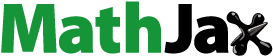
ABSTRACT
DNA methylation is an epigenetic regulator of gene transcription, which has been found to be both metastable and variable within human cohort studies. Currently, few studies have been done to identify metastable DNA methylation biomarkers associated with longitudinal lung function decline in humans. The identification of such biomarkers is important for screening vulnerable populations. We hypothesized that quantifiable blood-based DNA methylation alterations would serve as metastable biomarkers of lung function decline and aging, which may help to discover new pathways and/or mechanisms related to pulmonary pathogenesis. Using linear mixed models, we performed an Epigenome Wide Association Study (EWAS) between DNA methylation at CpG dinucleotides and longitudinal lung function (FVC, FEV1, FEF25–75%) decline and aging with initial discovery in the Normative Aging Study, and replication in the Cooperative Health Research in the Region of Augsburg cohort. We identified two metastable epigenetic loci associated with either poor lung function and aging, cg05575921 (AHRR gene), or lung function independently of aging, cg06126421 (IER3 gene). These loci may inform basic mechanisms associated with pulmonary function, pathogenesis, and aging. Human epigenomic variation, may help explain features of lung function decline and related pathophysiology not attributable to DNA sequence alone, such as accelerated pulmonary decline in smokers, former smokers, and perhaps non-smokers. Our EWAS across two cohorts, therefore, will likely have implications for the human population, not just the elderly.
Introduction
A number of genome-wide association studies have paved the way for identifying human genetic variants linked to lung function [Citation1–Citation3], yet nucleotide-level polymorphisms can only explain a limited amount of disease risk, suggesting that other, gene-regulatory mechanisms may also be at play [Citation4]. Lung function decline fits well into the epigenetic hypothesis of disease [Citation5], which posits that epigenetic variation across individuals may be an important intermediary between genetic variation and other factors that may ‘program’ the DNA [Citation6,Citation7]. Epigenetic mechanisms like DNA methylation may provide further explanation for poor lung function and accelerated decline and aging-related associations that are not explained entirely by the DNA sequence alone. This could be things such as the variable susceptibility to develop lung disease in smokers and the sustained, elevated risk for lung function decline years after smoking cessation [Citation2,Citation8–Citation12]. Epigenetics studies can shed novel insights into the pathogenesis and susceptibility to lung function and pulmonary disease.
DNA methylation (DNAm) commonly occurs at cytosine residues arranged within ‘CpG’ (cytosine-phosphate-guanine dinucleotide) sites. When DNAm does not change over time we denote the methylation at that CpG site as metastable. DNAm is the best-studied examples of DNA-level epigenetic mechanisms across species and research is ongoing to better map and understand how human DNAm shapes health, disease and disparities across persons, with transformative uses in the clinical setting [Citation7,Citation13–Citation15]. DNAm has been found to be associated with biological aging as well as a potential driver of biological aging [Citation16]. Therefore, the exploration of non-invasive, blood-based DNAm diagnostics to screen for and help identify persons at risk for poor lung function and/or accelerated decline is a public health priority, as decreased pulmonary function is highly associated with mortality [Citation17–Citation20].
In humans, lung function increases after birth until about age 20, which is when it peaks and begins to plateau up to about age 30, defining the starting point for the followed age-related decline, which typically is accelerated in smokers [Citation2]. In this study, we focused on three spirometric indices that provide slightly different information on lung function. Forced vital capacity (FVC, liters), is determined by the size of the gas exchanging region, the alveolar region of the lung. FVC decreases in cases of fibrosis as well as when the maximal respirable volume decreases for example in chronic obstructive pulmonary disease (COPD) particularly due to emphysema. Forced expiratory flow between 25–75% of FVC (FEF25–75%, liters per minute), measures the conducting airway function, and is determined by the size of the airways. A low flow rate of FEF25–75% reflects airflow limitation usually corresponding to airway narrowing or obstruction of the airways. Finally, forced expiratory volume in 1 second (FEV1, liters), which is affected by airway narrowing, is the volume that is expired in 1 second. It is often reduced in COPD and asthma but can also be reduced when the lung size is affected due to a decrease in maximal respirable volume, such as in fibrosis and emphysema.
Classically, smoking is a predominant environmental risk factor for lung function decline and relevant pathophysiological conditions, such as COPD, which is a leading cause of death in the USA [Citation21]. While there are DNA methylation biomarkers that are associated with smoking exposures [Citation22,Citation23] and correlated with adverse lung function [Citation24,Citation25], we sought to account for smoking in a way that would allow us to consider DNAm that is variable and/or stable over time in individuals, and could inform pulmonary function independent of smoking. This allows for using biomarkers that are informative more broadly across individuals, and, hopefully, less susceptible to influence by other variables that may confound their utility within clinical and research settings.
We used linear mixed models to perform an epigenome-wide association study of the association between DNAm at CpG sites and longitudinal lung function decline in the Normative Aging Study (NAS), a cohort of elderly men residing in the greater-Boston metropolitan area. The initial discovery phase was in the NAS cohort followed by validation in the Cooperative Health Research in the Region of Augsburg (KORA) cohort. Lung function decline in this paper is defined as a decrease in any of the three studied pulmonary function parameters (FVC, FEF25–75, or FEV1) between visits. We assess the association of DNAm effect with this decline by modeling its interaction effect with time of observation. Finally, given that lung function decline is often indicative of aging and/or risk of mortality in vulnerable individuals [Citation26–Citation29], we assessed the correlation between methylation of particular CpG sites of interest, pulmonary function, and an age-difference variable constructed from ‘DNA methylation age’ (DNAm age) [Citation30].
Results
In NAS, there were 633 men who contributed 2245 observations (average 3.5 observations/participant) over about a decade. The mean age was 72 years old at time of blood draw, with 28 current smokers, 426 former smokers, and 179 never smokers. KORA consisted of 868 people with age between 41–63 and 408 men (47%). displays descriptive statistics for the time varying variables in our study. It displays up to four visits prior to blood draw in NAS and up to one visit after blood draw in KORA as participants had varying amount of follow up time. displays the variables that were held constant in the analysis. These are variables such as cell type proportion, age at first visit, and height at first non-missing observation. We report in body mass index at blood draw, though we do not adjust for it in the model. In NAS, there were 2 underweight (.3%), 136 normal weight (21.5%), 340 overweight (53.7%), and 155 obese (24.5%) people in NAS. In KORA, there was 1 underweight (.1%), 278 with normal weight (32%), 344 overweight (39.6%), and 245 obese (28.2%) people. In NAS, the mean age at first visit was 63 in NAS, and 54 in KORA. The lung function measures saw a decline across the four visits (). The varying smoking status indicate varying levels of data being available. In NAS for example, of the 28 current smokers at time of blood draw, only 22 had up to three observations prior to time of blood draw (). In KORA, of the 180 current smokers, 120 had a secondary lung function observation.
Table 1. Time-varying characteristics obtained from the NAS and KORA participants.
Table 2. Non-time-varying and other characteristics obtained from participants in the NAS and KORA cohorts: blood-cell proportions, baseline age, and height.
We analyzed DNAm’s association with pulmonary function in NAS assuming that DNAm was metastable (i.e. does not change with time). DNAm was collected at the last time point and then treated as non-time varying when regressing on lung function measures collected prior to DNAm. We analyzed FEV1, FVC, and FEF25–75% separately in 477,927 probes. We tested for two different associations: association with lung function decline (interaction between DNAm and follow up time from first spirometric measure, βLD) and its cross sectional association (association between DNAm and lung function when follow up time is 0, βCS). The statistical model is provided in the methods section under biostatistical analysis. Variables included in the model were: season, follow up time, day of the week, plate, chip, mean centered weight, mean centered weight squared, the natural log of height, baseline age, vitamin C intake not from supplements, maximum years of education and, taking any of the following drugs-corticosteroids (inhaled (ICS) or systemic), beta-2-adrenergic agonists (typical LABA), sympathomimetic alpha AHFS, or long acting muscarinic antagonist (LAMA). We also adjusted for cell type composition [Citation31]. Significance was assessed either via a Holm adjusted p-value less than 0.05 or an FDR adjusted p-value less than 0.10 [Citation32,Citation33].
NAS lung function decline results (βLD)
We first assessed the interaction between follow up time and DNA methylation. As lung function decreases after the age of 30, the association between follow up time and lung function will be negative. The interaction between DNAm and follow up time then should denote the contribution of DNAm to lung function decline. When adjusting for smoking, nine unique CpG probes were significantly associated with the rate of decline in pulmonary function tests (PFTs) at the Holm significance level of 0.05 [Citation33],(-Group 1). Not adjusting for smoking, ten unique probes were associated with rate of decline (-Group 1). There were seven probes that were significant regardless of adjustment for smoking, three probes significant when not adjusting for smoking, and two probes significant only when adjusting for smoking (-Group 1). The parameter estimates between the smoking adjusted and unadjusted models remained the same for the seven probes (-Group 1). Quantile-quantile plots (QQplots) of the analysis showed some inflation for this analysis in the FEV1 and FVC analyses (,subplot A1, B1, C1, genomic inflation factor in caption of -Group 1). There were 1490 significant associations (mapping to 1374 unique probes) when adjusting for smoking at the false discovery rate (FDR) level of 0.1 and 986 (954 unique probes) when we did not adjust for smoking [Citation32]. A list of significant probes is provided in Supplement Table 1.
Table 3. DNAm and lung function decline(βLD): Results for βLD association in NAS, the measure of effect on DNAm on the rate of decline in lung function. The interaction between DNAm and follow up time, unique DNA methylation sites associated with a given Pulmonary Function Test (PFT) model organized by smoking analysis, unadjusted (left side) and adjusted for smoking (right side).
Figure 1. QQ plots of P-values for each spirometric model: those associated with βLD are located to the left (a, c, and e) and those with βCS on the right (b, d, and f).
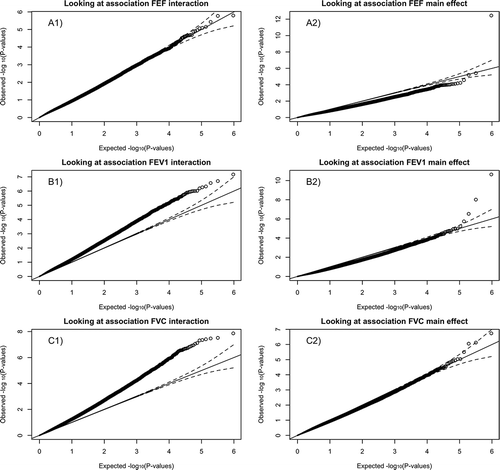
We reran the analysis excluding current smokers (n = 28) to ensure they were not influencing results (-Group 2). Five probes were still associated with rate of decline. Three (cg14292220, cg05644990 and cg26468478) were significant regardless of adjustment for smoking, while the other two (cg01249054 and cg09995068) were significant only when not adjusting for smoking. Probe cg14292220 was only significant in the whole sample (-Group 1) when not adjusting for smoking, while in the sub-group analysis it was significant irrespective of adjustment for smoking. There were 489 (477 unique probes) significant associations at FDR level of 0.1 when adjusting for smoking, and 368 (361 unique probes) when not adjusting for smoking. A list of significant probes and association is provided in Supplement Table 2.
NAS probes associated with cross sectional association (βCS)
When adjusting for smoking there were two probes associated with cross-sectional lung function via the Holm correction (-Group 1). Probe cg05575921 (aryl-hydrocarbon receptor repressor gene AHRR) gene, was associated with, FEV1 and FEF25–75%. The other, cg06126421 (immediate early response 3 gene, IER3), was associated with FEV1. This probe also had a FDR significant association with FVC. The cross-sectional analysis had some under inflation (), yet p-values appeared to follow a uniform distribution ( subplot A2, B2, C2, genomic inflation factor in caption of ). There were eight significant probes when not adjusting for smoking (-Group 1). The βCS estimates magnitudes decreased after adjustment for smoking. There were 5 (3 unique probes) associations when adjusting for smoking at the FDR level of 0.1 and 20 (9 unique probes) associations when not adjusting for smoking. When excluding current smokers, cg05575921 and cg06126421 still had their respective associations when adjusting for smoking (-Group2). There were 3 (2 unique probes) associations when adjusting for smoking and 14 (9 unique probes) associations when not adjusting for smoking the FDR level of 0.1.
Table 4. Cross sectional Associations of DNAm on lung function (βCS): Results for βCS association in NAS, the cross-sectional Association of DNA. Table shows unique DNA methylation sites associated with a given Pulmonary Function Test (PFT) model organized by smoking analysis, unadjusted (left side) and adjusted for smoking (right side).
There is a positive association between DNAm at cg05575921 and the lung function measures (FEV1, FEF25–75) that is modified by smoking in our sample (). In current smokers, there is a positive linear association between lung function measures and DNAm (FEV1 r2= 0.283, FEF25–75% r2= 0.302). For former and non-smokers we do not see as strong a linear relationship (FEV1 r2= 0.081, FEF25–75% r2 = 0.082). This strong difference by smoking status was not observed for cg06126421 and FEV1 (, subplot C, r2 0.13 and 0.07 for current smokers and non-current smokers). There was a similar pattern on the M-value scale (Supplement Figure 1).
KORA replication
In NAS, there were 1377 probes with a FDR significant association (0.1 level) with FEV1, FVC, or FEF25–75%, either via βCS or βLD after adjusting for smoking. KORA had 1052 of these 1377 probes. Four probes replicated in KORA (FDR-significant in KORA and association in the same direction- , more information Supplement Table 3). These four probes replicated in the analysis restricted to KORA men. Only three replicated within the non-sex stratified analysis adjusting for sex. These three probes (cg05575921, cg06126421, and cg15342087) all had significant βCS associations. These three probes association with FEV1 and FEF25–75% also replicated in older men in KORA (55 and up). Probe, cg01086847, was associated with lung function decline, but upon closer examination has a SNP (single nucleotide polymorphism) directly under it. Supplement Figure 2 displays cg15342087 which has a similar pattern of association as cg06126421 in its association with FEV1 (r2 in current smokers 0.09 and 0.03 in non-current smokers in NAS) and is in the same loci (IER3). The AHRR probe also replicated in the analysis restricted to KORA women.
Table 5. Replicated findings across the NAS and KORA cohorts with scaled parameter estimates.
Sensitivity analysis
We next performed a sensitivity analysis where we removed probes with nearby SNPs and also individual outliers (over 1.5 times the interquartile range away from the 1st and 3rd quartile) for each probe. This was done adjusting for medications-corticosteroids, beta adrenergic agonists, and muscarinic receptor antagonists. There were now 384,010 probes. This led to a different set of significant probes (Supplement Tables 4 and 5). There were 1527 (1344 unique probes) significant associations when adjusting for smoking and including current smokers. For βLD, 10 probes were significant via Holm adjustment (Supplement Tables 4 and 5). Eight were significant in the previous analysis either via Holm or FDR adjustment. The two new probes were cg00437258 (DAGLB gene) and cg14219256 (MYST4 gene). For βCS, the two Holm significant probes adjusting for smoking were cg05575921 and cg06126421. For βCS, there were 6 associations over 3 unique probes (cg05575921, cg06126421, and cg15342087). For βLD, the associations were for 1341 unique probes, 704 of which were not significant in the initial analysis (Supplement Tables 4 and 5). Of the 1377 probes found significant in the original analysis adjusting for smoking, 281 were removed either due to having a SNP nearby or being cross-reactive.
Metastabiliy
A subset of NAS men had a secondary DNAm measure which was used (in addition to individuals with only one DNAm observation) to assess our metastability assumption via estimating the intracalass correlation coefficient (ICC) in our 1377 probes. Probes cg05575921 (ICC 0.91) and cg06126421 (ICC of 0.93), had high ICCs suggestive of a level of metastability across time (). These two probes have been found stable in the literature, though low heritability was reported for cg05575921 [Citation34]. Plotting DNAm beta-values for these probes at the next available time point suggested metastability (). In cg15342087 we see less metastability (ICC estimate of 0.67), but the DNAm seen similar between time points (). Supplement Table 6 gives the ICC for each probe significant in the original analysis (sheet 1) or in the sensitivity analysis (sheet 2).
Association with difference in DNAm age and chronological age
We next tested for the correlation between DNAm and Δage, (DNAm age minus chronological age). In the 1377 probes, 663 were FDR significant (adjusting for 1377 tests, at FDR 0.05 level [Citation32]). DNAm at cg05575921 had a negative correlation with Δage (, quadrant IV). Of the 11 probes Holm significant in the NAS analysis adjusting for smoking, six were significantly associated with Δage (Supplement Table 7). In KORA, of the 663 probes associated with Δage in NAS, 497 were available. There were 36 probes that replicated in the KORA analysis (FDR-significant at 0.05 and the same Pearson correlation sign, Supplement Table 8). Probe cg05575921 was not significantly associated with Δage in KORA. In NAS, correlation between DNAm and Δage/age acceleration (residuals of regression of Δage and chronological age) were similar and are displayed in Supplement Table 9.
Figure 4. Scatterplot of DNAm beta estimates and Pearson correlation coefficients for CpGs significantly associated with lung function and significantly correlated with ∆age in the NAS. In the bottom right quadrant, cg05575921 (AHRR gene) is highlighted in red, as it was the only CpG whose association with lung function persisted in both the NAS and KORA, while also being significantly correlated with ∆age in the NAS.
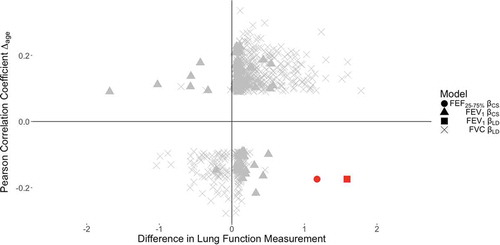
Discussion
In summary, in NAS there were 11 unique DNAm sites significant via Holm correction after adjusting for smoking-related covariates ( and Group 1). In KORA, 3 CpG sites reproduced (cg05575921, cg06126421, and cg15342087; comparison of effects in Table 5). We evaluated the scaled-effect estimate for one-unit change in the standard deviation of this data for cg05575921 and cg0612642. We did not evaluate cg15342087 as it was within 200 BP of cg06126421. For the AHRR probe, it had a similar magnitude of association in its FEV1 association and KORA men/older men (NAS: 0.141, KORA Men and older men: 0.1 and 0.21 respectively). We did not see much similarities for its FEF25–75% association, though they were still all in the same direction upon scaling. In cg06126421 there was a similar pattern for its association with FEV1 (NAS: 0.119, KORA men and older men: 0.127 and 0.146 respectively) but not as much so for its FVC association. The similarity of the scaled associations between NAS and KORA men adds strength to the credibility of the associations in addition to them replicating. In evaluating the correlation of these DNAm marks with biological aging [Citation30], 6 of the 11 NAS loci were significantly associated with Δage (Supplement Table 9). None of these 6 CpG sites overlapped with the CpGs in the algorithm to compute DNAm age [Citation30]. Collectively, this data provide additional evidence suggesting the utility of DNAm age as not only a predictor of chronological age in humans, but also as a potential biomarker of aging-related characteristics, such as lung function.
Examining the βLD probes associated in NAS, there were 12 probes significantly associated with FVC or FEV1. There were seven probes associated with FVC regardless of adjustment for smoking (). These seven probes had varying evidence of metastability (ICC 7.9e-14 to 0.99). One probe with high ICC was cg13532885 (ICC 0.93 SYN1) which was also associated with Δage. The ICC did not appear to vary by smoking status (0.9 and 0.94 in never and former smokers). Another probe, cg05644990, had very low ICC (7.9e-14, 4.34E-14, and 7.02e-17 in overall, never, and former respectively) and was also associated with Δage. These two probes may implicate different biological functions that influence PFTs. These findings for cg05644990 and cg13532885 did not reproduce in KORA. This failure to reproduce may be due to: the older age of the NAS, the smaller time interval studied in KORA, or the lack of metastability. In FEV1, βLD significant probes were much more likely to be affected by smoking variables as there were no probes significant regardless of smoking adjustment. Probe cg18476993 (ICC of 0.64, never vs former: 0.51 vs 0.67) was the only probe significant for FEV1 after adjusting for smoking in the larger group. The probes associated with FEV1 were not associated with FVC suggesting an association with airway function and less with the alveolar region of these probes. This agrees with the pathophysiologic understanding of cigarette smoke related COPD. The remaining probes associated with FEV1, were likely being driven by the small number of smokers in our study, as they were not significant in the analyses in just former and never smokers (-Group 2).
For the cross-sectional analysis (βCS), results were mainly observed for FEV1 and FEF25–75%, with little association in FVC (-Group 1). This suggests these probes are related to airway function. Upon adjustment for smoking we saw the magnitude of association attenuate (). Observed hits were in the three probes cg06126421, cg15342087, and cg05575921. These probes were associated with FEV1. All three were associated with FVC when not accounting for smoking status, with only cg06126421 still significant after adjustment. The AHRR probe was the only one associated with FEF25–75%. It should be noted that there has been evidence of SNPs near cg05575921, though these SNPs did not appear to confound the analysis in a reported study on maternal smoking [Citation35]. Probe cg15342087, while not HOLM significant, did pass the FDR threshold of 0.1 with FEV1 and did replicate in KORA. The other probes in are likely being driven by smoking status.
Poor lung function is either due to a low plateau or a rapid age-related decline and is a predictor for lung morbidity and mortality. In our analysis, there was no overlap between probes associated with cross sectional or longitudinal decline in FEV1, FVC, and FEF25–75%. This suggests that the βCS significant probes are mainly determined or associated with the growth phase of an individual, i.e. the leveling off of lung function around the age of 20 as discussed in the introduction and are only slightly modulated by the age-related decline. We can see this in the differences in the associations, with the probes with a significant βLD association being solely with measures of volume related spirometric indices (FVC or FEV1). Meanwhile, the probes found with a significant βCS association, were mainly associated with lung function parameters that are reflective of airway function, with only one being associated with FVC.
While our two top hits, cg05575021 and cg06126421, appeared metastable (high ICC and reported in the literature [Citation34]) with our study design it is impossible to prove our metastable assumptions. These associations may be driven by a persistent impact of smoking. We ran a stratified analysis of the three cross-sectional probes that replicated in KORA by former or never smokers in NAS. Testing DNA methylation at cg05575921 with FEV1 and FEF25–75% in never smokers was nominally significant (p-value of 0.04 and 0.07 respectively). DNAm at cg06126421 was not associated with FEV1 and FVC in never smokers (p-value of 0.33 and 0.49 respectively). In addition, the test for DNAm at cg15342087 and FEV1 was not significant (p-value of 0.26). In the analysis restricted to former smokers, we continued to adjust for pack-years and in addition include time since quitting. DNAm at cg05575921 was still significantly associated with FEV1 and FEF25–75% (p-values of 5.3e-08 and 2.3e-07 respectively), as was cg06126421 with FEV1 and FVC (p-values of 4.28e-06 and 1.03e-04 respectively), and cg15342087 (p-value of 4.2e-05 for FEV1). However, we cannot rule out persistent smoking effects that are not adequately modeled by pack years or time since quitting. There could be an issue of power as there are fewer never smokers in our analysis than former smokers (n = 179/426 never vs former). We believe the analysis is still useful as it highlights potential biological pathways related to lung function that future studies can elucidate.
Another potential limitation to our study is potential bias due to unmeasured diseases. To examine this, we analyzed the three probes (cg06126421, cg15342087, and cg05575921) adjusting for chronic lung diseases (either asthma and/or COPD defined by FEV1/FVC < 0.7). This led to a decrease in effect size, about 25% lower, but still highly significant association (Supplement Table 10). This suggests that the observed associations are not primarily driven by chronic lung diseases but appear to be amplified by these diseases. In addition, the differing study designs of KORA and NAS may be the reason we failed to replicate any lung function decline associations within KORA. The associations in NAS may be indicative of previous smoking exposure, while in KORA it may be interactive associations.
This difference in study design is also a strength as the NAS cohort was assessed retrospectively (i.e. blood samples were drawn at the end of a series of lung function testing at multiple times over 10 plus years for each individual), whereas KORA was assessed prospectively (i.e. blood samples were taken at baseline and followed by lung function testing at multiple times over a similar timespan) (). This design helped in facilitating: (i.) the discovery of potentially metastable DNAm sites retrospectively for evaluating lung function decline within an elderly population (NAS cohort); and (ii.) replication and confirmation of these methylation sites that may precede lung function decline in younger humans, too (KORA). However, this differing study designs of KORA and NAS may be the reason we failed to replicate any lung function decline associations within KORA. The associations in NAS may be indicative of previous smoking exposure, while in KORA it may be interactive associations. For our top three probes that replicated in KORA there was strong evidence of metastability in our sample (). Throughout our work, metastability was assessed by calculating the ICC of DNAm from blood samples taken 3–5 years apart ( and ); and reported in the literature [Citation34].
All of these findings led us to a novel working model, which can serve to integrate and contextualize our results. As discussed in the literature [Citation22,Citation23], DNAm status is highly sensitivity to smoking status (seen in our study , subplot A and B). Here we show that it is also associated with PFTs, and, in some settings, with aging (Δage). These findings are plausible as AHRR has been implicated in the detoxification of tobacco smoke components protecting against oxidative stress and inflammation as well as having tumor suppressing effects [Citation36]. Mechanistically, AHRR has been shown to be induced eg. by wood smoke exposure leading to a reduction of AHR-mediated anti-inflammatory action of the arachidonic acid pathway [Citation37]. The data ( and and Supplement Table 8) shows that high DNAm status at cg05575921 in vivo correlates with greater pulmonary function and decreased aging (). To our knowledge, this is the first time that directional changes in aging have been associated with DNAm status at cg05575921 in the literature. The other consistent probe, cg06126421 (IER3) did not appear to have an effect modification with smoking or be associated with Δage. This probe has been found to be associated with smoking and all cause mortality in previous studies [Citation38–Citation41]. These two CpG loci may inform pulmonary function studies in complementary and nuanced ways, given that they may represent different epigenetic networks in vivo. The probe cg15342087 is near cg06126421 and has been found as well to be associated with all-cause mortality as well [Citation41]. IER3 has been described to be involved in the immune response and inflammation, however, it is induced by a variety of external and internal factors [Citation42] and a possibly more specific role for the respiratory system remains to be elucidated. Further investigation into DNAm at the AHRR gene may help to uncover tools/strategies to better dissect the link between lung function, aging, and smoking. DNAm at the IER3 gene, however, may be more directly linked to lung function and respiratory outcomes irrespective of factors such as smoking/aging.
Materials and methods
Normative aging study cohort (NAS)
The study included 633 participants from the NAS, a closed cohort of community-dwelling men living within the Greater Boston metropolitan area [Citation43]. We obtained and analyzed DNA samples specifically from 1999 to 2007. As detailed in , participants underwent up to 4 total examinations (or less) with a 3- to 5-year space between visits. Blood was isolated at the last visit and a number of measures were collected, such as age, education, height, weight, and medication status (e.g., use of sympathomimetic alpha and beta, anticholinergics, etc.). Pathophysiological lung conditions – e.g., asthma, chronic bronchitis, emphysema, etc. – were evaluated by a physician, and smoking data was collected via the American Thoracic Society (ATS) questionnaire [Citation44]. We used FVC, FEV1, and FEF25–75% to assess pulmonary function. These spirometric parameters were measured following the ATS guidelines as previously reported [Citation45,Citation46], and were measured over time going back at most four visits for our study. Further, a methacholine challenge test was administered to assist in the diagnosis of asthma [Citation47]. This study was approved by the Institutional Review Boards of all relevant institutions, and all participants provided signed informed consent.
Cooperative health research in the region of Augsburg cohort (KORA)
The present work included participants from the population-based study KORA F4 and its follow-up study, KORA F4L. Blood for buffy coat was sampled in an EDTA-Falcon-Tube (9 ml) and collected via venepuncture from the cubital fossa. Experimental design details about these studies has been reported previously [Citation48–Citation50]. Information on the epigenetic design and DNA methylation processing has been published in Zeilinger et al and Panni et al [Citation23,Citation51]. Briefly, spirometry was performed in 1321 human participants aged 41–63 years from the Augsburg region between 2006–2008 in KORA F4. Spirometry was then re-measured after approximately 3.1 years in participants of the KORA F4L study. Spirometry was performed in accordance with European Respiratory Society and ATS guidelines [Citation45,Citation49,Citation52], and information about the presence of respiratory diseases, medication and smoking status was assessed by a standardized interview and/or questionnaire (as published previously). The Ethics Committee of the Bavarian Medical Association approved both studies, KORA F4 and KORA F4L, and informed consent was obtained from all research study participants [Citation51]. Of this 1321 study participants, 868 had DNA methylation and passed quality control.
Detection and analysis of DNA methylation
Buffy coat DNA was isolated from each sample via the QIAamp DNA Blood Kit (QIAGEN, Valencia, CA) and a 0.5 µg aliquot was bisulfite converted with the EZ-96 DNA Methylation Kit (Zymo Research, Orange, CA). In the NAS, this was done on blood collected between 1999 and 2007. In the NAS, DNA methylation was detected by the Infinium HumanMethylation450 BeadChip platform (Northwestern University, Feinberg School of Medicine, Center for Genetic Medicine). Technical effects due to the plate/chip were minimized by utilizing a two-stage age-stratified algorithm to randomize the samples, thereby ensuring comparable age distribution across plates/chips. Specifically, we arranged the samples by stratified randomization onto blocks for the Illumina BeadChips (grouped in sets of 12) and analytic plates (sets of 8 BeadChips), ensuring that repeated DNA measures from a person were located on the same BeadChip and stratified by age quartile; we also employed statistical validations to confirm evenness across plates/chips by age and other cohort characteristics.
Quality control samples consisted of replicate pairs and a single sample that was run within and between plates/chips to help detect batch effects. Analytic plates were run consecutively, by the same technician, and processed and read on the same scanner. Quality control approaches also included the detection and removal of 15 DNA samples and 949 probes via the pfilter command in the Bioconductor wateRmelon package [Citation53], which excluded DNA samples containing >1% of probes with detection P-values >0.05 and probes having >1% of samples with detection P-value >0.05 (after omitting samples excluded above). Furthermore, we also excluded probes with specific design and/or annotation, namely: 65 with genotyping function; 3091 used for detecting CpH methylation (CpH are non CpG methylation sites); and 3688 containing a SNP in the last 10 bases with a minor allele frequency greater than 0.01 in the CEU reference set. A number of these probes were already excluded by the pfilter command, so after these steps we finally obtained 477,927 probes, i.e. ~98.4% out of 485,512, which were used to obtain DNA methylation. Lastly, we applied a 3-part, pre-processing pipeline to our data: (i.) background correction via the out-of-band (noob) method by Triche et al [Citation54].; (ii.) dye-bias adjustment by the Bioconductor methylumi package [Citation55]; and (iii.) probe-type correction with BMIQ according to Teschendorff et al. (2013) [Citation56], as provided by wateRmelon [Citation53]. DNA samples from the KORA cohort were analyzed similarly to the detailed scheme that is provided above for the NAS, as is reported in the literature [Citation51].
Biostatistical analyses
In the NAS, we analyzed FEF25–75%, FEV1, and FVC. We fit a mixed-effect model to analyze the data, holding the DNAm at the probe constant and using lung function measures at the time of blood drawn and the three prior visits of blood being drawn as the dependent variable. DNAm was the independent variable of interest. Doing this allowed us to account for the correlation between lung function measures from the same individual. FEF25–75% was square root transformed to better approximate a normal distribution. The predictor for lung function was percent of DNAm (i.e. the beta-value). This analysis was done using the lme4 package in R [Citation57]. Given the large number of observations, we treated the resulting t-statistic as normal to perform inference. The model is given below for the DNA methylation at probe for spirometric measure at time k for individual
. Xi is the set of confounders. The follow up time (time between first included visit and current visit) is
, with
if k = 1. A study participant could have k up to 4.
We included an interaction between the beta-value and follow-up time. There are two associations of interest: (i.) the cross-sectional association of DNAm on lung function, βCS (when follow-up time is 0, CS for cross sectional), and (ii.) the interaction between DNAm and follow-up time, βLD (LD for longitudinal decline). Furthermore, we examined models without and with adjustment for smoking-related confounders (smoking status and pack years). General covariates common to all models were: season, follow up time, day of the week, plate, chip, weight mean centered, weight mean centered squared, the natural log of height, baseline age, vitamin C intake not from vitamin pills, maximum years of education, and taking any of the following drugs-corticosteroids (inhaled (ICS) or systemic), beta-2-adrenergic agonists (typical LABA), sympathomimetic alpha AHFS, or long acting muscarinic antagonist (LAMA) ( and ). Cell-type proportions were estimated via Houseman et al [Citation31]., (), and were included as additional covariates. To see if results were influenced by the (current) smokers, we reran the models restricted to the former and never smokers. For each DNAm probe, we again tested for the cross-sectional (βCS) and interaction (βLD) terms defined above.
A probe was considered genome-wide-significant if it had a Holm adjusted p-value less than 0.05 [Citation33] or significant at an FDR level of 0.10 [Citation32]. Holm procedure is a more conservative approach than FDR and controls the family wise error rate. Replication was performed in the KORA cohort. Replication in KORA was done in: (A) use all participants/ages (controlling for sex); B) restricted to men; (C) only the oldest men (55 and up, to mirror the NAS); D) restricted to women. This methodology was adopted to ensure comparability of our results, as the NAS is an all-male cohort. We adjusted our KORA models for cigarette use (smoking status and pack-years).
To compute DNAm metastability, we utilized second observations of methylation on a small proportion of NAS individuals while still using the individuals’ observations with one observation. We computed the intraclass correlation coefficient (ICC) using random effects model. for each probe of interest to assess DNAm stability over time. The ICC was calculated using random effect modeling and measuring the proportion of total variation coming from the shared variance. The ICC gives a measure of the validity of the metastability assumption. A value closer to one indicates metastability.
For sensitivity, we re-ran the analysis removing cross-reactive probes, probes that were associated with a SNP with MAF greater 5%, non-specific probes, polymorphic CpG sites, and probes with a SNP within 10BP of the target site [Citation58]. We also dropped sympathomimetic alpha AHFS from our definition of medicine taking as it is not often taken with COPD. This brought the number of probes down to 384,010. For each probe, we reran the analysis removing any outliers where the DNAm beta-value was greater than 3 interquartile ranges outside the 25% and 75% quartiles for that probe.
Calculation of dnam age, Δage, and relationship with lung function associated CpGs
DNAm age is a predictor of chronological age calculated by using DNAm measurements from 353 CpG sites shared by both the Illumina HumanMethylation27 and HumanMethylation450 BeadChip platforms [Citation30]. This biocalculator has been shown to maintain predictive accuracy across human tissues [Citation30]. We calculated an age-difference variable, ‘Δage,’ by subtracting a participant’s DNAm age (predicted age given their epigenetic profile) from his/her chronological age (their actual age). A positive Δage is illustrative of enhanced/increased aging, and vice versa. We tested if any of the significant probes (βCS or βLD) from the lung function models, were also significantly associated with Δage. This was done via a Pearson correlation test between the two measures. We also examined age acceleration which are the residuals after regression biological age from DNAm age.
Author contributions
J.J.C. and R.T.B. contributed equally to this work as first authors; J.J.C and R.T.B. conceived of the study, participated in study design and coordination, and wrote the manuscript. R.T.B analyzed the data and generated figures. R.T.B., T.P., and J.N.H., designed and performed bioinformatic analyses. J.N-E., A.C.J., E.C., S.K., S.W., S.K., N.J., Y.Z., L.H., D.L.D., A.A.L., P.S.V., and A.P. participated in study design and expert input regarding data analysis throughout the project’s life course. As senior faculty mentors, X.L., J.S., H.S., and A.A.B. co-conceived of the study with the co-first authors, participated in study design, helped to coordinate the study, and supervised all aspects of the work.
Declarations
Information pertaining to ethical approval statements is listed in the Materials and methods section. All authors read and approved the manuscript.
Supplemental Material
Download Zip (1.5 MB)Acknowledgments
We thank all of the human participants involved in both the NAS and KORA cohorts: we are grateful for their participation and cooperation. We also thank the clinical staff and scientific teams associated with these cohorts based in the USA and Germany for their excellent care of all participants. The NAS computations in this paper were run on the Odyssey cluster supported by the FAS Division of Science, Research Computing Group at Harvard University. Lastly, we also thank the Genomics Core Facility at Northwestern University for their technical assistance regarding the Infinium HumanMethylation450K BeadChip technology.
Disclosure statement
No potential conflict of interest was reported by the authors.
Supplementary material
Supplemental data for this article can be accessed here.
Additional information
Funding
References
- Soler Artigas M, Wain LV, Miller S, et al. Sixteen new lung function signals identified through 1000 Genomes Project reference panel imputation. Nat Commun. 2015;6:8658.
- Weiss ST. Lung function and airway diseases. Nat Genet. 2010;42:14–16.
- Wain LV, Shrine N, Artigas MS, et al. Genome-wide association analyses for lung function and chronic obstructive pulmonary disease identify new loci and potential druggable targets. Nat Genet. 2017;49:416–425.
- Maher B. Personal genomes: the case of the missing heritability. Nature. 2008;456:18–21.
- Morrow JD, Cho MH, Hersh CP, et al. DNA methylation profiling in human lung tissue identifies genes associated with COPD. Epigenetics. 2016;11:730–739.
- Obeidat M, Hao K, Bosse Y, et al. Molecular mechanisms underlying variations in lung function: a systems genetics analysis. Lancet Respir Med. 2015;3:782–795.
- Piletic K, Kunej T. MicroRNA epigenetic signatures in human disease. Arch Toxicol. 2016;90:2405–2419.
- Joehanes R, Just AC, Marioni RE, et al. Epigenetic signatures of cigarette smoking. Circ Cardiovasc Genet. 2016.
- LaCroix AZ, Omenn GS. Older adults and smoking. Clin Geriatr Med. 1992;8:69–87.
- Kerstjens HA, Rijcken B, Schouten JP, et al. Decline of FEV1 by age and smoking status: facts, figures, and fallacies. Thorax. 1997;52:820–827.
- Speizer FE, Tager IB. Epidemiology of chronic mucus hypersecretion and obstructive airways disease. Epidemiol Rev. 1979;1:124–142.
- Godtfredsen NS, Lam TH, Hansel TT, et al. COPD-related morbidity and mortality after smoking cessation: status of the evidence. Eur Respir J. 2008;32:844–853.
- Vercelli D. Does epigenetics play a role in human asthma? Allergol Int. 2016;65:123–126.
- Gruzieva O, Merid SK, Melen E. An update on epigenetics and childhood respiratory diseases. Paediatr Respir Rev. 2014;15:348–354.
- Comer BS, Ba M, Singer CA, et al. Epigenetic targets for novel therapies of lung diseases. Pharmacol Ther. 2015;147:91–110.
- Pal S, Tyler JK. Epigenetics and aging. Sci Adv. 2016;2:e1600584.
- Sin DD, Wu L, Man SF. The relationship between reduced lung function and cardiovascular mortality: a population-based study and a systematic review of the literature. Chest. 2005;127:1952–1959.
- Hole DJ, Watt GC, Davey-Smith G, et al. Impaired lung function and mortality risk in men and women: findings from the Renfrew and Paisley prospective population study. BMJ. 1996;313: 711–715. discussion 5–6.
- Lee HM, Le H, Lee BT, et al. Forced vital capacity paired with Framingham Risk Score for prediction of all-cause mortality. Eur Respir J. 2010;36:1002–1006.
- Higgins M. Risk factors associated with chronic obstructive lung disease. Ann N Y Acad Sci. 1991;624:7–17.
- Hoyert DL, Xu J. Deaths: preliminary data for 2011. Natl Vital Stat Rep. 2012;61:1–51.
- Fasanelli F, Baglietto L, Ponzi E, et al. Hypomethylation of smoking-related genes is associated with future lung cancer in four prospective cohorts. Nat Commun. 2015;6:10192.
- Zeilinger S, Kuhnel B, Klopp N, et al. Tobacco smoking leads to extensive genome-wide changes in DNA methylation. PLoS One. 2013;8:e63812.
- Bauer T, Trump S, Ishaque N, et al. Environment-induced epigenetic reprogramming in genomic regulatory elements in smoking mothers and their children. Mol Syst Biol. 2016;12:861.
- Gibbs K, Collaco JM, McGrath-Morrow SA. Impact of tobacco smoke and nicotine exposure on lung development. Chest. 2016;149:552–561.
- Drummond MB, Hansel NN, Connett JE, et al. Spirometric predictors of lung function decline and mortality in early chronic obstructive pulmonary disease. Am J Respir Crit Care Med. 2012;185:1301–1306.
- Donaldson GC, Seemungal TA, Bhowmik A, et al. Relationship between exacerbation frequency and lung function decline in chronic obstructive pulmonary disease. Thorax. 2002;57:847–852.
- Mannino DM, Buist AS, Petty TL, et al. Lung function and mortality in the United States: data from the First National Health and Nutrition Examination Survey follow up study. Thorax. 2003;58:388–393.
- Anthonisen NR, Connett JE, Murray RP. Smoking and lung function of Lung Health Study participants after 11 years. Am J Respir Crit Care Med. 2002;166:675–679.
- Horvath S. DNA methylation age of human tissues and cell types. Genome Biol. 2013;14:R115.
- Houseman EA, Accomando WP, Koestler DC, et al. DNA methylation arrays as surrogate measures of cell mixture distribution. BMC Bioinformatics. 2012;13:86.
- Benjamini Y, Hochberg Y. Controlling the false discovery rate: a practical and powerful approach to multiple testing. J Royal Stat Soc Ser B (Methodological). 1995;57:289–300.
- Holm S. A simple sequentially rejective multiple test procedure. Scand J Stat. 1979;6:65–70.
- Shah S, McRae AF, Marioni RE, et al. Genetic and environmental exposures constrain epigenetic drift over the human life course. Genome Res. 2014;24:1725–1733.
- Gonseth S, de Smith AJ, Roy R, et al. Genetic contribution to variation in DNA methylation at maternal smoking-sensitive loci in exposed neonates. Epigenetics. 2016;11:664–673.
- Traboulsi H, Guerrina N, Iu M, et al. Inhaled pollutants: the molecular scene behind respiratory and systemic diseases associated with ultrafine particulate matter. Int J Mol Sci. 2017;18:243.
- Awji EG, Chand H, Bruse S, et al. Wood smoke enhances cigarette smoke-induced inflammation by inducing the aryl hydrocarbon receptor repressor in airway epithelial cells. Am J Respir Cell Mol Biol. 2015;52:377–386.
- Elliott HR, Tillin T, McArdle WL, et al. Differences in smoking associated DNA methylation patterns in South Asians and Europeans. Clin Epigenetics. 2014;6:4.
- Shenker NS, Polidoro S, van Veldhoven K, et al. Epigenome-wide association study in the European Prospective Investigation into Cancer and Nutrition (EPIC-Turin) identifies novel genetic loci associated with smoking. Hum Mol Genet. 2013;22:843–851.
- Zhang Y, Schottker B, Florath I, et al. Smoking-associated DNA methylation biomarkers and their predictive value for all-cause and cardiovascular mortality. Environ Health Perspect. 2016;124:67–74.
- Zhang Y, Wilson R, Heiss J, et al. DNA methylation signatures in peripheral blood strongly predict all-cause mortality. Nat Commun. 2017;8:14617.
- Arlt A, Schafer H. Role of the immediate early response 3 (IER3) gene in cellular stress response, inflammation and tumorigenesis. Eur J Cell Biol. 2011;90:545–552.
- Bell B, Rose CL, Damon A. The Veterans Administration longitudinal study of healthy aging. Gerontologist. 1966;6:179–184.
- Ferris BG. Epidemiology standardization project (American Thoracic Society). Am Rev Respir Dis. 1978;118:1–120.
- Miller MR, Hankinson J, Brusasco V, et al. Standardisation of spirometry. Eur Respir J. 2005;26:319–338.
- Sparrow D, O’Connor G, Colton T, et al. The relationship of nonspecific bronchial responsiveness to the occurrence of respiratory symptoms and decreased levels of pulmonary function. The normative aging study. Am Rev Respir Dis. 1987;135:1255–1260.
- Chatham M, Bleecker ER, Norman P, et al. A screening test for airways reactivity. An abbreviated methacholine inhalation challenge. Chest. 1982;82:15–18.
- Holle R, Happich M, Lowel H, et al. KORA–a research platform for population based health research. Gesundheitswesen. 2005;67 Suppl 1:S19–25.
- Karrasch S, Flexeder C, Behr J, et al. Spirometric reference values for advanced age from a South german population. Respiration. 2013;85:210–219.
- Tang W, Kowgier M, Loth DW, et al. Large-scale genome-wide association studies and meta-analyses of longitudinal change in adult lung function. PLoS One. 2014;9:e100776.
- Panni T, Mehta AJ, Schwartz JD, et al. A genome-wide analysis of DNA methylation and fine particulate matter air pollution in three study populations: KORA F3, KORA F4, and the normative aging study. Environ Health Perspect. 2016;124:983.
- Miller MR, Crapo R, Hankinson J, et al. General considerations for lung function testing. Eur Respir J. 2005;26:153–161.
- Pidsley R, Wong CCY, Volta M, et al. A data-driven approach to preprocessing Illumina 450K methylation array data. BMC Genomics. 2013;14:293.
- Triche TJ Jr., Weisenberger DJ, Van Den Berg D, et al. Low-level processing of Illumina Infinium DNA Methylation BeadArrays. Nucleic Acids Res. 2013;41:e90.
- Davis S, Du P, Bilke S, et al. methylumi: handle Illumina methylation data. 2015.
- Teschendorff AE, Marabita F, Lechner M, et al. A beta-mixture quantile normalization method for correcting probe design bias in Illumina Infinium 450 k DNA methylation data. Bioinformatics. 2013;29:189–196.
- Bates D, McHler M, Bolker BM, et al. Fitting linear mixed-effects models using lme4. J Stat Softw. 2015;67:1–48.
- Chen YA, Lemire M, Choufani S, et al. Discovery of cross-reactive probes and polymorphic CpGs in the Illumina Infinium HumanMethylation450 microarray. Epigenetics. 2013;8:203–209.