ABSTRACT
DNA methylation plays an important role in the etiology and pathogenesis of head and neck squamous cell carcinoma (HNSCC). The current study aimed to identify aberrantly methylated-differentially expressed genes (DEGs) by a comprehensive bioinformatics analysis. In addition, we screened for DEGs affected by DNA methylation modification and further investigated their prognostic values for HNSCC. We included microarray data of DNA methylation (GSE25093 and GSE33202) and gene expression (GSE23036 and GSE58911) from Gene Expression Omnibus. Aberrantly methylated-DEGs were analyzed with R software. The Cancer Genome Atlas (TCGA) RNA sequencing and DNA methylation (Illumina HumanMethylation450) databases were utilized for validation. In total, 27 aberrantly methylated genes accompanied by altered expression were identified. After confirmation by The Cancer Genome Atlas (TCGA) database, 2 hypermethylated-low-expression genes (FAM135B and ZNF610) and 2 hypomethylated-high-expression genes (HOXA9 and DCC) were identified. A receiver operating characteristic (ROC) curve confirmed the diagnostic value of these four methylated genes for HNSCC. Multivariate Cox proportional hazards analysis showed that FAM135B methylation was a favorable independent prognostic biomarker for overall survival of HNSCC patients.
Introduction
Head and neck squamous cell carcinoma (HNSCC) is a collective term for malignancies originating from the oral and nasal cavities, pharynx, or larynx, which show microscopic evidence of squamous differentiation [Citation1]. HNSCC is one of the most common malignant tumors, ranking sixth in incidence worldwide [Citation2]. Approximately 50,000 new cases and 10,000 deaths from HNSCC were recorded in 2017 in the US. The incident rates increase by about 1% per year among whites, and are more than twice as high in men as in women [Citation3].
Early diagnosis of cancer is one of the most important factors contributing to less extensive and more successful treatment and better prognosis for patients[Citation4]. It has been reported that the 5-year relative survival rate for HNSCC is about 47% for blacks, while only about 30% of cases are diagnosed at an early local stage, for which the 5-year survival is about 79% [Citation3]. Unfortunately, the majority of patients are diagnosed with advanced stage HNSCC due to the asymptomatic nature of early stage disease and lack of effective screening modalities, resulting in very low 5-year survival rates [Citation5–Citation7]. Therefore, it is essential to identify useful early diagnostic and prognostic molecular biomarkers for HNSCC.
Multiple factors contribute to the incidence of HNSCC. These factors include cigarette-smoking behavior, alcohol consumption [Citation8–Citation10], and human papillomavirus (HPV) infection [Citation11–Citation13], which might induce accumulation of various genetic alterations in normal squamous cells regarded as the processes driving the initiation and progression of HNSCC [Citation14,Citation15]. In recent years, increasing evidence of epigenetic modifications as a cause of HNSCC has been documented [Citation16,Citation17]. DNA methylation, a heritable modification known to alter gene expression that is not mediated by changes in the DNA sequence [Citation18,Citation19], is the most widely investigated epigenetic modification. Aberrant methylation in the promoter region of tumor suppressor genes could induce their abnormal expression to influence some crucial biological processes, such as proliferation, apoptosis, cell cycle, DNA repair, tumor invasion, and metastasis [Citation20–Citation23]. Thus, identification of DNA methylation biomarkers has emerged as one of the most promising approaches to improve cancer diagnosis, as it presents several advantages compared to other markers [Citation24,Citation25].
In previous studies, a variety of epigenetic biomarkers has been evaluated in HNSCC for early detection and prognostic prediction; however, most of them focused on single genes. Abnormal hypermethylation of P16, MGMT, and DAPK promoters were considered as biomarkers for HNSCC detection and prognostic prediction [Citation1,Citation26,Citation27]. In recent years, the introduction of high-throughput technologies enabled the simultaneous analysis of thousands of genes in single experiments, which could serve as promising tools to screen for useful genetic and epigenetic biomarkers for diagnosis and prognosis of cancer [Citation28]. A number of gene expression profiling studies have been conducted to find various differentially expressed genes (DEGs) in HNSCC [Citation29–Citation31]. Aberrant methylation profiling has also been analyzed in HNSCC [Citation16,Citation32–Citation34]. Although numerous studies have demonstrated abnormal methylation and expression of certain genes in HNSCC, few studies have comprehensively investigated gene methylation and expression profiling in HNSCC and their potential prognostic values.
In the present study, genomic methylation microarrays (GSE25093 and GSE33202) and gene expression profiling (GSE23036 and GSE58911) datasets were integrated and analyzed using a series of bioinformatics tools. These four independent databases from Gene Expression Omnibus (GEO) were produced by different researchers from different countries. However, all of them possess limited numbers of subjects. Therefore, we identified overlapping aberrantly methylated-DEGs profiled in the four databases and then validated them by TCGA database, which has the characteristic of owing large-size samples. Using this approach, we aimed to find novel aberrantly methylated genes with small regions of heterogeneity, and then explore their prognostic value by taking advantage of TCGA database (with large sample size and detailed clinical characteristics).
Materials and methods
Microarray datasets
In the current study, we used methylation profiling (GSE25093 and GSE33202) and gene expression (GSE23036 and GSE58911) datasets from Gene Expression Omnibus (GEO, https://www.ncbi.nlm.nih.gov/geo/) of The National Center for Biotechnology Information (NCBI). Specimens from a total of 50 cases and 25 controls were enrolled in the GSE33202 program, while 91 head and neck cancer and 18 normal control specimens were in the GSE25093 program. Both of these two methylation array datasets utilized GPL8490 platform (Illumina HumanMethylation27 BeadChip). For the expression microarray datasets, the profiling of GSE58911 included 15-paired cases and the corresponding normal control specimens (platform: Affymetrix Human Gene 1.0 ST Array), while GSE23036 included 63 cases and 5 controls (platform: Affymetrix Human Genome U133A 2.0 Array).
In addition, in order to confirm the results, hypomethylated-high-expression and hypermethylated-low-expression hub genes were further validated using other datasets from TCGA database. This database includes numerous microarray data of various human cancers genomes and has generated comprehensive, multi-dimensional maps of genomic changes in human cancers.
Data processing
The microarray data of methylation and expression were analyzed by R 3.1.2 software (https://www.r-project.org/). Generally, all the microarray data after normalization were analyzed by R software. Aberrant methylation and expression between cases and controls were identified using Bioconductor minfi and limma packages [Citation35,Citation36], respectively. Benjamini-Hochberg false-discovery rate (FDR) method of adjusted P value of each CpG site and gene was calculated. An FDR-adjusted P value less than 0.05 was used as the cut-off criteria to identify differentially methylated CpG sites (DMCs) and DEGs in the microarray datasets. Similarly, FDR adjusted P value less than 0.05 and delta β value either more than 0.2 (termed hypermethylated gene) or less than −0.2 (termed hypomethylated gene) were used as cut-off values for methylation microarray datasets. Adjusted P value less than 0.05 and delta expression value either more than 1 (termed upregulated gene) or less than −1 (termed downregulated gene) were used as cut-off values for expression microarray data. All the DMCs were annotated into corresponding differentially methylated genes (DMGs) based on the platform annotation file. Subsequently, matched function was adopted to identify overlapping genes in the two methylation (GSE25093 and GSE33202) and expression (GSE23036 and GSE58911) datasets. Eventually, hypermethylated-low-expression hub genes were identified from the overlapping hypermethylated and downregulated genes, while hypomethylated-high-expression hub genes were obtained from the overlapping hypomethylated and upregulated genes.
Validation of the hub genes in TCGA database
In order to confirm the results, we also downloaded the methylation (platform: Illumina HumanMethylation450 BeadChip) and expression (IlluminaHiSeq) microarray data from TCGA database for validation. Based on the annotation file of HumanMehtylation450, there are more than 450,000 CpG sites in the human genome. These include several CpG sites located in the hub gene body that do not participate in regulating hub gene expression. Therefore, the target CpG sites used for further analysis had to meet the following two criteria: i) the target CpG site must negatively correlate with the expression of the hub gene; ii) the Pearson’s correlation among target CpG sites of each of the hub genes must be more than 0.4. Subsequently, we extracted the clinical characteristics including gender, age, cigarette smoking, alcohol consumption and clinical stage of each HNSCC patient. Survival data including overall survival (OS) and relapse-free survival (RFS) were also obtained. Finally, we constructed a logistic model using the methylation level of identified CpG sites, gender, age, clinical stage, and survival data to uncover any differences in survival duration with different methylation levels.
Statistical analysis
The methylation and expression data from GEO and TCGA were analyzed with R 3.1.2 software (https://www.r-project.org/) using Bioconductor minfi and limma packages [Citation35,Citation36]. Both methylation and expression data were normalized before further analysis. The DMCs and expressed genes between HNSCC and normal controls were identified. Benjamini-Hochberg FDR method of adjusted P value of each CpG site and gene was calculated. Both DMCs and DEGs are highlighted by red points in the volcano plots (). Pearson’s correlation analysis was applied to estimate the association of CpG sites with the expression level of the corresponding gene.
Figure 1. Volcano plots of methylation difference for methylation datasets (GSE33202, GSE250932) and expression difference for expression datasets (GSE23036, GSE58911).
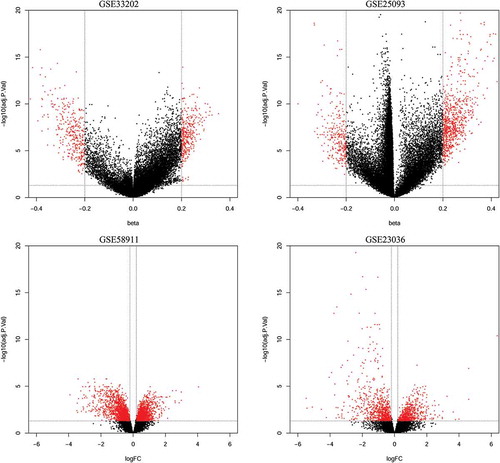
The diagnostic value of validated genes was assessed using receiver operating characteristic (ROC) curve and area under the curve (AUC). A multivariate Cox proportional hazards analysis was performed to evaluate OS and relapse-free survival (RFS) of HNSCC patients with validated gene methylation. The Pearson’s correlation, diagnostic, and survival analyses were executed with Statistical Program for Social Sciences (SPSS) software 13.0 (SPSS, Inc., Chicago, IL, USA).
Results
Identification of aberrantly methylated genes in HNSCC
Methylation data from GSE25093 and GSE33202 were downloaded from GEO. There were 50 HNSCCs and 25 controls in GSE33202, 91 HNSCC and 18 control specimens in GSE25093. The DMCs of these two methylation datasets were analyzed separately. A total of 9,894 DMCs were identified in GSE33202, among which a total of 359 were hypermethylated and 350 hypomethylated CpG sites. Similarly, a total of 20,041 DMCs were found in GSE25093, of which there were 796 hypermethylated and 275 hypomethylated CpG sites. Subsequently, after annotation of these DMCs in the two methylation datasets, there were 587 DMGs consisting of 321 hypermethylated and 266 hypomethylated genes in GSE33202, while a total of 925 DMGs consisting of 690 hypermethylated and 235 hypomethylated genes were identified in GSE25093. The differentially methylated CpG sites in the methylation microarray data are shown as volcano plots in .
Identification of degs in HNSCC
The expression data from GSE58911 and GSE23036 were utilized to analyze for differential expression of genes in HNSCC. These two-microarray datasets included 78 HNSCC specimens and 30 controls. The platforms used for these two expression datasets were different; therefore, DEGs were screened separately and annotated in their corresponding annotation files. Ultimately, a total of 1,646 and 3,384 DEGs were identified in GSE23036 and GSE58911, respectively. Among the DEGs, 176 upregulated and 445 downregulated genes were found in GSE58911, while a total of 257 upregulated and 327 downregulated genes were identified in GSE23036. The volcano plots of DEGs are shown in .
Identification of two series of hub genes
After identification of multiple DMCs and DEGs from these microarray datasets, a matching function was performed to identify overlapping genes with hypermethylated-low-expression and hypomethylated-high-expression genes in HNSCC. A total of 14 hypermethylated and downregulated genes were obtained by overlapping 164 hypermethylated and 141 low-expression genes, while a total of 13 hypomethylated and upregulated genes were found by overlapping 115 hypomethylated and 68 high-expression genes (). The hypermethylated-low-expression and hypomethylated-high-expression genes are listed in . The heat maps of all included datasets and of the two series of hub genes are shown in ), respectively.
Table 1. The hypermethylated-low expression and hypomethylated-high expression genes validated by TCGA database.
Validation of the hub genes in TCGA cohort
The hypermethylated-low-expression and hypomethylated-high-expression genes were validated in another database (TCGA) to confirm the results. TCGA database had registered 530 HNSCC patient and 74 normal control specimens from HNSCC patients. Among these samples, a total of 520 HNSCC and 44 normal control specimens were analyzed by genomic expression and methylation microarray. We first used the expression data to validate our results.
Approximately half of the genes in GEO datasets were confirmed by TCGA cohort. Six genes, DEFB118, FAM135B, CYP21A2, ZNF610, FCN2, and CEACAM7, were confirmed to be downregulated, while six other genes, NID2, HOXA9, DCC, TBX20, WT1, and GATA4, were confirmed to be upregulated in HNSCC (). The comparison among the expression of our hub genes is shown in Figure S1.
Figure 4. Six downregulated (DEFB118, FAM135B, CYP21A2, ZNF610, FCN2, and CEACAM7) and six upregulated (NID2, HOXA9, DCC, TBX20, WT1, and GATA4) genes confirmed by TCGA cohort.
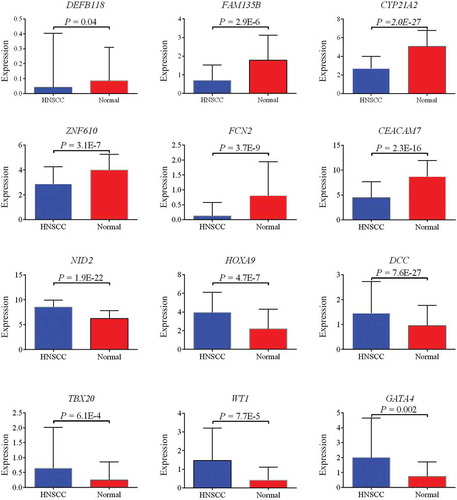
Subsequently, we downloaded all the methylation microarray data of
DEFB118, FAM135B, CYP21A2, ZNF610, FCN2, CEACAM7, NID2, HOXA9, DCC, TBX20, WT1, and GATA4 from TCGA database. All CpG sites located in the gene body region or 3'untranslated region (3'UTR) were removed. Pearson’s correlation was performed on the rest of the CpG sites to identify sites closely related to each other. These closely associated CpG sites were measured and the mean methylation used to assess the methylation difference between HNSCC and normal control. The methylation status of four genes, FAM135B, ZNF610, HOXA9, and DCC, in TCGA cohort were consistent with that in the GEO cohort. Hypermethylation of FAM135B and ZNF610 and hypomethylation of HOXA9 and DCC were validated in TCGA cohort (). A comparison among the methylation status of our hub genes is shown in Figure S2
Diagnostic and prognostic value of the four validated genes in TCGA cohort
A ROC curve was plotted to evaluate the diagnostic value of FAM135B, ZNF610, HOXA9, and DCC methylation (). The AUCs for the 4 genes were 0.792, 0.934, 0.939, and 0.939, respectively. The sensitivities and specificities with the maximum Youden indices were, respectively, 74.2% and 80.0% for FAM135B, 86.2% and 98.0% for ZNF610, 91.5% and 96.0% for HOXA9, and 88.9% and 90.0% for DCC (). In addition, we also performed multivariate Cox proportional hazards analysis to evaluate the prognostic value of FAM135B, ZNF610, HOXA9, and DCC methylation in OS and RFS of HNSCC by integration of clinical characteristics of HNSCC patients from TCGA. As shown in , our results confirmed a significant favorable OS in HNSCC patients with hypermethylation of FAM135B (hazard ratio: 0.121; 95% confidence interval (CI): 0.021–0.694). However, there was no correlation between ZNF610, HOXA9, and DCC methylation and OS or RFS of HNSCC.
Table 2. Sensitivity and specificity of methylated genes for diagnosis of HNSCC.
Table 3. Prognostic value of methylated genes for HNSCC.
Discussion
Elucidating the underlying mechanisms of the initiation and development of HNSCC could greatly benefit diagnostic and prognostic evaluation. HNSCC is a collective term used to describe carcinomas that originate from the oral cavity, pharynx, and larynx and are characterized by various activated pro-oncogenes and inactivated tumor suppressor genes [Citation37–Citation39]. Epigenetic changes alter the expression of critical genes responsible for the initiation and development of cancers [Citation40–Citation42]. Multiple types of epigenetic modifications have been identified, including methylation, acetylation, phosphorylation, ubiquitination, and sumolyation [Citation43,Citation44]. However, the best well known epigenetic change is DNA methylation, which modifies the expression of key genes involved in various biological processes. Previous studies have reported hypermethylation of genes including CDKN2A [Citation45], DAPK [Citation46], RASSF1A [Citation22], RARβ 2[Citation47], MGMT [Citation22], and E-cadherin [Citation48] in HNSCC, and have proposed that these aberrantly methylated genes are associated with cell proliferation, cell-cell adhesion [Citation48], and apoptosis[Citation49]. Hypermethylation-associated silencing in multiple biological processes serves an important role throughout the progression of HNSCC [Citation50]. Worsham et al. have delineated an epigenetic continuum in HNSCC by identification of a series of abnormally methylated genes from benign papillomas to HNSCC and concluded that DNA methylation plays an important role in the development continuum from benign neoplasm to malignancy [Citation51]. In addition, the methylation profile of gene promoters was different for each type of tumor [Citation46], suggesting that the detection of aberrant gene promoter methylation could serve as a potential molecular biomarker for tumors. The evaluation of methylation profiles as a tool for the diagnosis or prognosis of tumors has been described for many cancers, such as colorectal cancer [Citation52], nasopharyngeal carcinoma [Citation53], laryngeal squamous cell carcinoma [Citation54], and HNSCC [Citation55].
In the present study, we investigated genome-wide DNA methylation patterns of 141 HNSCC patients and 43 normal controls by microarray and identified 29,935 probes showing significant differential DNA methylation in cancer tissues. By integrating DNA methylation and RNA sequencing microarray data, 27 aberrantly methylated genes accompanied by altered expression were identified. To verify the results of GEO microarray dataset, an independent validation of these aberrantly methylated-DEGs was performed using TCGA database. In total, two hypermethylated-low-expression (FAM135B and ZNF610) and two hypomethylated-high-expression (HOXA9 and DCC) genes were validated. The diagnostic value of these genes was evaluated, and the sensitivity and specificity of the four methylated genes for diagnosis of HNSCC were >70% and >80%, respectively, indicating that these aberrantly methylated genes could serve as a useful tool for early diagnosis of HNSCC. Furthermore, the four methylated genes were evaluated for their potential patient prognosis of HNSCC; only FAM135B hypermethylation was associated with favorable patient survival.
Hypermethylation of ZNF610 with impaired transcriptional capacity was reported in previous studies [Citation56,Citation57] and also identified in our study. As a member of the Krüppel C2H2-type zinc finger protein family, ZNF610 encodes zinc figure protein 610 that consists of 9 C2H2-type zinc fingers and 1 Krüppel-associated box (KRAB) domain. The KRAB domain could behave as a transcriptional repressor by recruiting corepressor proteins [Citation58], and has been implicated in the maintenance of the nucleolus, cell proliferation, cell differentiation, and apoptosis [Citation59]. In gastric carcinomas, approximately 70% of patients were shown to harbor hypermethylated and decreased expression of ZNF610 [Citation60]. However, hypermethylated FAM135B has been less investigated and its association with cancer has been less reported. Only one study has implicated FAM135B methylation with cancer, and this study elaborated on its ability to promote esophageal squamous cell carcinoma (ESCC). This study further concluded that FAM135B mutation was associated with poor survival of ESCC patients [Citation61]. Deletion of FAM135B could attenuate cellular malignant phenotype, such as cell growth, colony formation, migration, and invasion [Citation61]. From this perspective, downregulated expression of FAM135B caused by promoter methylation might be a favorable prognostic factor for cancer patients. In the current study, our results show that hypermethylation of FAM135B could be considered a favorable prognostic factor of HNSCC.
In summary, we have identified four aberrantly methylated genes (FAM135B, ZNF610, HOXA9, and DDC) that could serve as useful biomarkers for diagnosis and prognosis of HNSCC. Additionally, we have uncovered hypermethylation of FAM135B to be associated with favorable survival of HNSCC patients.
Supplemental Material
Download JPEG Image (210.8 KB)Supplemental Material
Download JPEG Image (235 KB)Disclosure statement
No potential conflict of interest was reported by the authors.
Supplementary material
Supplemental data for this article can be accessed here.
Additional information
Funding
References
- Pai SI, Westra WH. Molecular pathology of head and neck cancer: implications for diagnosis, prognosis, and treatment. Annu Rev Pathol. 2009;4:49–70. PubMed PMID: 18729723; PubMed Central PMCID: PMCPMC3703474.
- Vokes EE, Weichselbaum RR, Lippman SM, et al. Head and neck cancer. N Engl J Med. 1993 Jan 21;328(3):184–194. PubMed PMID: 8417385.
- Society AC. Cancer facts and figures, 2018. [cited 2018 Jan 04]. Available from: https://www.cancer.org/content/dam/cancer-org/research/cancer-facts-and-statistics/annual-cancer-facts-and-figures/2018/cancer-facts-and-figures-2018.pdf
- Arantes L, De Carvalho AC, Melendez ME, et al. Serum, plasma and saliva biomarkers for head and neck cancer. Expert Rev Mol Diagn. 2018 Jan;18(1):85–112. PubMed PMID: 29134827.
- Carvalho AL, Nishimoto IN, Califano JA, et al. Trends in incidence and prognosis for head and neck cancer in the United States: a site-specific analysis of the SEER database. Int J Cancer. 2005 May 1;114(5):806–816. PubMed PMID: 15609302.
- Cmelak AJ, Arneson K, Chau NG, et al. Locally advanced head and neck cancer. Am Soc Clin Oncol Educ Book. 2013;237–244. PubMed PMID: 23714512. doi:10.1200/EdBook_AM.2013.33.237
- Matar N, Haddad A. New trends in the management of head and neck cancers. J Med Liban. 2011 Oct-Dec;59(4):220–226. PubMed PMID: 22746011.
- Argiris A, Eng C. Epidemiology, staging, and screening of head and neck cancer. Cancer Treat Res. 2003;114: 15–60. PubMed PMID: 12619537.
- Blot WJ, McLaughlin JK, Winn DM, et al. Smoking and drinking in relation to oral and pharyngeal cancer. Cancer Res. 1988 Jun 1;48(11):3282–3287. PubMed PMID: 3365707.
- Vineis P, Alavanja M, Buffler P, et al. Tobacco and cancer: recent epidemiological evidence. J Natl Cancer Inst. 2004 Jan 21;96(2):99–106. PubMed PMID: 14734699.
- D’Souza G, Kreimer AR, Viscidi R, et al. Case-control study of human papillomavirus and oropharyngeal cancer. N Engl J Med. 2007 May 10;356(19):1944–1956. PubMed PMID: 17494927.
- Kreimer AR, Clifford GM, Boyle P, et al. Human papillomavirus types in head and neck squamous cell carcinomas worldwide: a systematic review. Cancer Epidemiol Biomarkers Prev. 2005 Feb;14(2):467–475. PubMed PMID: 15734974.
- O’Rorke MA, Ellison MV, Murray LJ, et al. Human papillomavirus related head and neck cancer survival: a systematic review and meta-analysis. Oral Oncol. 2012 Dec;48(12):1191–1201. PubMed PMID: 22841677.
- Munger K, Howley PM. Human papillomavirus immortalization and transformation functions. Virus Res. 2002 Nov;89(2):213–228. PubMed PMID: 12445661.
- Slebos RJ, Yi Y, Ely K, et al. Gene expression differences associated with human papillomavirus status in head and neck squamous cell carcinoma. Clin Cancer Res. 2006 Feb 1;12(3 Pt 1):701–709. PubMed PMID: 16467079.
- Marsit CJ, Christensen BC, Houseman EA, et al. Epigenetic profiling reveals etiologically distinct patterns of DNA methylation in head and neck squamous cell carcinoma. Carcinogenesis. 2009 Mar;30(3):416–422. PubMed PMID: 19126652; PubMed Central PMCID: PMCPMC2650795.
- Worsham MJ, Chen KM, Meduri V, et al. Epigenetic events of disease progression in head and neck squamous cell carcinoma. Arch Otolaryngol Head Neck Surg. 2006 Jun;132(6):668–677. PubMed PMID: 16785414.
- Bird AP. CpG-rich islands and the function of DNA methylation. Nature. 1986 May 15–21;321(6067):209–213. PubMed PMID: 2423876.
- Tate PH, Bird AP. Effects of DNA methylation on DNA-binding proteins and gene expression. Curr Opin Genet Dev. 1993 Apr;3(2):226–231. PubMed PMID: 8504247.
- Dong SM, Sun DI, Benoit NE, et al. Epigenetic inactivation of RASSF1A in head and neck cancer. Clin Cancer Res. 2003 Sep 1;9(10 Pt 1):3635–3640. PubMed PMID: 14506151.
- Liu Z, Zhao J, Chen XF, et al. CpG island methylator phenotype involving tumor suppressor genes located on chromosome 3p in non-small cell lung cancer. Lung Cancer. 2008 Oct;62(1):15–22. PubMed PMID: 18358560.
- Rosas SL, Koch W, da Costa Carvalho MG, et al. Promoter hypermethylation patterns of p16, O6-methylguanine-DNA-methyltransferase, and death-associated protein kinase in tumors and saliva of head and neck cancer patients. Cancer Res. 2001 Feb 1;61(3):939–942. PubMed PMID: 11221887.
- Takahashi T, Shigematsu H, Shivapurkar N, et al. Aberrant promoter methylation of multiple genes during multistep pathogenesis of colorectal cancers. Int J Cancer. 2006 Feb 15;118(4):924–931. PubMed PMID: 16108009.
- Delpu Y, Cordelier P, Cho WC, et al. DNA methylation and cancer diagnosis. Int J Mol Sci. 2013 Jul 18;14(7):15029–15058. PubMed PMID: 23873296; PubMed Central PMCID: PMCPMC3742286.
- Zhang XM, Guo MZ. The value of epigenetic markers in esophageal cancer. Front Med China. 2010 Dec;4(4):378–384. . PubMed PMID: 21107750.
- Sanchez-Cespedes M, Esteller M, Wu L, et al. Gene promoter hypermethylation in tumors and serum of head and neck cancer patients. Cancer Res. 2000 Feb 15;60(4):892–895. PubMed PMID: 10706101.
- Steinmann K, Sandner A, Schagdarsurengin U, et al. Frequent promoter hypermethylation of tumor-related genes in head and neck squamous cell carcinoma. Oncol Rep. 2009 Dec;22(6):1519–1526. PubMed PMID: 19885608.
- Kulasingam V, Diamandis EP. Strategies for discovering novel cancer biomarkers through utilization of emerging technologies. Nat Clin Pract Oncol. 2008 Oct;5(10):588–599. PubMed PMID: 18695711.
- Chung CH, Parker JS, Karaca G, et al. Molecular classification of head and neck squamous cell carcinomas using patterns of gene expression. Cancer Cell. 2004 5;May(5):489–500. PubMed PMID: 15144956.
- Demokan S, Chuang AY, Chang X, et al. Identification of guanine nucleotide-binding protein gamma-7 as an epigenetically silenced gene in head and neck cancer by gene expression profiling. Int J Oncol. 2013 Apr;42(4):1427–1436. PubMed PMID: 23403885; PubMed Central PMCID: PMCPMC3981008.
- Roepman P, Wessels LF, Kettelarij N, et al. An expression profile for diagnosis of lymph node metastases from primary head and neck squamous cell carcinomas. Nat Genet. 2005 Feb;37(2):182–186. PubMed PMID: 15640797.
- Calmon MF, Colombo J, Carvalho F, et al. Methylation profile of genes CDKN2A (p14 and p16), DAPK1, CDH1, and ADAM23 in head and neck cancer. Cancer Genet Cytogenet. 2007 Feb;173(1):31–37. PubMed PMID: 17284367.
- Viswanathan M, Tsuchida N, Shanmugam G. Promoter hypermethylation profile of tumor-associated genes p16, p15, hMLH1, MGMT and E-cadherin in oral squamous cell carcinoma. Int J Cancer. 2003 May 20;105(1):41–46. . PubMed PMID: 12672028.
- Yalniz Z, Demokan S, Suoglu Y, et al. Simultaneous methylation profiling of tumor suppressor genes in head and neck cancer. DNA Cell Biol. 2011 Jan;30(1):17–24. PubMed PMID: 20860434.
- Aryee MJ, Jaffe AE, Corrada-Bravo H, et al. Minfi: a flexible and comprehensive Bioconductor package for the analysis of Infinium DNA methylation microarrays. Bioinformatics. 2014 May 15;30(10):1363–1369. PubMed PMID: 24478339; PubMed Central PMCID: PMCPMC4016708.
- Ritchie ME, Phipson B, Wu D, et al. limma powers differential expression analyses for RNA-sequencing and microarray studies. Nucleic Acids Res. 2015 Apr 20;43(7):e47. PubMed PMID: 25605792; PubMed Central PMCID: PMCPMC4402510.
- Califano J, van der Riet P, Westra W, et al. Genetic progression model for head and neck cancer: implications for field cancerization. Cancer Res. 1996 Jun 1;56(11):2488–2492. PubMed PMID: 8653682.
- Field JK. Oncogenes and tumour-suppressor genes in squamous cell carcinoma of the head and neck. Eur J Cancer B Oral Oncol. 1992 Jul;28B(1):67–76 PubMed PMID: 1330149.
- Field JK. The role of oncogenes and tumour-suppressor genes in the aetiology of oral, head and neck squamous cell carcinoma. J R Soc Med. 1995 Jan;88(1):35P–39P. PubMed PMID: 7884768; PubMed Central PMCID: PMCPMC1295074.
- Feinberg AP, Ohlsson R, Henikoff S. The epigenetic progenitor origin of human cancer. Nat Rev Genet. 2006 Jan;7(1):21–33. PubMed PMID: 16369569.
- Jones PA, Baylin SB. The fundamental role of epigenetic events in cancer. Nat Rev Genet. 2002 Jun;3(6):415–428. PubMed PMID: 12042769.
- Nephew KP, Huang TH. Epigenetic gene silencing in cancer initiation and progression. Cancer Lett. 2003 Feb 20;190(2):125–133. PubMed PMID: 12565166.
- Nakao M. Epigenetics: interaction of DNA methylation and chromatin. Gene. 2001 Oct 31;278(1–2):25–31. PubMed PMID: 11707319.
- Strahl BD, Allis CD. The language of covalent histone modifications. Nature. 2000 Jan 6;403(6765):41–45. PubMed PMID: 10638745.
- Miracca EC, Kowalski LP, Nagai MA. High prevalence of p16 genetic alterations in head and neck tumours. Br J Cancer. 1999 Oct;81(4):677–683. PubMed PMID: 10574255; PubMed Central PMCID: PMCPMC2362902.
- Esteller M, Corn PG, Baylin SB, et al. A gene hypermethylation profile of human cancer. Cancer Res. 2001 Apr 15;61(8):3225–3229. PubMed PMID: 11309270.
- Olasz J, Juhasz A, Remenar E, et al. RAR beta2 suppression in head and neck squamous cell carcinoma correlates with site, histology and age. Oncol Rep. 2007 Jul;18(1):105–112. PubMed PMID: 17549354.
- Hasegawa M, Nelson HH, Peters E, et al. Patterns of gene promoter methylation in squamous cell cancer of the head and neck. Oncogene. 2002 Jun 20;21(27):4231–4236. PubMed PMID: 12082610.
- Weber A, Hengge UR, Bardenheuer W, et al. SOCS-3 is frequently methylated in head and neck squamous cell carcinoma and its precursor lesions and causes growth inhibition. Oncogene. 2005 Oct 6;24(44):6699–6708. PubMed PMID: 16007169.
- Tripathi Bhar A, Banerjee S, Chunder N, et al. Differential alterations of the genes in the CDKN2A-CCND1-CDK4-RB1 pathway are associated with the development of head and neck squamous cell carcinoma in Indian patients. J Cancer Res Clin Oncol. 2003 Nov;129(11):642–650. PubMed PMID: 14586645.
- Worsham MJ, Stephen JK, Chen KM, et al. Delineating an epigenetic continuum in head and neck cancer. Cancer Lett. 2014 Jan 28;342(2):178–184. PubMed PMID: 22388100; PubMed Central PMCID: PMCPMC3371397.
- Li J, Zhou C, Ni S, et al. Methylated claudin-11 associated with metastasis and poor survival of colorectal cancer. Oncotarget. 2017 Nov 10;8(56):96249–96262. PubMed PMID: 29221203; PubMed Central PMCID: PMCPMC5707097.
- Li J, Zhou C, Wang G, et al. Promoter hypermethylation of SLIT2 is a risk factor and potential diagnostic biomarker for nasopharyngeal carcinoma. Gene. 2017 Nov 8 PubMed PMID: 29107007. DOI:10.1016/j.gene.2017.10.059.
- Shen Z, Zhou C, Li J, et al. SHISA3 Promoter methylation is a potential diagnostic and prognostic biomarker for laryngeal squamous cell carcinoma. Biomed Res Int. 2017;2017:9058749. PubMed PMID: 28299336; PubMed Central PMCID: PMCPMC5337399. publication of this article.
- Sailer V, Gevensleben H, Dietrich J, et al. Clinical performance validation of PITX2 DNA methylation as prognostic biomarker in patients with head and neck squamous cell carcinoma. PLoS One. 2017;12(6):e0179412. PubMed PMID: 28617833; PubMed Central PMCID: PMCPMC5472307.
- Schneider BG, Mera R, Piazuelo MB, et al. DNA Methylation predicts progression of human gastric lesions. Cancer Epidemiol Biomarkers Prev. 2015 Oct;24(10):1607–1613. PubMed PMID: 26269563; PubMed Central PMCID: PMCPMC4592454.
- Schneider BG, Piazuelo MB, Sicinschi LA, et al. Virulence of infecting Helicobacter pylori strains and intensity of mononuclear cell infiltration are associated with levels of DNA hypermethylation in gastric mucosae. Epigenetics. 2013 Nov;8(11):1153–1161. PubMed PMID: 24128875; PubMed Central PMCID: PMCPMC3927747.
- Urrutia R. KRAB-containing zinc-finger repressor proteins. Genome Biol. 2003;410:231. PubMed PMID: 14519192; PubMed Central PMCID: PMCPMC328446.
- Tian C, Xing G, Xie P, et al. KRAB-type zinc-finger protein Apak specifically regulates p53-dependent apoptosis. Nat Cell Biol. 2009 May;11(5):580–591. PubMed PMID: 19377469.
- Cancer Genome Atlas Research N. Comprehensive molecular characterization of gastric adenocarcinoma. Nature. 2014 Sep 11;513(7517):202–209. PubMed PMID: 25079317; PubMed Central PMCID: PMCPMC4170219.
- Song Y, Li L, Ou Y, et al. Identification of genomic alterations in oesophageal squamous cell cancer. Nature. 2014 May 1;509(7498):91–95. PubMed PMID: 24670651.