ABSTRACT
Long noncoding RNAs (lncRNAs) are critical regulators of cell biology whose alteration can lead to the development of diseases such as cancer. The potential role of lncRNAs and their epigenetic regulation in response to platinum treatment are largely unknown. We analyzed four paired cisplatin-sensitive/resistant non-small cell lung cancer and ovarian cancer cell lines. The epigenetic landscape of overlapping and cis-acting lncRNAs was determined by combining human microarray data on 30,586 lncRNAs and 20,109 protein coding mRNAs with whole-genome bisulfite sequencing. Selected candidate lncRNAs were further characterized by PCR, gene-ontology analysis, and targeted bisulfite sequencing. Differential expression in response to therapy was observed more frequently in cis-acting than in overlapping lncRNAs (78% vs. 22%, fold change ≥1.5), while significantly altered methylation profiles were more commonly associated with overlapping lncRNAs (29% vs. 8%; P value <0.001). Moreover, overlapping lncRNAs contain more CpG islands (CGIs) (25% vs. 17%) and the majority of CGI-containing overlapping lncRNAs share these CGIs with their associated coding genes (84%). The differences in expression between sensitive and resistant cell lines were replicated in 87% of the selected candidates (P<0.05), while our bioinformatics approach identifying differential methylation was confirmed in all of the selected lncRNAs (100%). Five lncRNAs under epigenetic regulation appear to be involved in cisplatin resistance (AC091814.2, AC141928.1, RP11-65J3.1-002, BX641110, and AF198444). These novel findings provide new insights into epigenetic mechanisms and acquired resistance to cisplatin that highlight specific lncRNAs, some with unknown function, that may signal strategies in epigenetic therapies.
Abbreviations
lncRNAs | = | long noncoding RNAs |
WGBS | = | whole-genome bisulfite sequencing |
NSCLC | = | non-small cell lung cancer |
CGI | = | CpG island |
DM | = | differential methylation |
CDDP | = | cisplatin |
qRT-PCR | = | quantitative real-time PCR |
BS | = | bisulfite sequencing |
GO | = | gene-ontology |
Introduction
The central dogma of molecular biology maintains that the RNA molecule is merely an intermediary between DNA and proteins, which are the main protagonists of cellular functions [Citation1,Citation2]. This idea was reinforced after completion of the Human Genome Project, which revealed a vast amount of genomic space with no apparent function because this space is not occupied by protein-coding genes [Citation3].
However, data from the Encyclopedia of DNA Elements (ENCODE) project revealed that more than 70% of the genome is pervasively transcribed into noncoding RNAs. Many noncoding RNAs had been characterized prior to the ENCODE project, because they are involved in several cellular functions, such as ribosomal or transcriptional RNA [Citation4]. ENCODE, however, contributed to the identification of novel groups of regulatory noncoding RNAs, including microRNAs and long noncoding RNAs (lncRNAs) [Citation5].
lncRNAs are RNA transcripts of more than 200 nucleotides in length that lack evident open reading frames [Citation6]. The first and best-known lncRNAs identified to date are involved in chromosome dosage compensation (e.g., Xist) and the genomic imprinting and silencing of maternal or paternal genes (e.g., H19), necessary for correct embryonic development [Citation7–Citation9]. Because lncRNAs are involved in several processes important to the normal functioning of the cell, alterations in lncRNAs have been shown to contribute to the development and progression of various human diseases, including cancer. One of the most studied cancer-associated lncRNAs is metastasis-associated lung adenocarcinoma transcript 1 (MALAT1), a lncRNA involved in the mRNA splicing process [Citation10]. MALAT1 is overexpressed in non-small cell lung cancer (NSCLC) metastatic tumors and could be used as a prognostic biomarker in Stage I disease [Citation11]. Overexpression of MALAT1 plays an oncogenic role in ovarian cancer, increasing cell viability, colony formation, and migration, together with a metastatic phenotype in patients with ovarian cancer [Citation12].
Recent evidence suggests that lncRNAs are involved in chemoresistance to various anticancer therapies. One example is HOTTIP, a lncRNA regulating 5’ HOXA gene transcription, which has been associated with cell proliferation, invasion and chemoresistance in osteosarcoma, liver, and pancreatic cancers [Citation13,Citation14]. Other lncRNAs, such as UCA1 and ROR, have been associated with the resistance of cancer cells to platinum-based treatments in bladder and nasopharyngeal cancers, respectively [Citation15,Citation16].
Cisplatin (CDDP) is the most widely used chemotherapeutic for solid tumors, such as lung, ovarian, testis, and head and neck cancers, among others. Although cisplatin is a first line cancer treatment, CDDP resistance develops in a high percentage of cancer patients [Citation17–Citation20]. It has been previously shown that CDDP treatment induces de novo methylation of gene and miRNA promoters, which contributes to the development of resistance to CDDP in several tumor types [Citation21–Citation24]. Although our understanding of lncRNAs is increasing, little is known of their regulation in the development of resistance to CDDP. In the present study, we integrated a global methylation analysis with lncRNA and mRNA transcriptomics to identify the epigenetic regulation of lncRNAs that could contribute to the development of acquired CDDP resistance in NSCLC and ovarian cancer cells.
Results
Approach to identify and validate lncRNAs regulated by CDDP resistance
All data are based on eight CDDP-sensitive and CDDP-resistant NSCLC (H23S/R and H460S/R) and ovarian cancer (A2780S/R and OVCAR3S/R) cell lines previously established in our laboratory [Citation22,Citation24] (Supplementary Figure 1). We generated a global transcriptome and DNA methylome profile of lncRNAs and mRNAs to identify those that had a change in expression levels after the development of platinum resistance ()).
Figure 1. Experimental design and general overview of expression changes. (A) Pipeline of the followed steps for this study. Arrays combining lncRNAs and mRNAs probes were performed for four paired sensitive/resistant cell lines from lung and ovarian cancer. The threshold for selection was fold change ≥1.5. Inclusion of WGBS data was used to identify lncRNAs under epigenetic regulation by DNA methylation. Selection and further validation of lncRNAs was performed to confirm observed changes in expression and methylome analysis. (B and C) Venn diagram of shared lncRNAs (B) and mRNAs (C) that change in resistance in both lung cancer cell lines (top), both ovarian cancer cells (middle) or when comparing lung and ovarian cells (bottom).
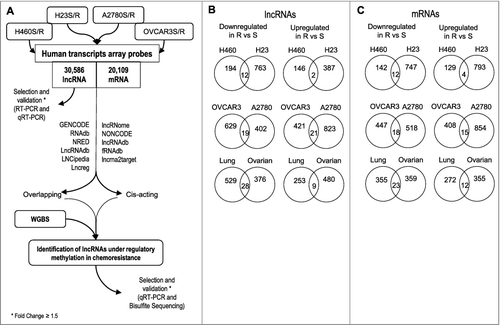
Among the 30,586 lncRNAs (19,590 intergenic lncRNAs and 10,996 overlapping) and the 20,109 mRNA transcripts, we found a percentage of expression changes of approximately 1.5% and 2.0%, respectively, for all the contrasts analyzed, with a fold change ≥1.5 (). We also compared the common lncRNAs or mRNAs with detectable changes in expression between sensitive and resistant cell lines and tissue type, and found a similar percentage change ((B and C)).
Table 1. Overall view of changes for lncRNA (left) and mRNAs (right) observed in the arrays.
We next selected a representative group of 30 lncRNA transcripts to validate the expression changes between resistant and sensitive cells observed in the array analysis by semi-quantitative PCR. Validation was successful in 11 out of 15 downregulated and 14 out of 15 upregulated lncRNAs in the cancer cell lines used for the array (Supplementary Figure 2(A and C)). We further tested the expression of six transcripts in a selection of two additional paired CDDP-resistant/sensitive cancer cell lines, a different pair A2780/A2780CP, and the pair OV2008/OVC13 (Supplementary Figure 2D). summarizes the total lncRNAs analyzed and validated, as well as their associated coding genes. We performed a gene ontology analysis with the described associated coding genes of the 25 validated lncRNAs, which are included into the Arraystar platform. Most of the lncRNA probes included in the array have at least one associated coding gene. Based on this GO analysis we selected 16 lncRNAs due to biological plausibility for their involvement in cancer, or published evidence of a role in cancer, as is the case of CRNDE [Citation25,Citation26]. We were able to confirm the expression changes by quantitative RT-PCR for 6 out of 7 downregulated ((A)) and 8 out of 9 upregulated lncRNA candidates ((B)). A summary of the selection process is detailed in Supplementary Figure 2E.
Table 2. Summary of selected lncRNAs.
Figure 2. Quantitative validation of lncRNA microarray expression changes in 15 lncRNAs based on their possible biological implication in cancer. qRT-PCR to confirm the quantitative expression changes of the downregulated (A) and upregulated (B) lncRNAs that were validated in the resistant subtypes compared with the expression of the sensitive parental cells in the lung cancer model (H23S/R and H460S/), in the ovarian cancer model (A2780S/R and OVCAR3S/R) and two additional ovarian cancer cell lines (A2780S-C/A2780CP and OV2008/OVC13). The data represent the results from at least two different experiments measured by triplicate in Relative Quantification (RQ) scale ± SD. *P < 0.05; **P < 0.01; ***P < 0.001 (Student's T-test).
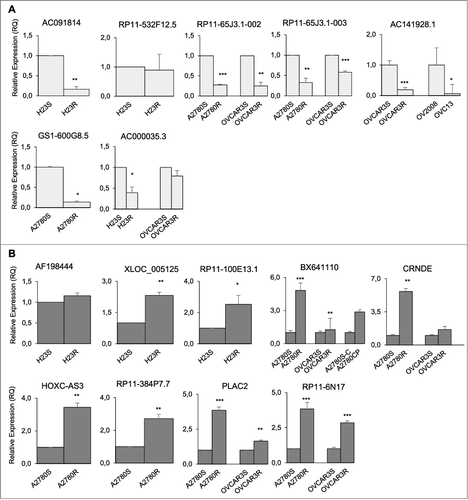
Cis-acting lncRNAs are more frequently altered in CDDP-resistant cells than overlapping lncRNAs
Further bioinformatics analyses allowed us to classify the lncRNAs that changed in resistance into two groups according to their relationship with the mRNA of a coding gene [Citation27,Citation28]. These analyses included (a) transcript and lncRNA genomic annotations in order to designate their positional relation that could help determine their functional relationship with their possible associated coding gene (ACG) and (b) a restrictive statistical analysis selecting only those lncRNAs and mRNAs with statistically significant changes in expression ((A)). Those lncRNAs sharing a genomic location with an ACG and both showing statistically significant expression changes in the array were classified as “overlapping lncRNAs,” including sense, antisense, and bidirectional lncRNAs. This group was represented by 176 unique lncRNA transcripts, which were associated with 185 unique mRNA transcripts. lncRNAs encoded in the 1-300 kb upstream region that did not overlap with another coding gene were included in the “cis-acting lncRNA” group [Citation29,Citation30]. This group was represented by 613 lncRNA transcripts interacting with 662 mRNA transcripts ((A)). Among the lncRNAs represented in the arrays with known genomic location, the observed vs. expected ratio was increased for cis-acting lncRNA (78% vs. 64%) but decreased for the overlapping lncRNAs (22% vs. 36%). When analyzing the global expression changes from both groups, we observed that the majority of the overlapping lncRNAs showed the same expression pattern as the associated mRNA ((A), left panel). For cis-acting lncRNAs, we observed both similar and opposing expression changes with their associated mRNAs in resistant compared to sensitive cell lines ((A), right panel).
Figure 3. Bioinformatic and in silico analysis of lncRNA epigenetic regulation in resistance. (A) Overall view of the lncRNAs with significant changes in expression between resistant and sensitive cell lines, according to their relationship with the associated mRNA transcript identified in the array. The left panel represents overlapping lncRNAs, whereas the right panel represents cis-acting lncRNAs. (B) Identification of possible regulatory regions under methylation and distribution according to overlapping or cis-acting groups. The graphic in the middle represents the number of lncRNAs grouped by expression pattern and according to the location of their CGI. (C) Distribution of the methylation detected by WGBS in the six groups indicated with squares and comparison between cis and overlapping lncRNAs. Chi-squared test was used for statistical analysis and P < 0.05 was considered statistically significant.
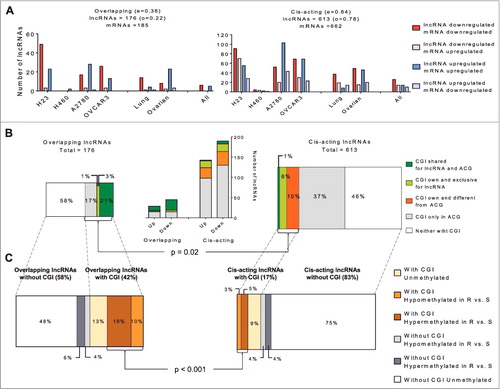
CpG islands and aberrant methylation are more frequent in overlapping than cis-acting lncRNAs in CDDP resistance
To identify the role of epigenetic regulation of lncRNAs in CDDP resistance we interrogated the whole-genome bisulfite sequencing (WGBS) data obtained from our lung and ovarian experimental models (Supporting Dataset). We searched for canonical CpG islands (CGIs) and then classified the lncRNAs according to their island position. We first observed that 44 of the 176 overlapping lncRNAs (25%) have a defined CGI for themselves or for their ACG, whereas a defined CGI was found in only 17% (105 of 613) of the cis-acting lncRNAs. It is interesting to highlight that the majority of the overlapping lncRNAs with a defined CGI share this island with their ACG (84%). Those lncRNAs are increased in the downregulated group of lncRNAs ((B)). Only 14% have an exclusive CGI and a small percentage of these lncRNAs (2%) belonged to a group with one CGI for the lncRNA and a different CGI for the ACG ((B), left bars). Conversely, among the cis-acting lncRNAs, there was a small percentage of lncRNAs sharing the CGI with the ACG (1%), with 6% showing an exclusive CGI and the majority represented by lncRNAs that have CGIs different from the CGI of their ACG (10%). This association between the presence or absence of CGIs and the lncRNA location (overlapping or cis-acting) was statistically significant (Chi-square test, P = 0.02). Thus, to identify whether CGI methylation was associated with the observed changes in lncRNA expression, we divided them into lncRNAs carrying or not a CGI and another group based on where the CGI was located (Supplementary Figure 3A). For overlapping lncRNAs, we included all the lncRNAs with a possible CGI at their regulatory region or at their ACGs in the first group (Supplementary Figure 3A, top). For cis-acting lncRNAs, we included only those lncRNAs with a possible CGI in their regulatory region (Supplementary Figure 3A, bottom). This contrast revealed that the overlapping lncRNAs are similarly represented in both groups, with and without CGIs (42% and 58%, respectively), but cis-acting lncRNAs are richer in lncRNAs without CGIs (17% vs. 83%) ((C)).
Following the identification of the possible CGIs that could be involved in the regulation of expression changes, we analyzed the differentially methylated CpG positions identified by WGBS. To avoid losing any possible methylated candidates, our bioinformatics study also included the analysis of a longer region starting at -2000 bp and ending at +500 bp from the lncRNA transcription start site (TSS) for those lncRNAs without a CGI (Supplementary Figure 3B, drawing). Among the overlapping lncRNAs with a CGI, 29% demonstrated differential methylation (DM) between resistant and sensitive cells at more than one position, compared with 8% of cis-acting lncRNAs ((C)). The difference in methylation by location of the lncRNAs (overlapping or cis-acting) was statistically significant (Chi-square test P <0.001). For overlapping lncRNAs, the methylation pattern is associated with downregulation in platinum resistance, 73% of all differentially methylated overlapping lncRNAs in comparison with the 50% observed for cis-acting lncRNAs (Supplementary Figure 3C). The results show that the -2000/+500 bp region was essentially the same for overlapping lncRNAs and their ACG; while the -2000/+500 bp region for cis-acting lncRNAs is far away (>100,000 bp) from the -2000/+500 bp region observed in their ACG, assuming that the gene DM does not interfere with the cis-lncRNA epigenetic regulation. Analyses of the −2000/+500 bp region for those lncRNAs without CGI revealed similar percentages of differential methylation for overlapping (10%) and for cis-acting (8%) lncRNAs ((C)).
Whole-genomic bisulfite sequencing validation confirms the selection criteria of our approach
Finally, we validated the methylation observed by WGBS in our cell lines. We selected eight candidates out of the 14 lncRNAs validated by qRT-PCR based on the bioinformatics analysis, the significant expression changes in both the lncRNA and the candidate ACG, and the differentially methylated positions observed by WGBS (Supplementary Figure 2E). These candidates were AC091814.2, AC141928.1, RP11-65J3.1-002, RP11-65J3.1-003, BX641110, AF198444, XLOC_005125, and RP11-100E13.1 (). Our first approach included the validation of general changes in expression after epigenetic reactivation treatment in the resistant cells, combining 5-Aza-2-deoxycytidine (5Aza-dC), a demethylating agent, and trichostatin A (TSA), a histone deacetylase inhibitor [Citation24]. RT-PCR ((A)) and qRT-PCR ((B)) confirmed our first expression results observed in the cell lines for the six candidates, AC091814.2, AC141928.1, RP11-65J3.1-002, BX641110, AF198444, and XLOC_005125.
Table 3. Main characteristics of the selected lncRNAs for methylation validation.
Figure 4. Validation of epigenetic changes in lncRNA expression. (A) RT-PCR comparing expression changes among sensitive (S), resistant (R), and resistant treated with epigenetic reactivation (RT). Each assay was performed at least three times to confirm the results. (B) qRT-PCR to confirm the quantitative expression changes in the same experimental groups, only for those samples that showed differentially methylated positions by WGBS. The data represent the results from two different experiments in triplicate in Log10 scale ± SD. *P < 0.05; **P < 0.01; ***P < 0.001 (Student's T-test).
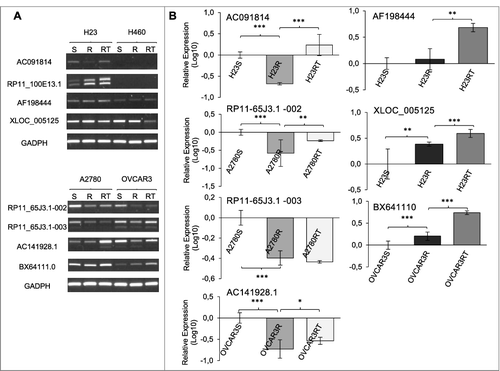
Bisulfite sequencing of the differentially methylated positions between sensitive (S) and resistant (R) cells confirmed the gain of methylation in the resistant subtypes for candidates AC091814.2, AC141928.1, and RP11-65J3.1-002, and loss of methylation for AF198444 and BX641110 ().
Figure 5. Bisulfite sequencing validation of the differentially methylated positions found by WGBS. Figure shows the genomic location of lncRNAs interrogated and the most representative positions after Sanger sequencing (left). Red asterisks indicate those positions with differential methylation between S and R cells. The right part of the panel shows the comparison with WGBS, where white squares indicate unmethylation, grey hemimethylation, and black shows methylated positions. The crosses indicate an absence of information. Red chromosomal positions are marked with an asterisk.
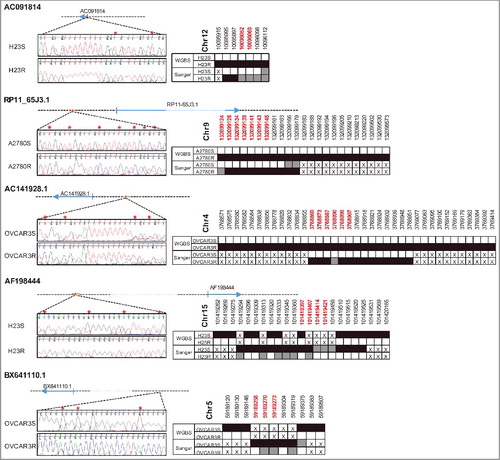
Discussion
The main objective of the current study was to test the hypothesis that differential regulation of lncRNAs underlies CDDP resistance in NSCLC and ovarian cancer, which are frequently treated with platinum-derived therapies. We sought to identify lncRNAs whose expression is different and could be under epigenetic regulation when comparing CDDP-resistant with their CDDP-sensitive parental cells.
Consistent with previous reports on NSCLC [Citation31] we observed a small percentage of expression changes among all the transcripts investigated, results that were consistent across bioinformatics contrasts. Moreover, we found a similar percentage change between ovarian and lung human cancer cell lines and limited to a relatively small number of transcripts. In fact, some of the common lncRNAs are associated with coding genes that belong to GO categories involved in cancer initiation and progression, such as the PLCE and PDE11A genes [Citation32,Citation33]. The validation of the expression changes in a selected group of lncRNAs was successful in 87% of the candidates analyzed by quantitative methodology, which is similar to the percentage found in a previous study [Citation34]. The remaining lncRNAs are novel, with unknown associated coding genes, highlighting the potential utility of the methodology employed. Although they were not included in the GO analysis, these lncRNAs cannot be completely refuted and additional research is needed to confirm the functional involvement of these candidates in cancer and chemoresistance. Some of the targets identified in the current study, AC091814.2, AC000035.3, XLOC_005125, BX641110, and RP11-384P7.7, are associated with coding genes that have been previously reported in the cancer literature; however, they have not been previously related to cancer development or cisplatin-resistance, which increases their interest for further studies.
Our bioinformatics analyses classified the lncRNAs that changed in resistance into overlapping and cis-acting lncRNAs according to their relationship with the mRNA of a coding gene [Citation27–Citation30]. One lncRNA transcript can be associated with one or more mRNA transcripts, and the number of cis-acting lncRNAs that change in the development of CDDP resistance is 3.5 times higher than the number of overlapping lncRNAs. This result is expected, because overlapping lncRNAs are encoded within the sequence of a coding gene, which represents less than 2% of the genome [Citation35,Citation36], whereas cis-acting lncRNAs can be found anywhere in a larger region (299 kb) on the same chromosome as an ACG. Trans-acting lncRNAs can exert a widespread action over the entire genome; thus, we limited our study to overlapping and cis-acting lncRNAs. The inclusion of trans-acting lncRNAs would necessitate a wider analysis in order to integrate all potential interactions between the transcriptome and the lncRNome, to discover new potential lncRNA-ACG pairs and to validate them. Therefore, this study is extensive and beyond the scope of the current work.
In terms of global expression changes, our results suggest that in the development of CDDP resistance the expression of a lncRNA overlapping with a coding gene is directly related to the expression of mRNA. Presumably, this is because their transcription is controlled by the same regulatory mechanisms. Conversely, cis-acting lncRNAs may promote or interfere with the expression of their ACG, as has been previously shown [Citation37,Citation38]. These results are in accordance with our bioinformatics methylation analysis performed on the data obtained from the WGBS, suggesting that the possible epigenetic regulation of overlapping lncRNAs can be mediated by CGIs located in their regulatory region or in one of their ACGs. By contrast, cis-acting lncRNAs could be primarily regulated by their own CGIs. Therefore, as overlapping lncRNA have a higher fraction of CGI, they are more likely to be unmethylated in normal/sensitive cells and more likely to be silenced by aberrant DNA methylation. Indeed, we found that the methylation in overlapping lncRNAs was more frequent than the cis-acting lncRNAs, reinforcing the idea that cis-acting lncRNAs could be regulated by mechanisms different from those of overlapping lncRNAs during the development of resistance to CDDP.
Although we observed that the occurrence of CpG islands in overlapping lncRNAs is higher than in cis-acting IncRNAs, this result does not reach the estimated 50%–60% of coding genes showing defined CGIs [Citation39], suggesting that lncRNAs might be less regulated by DNA methylation. The inclusion of the -2000/+500 bp region surrounding the lncRNA and the mRNAs TSS in our analysis was an inefficient approach to increasing the number of possible candidates under epigenetic regulation because the scrutiny had to be extended to 508 cis-acting lncRNAs with a one-by-one candidate approach. All together, these results suggest that the overlapping lncRNAs could be epigenetically regulated through the ACG's CGIs, thus implying that these lncRNAs would be acting on regulatory loops with their ACG due to sequence complementarity. Conversely, cis-acting lncRNAs appear to be regulated by their own CGIs, being therefore able to regulate their ACG by other means. Although various studies have analyzed the epigenetic regulation by DNA methylation of lncRNAs in cancer [Citation40,Citation41] our results are the first to identify differential epigenetic regulation for overlapping and cis-acting lncRNAs in cancer chemoresistance.
Experimental validation at the level of lncRNA expression was successful for all the selected candidates, suggesting an epigenetic regulation of these lncRNAs in resistance. Furthermore, bisulfite sequencing of the regions identified by WGBS confirmed hypermethylation in resistance for AC091814.2, AC141928.1, and RP11-65J3.1-002 lncRNAs. In addition, we identified several positions that lost methylation in the resistant subtypes of our models in the regulatory regions of AF198444 and BX641110, suggesting that CDDP also leads to epigenetic changes that decrease methylation levels. We found more differentially methylated positions by Sanger sequencing than those first identified by WGBS. The more restrictive analysis of coverage and reads for WGBS showed no information for various regions along the genome. However, it has been reported that the methylation patterns show the same behavior in proximal regions, explaining the results in our cell lines [Citation42,Citation43]. We could not validate the methylated positions of XLOC_005125, because they were separately located along the CGI and no pair of primers was available to cover the entire region. However, we were able to validate the expected methylation pattern for 100% of the selected candidates.
Our approach has allowed us to identify and characterize the molecular behavior of lncRNAs in the development of CDDP resistance in cancer. We have first shown that variation in lncRNAs and mRNAs after CDDP treatment leads to similar ratios of differences, thus identifying a small group of candidates whose expression is altered in both NSCLC and ovarian tumor types as a result of platinum treatment. This outcome is of interest for future studies focused on the potential role of lncRNAs and mRNAs in acquired resistance. Moreover, our bioinformatics analyses have identified two groups of lncRNAs according to the relationship with their associated coding gene, indicating and reinforcing that overlapping and cis-acting lncRNAs could play different regulatory roles. Further, the whole-methylome scope of our study revealed differences in methylation patterns for overlapping and cis-acting lncRNAs. We clearly observed that overlapping and cis-acting lncRNAs are differentially regulated by DNA methylation, suggesting that overlapping lncRNAs that show a positive correlation of expression with their host gene are probably regulated by the shared CGI. This regulation has been shown for miRNAs, such as miR-335 and its host gene MEST and miR-31 and its host lncRNA LOC554202 [Citation44,Citation45]; however, to the best of our knowledge, this is the first report providing this finding for lncRNAs. Furthermore, our results indicate that cis-acting lncRNAs are probably regulated by transcriptional mechanisms other than DNA methylation and thus, alternative analyses are required to study the regulation of these lncRNAs. Our research could be of great importance for future analyses involving the identification of new diagnostic and predictive cancer biomarkers based on epigenetics and lncRNA regulation.
Materials and methods
Cell lines and reagents
A total of 12 cell lines were purchased from ATCC and ECACC (Sigma-Aldrich) and cultured as recommended. To analyze the changes in the transcriptome as a result of CDDP treatment, we established the CDDP-resistant variants of H23-R, H460-R, A2780-R, and OVCAR3-R from the parental-sensitive variants H23, H460, A2780, and OVCAR3, after exposure to increasing doses of CDDP treatment over a time period of 6-18 months [Citation21,Citation22]. In order to unmask epigenetic silencing caused by cisplatin, resistant cells received a combination of the epigenetic reactivation drugs 5-Aza-2-deoxycytidine (5Aza-dC) and trichostatin A (TSA) [as previously described [Citation22,Citation46] as an epigenetic reactivating treatment to generate the resistant-treated subtypes (H23RT, H460RT, A2780RT and OVCAR3RT)]. Cell authentication is provided in Supplementary Table S1.
RNA isolation and arraystar human LncRNA microarray V3.0
Total RNA from S and R cells was extracted by the guanidine thiocyanate method using TRIzol reagent (Invitrogen) and followed by a DNAse treatment (Qiagen). RNA integrity and concentration were assessed by Nanodrop ND-1000 and 2100 Bioanalyzer (Agilent Technologies).
mRNA and lncRNA expression profiling was performed using the Arraystar Human LncRNA Microarray V3.0 (Arraystar) in two independent biological replicates per sample (GSE108139). This lncRNA microarray interrogates lncRNAs, together with mRNAs on the same chip, which are labeled along the entire length without 3’ bias, even for degraded RNA at low amounts. LncRNAs as a population are ∼10x less represented than mRNA. The overlapping lncRNAs have partial or total regions in common with their host gene [Citation47]. Thus, strand and transcript-specific detection is crucial to accurate detection of multiple transcript isoforms. The use of a specific exon or splice junction probe can specifically detect transcripts that overlap with other transcripts on the sense strand. The expression profiling was based on the manufacturer's standard protocols with minor modifications. Briefly, mRNA was purified from total RNA after removal of rRNA (mRNA-ONLY™ Eukaryotic mRNA Isolation Kit, Epicentre). Next, each sample was amplified and transcribed into fluorescent cRNA along the entire length of the transcripts without 3’ bias, using a random priming method (Arraystar Flash RNA Labeling Kit, Arraystar). The labeled cRNAs were hybridized onto the Human LncRNA Array v3.0 (8 × 60 K, Arraystar). The slides were washed and the arrays were scanned by the Agilent Scanner G2505C. Agilent Feature Extraction software (version 11.0.1.1) was used to analyze acquired array images. Quantile normalization and subsequent data processing were performed using the GeneSpring GX v12.1 software package (Agilent Technologies). After quantile normalization of the raw data, lncRNAs and mRNAs that, in at least 1 of 16 samples, had flags in Present or Marginal (“all targets value”) were chosen for further data analysis. Differentially expressed lncRNAs and mRNAs with statistical significance between the two groups were identified through fold change ≥1.5, P value ≤0.05.
Semi-quantitative RT-PCR and quantitative RT-PCR
Total RNA was isolated using TRIzol reagent following the manufacturer's protocol and was used to generate cDNA with the High Capacity cDNA Reverse Transcription Kit (Life Technologies) and PrimeScript™ RT Master (Clontech-Takara). Briefly, 500 ng of total RNA were used for RT reaction, and 2 µl of the RT product (diluted 1:5) was used for subsequent semi-quantitative PCR or qPCR reactions with either Promega Green Mix or Promega PCR Mix (Promega) and SYBR Green PCR Mix (Applied Biosystems), respectively. Real-Time PCR was performed under the following conditions: (a) One cycle of 95°C for 2 min; (b) Number of amplification cycles are between 25 to 37 at 95°C for 1 min and annealing temperatures between 56°C to 62°C for 1 min depending on each pair of primers (detailed in Supplementary ) and then 72°C for 1 min; (c) Extension of 5 min at 72°C. RT-PCR products were run on a 1.5% agarose gel, using the 100 bp Molecular size Marker (New England Biolabs) for appropriate identification of band size. qRT-PCR relative quantification was calculated according to the 2−∆∆Ct using GAPDH as endogenous control and the sensitive-parental cell line as a calibrator and represents the change of expression in RQ and Log10. Deviation bars show the maximum estimate (RQ Max) and the minimum estimate (RQ Min) expression levels, representing the standard deviation of the average expression levels of two experiments measured by triplicate. Primers were designed flanking the probe on the array, when possible, and for specific lncRNAs transcripts that significantly showed changes in the arrays; GAPDH was used as an endogenous control; all primers and specific amplification conditions are listed in Supplementary . The RNA obtained from the paired A2780/A2780CP and OV2008/OVC13 cell lines was generously provided by Dr. Cheng (Moffitt Cancer Center) and was used for further validations.
Whole-genome bisulfite sequencing
The DNA from H23S/R, H460S/R, A2780S/R, and OVCAR3S/R was isolated as described [Citation48] and sent to the National Centre for Genome Analysis [Centro Nacional de Análisis Genómico (CNAG)] for WGBS (GSE109317). Briefly, 2 µg of genomic DNA was mixed with unmethylated DNA from lambda phage in a proportion of 5 ng for each µg of genomic DNA. Libraries were prepared using the “preparation samples kit” TruSeq™ DNA v2 (Illumina, Inc.) following the manufacturer's indications with minimum changes. DNA was sonicated using Covaris E220 (Covaris, Inc.) to generate fragments of 50-500 bp. The selected size for library preparation was 150-300 bp. These fragments were purified using AMPure XP spheres (Agencourt Bioscience Corp). Following methodologies included end repair, adenylation and pairing with specific adaptors for the “paired-end” methodology from Illumina, as described previously in-depth [Citation49]. After ligation, fragments were sodium-bisulfite modified using the EpiTect Bisulfite kit (Qiagen) following the manufacturer's protocol. DNA was amplified in 7 PCR cycles using DNA polymerase PfuTurboCx Hotstart (Agilent Technologies). Quality control of the library was performed by Bioanalyzer 75000 (Agilent Technologies). The library was sequenced on HiSeq2000 (Illumina, Inc.) following the manufacturer's protocol, in paired end mode with a read length of 2 × 101 bp. Images analysis, base calling and quality scoring of the run were processed using the manufacturer's software Real Time Analysis (RTA 1.13.48). The average million read-pairs was ∼500 reads and the mean coverage was ∼30X per sample. The mapping was carried out using GEM 1.242 and the methylation calling with BScall.
Epigenetic validation: bisulfite modification and bisulfite sequencing
Isolated DNA from the H23S/R, H460S/R, A2780S/R, and OVCAR3S/R samples was bisulfite-modified and used for bisulfite sequencing as previously described [Citation50]. For bisulfite sequencing, primers were designed, when possible, to exclude binding to any CpG dinucleotides to ensure amplification of either methylated or unmethylated sequences. Primers are listed in Supplementary . PCR reactions were used for cell lines and were performed under the following conditions: (a) One cycle of 95°C for 5 min; (b) Number of amplification cycles are between 40 to 42 of 95°C for 1 min, annealing temperatures between 56°C to 62°C for 1 min depending on each pair of primers (detailed in Supplementary ) and 72°C for 1 min; (c) Extension of 8 min at 72°C. The PCR products were run on a 1.5% agarose gel, using the 100 bp Molecular size Marker (New England Biolabs) for appropriate identification of band size, then cut and cleaned by the MinElute gel extraction kit (Qiagen). Direct sequencing was performed on all the genes, rather than subcloning of a mixed population of alleles, to avoid potential cloning efficiency bias [Citation51] and artifacts [Citation52].
Bioinformatics analysis of expression and methylation
To identify differentially expressed lncRNAs and mRNAs with in silico complementarity and under potential epigenetic regulation, we interrogated the available databases with lncRNAs annotations (GENCODE [Citation53]; RNAdb [Citation54]; NRED [Citation55]; LncRNAdb [Citation56]; LNCipedia [Citation57], lncRNome [Citation58]; NONCODE [Citation59]; fRNAdb [Citation60]; lncrna2target [Citation61]) and selected those lncRNAs and mRNAs that changed significantly at three different contrasts: (1) resistant vs. sensitive for each cell line; (2) resistant vs. sensitive for each tumor type; and (3) resistant vs. sensitive for all. Based on the chromosomal relationship of the lncRNA with the mRNA, we defined as overlapping lncRNAs those within the body of the gene or oriented head to head with a protein-coding gene within 1 kb; and as cis-acting lncRNAs those at least 1 kb away from the nearest protein-coding gene but no more than 300 kb [Citation29, Citation30], including enhancer-like function LncRNAs [Citation62] – excluding overlapping lncRNAs of this group. Finally, for the identification of CGIs based on the characteristics of Takai and Jones [Citation63] in our WGBS data, we interrogated a region from 5000 bp upstream to the end of lncRNAs or mRNAs regions, and for individual CpGs from 2000 bp upstream to 500 bp downstream of TSS (Supporting Dataset, Sheets 2-5). The selection of differentially methylated (DM) CpG positions was based on previous results from our laboratory that established an experimentally validated cut-off point for the CpG site methylation level (ratio of reads with methylation out of the total number of reads covering this position). To be selected, the candidates must had to have a ratio of resistance >0.4 and sensitivity <0.23, with a minimum coverage of 10X, and at least five individuals DM CpGs. The association between qualitative variables was studied with the Chi-squared test with Yate's continuity correction and was considered statistically significant with P value <0.05.
Disclosure of potential conflicts of interest
All the authors have read the journal's authorship statement and have no conflicts of interest to declare.
Author contributions
IIC: Conception and design
OV, CRA and IIC: acquisition, analysis and interpretation of data
OV: technical methodology
CRA: bioinformatic analysis
JdC: data interpretation
FAK, TAS data interpretation, and institutional support for the development of some experimental procedures
All authors wrote, reviewed, and/or revised the manuscript.
Sup-mat-An_epigenomic_approach_to_identifying_differential_-Vera.rar
Download (5.9 MB)Acknowledgments
The authors thank ServingMed.com and Hayley Pickett for editing services. The authors also acknowledge Dr. Jin Q. Cheng for his advice and total RNA provided from the A2780/A2780CP and OV2008/OVC13 cell lines.
Data availability statement
All the data generated or analyzed during this study are included in this published article.
Additional information
Funding
References
- Crick F. Central dogma of molecular biology. Nature. 1970 Aug 08;227(5258):561–563. PubMed PMID: 4913914; eng. doi:10.1038/227561a0.
- Kung JT, Colognori D, Lee JT. Long noncoding RNAs: past, present, and future. Genetics. 2013 Mar;193(3):651–669. doi: 193/3/651 [pii] 10.1534/genetics.112.146704. PubMed PMID: 23463798; eng.
- Mattick JS, Makunin IV. Non-coding RNA. Hum Mol Genet. 2006 Apr 15; 15 Spec No 1:R17-29. doi:10.1093/hmg/ddl046. PubMed PMID: 16651366.
- Gibb EA, Brown CJ, Lam WL. The functional role of long non-coding RNA in human carcinomas. Mol Cancer. 2011 Apr 13;10:38. doi:10.1186/1476-4598-10-38. PubMed PMID: 21489289; PubMed Central PMCID: PMC3098824.
- Djebali S, Davis CA, Merkel A, et al. Landscape of transcription in human cells. Nature. 2012 Sep 06;489(7414):101–108. doi: nature11233 [pii] 10.1038/nature11233. PubMed PMID: 22955620; eng.
- Dey BK, Mueller AC, Dutta A. Long non-coding RNAs as emerging regulators of differentiation, development, and disease. Transcription. 2014;5(4):e944014. doi:10.4161/21541272.2014.944014. PubMed PMID: 25483404; eng.
- Bartolomei MS, Zemel S, Tilghman SM. Parental imprinting of the mouse H19 gene. Nature. 1991 May 09;351(6322):153–155. doi:10.1038/351153a0. PubMed PMID: 1709450; eng.
- Brannan CI, Dees EC, Ingram RS, et al. The product of the H19 gene may function as an RNA. Mol Cell Biol. 1990 Jan;10(1):28–36. PubMed PMID: 1688465; eng. doi:10.1128/MCB.10.1.28.
- Brown SD. XIST and the mapping of the X chromosome inactivation centre. Bioessays. 1991 Nov;13(11):607–612. doi:10.1002/bies.950131112. PubMed PMID: 1772416; eng.
- Tripathi V, Ellis JD, Shen Z, et al. The nuclear-retained noncoding RNA MALAT1 regulates alternative splicing by modulating SR splicing factor phosphorylation. Molecular cell. 2010 Sep 24;39(6):925–938. doi:10.1016/j.molcel.2010.08.011. PubMed PMID: 20797886; PubMed Central PMCID: PMC4158944.
- Ji P, Diederichs S, Wang W, et al. MALAT-1, a novel noncoding RNA, and thymosin beta4 predict metastasis and survival in early-stage non-small cell lung cancer. Oncogene. 2003 Sep 11;22(39):8031–8041. doi:10.1038/sj.onc.1206928 1206928 [pii]. PubMed PMID: 12970751; eng.
- Zhou Y, Xu X, Lv H, et al. The Long Noncoding RNA MALAT-1 Is Highly Expressed in Ovarian Cancer and Induces Cell Growth and Migration. PLoS One. 2016;11(5):e0155250. doi:10.1371/journal.pone.0155250 PONE-D-16-00259 [pii]. PubMed PMID: 27227769; eng.
- Li F, Cao L, Hang D, et al. Long non-coding RNA HOTTIP is up-regulated and associated with poor prognosis in patients with osteosarcoma. Int J Clin Exp Pathol. 2015;8(9):11414–20. PubMed PMID: 26617868; PubMed Central PMCID: PMC4637684.
- Quagliata L, Matter MS, Piscuoglio S, et al. Long noncoding RNA HOTTIP/HOXA13 expression is associated with disease progression and predicts outcome in hepatocellular carcinoma patients. Hepatology. 2014 Mar;59(3):911–923. doi:10.1002/hep.26740. PubMed PMID: 24114970; eng.
- Li L, Gu M, You B, et al. Long non-coding RNA ROR promotes proliferation, migration and chemoresistance of nasopharyngeal carcinoma. Cancer science. 2016 Sep;107(9):1215–1222. doi:10.1111/cas.12989. PubMed PMID: 27311700; PubMed Central PMCID: PMC5021023.
- Fan Y, Shen B, Tan M, et al. Long non-coding RNA UCA1 increases chemoresistance of bladder cancer cells by regulating Wnt signaling. FEBS J. 2014 Apr;281(7):1750–1758. doi:10.1111/febs.12737. PubMed PMID: 24495014; eng.
- Kartalou M, Essigmann JM. Mechanisms of resistance to cisplatin. Mutat Res. 2001 Jul 1;478(1-2):23–43. PubMed PMID: 11406167. doi:10.1016/S0027-5107(01)00141-5.
- Ho GY, Woodward N, Coward JI. Cisplatin versus carboplatin: comparative review of therapeutic management in solid malignancies. Crit Rev Oncol Hematol. 2016 Mar 24; doi:10.1016/j.critrevonc.2016.03.014. PubMed PMID: 27105947.
- Lee SY, Jung DK, Choi JE, et al. PD-L1 polymorphism can predict clinical outcomes of non-small cell lung cancer patients treated with first-line paclitaxel-cisplatin chemotherapy. Scientific reports. 2016 May 16;6:25952. doi:10.1038/srep25952. PubMed PMID: 27181838; PubMed Central PMCID: PMC4867646.
- French JD, Johnatty SE, Lu Y, et al. Germline polymorphisms in an enhancer of PSIP1 are associated with progression-free survival in epithelial ovarian cancer. Oncotarget. 2016 Feb 9;7(6):6353–6368. doi:10.18632/oncotarget.7047. PubMed PMID: 26840454; PubMed Central PMCID: PMC4872719.
- Cortes-Sempere M, de Miguel MP, Pernia O, et al. IGFBP-3 methylation-derived deficiency mediates the resistance to cisplatin through the activation of the IGFIR/Akt pathway in non-small cell lung cancer. Oncogene. 2012 Mar 07;32(10):1274–1283. doi: onc2012146 [pii] 10.1038/onc.2012.146. PubMed PMID: 22543588; eng.
- Ibanez de Caceres I, Cortes-Sempere M, Moratilla C, et al. IGFBP-3 hypermethylation-derived deficiency mediates cisplatin resistance in non-small-cell lung cancer. Oncogene. 2010 Mar 18;29(11):1681–1690. doi:10.1038/onc.2009.454. PubMed PMID: 20023704.
- Heyn H, Esteller M. DNA methylation profiling in the clinic: applications and challenges. Nat Rev Genet. 2012 Oct;13(10):679–692. doi:10.1038/nrg3270. PubMed PMID: 22945394.
- Vera O, Jimenez J, Pernia O, et al. DNA Methylation of miR-7 is a Mechanism Involved in Platinum Response through MAFG Overexpression in Cancer Cells. Theranostics. 2017;7(17):4118–4134. doi:10.7150/thno.20112. PubMed PMID: 29158814; PubMed Central PMCID: PMC5695001.
- Ellis BC, Molloy PL, Graham LD. CRNDE: A Long Non-Coding RNA Involved in CanceR, Neurobiology, and DEvelopment. Front Genet. 2012;3:270. doi:10.3389/fgene.2012.00270. PubMed PMID: 23226159; PubMed Central PMCID: PMCPMC3509318.
- Graham LD, Pedersen SK, Brown GS, et al. Colorectal Neoplasia Differentially Expressed (CRNDE), a Novel Gene with Elevated Expression in Colorectal Adenomas and Adenocarcinomas. Genes Cancer. 2011 Aug;2(8):829–840. doi:10.1177/1947601911431081. PubMed PMID: 22393467; PubMed Central PMCID: PMCPMC3278902.
- Ma L, Bajic VB, Zhang Z. On the classification of long non-coding RNAs. RNA Biol. 2013 Jun;10(6):925–933. doi: 24604 [pii] 10.4161/rna.24604. PubMed PMID: 23696037; eng.
- Wang KC, Chang HY. Molecular mechanisms of long noncoding RNAs. Mol Cell. 2011 Sep 16;43(6):904–914. doi: S1097-2765(11)00636-8 [pii] 10.1016/j.molcel.2011.08.018. PubMed PMID: 21925379; eng.
- Guttman M, Rinn JL. Modular regulatory principles of large non-coding RNAs. Nature. 2012 Feb 15;482(7385):339–346. doi:10.1038/nature10887. PubMed PMID: 22337053; PubMed Central PMCID: PMC4197003.
- Chen LL. Linking Long Noncoding RNA Localization and Function. Trends Biochem Sci. 2016 Sep;41(9):761–772. doi:10.1016/j.tibs.2016.07.003. PubMed PMID: 27499234.
- Yang Y, Li H, Hou S, et al. The noncoding RNA expression profile and the effect of lncRNA AK126698 on cisplatin resistance in non-small-cell lung cancer cell. PLoS One. 2013;8(5):e65309. doi:10.1371/journal.pone.0065309 PONE-D-12-23589 [pii]. PubMed PMID: 23741487; eng.
- Cui XB, Peng H, Li S, et al. Prognostic value of PLCE1 expression in upper gastrointestinal cancer: a systematic review and meta-analysis. Asian Pac J Cancer Prev. 2014;15(22):9661–9666. PubMed PMID: 25520085; eng. doi:10.7314/APJCP.2014.15.22.9661.
- Pathak A, Stewart DR, Faucz FR, et al. Rare inactivating PDE11A variants associated with testicular germ cell tumors. Endocr Relat Cancer. 2015 Dec;22(6):909–917. doi: ERC-15-0034 [pii] 10.1530/ERC-15-0034. PubMed PMID: 26459559; eng.
- Rajeevan MS, Vernon SD, Taysavang N, et al. Validation of array-based gene expression profiles by real-time (kinetic) RT-PCR. J Mol Diagn. 2001 Feb;3(1):26–31. doi: S1525-1578(10)60646-0 [pii] 10.1016/S1525-1578(10)60646-0. PubMed PMID: 11227069; eng.
- Venter JC, Adams MD, Myers EW, et al. The sequence of the human genome. Science. 2001 Feb 16;291(5507):1304–1351. doi:10.1126/science.1058040 291/5507/1304 [pii]. PubMed PMID: 11181995; eng.
- Jiang BC, Sun WX, He LN, et al. Identification of lncRNA expression profile in the spinal cord of mice following spinal nerve ligation-induced neuropathic pain. Mol Pain. 2015 Jul 17;11:43. doi:10.1186/s12990-015-0047-9 10.1186/s12990-015-0047-9 [pii]. PubMed PMID: 26184882; eng.
- Ozes AR, Miller DF, Ozes ON, et al. NF-kappaB-HOTAIR axis links DNA damage response, chemoresistance and cellular senescence in ovarian cancer. Oncogene. 2016 Oct 13;35(41):5350–5361. doi: onc201675 [pii] 10.1038/onc.2016.75. PubMed PMID: 27041570; eng.
- Wang Y, Zhang D, Wu K, et al. Long noncoding RNA MRUL promotes ABCB1 expression in multidrug-resistant gastric cancer cell sublines. Mol Cell Biol. 2014 Sep;34(17):3182–3193. doi: MCB.01580-13 [pii] 10.1128/MCB.01580-13. PubMed PMID: 24958102; eng.
- Deaton AM, Webb S, Kerr AR, et al. Cell type-specific DNA methylation at intragenic CpG islands in the immune system. Genome Res. 2011 Jul;21(7):1074–1086. doi: gr.118703.110 [pii] 10.1101/gr.118703.110. PubMed PMID: 21628449; eng.
- Yang Y, Chen L, Gu J, et al. Recurrently deregulated lncRNAs in hepatocellular carcinoma. Nat Commun. 2017 Feb 13;8:14421. doi: ncomms14421 [pii] 10.1038/ncomms14421. PubMed PMID: 28194035; eng.
- Hu H, Shu M, He L, et al. Epigenomic landscape of 5-hydroxymethylcytosine reveals its transcriptional regulation of lncRNAs in colorectal cancer. Br J Cancer. 2017 Feb 28;116(5):658–668. doi: bjc2016457 [pii] 10.1038/bjc.2016.457. PubMed PMID: 28141796; eng.
- Shoemaker R, Deng J, Wang W, et al. Allele-specific methylation is prevalent and is contributed by CpG-SNPs in the human genome. Genome Res. 2010 Jul;20(7):883–889. doi:10.1101/gr.104695.109. PubMed PMID: 20418490; PubMed Central PMCID: PMC2892089.
- Guo S, Diep D, Plongthongkum N, et al. Identification of methylation haplotype blocks aids in deconvolution of heterogeneous tissue samples and tumor tissue-of-origin mapping from plasma DNA. Nat Genet. 2017 Apr;49(4):635–642. doi:10.1038/ng.3805. PubMed PMID: 28263317; PubMed Central PMCID: PMC5374016.
- Dohi O, Yasui K, Gen Y, et al. Epigenetic silencing of miR-335 and its host gene MEST in hepatocellular carcinoma. Int J Oncol. 2013 Feb;42(2):411–418. doi:10.3892/ijo.2012.1724. PubMed PMID: 23229728; eng.
- Augoff K, McCue B, Plow EF, et al. miR-31 and its host gene lncRNA LOC554202 are regulated by promoter hypermethylation in triple-negative breast cancer. Mol Cancer. 2012 Jan 30;11:5. doi: 1476-4598-11-5 [pii] 10.1186/1476-4598-11-5. PubMed PMID: 22289355; eng.
- Vera O, Jimenez J, Pernia O, et al. DNA Methylation of miR-7 is a Mechanism Involved in Platinum Response through MAFG Overexpression in Cancer Cells. Theranostics. 2017 Sept;7(17):4118–4134. doi:10.7150/thno.20112.
- Ning Q, Li Y, Wang Z, et al. The Evolution and Expression Pattern of Human Overlapping lncRNA and Protein-coding Gene Pairs [Article]. Sci Rep. 2017 03/27/online.7:42775. doi:10.1038/srep42775 https://www.nature.com/articles/srep42775-supplementary-information.
- Pernia O, Belda-Iniesta C, Pulido V, et al. Methylation status of IGFBP-3 as a useful clinical tool for deciding on a concomitant radiotherapy. Epigenetics. 2014 Nov;9(11):1446–1453. doi:10.4161/15592294.2014.971626. PubMed PMID: 25482372; PubMed Central PMCID: PMCPMC4622698.
- Soto J, Rodriguez-Antolin C, Vallespin E, et al. The impact of next-generation sequencing on the DNA methylation-based translational cancer research. Transl Res. 2014 Mar;169:1–18, e1. doi: S1931-5244(15)00407-7 [pii] 10.1016/j.trsl.2015.11.003. PubMed PMID: 26687736; eng.
- Ibanez de Caceres I, Dulaimi E, Hoffman AM, et al. Identification of novel target genes by an epigenetic reactivation screen of renal cancer. Cancer Res. 2006 May 15;66(10):5021–5028. doi: 66/10/5021 [pii] 10.1158/0008-5472.CAN-05-3365. PubMed PMID: 16707423; eng.
- Grunau C, Clark SJ, Rosenthal A. Bisulfite genomic sequencing: systematic investigation of critical experimental parameters. Nucleic Acids Res. 2001 Jul 1;29(13):E65–E65. PubMed PMID: 11433041; PubMed Central PMCID: PMCPMC55789. doi:10.1093/nar/29.13.e65.
- Sandovici I, Leppert M, Hawk PR, et al. Familial aggregation of abnormal methylation of parental alleles at the IGF2/H19 and IGF2R differentially methylated regions. Hum Mol Genet. 2003 Jul 1;12(13):1569–1578. PubMed PMID: 12812984. doi:10.1093/hmg/ddg167.
- Harrow J, Frankish A, Gonzalez JM, et al. GENCODE: the reference human genome annotation for The ENCODE Project. Genome Res. 2012 Sep;22(9):1760–1774. doi:10.1101/gr.135350.111. PubMed PMID: 22955987; PubMed Central PMCID: PMCPMC3431492.
- Pang KC, Stephen S, Dinger ME, et al. RNAdb 2.0–an expanded database of mammalian non-coding RNAs. Nucleic Acids Res. 2007 Jan;35(Database issue):D178–D182. doi:10.1093/nar/gkl926. PubMed PMID: 17145715; PubMed Central PMCID: PMCPMC1751534.
- Dinger ME, Pang KC, Mercer TR, et al. NRED: a database of long noncoding RNA expression. Nucleic Acids Res. 2009 Jan;37(Database issue):D122–D126. doi:10.1093/nar/gkn617. PubMed PMID: 18829717; PubMed Central PMCID: PMCPMC2686506.
- Amaral PP, Clark MB, Gascoigne DK, et al. lncRNAdb: a reference database for long noncoding RNAs. Nucleic Acids Res. 2011 Jan;39(Database issue):D146–D151. doi:10.1093/nar/gkq1138. PubMed PMID: 21112873; PubMed Central PMCID: PMCPMC3013714.
- Volders PJ, Verheggen K, Menschaert G, et al. An update on LNCipedia: a database for annotated human lncRNA sequences. Nucleic Acids Res. 2015 Jan;43(Database issue):D174–D180. doi:10.1093/nar/gku1060. PubMed PMID: 25378313; PubMed Central PMCID: PMCPMC4383901.
- Bhartiya D, Pal K, Ghosh S, et al. lncRNome: a comprehensive knowledgebase of human long noncoding RNAs. Database (Oxford). 2013;2013:bat034. doi:10.1093/database/bat034. PubMed PMID: 23846593; PubMed Central PMCID: PMCPMC3708617.
- Bu D, Yu K, Sun S, et al. NONCODE v3.0: integrative annotation of long noncoding RNAs. Nucleic Acids Res. 2012 Jan;40(Database issue):D210–D215. doi:10.1093/nar/gkr1175. PubMed PMID: 22135294; PubMed Central PMCID: PMCPMC3245065.
- Kin T, Yamada K, Terai G, et al. fRNAdb: a platform for mining/annotating functional RNA candidates from non-coding RNA sequences. Nucleic Acids Res. 2007 Jan;35(Database issue):D145–D148. doi:10.1093/nar/gkl837. PubMed PMID: 17099231; PubMed Central PMCID: PMCPMC1669753.
- Jiang Q, Wang J, Wu X, et al. LncRNA2Target: a database for differentially expressed genes after lncRNA knockdown or overexpression. Nucleic Acids Res. 2015 Jan;43(Database issue):D193–D196. doi:10.1093/nar/gku1173. PubMed PMID: 25399422; PubMed Central PMCID: PMC4383967.
- Orom UA, Derrien T, Beringer M, et al. Long noncoding RNAs with enhancer-like function in human cells. Cell. 2010 Oct 01;143(1):46–58. doi:10.1016/j.cell.2010.09.001. PubMed PMID: 20887892; PubMed Central PMCID: PMCPMC4108080. doi:10.1016/j.cell.2010.09.001.
- Takai D, Jones PA. The CpG island searcher: a new WWW resource. In Silico Biol. 2003;3(3):235–240. PubMed PMID: 12954087.