological HDAC inhibition (). We found suppressed EP300 target genes were strongly associated with histone deacetylation by the hydroxamic acids SAHA () and TSA (Figure S2d-e). Classifications of genes by function show biological processes that include RNA and protein processing, immunity and inflammation, the cell cycle and lipid metabolism, suggesting that EP300 is a critical driver of gene expression of key pathways altered by HDAC inhibition (, Figure S2f).
Deacetylation of diabetes-associated EP300 target genes
Based on the pathways associated with HDAC inhibition and published pre-clinical and clinical trials, we identified a number of diseases that could potentially be treated by HDAC inhibition. These include cancer (cell cycle, telomeres, DNA repair), epilepsy, Huntington's and Alzheimer's disease (neuronal systems), inflammatory disorders and infection (immunity, signaling), cardiovascular disease (inflammation, developmental biology), and metabolic disorders such as metabolic syndrome and diabetes (insulin receptor signaling, lipid metabolism, immunity and trafficking). We questioned whether HDAC inhibition could regulate the expression of genes implicated in diabetic vascular disease, in an EP300-dependent manner. To do this, primary human aortic endothelial cells derived from non-diabetic (nHAEC) and type 1 diabetic (dHAEC) individuals were exposed to HDAC inhibition. We selected SAHA, an FDA approved drug, previously shown to regulate inflammatory gene expression via EP300-dependent histone deacetylation in endothelial cells.Citation38 Network analysis confirmed suppression of pathways related to the cell cycle, the immune system, and RNA and protein metabolism in diabetic HAECs treated with SAHA ().
Figure 6. Network analysis identifies pathways altered by SAHA in diabetes HAECs. Gene set enrichment results for REACTOME pathways altered by SAHA treatment in HAECs cells of diabetic origin were visualized using Enrichment Map in Cytoscape (FDR P value <0.05; overlap coefficient cut-off 0.8, combined constant 0.5). Clusters were broadly classified based on biological function. The node colors indicate pathway activation (red) or suppression (blue). Green lines indicate the overlap between two nodes.
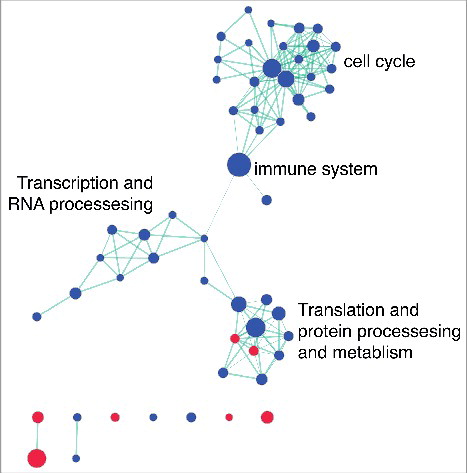
Next, we examined the role of EP300 using the small molecule inhibitor C646 as well as somatic knockdown using EP300 siRNA. Gene expression profiles from non-diabetic and diabetic HAECs exposed to SAHA are highly correlated (r = 0.9) (, Figure S3a). Gene expression response following C646 is also highly correlated between non-diabetic and diabetic HAECs (r = 0.89) (). In contrast, the correlation (r = 0.79) between non-diabetic and diabetic HAECs following EP300 KD by siRNA was not as strong ().
Figure 7. Inhibition of HATs and HDACs mediates robust changes in gene expression. (a) Correlation plots with the linear model and Pearson's correlation for non-diabetic (nHAEC) and diabetic HAECs (dHAEC) exposed to SAHA, C646 (pharmacological EP300/CREBBP inhibitor) or EP300 siRNA. (b) Density plots show the relationship between gene expression (RNA-seq) and histone acetylation at promoters (ChIP-seq) for all genes and the top 1000 genes suppressed by SAHA, C656 or EP300 KD in diabetic HAECs (legend: relative gene density).
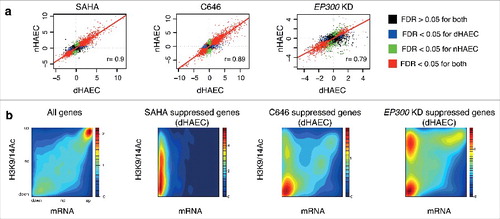
Furthermore, we identify genes suppressed by both HDAC and HAT inhibition (Figure S3b). To confirm deacetylation of EP300 target genes, we compared gene suppression patterns from SAHA, C646 and EP300KD experiments in diabetic HAECs with independent RNA-seq and histone acetylation ChIP-seq derived from non-diabetic HAECs stimulated with SAHA (). These data show genes suppressed by SAHA and C646 in diabetic HAECs strongly correlate with histone deacetylation in non-diabetic HAECs. We also observe a similar association for EP300 KD, supporting the notion that EP300 target genes deacetylated by SAHA are implicated in diabetes.
We hypothesize that pharmacological HDAC inhibition may reverse gene expression patterns in diabetic HAECs by an EP300 dependent mechanism. To test this hypothesis, we inhibited HDAC activities using SAHA and examined genes activated in diabetes in dHAECs (). We identified three distinct signatures for diabetes-associated genes. The first cluster shows diabetes genes suppressed by SAHA, C646, and EP300 KD. These results support a possible role for acetylation by EP300 in diabetic gene activation and the notion of functional reversal by SAHA. The second cluster consists of genes suppressed by SAHA and C646, but not EP300 KD, suggesting a role for HATs other than EP300, such as CREBBP. Finally, in clusters 3 and 4 we observe genes suppressed by SAHA that are regulated independently of EP300 (). In summary, these results suggest that SAHA inhibits the inflammatory expression signature in diabetic HAECs by an EP300 dependent mechanism.
Figure 8. SAHA reverses gene expression in diabetic HAECs by EP300 dependent mechanism (a) A heatmap showing genes that are upregulated in diabetes and that are suppressed by SAHA in this dataset. Clustering identifies diabetic gene expression likely to be EP300-dependent or independent. Validation of gene expression was performed by RT-PCR. (b) Relative expression of genes associated with diabetes in diabetic HAECs relative to non-diabetic HAECs. (c) Relative expression of genes associated with diabetes in response to SAHA or C646 (relative to DMSO control) or (d) EP300 siRNA (relative to non-target siRNA). Gene expression was determined by normalizing against the housekeeping gene HPRT1, which was stably expressed. Error bars represent SEM. n = 3, (*) P value <0.05, (**) P value <0.005, (***) P value <0.0005, (****) P value <0.0001, unpaired t-test.
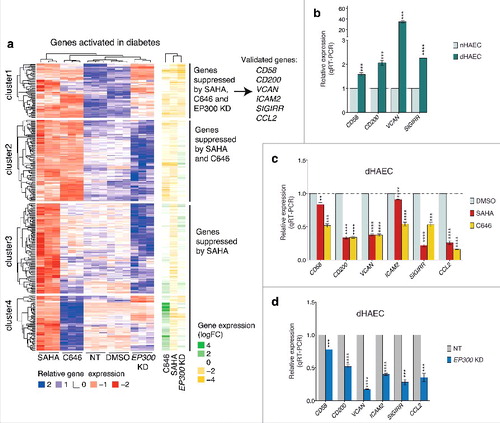
SAHA reverses the expression of EP300 target genes in diabetic HAECs
To validate the role for EP300 in diabetic HAECs identified from our integrative meta-analysis, we selected candidate genes that were activated in diabetes and suppressed by EP300KD in diabetic HAECs. The expression of VCAN, CD58, CD200, and SIGIRR genes were upregulated in diabetic HAECs (). For example, VCAN gene expression was highly ranked with an almost 40-fold increase using qRT-PCR. This gene encodes versican, a large extracellular matrix proteoglycan involved in inflammation, and implicated in diabetes.Citation2,54 We observed increased H3K9/14ac at the VCAN gene promoter (Figure S4). The cell adhesion molecule CD58, which is involved in immunoregulationCitation34 and CD200, a cell surface molecule involved in endothelial T-cell interactions,Citation24 were also activated, as was the immunoregulatory protein SIGIRR.Citation16 Exposure of dHAECs to SAHA and C646 reversed VCAN, CD58, CD200, and SIGIRR gene expression (). Indeed, we observe greater than 50% reduction in the expression of CD200, VCAN, and SIGIRR by SAHA and C646. The expression of CD58 was suppressed more strongly by C646 compared to SAHA (). Furthermore, we also observe strong suppression of the adhesion molecule ICAM2Citation29 and pro-inflammatory chemokine CCL2Citation42 by both SAHA and C646. These findings show that both SAHA and C646 suppress the expression of pro-inflammatory genes in diabetic cells, suggesting a EP300-dependent mechanism.
To test this hypothesis, we examined whether knockdown of EP300 in dHAECs would suppress diabetes-related genes. We show suppression of the genes CD58, SIGIRR, CD200, ICAM2, and CCL2 is consistent with gene suppression induced by SAHA exposure (). In particular, VCAN was more susceptible to suppression by EP300KD than by SAHA or C646 exposure. Collectively, these findings suggest that gene suppression mediated by SAHA is dependent on EP300 in diabetic endothelial cells.
Discussion
HDAC inhibitors are a diverse group of epigenetic modifying drugs with potent gene regulatory properties. Three compounds, SAHA, romidepsin, and panbinostat, are currently FDA approved.Citation35 Many other drugs are in phase II clinical trials for both hematological and solid tumors. While these compounds are most well known for their antineoplastic properties, there is less data available for their applicability in non-oncological diseases. For example, VPA has a long-standing history as an antiepileptic drug, while other agents that inhibit HDAC activity show pre-clinical applicability in diabetes and cardiovascular disease.Citation8,25,36
Current clinical use indicates some consistency in the therapeutic potential of diverse HDAC inhibitors. For example, SAHA and romidepsin, two structurally unrelated HDAC inhibitors, are both currently approved for the treatment of cutaneous T-cell lymphoma, while panbinostat and belinostat, both hydroxamic acids like SAHA, are in late stage clinical trials for the same malignancy. This suggests that HDAC inhibitors may regulate common pathways, despite diversity in drug class or chemical structure. To test this hypothesis, we integrated thirty-three microarray studies for gene expression changes conferred by a diverse group of pharmacological agents. Despite the diversity of drug and cell types, we observe comparable gene expression changes in response to HDAC inhibition. The strong overlap of gene expression changes conferred by pan- and Class I-specific HDAC inhibitors implicates Class I HDAC enzymes, rather than Class II, as potential drivers of gene expression. This is likely associated with the ubiquitous expression of Class I HDACs in human cell types. In contrast, Class II enzymes are cell type specific. For example, Class IIA HDAC expression is generally limited to smooth muscle, the brain and heart. Therefore, detection of expression signatures mediated by inhibition of this class of HDACs would not be possible using the publically available datasets access from GEO.
Consortium-based studies have shown the power of integrative approaches to multiomics. For example, the ENCODE project is the quintessential integrative analysis of transcriptomic and epigenomic datasets that have been highly informative of chromatin biology and gene function.Citation1 The Genotype-Tissue Expression (GTEx) project has characterized inter-individual transcriptomic variation across different tissuesCitation32 and has identified how genetic variation can affect gene expression.Citation9 Collectively, such consortia are a powerful resource that can be leveraged by computational biologists. For example, one study utilizing data from the ENCODE project identified that changes in gene expression between individuals were associated with genetic variants that altered chromatin accessibility and transcription factor binding.Citation11
Currently, meta-analyses are widely used for combining clinical datasets and to gain deeper insights into biological questions. For example, a recent GWAS meta-analysis of more than 200,000 individuals identified novel genetic loci implicated in body fat distribution.Citation44 While there is no doubt that large datasets are informative for GWAS studies, there is still very little integration investigating epigenomic and regulatory mechanisms. The rapid generation of microarray and high throughput sequencing data available on public depositories in recent years provides a valuable resource to researchers, especially in the context of meta-analyses, pathway analysis, and systems biology.
Complementary to a systems biology understanding of disease, is the extension of network-based approaches to gain a deeper appreciation of the transcriptional and cellular effects of drugs. The integration of this ‘systems pharmacology’ approach with network-based analysis of disease provides a rational, cost-effective way and evidence based solution to repurposing clinically approved drugs for human diseases.Citation7 This is a viable and cost-effective alternative to the costly and time-consuming development of new drugs. Furthermore, this approach could also provide a rational approach to drug combinatorial approaches, as well as the prediction of drug-drug interactions and off-target effects.Citation28 Furthermore, the rapidly declining cost of high throughput sequencing presents an opportunity for personalized medicine.Citation3 For example, an in silico computational approach was utilized to predict that the anticonvulsant drug topiramate could treat inflammatory bowel disease.Citation14 More recently, a similar approach identifies that dexamethasone may inhibit the activity of EGR, an oncogenic transcription factor.Citation17
HDAC inhibitors, in particular, are excellent candidates for integrative meta-analysis given their extensive molecular characterization and the diverse compounds that exist in this class. Our meta-analysis approach integrated data from the ENCODE project with microarray expression profiles to show EP300 target genes suppression by HDAC inhibition, including genes implicated in diabetes.
Our findings of histone deacetylation suggest altered EP300 activity following HDAC inhibition. Currently, the mechanism by which HDAC inhibition alters EP300 activity remains unclear. However, we know that EP300 can auto-acetylate and acquire acetylation by other HATs,Citation6,45 and is deacetylated by HDAC enzymes.Citation6,48 While early studies suggest that auto-acetylation of EP300 enhances its activity and stimulates interactions with other proteins,Citation18,45 more recent evidence suggests that auto-acetylation of EP300 impairs its ability to acetylate K18 on histone H3.Citation55 Although the exact effects of EP300 acetylation status remain unclear, we propose that altered EP300 acetylation following the inhibition of HDAC enzyme activity may account for the histone deacetylation patterns observed in our study. Furthermore, EP300 auto-acetylation may also indirectly regulate gene expression via its altered interactions with other transcription factors.
As for the importance of chromatin modification, several regulatory determinants involved in histone acetylation have been described in the regulation of insulin resistance and diabetes.Citation43 For example, the activation of mouse insulin resistance genes is associated with both increased H3K27 acetylation and increased glucocorticoid receptor recruitment at gene enhancers in adipocytes.Citation21 Furthermore, the activity of HDAC3 is elevated in peripheral blood mononuclear cells derived from individuals with type 2 diabetes when compared to non-diabetic controls.Citation41 These results suggest changes to histone and non-histone substrates conferred by HDAC3 could be involved in the pathogenesis of diabetic complications. In addition, HDAC inhibition is associated with increased angiogenesis in endothelial cells.Citation20 In a previous study, we show the suppression of pro-inflammatory genes is mediated in part by EP300 activity.Citation38 In the current study, we extend these results showing pharmacological inhibition of HDAC activity is associated with histone deacetylation at EP300 target genes implicating the reversal of pro-inflammatory gene expression in diabetic endothelial cells. While the clinical implications for direct EP300 inhibition remains to be functionally confirmed, the current study adds to the growing body of literature of the potential therapeutic benefit of HDAC inhibitors in non-malignant disease.
While the datasets assessed in this study were derived from malignant cell types, our findings were informative of therapeutic potential in non-malignant disease. Recent studies have shown therapeutic benefit of HDAC inhibitors in diseases other than cancer. For example, SAHA and givinostat prevent the development of type 1 diabetes in a pre-clinical animal model.Citation8 In that study, acetylation of the NFKB subunit p65 is linked to suppression of genes involved with inflammation. While that study focused on the prevention of type 1 diabetes in mice, our approach examined primary human vascular endothelial cells derived from diabetic and non-diabetic individuals. Endothelial cells form a critically important and highly functional barrier between the circulation and the blood vessel wall.Citation39 Endothelial cells are also among the first non-hematopoietic cells exposed to extracellular stimulation derived from blood, including medication, but also disease-causing stimuli such as elevated blood glucose. In particular, prolonged hyperglycemia can lead to endothelial dysfunction and is important in the progression of diabetic complications and the development of atherosclerosis.
Our studies identified pathways that are regulated by HDAC inhibition, with implications for novel drug repurposing for different diseases. For example, we show strong regulation of neuronal systems, implicating that HDACi may have therapeutic potential as treatments for neurological disorders. Indeed, recently, in an animal model of Alzheimer's disease involving severe amyloid deposition, treatment with SAHA improved spatial reference memory without altering plaque load.Citation4 Further integrative analysis of genome-wide expression and histone acetylation data revealed that SAHA rescued the expression of genes associated with synaptic plasticity and inflammation. HDAC inhibitors are also protective in models of Huntington's disease.Citation19 Collectively, these findings suggest that our approach to studying HDAC inhibition may be informative for identifying potential drug-diseases pairs.
While this study provides important insights into HDAC inhibition, the lack of diverse datasets limited the scope of our meta-analysis. For example, HDAC inhibitors are known to induce apoptosis at much higher rates in cancer and immortalized cell types when compared to primary cells. However, due to a lack of studies in primary cell types, comparison between HDAC inhibition in primary versus malignant cells was not feasible. We could not compare Class I and II HDAC inhibitors because many of the studies in GEO used pan- or Class I-specific HDAC inhibitors. RNA-seq and ChIP-seq datasets from these studies were also limited, and while we had access to H3K9/14ac profiles following HDAC inhibition, other histone acetylation marks such as H3K27ac were not assessed. Unlike H3K9/14ac, which is predominantly localized at gene promoters, H3K27ac regulates gene expression via acetylation of histones localized at both promoters and enhancers. In addition, further studies are required to investigate the acetylation status of EP300 post-HDAC inhibition. With the recent advances in ChIP sequencing technologies in non-malignant disease we believe more comprehensive meta-analysis will be achievable.
In summary, our integrative systems biology approach examining pharmacological HDAC inhibition identified key pathways regulated by SAHA with implications for novel therapeutic applications. As major consortia continue to grow, the integrative approach to meta-analysis and transcription factor analysis presenting in this paper will become increasingly relevant to further elucidating mechanistic pathways.Citation23 Furthermore, while cellular and molecular pathways, such as the cell cycle and immunity, are well defined by databases, such as REACTOME and KEGG, extensive disease-specific databases that integrate new and existing expression datasets are still lacking. As the field moves forward, establishing a better understanding of genomic and epigenomic mechanisms of drug action and disease etiology via systems biology approaches will be key for the repurposing of pharmaceutical therapies for existing diseases as well as rational drug design.
Experimental procedures
Screening for public HDAC inhibitor datasets
GEO (gene expression omnibus) was mined for independent HDAC inhibitor microarray or next generation sequencing using the ‘series’ filter. The search terms “HDAC inhibitors”, “HDAC inhibition” and “HDACi” were used for human datasets (searches performed between the 1st and 6th of May, 2014 and on the 21st of April 2015).
General inclusion criteria
Independent experiments in primary and transformed human cells were included. Tissue types include whole organs, organ components, or tumors. Pharmacological exposure to isotype specific and pan-HDAC inhibitors was included, with no restrictions on length or dose of exposure. Experiments involving exposure to a combination of HDACi were also included.
General exclusion criteria
HDAC gene knockout/down and co-exposure to a non-HDACi drug or siRNA/shRNA were not included.
Additional exclusion criteria for microarray
Gene expression datasets that match the following criteria were excluded from analysis: two-channel microarrays, experiments with no replicates (minimum n = 2), data that is not normalized, and arrays other than gene arrays (exon, miRNA, ChIP arrays). Datasets with less than 50 genes with a FDR P value of less than 0.05 were also excluded. Further details are described in the Supplementary Experimental Procedures.
Public microarray and NGS datasets included for meta-analysis
A total of 13 different isotype specific and pan-HDAC inhibitors are represented in this study (Table S1). The six Class I specific HDAC inhibitors are VPA, romidepsin, largazole, CHR-3996, ITF-A and ITF-B. The seven pan-HDAC inhibitors include TSA, SAHA, panbinostat, givinostat, HC-toxin, sodium butyrate (NaB), and ST7612AA1.
Differential gene expression of public microarray data
Thirty-three human microarray datasets were included for meta-analysis (Table S2). Normalized microarray data were obtained from GEO and analysis was performed using the limmaCitation47 and GEO2R (default parameters). Differential gene expression used the Benjamini-Hochberg adjusted FDR P value <0.05Citation5 with fold change greater than log2(1.2). Probes not annotated by gene name were removed from the analysis.
Differential gene expression and histone acetylation of public NGS data
NGS datasets analyzed are summarized in Table S3. For each study, data obtained in SRA format was converted to fastq using fastq-dump (sratoolkit.2.3.5-2-ubuntu64). RNA-seq: fastq files were aligned to the hg19 RefSeq transcriptome (download from UCSC) using the Burrows-Wheeler Aligner, (BWA).Citation26 A matrix was generated to summarize transcript reads and edgeR software was used to determine differential gene expression (Robinson et al.,Citation40 Significance is defined as FDR P value <0.05. ChIP-seq: fastq files were aligned to the hg19 (human) or mm9 (mouse) RefSeq genomes using BWA. Reads from each sample were counted at gene promoters (2.5 kb either side of the TSS) using bedtoolsCitation37 and edgeR was used to determine differential histone acetylation. Significance is defined as FDR P value <0.05.
Gene set enrichment analysis
GSEA was used for pathway and transcription factor analysis.Citation46 Transcripts from microarray and NGS expression data were assigned a score based on the negative log10 of the P value multiplied by the sign of the fold change (–logP*signFC). GSEA was run using classical scoring with 1000 permutations. Only gene sets between 30 and 2000 genes were included in the analysis, with the exception of GSEA on MSigDB pathways (version 4) (<1000 genes). For transcription factor analysis, gene sets of transcription factor target genes were generated from ENCODE transcription factor binding site (TFBS) ChIP-seq bed files. TFBS bed file represents a list of genome regions targeted by transcription factor binding. Target genes were defined as possessing a TFBS within 3 kb either side of the TSS.
Network analysis
GSEA of REACTOME pathways was performed on a single rank file generated from the gene expression results from the meta-analysis. REACTOME pathways altered by HDAC inhibition were visualized using Enrichment MapCitation33 in Cytoscape (FDR P value <0.05; overlap coefficient cut-off 0.8, combined constant 0.5). The REACTOME database was selected because it is a comprehensive curated database of human pathways used for pathway analysis.Citation10,50
Analysis
Integrative analyses for gene expression, network pathways and ENCODE (TFBS) transcription factor binding sites (using GSEA) were performed using Fisher's method for combining P values from the R package MADAM. To perform Fisher's test, a matrix of P values for genes derived from all datasets was generated. To test for gene activation, P values for suppressed genes were replaced by a P value of 1 (to indicate that they are not activated) and Fisher's method was performed on the matrix. To test for gene suppression, all P values for activated genes were replaced with 1 (to indicate that they are not suppressed) and Fisher's method was performed on the matrix. Because this test is performed twice, some genes may be identified as both activated and suppressed, and were excluded. Genes that are not represented in at least 70% of arrays (i.e., are not included in the microarray platform) were excluded from the test. Furthermore, genes not represented in pan- and iso-HDACi datasets were excluded from analysis (minimum requirement of 30% of datasets pan-HDACi and 30% iso-HDACi). Results are reported as the S-statistic and the FDR P value.
Primary human cells
Human aortic endothelial cells (HAEC) from type 1 diabetic (CC-2919, Lonza) and non-diabetic (CC-2535, Lonza) individuals, obtained at autopsy, were maintained in endothelial cell basal growth media-2 (EBM-2, Lonza) with additional EBM-2 growth factors and supplements and with 10% heat-inactivated fetal bovine serum (Gibco). HAECs used in this study were between 5–9 passages. Cells were exposed to DMSO, SAHA (2 μM) or C646 (10 μM) for 12 hours. EP300 knockdown was generated using ON-TARGETplus Human EP300 (2033) siRNA – SMARTpool (L-003486-00-0005, Dharmacon). Cells were cultured in Opti-MEM (Life Technologies) media with Lipofectamine RNAiMAX Reagent (13778-075, Life Technologies) with either non-target (ON-TARGETplus Non-targeting Pool, D-001810-10-05, Dharmacon) or 25 nM EP300 siRNA for four hours. Media was replaced with EBM-2 and RNA was harvested after 48 hours.
Quantitative Reverse Transcriptase-PCR (qRT-PCR) amplification was performed using a 7500 Fast Real-Time PCR System (Applied Biosystems). cDNA was synthesized from extracted RNA using a High-Capacity cDNA Reverse Transcription Kit using manufacturers protocol (Applied Biosystems). FAST SYBR Green Master Mix (Roche), cDNA template and 5 pmoles of forward and reverse primer were mixed to a final volume of 20 μl. Reactions were incubated for 10 minutes at 95°C, followed by 40 cycles of 95°C for 10 seconds and 60°C for 30 seconds. Gene expression changes were determined by normalizing against HPRT1.
RNA sequencing and analysis
Total RNA was extracted in TRIzol (Invitrogen) and purified using the Direct-zol RNA MiniPrep kit (cat. no: R2052, Zymo Research) according to the manufacturer's instructions. RNA was quantified on the MultiNA Bioanalyzer (Shimadzu). NEBNext Poly(A) mRNA Magnetic Isolation Module was used to enrich mRNA from 1 μg of total RNA. The NEBNext Ultra Directional RNA Library Prep Kit for Illumina was used to generate barcodes. Libraries were quantified on the MultiNA Bioanalyzer (Shimadzu) and pooled to equimolar ratios for sequencing. Cluster generation was performed at a concentration of 12 pM (TruSeq SR Cluster Kit v4-cBot-HS) and the flow cell was run on Illumina HiSeq2500 generating 100 nt reads. Fastq files were aligned to the hg19 human genome (ensembl GRCh37 release 75) using STAR.Citation12 The tool featureCountsCitation27 was used to generate a matrix of read counts per gene and the edgeR software was then used to determine differential gene expression.Citation40 Sex-linked genes were removed from the analysis. Significance is defined as FDR P value <0.05.
Conflict of interest
There is no conflict of interest to disclose.
Data access
Original RNA-seq data can be accessed from the NCBI Gene Expression Omnibus (GEO) with the accession number GSE77108.
Consent for publication
Not applicable.
Author contributions
H.R. and A.E. designed the study. H.R., J.O. and M.Z. carried out the experiments. H.R. performed the bioinformatics analysis with support from A.K. and M.Z. T.C.K. supported experimental design and manuscript writing. H.R. and A.E. wrote the manuscript.
El_Osta_Supplementary_Methods_and_Results_1_1_.docx
Download MS Word (1.2 MB)Supplementary_Info.docx
Download MS Word (1.2 MB)Acknowledgments
We thank Dr. Ross Lazarus for bioinformatics support. We also acknowledge grant and fellowship support from the National Health and Medical Research Council (NHMRC). Supported in part by the Victorian Government's Operational Infrastructure Support Program.
References
- The ENCODE (ENCyclopedia Of DNA Elements) Project. Science. 2004;306:636-640. doi:10.1126/science.1105136. PMID:15499007.
- Andersson-Sjoland A, Hallgren O, Rolandsson S, Weitoft M, Tykesson E, Larsson-Callerfelt AK, Rydell-Tormanen K, Bjermer L, Malmstrom A, Karlsson JC, et al. Versican in inflammation and tissue remodeling: the impact on lung disorders. Glycobiology. 2015;25:243-251. doi:10.1093/glycob/cwu120. PMID:25371494
- Ashley EA, Butte AJ, Wheeler MT, Chen R, Klein TE, Dewey FE, Dudley JT, Ormond KE, Pavlovic A, Morgan AA, et al. Clinical assessment incorporating a personal genome. Lancet. 2010;375:1525-1535. doi:10.1016/S0140-6736(10)60452-7. PMID:20435227
- Benito E, Urbanke H, Ramachandran B, Barth J, Halder R, Awasthi A, Jain G, Capece V, Burkhardt S, Navarro-Sala M, et al. HDAC inhibitor-dependent transcriptome and memory reinstatement in cognitive decline models. The Journal of clinical investigation. 2015;125:3572-3584. doi:10.1172/JCI79942. PMID:26280576
- Benjamini Y, Hochberg Y. Controlling the false discovery rate: a practical and powerful approach to multiple testing. J Roy Statist Soc Ser B (Methodological). 1995;57:289-300.
- Black JC, Mosley A, Kitada T, Washburn M, Carey M. The SIRT2 deacetylase regulates autoacetylation of p300. Molecular cell. 2008;32:449-455. doi:10.1016/j.molcel.2008.09.018. PMID:18995842
- Chan SY, Loscalzo J. The emerging paradigm of network medicine in the study of human disease. Circ Res. 2012;111:359-374. doi:10.1161/CIRCRESAHA.111.258541. PMID:22821909
- Christensen DP, Gysemans C, Lundh M, Dahllof MS, Noesgaard D, Schmidt SF, Mandrup S, Birkbak N, Workman CT, Piemonti L, et al. Lysine deacetylase inhibition prevents diabetes by chromatin-independent immunoregulation and beta-cell protection. Proc Natl Acad Sci U S A. 2014;111:1055-1059.
- Consortium GT. Human genomics. The Genotype-Tissue Expression (GTEx) pilot analysis: multitissue gene regulation in humans. Science. 2015;348:648-660. doi:10.1126/science.1262110. PMID:25954001
- Croft D, O'Kelly G, Wu G, Haw R, Gillespie M, Matthews L, Caudy M, Garapati P, Gopinath G, Jassal B, et al. Reactome: a database of reactions, pathways and biological processes. Nucleic acids research. 2011;39:D691-697. doi:10.1093/nar/gkq1018. PMID:21067998
- Degner JF, Pai AA, Pique-Regi R, Veyrieras JB, Gaffney DJ, Pickrell JK, De Leon S, Michelini K, Lewellen N, Crawford GE, et al. DNase I sensitivity QTLs are a major determinant of human expression variation. Nature. 2012;482:390-394. doi:10.1038/nature10808. PMID:22307276
- Dobin A, Davis CA, Schlesinger F, Drenkow J, Zaleski C, Jha S, Batut P, Chaisson M, Gingeras TR. STAR: ultrafast universal RNA-seq aligner. Bioinformatics. 2013;29:15-21. doi:10.1093/bioinformatics/bts635. PMID:23104886
- Dokmanovic M, Clarke C, Marks PA. Histone deacetylase inhibitors: overview and perspectives. Molecular cancer research: MCR. 2007;5:981-989. doi:10.1158/1541-7786.MCR-07-0324. PMID:17951399
- Dudley JT, Sirota M, Shenoy M, Pai RK, Roedder S, Chiang AP, Morgan AA, Sarwal MM, Pasricha PJ, Butte AJ. Computational repositioning of the anticonvulsant topiramate for inflammatory bowel disease. Sci Transl Med. 2011;3:96ra76. doi:10.1126/scitranslmed.3002648. PMID:21849664
- Falkenberg KJ, Johnstone RW. Histone deacetylases and their inhibitors in cancer, neurological diseases and immune disorders. Nature reviews Drug discovery. 2014;13:673-691. doi:10.1038/nrd4360. PMID:25131830
- Garlanda C, Dinarello CA, Mantovani A. The interleukin-1 family: back to the future. Immunity. 2013;39:1003-1018. doi:10.1016/j.immuni.2013.11.010. PMID:24332029
- Gayvert KM, Dardenne E, Cheung C, Boland MR, Lorberbaum T, Wanjala J, Chen Y, Rubin MA, Tatonetti NP, Rickman DS, et al. A Computational Drug Repositioning Approach for Targeting Oncogenic Transcription Factors. Cell Rep. 2016;15:2348-2356. doi:10.1016/j.celrep.2016.05.037. PMID:27264179
- Hansson ML, Popko-Scibor AE, Saint Just Ribeiro M, Dancy BM, Lindberg MJ, Cole PA, Wallberg AE. The transcriptional coactivator MAML1 regulates p300 autoacetylation and HAT activity. Nucleic acids research. 2009;37:2996-3006. doi:10.1093/nar/gkp163. PMID:19304754
- Jia H, Morris CD, Williams RM, Loring JF, Thomas EA. HDAC inhibition imparts beneficial transgenerational effects in Huntington's disease mice via altered DNA and histone methylation. Proc Natl Acad Sci U S A. 2015;112:E56-64. doi:10.1073/pnas.1415195112. PMID:25535382
- Jin G, Bausch D, Knightly T, Liu Z, Li Y, Liu B, Lu J, Chong W, Velmahos GC, Alam HB. Histone deacetylase inhibitors enhance endothelial cell sprouting angiogenesis in vitro. Surgery. 2011;150:429-435. doi:10.1016/j.surg.2011.07.001. PMID:21878227
- Kang S, Tsai LT, Zhou Y, Evertts A, Xu S, Griffin MJ, Issner R, Whitton HJ, Garcia BA, Epstein CB, et al. Identification of nuclear hormone receptor pathways causing insulin resistance by transcriptional and epigenomic analysis. Nat Cell Biol. 2015;17:44-56. doi:10.1038/ncb3080. PMID:25503565
- Karagiannis TC, El-Osta A. Will broad-spectrum histone deacetylase inhibitors be superseded by more specific compounds? Leukemia. 2007;21:61-65. doi:10.1038/sj.leu.2404464. PMID:17109024
- Keating ST, El-Osta A. Epigenetics and metabolism. Circ Res. 2015;116:715-736. doi:10.1161/CIRCRESAHA.116.303936. PMID:25677519
- Ko YC, Chien HF, Jiang-Shieh YF, Chang CY, Pai MH, Huang JP, Chen HM, Wu CH. Endothelial CD200 is heterogeneously distributed, regulated and involved in immune cell-endothelium interactions. J Anat. 2009;214:183-195. doi:10.1111/j.1469-7580.2008.00986.x. PMID:19166481
- Kong Y, Tannous P, Lu G, Berenji K, Rothermel BA, Olson EN, Hill JA. Suppression of class I and II histone deacetylases blunts pressure-overload cardiac hypertrophy. Circulation. 2006;113:2579-2588. doi:10.1161/CIRCULATIONAHA.106.625467. PMID:16735673
- Li H, Durbin R. Fast and accurate short read alignment with Burrows-Wheeler transform. Bioinformatics. 2009;25:1754-1760. doi:10.1093/bioinformatics/btp324. PMID:19451168
- Liao Y, Smyth GK, Shi W. featureCounts: an efficient general purpose program for assigning sequence reads to genomic features. Bioinformatics. 2014;30:923-930. doi:10.1093/bioinformatics/btt656. PMID:24227677
- Loscalzo J. Personalized cardiovascular medicine and drug development: time for a new paradigm. Circulation. 2012;125:638-645. doi:10.1161/CIRCULATIONAHA.111.089243. PMID:22294708
- Lyck R, Enzmann G. The physiological roles of ICAM-1 and ICAM-2 in neutrophil migration into tissues. Curr Opin Hematol. 2015;22:53-59. doi:10.1097/MOH.0000000000000103. PMID:25427141
- Marks PA. Histone deacetylase inhibitors: a chemical genetics approach to understanding cellular functions. Biochimica et biophysica acta. 2010;1799:717-725. doi:10.1016/j.bbagrm.2010.05.008. PMID:20594930
- Marks PA, Xu WS. Histone deacetylase inhibitors: Potential in cancer therapy. Journal of cellular biochemistry. 2009;107:600-608. doi:10.1002/jcb.22185. PMID:19459166
- Mele M, Ferreira PG, Reverter F, DeLuca DS, Monlong J, Sammeth M, Young TR, Goldmann JM, Pervouchine DD, Sullivan TJ, et al. Human genomics. The human transcriptome across tissues and individuals. Science. 2015;348:660-665. doi:10.1126/science.aaa0355. PMID:25954002
- Merico D, Isserlin R, Stueker O, Emili A, Bader GD. Enrichment map: A network-based method for gene-set enrichment visualization and interpretation. PloS one. 2010;5:e13984. doi:10.1371/journal.pone.0013984. PMID:21085593
- Mestas J, Hughes CC. Endothelial cell costimulation of T cell activation through CD58-CD2 interactions involves lipid raft aggregation. J Immunol. 2001;167:4378-4385. doi:10.4049/jimmunol.167.8.4378. PMID:11591762
- Nervi C, De Marinis E, Codacci-Pisanelli G. Epigenetic treatment of solid tumours: a review of clinical trials. Clin Epigenetics. 2015;7:127. doi:10.1186/s13148-015-0157-2. PMID:26692909
- Ooi JY, Tuano NK, Rafehi H, Gao XM, Ziemann M, Du XJ, El-Osta A. HDAC inhibition attenuates cardiac hypertrophy by acetylation and deacetylation of target genes. Epigenetics. 2015;10:418-430. doi:10.1080/15592294.2015.1024406. PMID:25941940
- Quinlan AR, Hall IM. BEDTools: a flexible suite of utilities for comparing genomic features. Bioinformatics. 2010;26:841-842. doi:10.1093/bioinformatics/btq033. PMID:20110278
- Rafehi H, Balcerczyk A, Lunke S, Kaspi A, Ziemann M, Kn H, Okabe J, Khurana I, Ooi J, Khan AW, et al. Vascular histone deacetylation by pharmacological HDAC inhibition. Genome Res. 2014;24:1271-1284. doi:10.1101/gr.168781.113. PMID:24732587
- Rafii S, Butler JM, Ding BS. Angiocrine functions of organ-specific endothelial cells. Nature. 2016;529:316-325. doi:10.1038/nature17040. PMID:26791722
- Robinson MD, McCarthy DJ, Smyth GK. EdgeR: A Bioconductor package for differential expression analysis of digital gene expression data. Bioinformatics. 2010;26:139-140. doi:10.1093/bioinformatics/btp616. PMID:19910308
- Sathishkumar C, Prabu P, Balakumar M, Lenin R, Prabhu D, Anjana RM, Mohan V, Balasubramanyam M. Augmentation of histone deacetylase 3 (HDAC3) epigenetic signature at the interface of proinflammation and insulin resistance in patients with type 2 diabetes. Clin Epigenetics. 2016;8:125. doi:10.1186/s13148-016-0293-3. PMID:27904654
- Seok SJ, Lee ES, Kim GT, Hyun M, Lee JH, Chen S, Choi R, Kim HM, Lee EY, Chung CH. Blockade of CCL2/CCR2 signalling ameliorates diabetic nephropathy in db/db mice. Nephrol Dial Transplant. 2013;28:1700-1710. doi:10.1093/ndt/gfs555. PMID:23794669
- Sharma S, Taliyan R. Histone deacetylase inhibitors: Future therapeutics for insulin resistance and type 2 diabetes. Pharmacol Res. 2016;113:320-326. doi:10.1016/j.phrs.2016.09.009. PMID:27620069
- Shungin D, Winkler TW, Croteau-Chonka DC, Ferreira T, Locke AE, Magi R, Strawbridge RJ, Pers TH, Fischer K, Justice AE, et al. New genetic loci link adipose and insulin biology to body fat distribution. Nature. 2015;518:187-196. doi:10.1038/nature14132. PMID:25673412
- Stiehl DP, Fath DM, Liang D, Jiang Y, Sang N. Histone deacetylase inhibitors synergize p300 autoacetylation that regulates its transactivation activity and complex formation. Cancer research. 2007;67:2256-2264. doi:10.1158/0008-5472.CAN-06-3985. PMID:17332356
- Subramanian A, Tamayo P, Mootha VK, Mukherjee S, Ebert BL, Gillette MA, Paulovich A, Pomeroy SL, Golub TR, Lander ES, et al. Gene set enrichment analysis: a knowledge-based approach for interpreting genome-wide expression profiles. Proc Natl Acad Sci U S A. 2005;102:15545-15550. doi:10.1073/pnas.0506580102. PMID:16199517
- Symth GK. Limma: Linear Models for Microarray Data Bioinformatics and Computational Biology Solutions Using R and Bioconductor Statistics for Biology and Health. 2005;4:397-420.
- Thompson PR, Wang D, Wang L, Fulco M, Pediconi N, Zhang D, An W, Ge Q, Roeder RG, Wong J, et al. Regulation of the p300 HAT domain via a novel activation loop. Nature structural & molecular biology. 2004;11:308-315.
- Van Lint C, Emiliani S, Verdin E. The expression of a small fraction of cellular genes is changed in response to histone hyperacetylation. Gene Expr. 1996;5:245-253. PMID:8723390
- Wood AR, Esko T, Yang J, Vedantam S, Pers TH, Gustafsson S, Chu AY, Estrada K, Luan J, Kutalik Z, et al. Defining the role of common variation in the genomic and biological architecture of adult human height. Nat Genet. 2014;46:1173-1186. doi:10.1038/ng.3097. PMID:25282103
- Xie M, Kong Y, Tan W, May H, Battiprolu PK, Pedrozo Z, Wang ZV, Morales C, Luo X, Cho G, et al. Histone deacetylase inhibition blunts ischemia/reperfusion injury by inducing cardiomyocyte autophagy. Circulation. 2014;129:1139-1151. doi:10.1161/CIRCULATIONAHA.113.002416. PMID:24396039
- Ying Y, Taori K, Kim H, Hong J, Luesch H. Total synthesis and molecular target of largazole, a histone deacetylase inhibitor. J Am Chem Soc. 2008;130:8455-8459. doi:10.1021/ja8013727. PMID:18507379
- Zhang CL, McKinsey TA, Chang S, Antos CL, Hill JA, Olson EN. Class II histone deacetylases act as signal-responsive repressors of cardiac hypertrophy. Cell. 2002;110:479-488. doi:10.1016/S0092-8674(02)00861-9. PMID:12202037
- Zhang Z, Miao L, Wang L. Inflammation amplification by Versican: the first mediator. Int J Mol Sci. 2012;13:6873-6882. doi:10.3390/ijms13066873. PMID:22837669
- Zhao LJ, Loewenstein PM, Green M. The adenovirus E1A oncoprotein N-terminal transcriptional repression domain enhances p300 autoacetylation and inhibits histone H3 Lys18 acetylation. Genes Cancer. 2015;6:30-37. PMID:25821559