ABSTRACT
DNA methylation is dynamic, varies throughout the life course, and its levels are influenced by lifestyle and environmental factors, as well as by genetic variation. The leading genetic variants at stroke risk loci identified to date explain roughly 1–2% of stroke heritability. Most of these single nucleotide polymorphisms are situated within a regulatory sequence marked by DNase I hypersensitivity sites, which would indicate involvement of an epigenetic mechanism. To detect epigenetic variants associated with stroke occurrence and stroke subtypes.
A two-stage case–control epigenome-wide association study was designed. The discovery sample with 401 samples included 218 ischaemic stroke (IS) patients, assessed at Hospital del Mar (Barcelona, Spain) and 183 controls from the REGICOR cohort. In two independent samples (N = 226 and N = 166), we replicated 22 CpG sites differentially methylated in IS in 21 loci, including 2 CpGs in locus ZFHX3, which includes known genetic variants associated with stroke. The pathways associated with these loci are inflammation and angiogenesis. The meta-analysis identified 384 differentially methylated CpGs, including loci of known stroke and vascular risk genetic variants, enriched by loci involved in lipid metabolism, adipogenesis, circadian clock, and glycolysis pathways.
We identified a set of 22 CpGs in 21 loci associated with IS. Our analysis suggests that DNA methylation changes may contribute to orchestrating gene expression that contributes to IS.
KEYWORDS:
Introduction
Ischaemic stroke (IS), the aetiology of 80% of all strokes, is a complex and heterogeneous disease with high rates of mortality and long-term disability. Stroke pathogenesis involves a number of different disease processes as well as interactions between environmental, vascular, systemic, genetic, and central nervous system factors [Citation1].
In recent years, genome-wide association studies (GWAS) have identified many genes robustly associated to IS and its subtypes which show a distinct genetic background, but the underlying genes and pathways are not yet fully understood. Half of the identified genes share a genetic association with other vascular traits; the greatest correlation is with blood pressure. However, the underlying biological pathways do not seem to involve known vascular risk factors and thus the new pathways may constitute new targets for stroke prevention [Citation2].
The heritability of stroke, as calculated from genome-wide data, has been estimated to be 30–40% [Citation3–Citation5]. The lead variants at stroke risk loci identified to date explain roughly 1–2% of this heritability and their number remains relatively small compared to other common conditions, such as coronary artery disease [Citation6]. Moreover, most of these leading single nucleotide polymorphisms (SNPs) reside within intergenic or intronic regions, and most are situated within a regulatory sequence marked by DNase I hypersensitivity sites, which would indicate the involvement of an epigenetic mechanism [Citation2,Citation7,Citation8].
DNA methylation (DNAm), an epigenetic mechanism essential for regulation of gene expression, consists of the covalent addition of a methyl group to a cytosine nucleotide, primarily in the context of a CpG dinucleotide. DNAm is dynamic, varies throughout the life course, and its levels are influenced by lifestyle and environmental factors, as well as by genetic variation [Citation9].
Stroke epigenetics research has revealed that stroke patients are globally hypomethylated and are epigenetically older than healthy controls. This accelerated ageing is strongly associated with 3-month IS outcome and mortality, independently of health profile, lifestyle, and genetic characteristics [Citation10–Citation13].
We hypothesized that DNA methylation markers would identify additional stroke risk loci and improve the mapping of genomic variability, providing new insights into stroke pathology. The aim of the present study was to detect epigenetic variants associated with IS occurrence and stroke subtypes.
Methods
The data that support the findings of this study are available from the corresponding author upon reasonable request.
Study design
A two-stage case–control epigenome-wide association study (EWAS) was designed, including discovery and replication analyses. The discovery sample consisted of 401 samples (183 controls and 218 IS patients). Two independent samples (N = 392,226 from Hospital del Mar [MAR-2] and 166 from Hospital Vall d’Hebron [HVH]) were used to replicate the top 500 methylation-variable positions (MVPs) identified in the discovery sample with an arbitrary p-value ≤7.1 × 10−6. Joint meta-analysis of all three samples was performed (N = 793). Stratification analysis by Trial of Org 10172 in Acute Stroke Treatment (TOAST) was performed. Functional and pathway analyses were conducted.
Study sample
European ancestry controls and patients with a diagnosis of IS according to World Health Organization criteria were selected.
Epigenome-wide association studies
After EWAS quality controls, association analysis was performed in controls and IS samples using a multivariate linear regression. The top MVPs identified in the discovery stage were analysed in the validation samples. The results were joined in a weighted z-score meta-analysis. Stratification analysis by TOAST criteria was performed using a multivariate linear regression for large-artery atherosclerosis (LAA), cardioembolic (CE), small vessel disease (SVD), and undetermined (UND) aetiologies. Functional and pathway analyses were conducted. All MVPs with p-values <1.39 × 10−7 were considered statistically significant for a genome-wide approach.
Results
Discovery stage. Genome-wide effect of ischaemic stroke on methylation status
Of the 865,859 initial CpG sites in Infinium MethylationEPIC Beadchip, a total of 358,709 (41.4%) that passed the quality controls and were common in HumanMethylation450 Beadchip were included in the discovery analysis (Table S1–2). Genome-wide DNAm analysis from whole blood was performed in the discovery sample (N = 401). Clinical and demographic differences between the IS and control individuals are shown in .
Table 1. Descriptive characteristics of the samples.
Top 500 CpG sites showing a suggestive association with methylation differences between IS and control samples were selected for replication, with an arbitrary p-value ≤7.1 × 10−6 (Supplementary Table S3). Manhattan and QQ plots are shown in .
Replication stage
After applying the same quality control steps and normalization as in the discovery analysis, the validation analysis included 392 individuals (226 from MAR-2 and 166 from HVH). The characteristics of the populations included in the replication stage are shown in –. We replicated 93 CpGs in one replication sample and 114 CpGs in the other; 22 MVPs in 21 loci were replicated in both samples. All of the 22 MVPs were hypomethylated in all the samples, except the one located at MAPK1; however, IS samples showed significantly higher methylation levels, compared to controls (, Supplementary Figure S1). Function and traits associated with the 21 loci that harbour the 22 CpGs identified are described in Supplementary Table S4–7.
Table 2. CpG sites differentially methylated between ischaemic stroke and controls in discovery sample validated in two replication samples and the meta-analysis.
We used the GeneMANIA algorithm on the 21 candidate genes (CAMSAP3, SLC35E1, ZFHX3, PIM3, MAPK1, LRRC26, HIF1A, RNF126, SENP3, ANAPC11, PLBD2, CCNL2, PUM1, ITPKB, NAPA, IL15RA, ACSL1, JMY, PUF60, CHSY1, BAMBI) and known IS-related genes [Citation2]. We found that our candidate genes have genetic interactions and are co-expressed with known IS genes (Supplementary Figure S2). FGA and ZFHX3 are involved in the same pathway, as are HIF1A and FURIN, HIF1A and MAPK1. Shared protein domains exist between HIF1A and TWIST1, LRRC26 and LRCH1, ANACPC11 and RNF126, ZFHX3 and ZNF318, and CHSY1 and ABO. CDK6 shares with MAPK1 and PIM3. Finally, there is co-localization expression of ITPKB and SH3PXD2A; NAPA and RGS7; NAPA, RGS7, ZFHX3, and MAPK1; ZFHX3 and TBX3; and IL15RA, CASZ1, and FOXF2.
The 22 validated CpGs include 2 CpGs in ZFHX3 loci that harbour known stroke variants. The pathway analysis showed HIF1A and MAPK1 are associated with angiogenesis (FDR p-values = 0.014). IL15RA and HIF1A are involved in inflammatory and interferon gamma response (FDR p-values = 0.034). Additionally, NAPA and MAPK1 are involved in protein secretion functions (FDR p-values = 0.024) (Supplementary Table S5). GTEX data indicate that 20 loci (the exception being LRRC26) are expressed in blood vessels and brain (Supplementary Figure S3).
These 22 MVPs are associated to CpG islands: 20 are in an enhancer-associated position, 2 are associated to H3K27AC (indicating active gene expression), and 21 are located in a DNase I hypersensitive site, functionally related to transcriptional activity (Supplementary Table S7).
Meta-analysis
Meta-analysis results of all 3 samples (N = 793) are shown in Supplementary Table S8. A total of 384 MVPs were significant after Bonferroni correction (multiple testing). However, 30 (7.8%) of these MVPs were only present in two samples.
The 384 significantly associated CpGs include 4 CpGs in ZFHX3, SH2B3, and WNK1 loci, which harbour known stroke variants. The traits and diseases associated with loci containing these CpGs are shown in . The functional analysis of the significant loci showed enrichment for genes involved in adipogenesis (FDR p-value = 4.13 × 10−4), triglyceride, and lipid metabolism (FDR p-value = 0.044), circadian clock (FDR p-value = 0.044), and regulation of glycolysis pathway (FDR p-value = 0.037) (Supplementary Tables S9–11).
Figure 2. Summary of loci harbouring significant CpGs in meta-analysis, overlapping known genome-wide associations to phenotypes and diseases. (a) 115 CpG (30%) of the 384 joint CpGs are associated with known variants. Bars represent the number of loci associated with each trait; 37 loci are associated with more than 1 trait. (b) List of loci with known associations. In bold, loci validated in discovery.
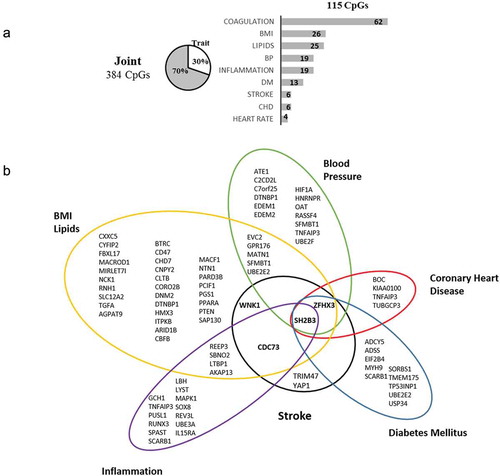
EWAS stratified analysis by IS subtypes
We combined the IS samples from the two Hospital del Mar samples (MAR_1 and MAR_2, N = 627) to increase the statistical power to identify MVP sites associated to IS subtypes (TOAST classification), stratified by LAA, CE, SVD, and UND. Clinical and demographic characteristics and EWAS results are shown in Supplementary Material (Table S12–16). Manhattan and QQ plots are in Supplemental Figure S4. In the meta-analysis, UND and SVD subtypes were the main contributors of significant IS-associated CpGs ().
Figure 3. Venn Diagrams showing the overlap of methylation-variable positions between meta-analysis and the ischaemic stroke subtypes. LAA, large-artery atherosclerosis: CE, cardiembolic; SVD, small vessel diseases; UND, undetermined IS subtypes.
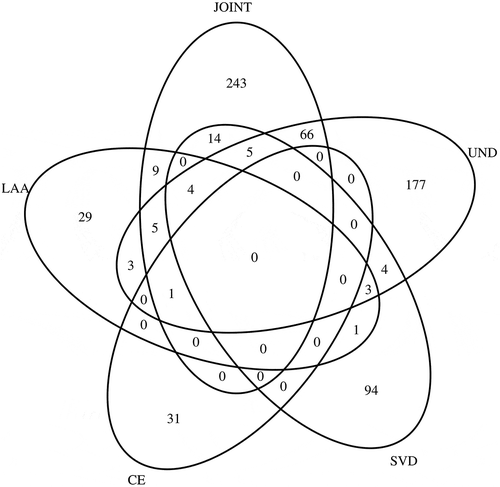
In LAA subtype, we identified 57 MVPs suggestive of statistical significance (Supplemental Table S13). There was an enrichment for genes involved in plasma high-density lipoprotein cholesterol (HDL-C) levels (FDR p-values = 0.039) and nominal significance for the adipogenesis pathway (p-values = 0.0023) (Supplementary Tables S17). In CE subtype, we identified 31 CpGs (Supplemental Table S14). The functional analysis showed the insulin signalling pathway as nominally significant (p-values = 1.7 × 10−3) (Supplementary Tables S18). In SVD subtype, we identified 121 MVPs suggestive of association (Supplemental Table S15). Enrichment analysis was significant for different traits associated with lipid metabolism (FDR p-values = 0.013495) and nominally significant for adipogenous hallmark genes (p-value = 0.0024) (Supplemental Table S19). In UND subtype, we identified 294 MVPs (Supplemental Table S16). Enrichment analysis was significant for traits associated with inflammation (FDR p-values = 0.0027) and cellular processes (Supplementary Tables S20).
The traits and diseases associated with loci that contain the suggestive CpGs associated with IS subtypes are shown in Supplementary Tables S20-S24. Approximately 30% of these MVPs overlap with known loci having genetic variants associated with vascular risk factors ().
Figure 4. Summary of loci harbouring nominally significant CpGs, by TOAST, associated with phenotypes and diseases in genome-wide association studies. One locus can be associated with more than one trait. LAA, large-artery atherosclerosis: CE, cardiembolic; SVD, small vessel diseases; UND, undetermined IS subtypes; BMI, body mass index; BP, blood pressure; DM, diabetes mellitus; CHD, coronary heart disease.
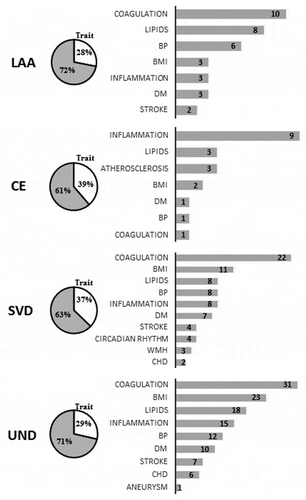
Discussion
Novel findings in the present study include an association between IS and differentially methylated positions of 22 CpGs located in 21 loci (CAMSAP3, SLC35E1, ZFHX3, PIM3, MAPK1, LRRC26, HIF1A, RNF126, SENP3, ANAPC11, PLBD2, CCNL2, PUM1, ITPKB, NAPA, IL15RA, ACSL1, JMY, PUF60, CHSY1, BAMBI). These CpGs are more methylated in IS than in control samples, except the one in the locus MAPK1. Two of these CpGs, cg00614832 and cg07786668, are situated in the ZFHX3 locus, a known stroke gene [Citation2]. Pathway analysis indicated that these 21 loci are involved in inflammation and angiogenesis pathways. Additionally, they share pathways, protein domains, genetic interaction, and co-expression with stroke-associated genes identified in previous GWAS studies [Citation2].
The repercussion of these findings in gene expression has not been studied; however, the 22 validated CpGs are located in CpG island-promoters, 20 of them in enhancer elements, and 21 in a DNase I hypersensitive site, functionally related to transcriptional activity; 12 are associated to H3K27AC, indicating active gene expression. All together, these results suggest a possible effect on gene expression.
The meta-analysis identified 384 MVPs significantly associated with IS, but replication is needed to confirm these results because of possible inflation of p-values. These MVPs were enriched for genes involved in adipogenesis, triglyceride and lipid metabolism, circadian clock, and regulation of glycolysis pathway. We also identified shared MVP associations with five genes (SH2B3, ZFHX3, WNK1 (beside NINJ2), PCIF1, TRIM47, YAP1) previously associated to stroke, to vascular risk factors and to traits such as diabetes mellitus, coagulation, blood pressure, aortic aneurysm, body mass index, white-matter hyperintensity, and coronary heart disease [Citation2,Citation14–Citation20].
The overlap between loci containing SNPs associated with vascular risk factors and those related to stroke highlights the importance of epigenetics in identifying possible new biomarkers of stroke risk or/and new causal variants related to stroke. The question of potential independent effects of MVPs and SNPs remains to be studied. Most of the stroke risk SNPs identified are located in intergenic or intronic regions, and MVPs that were differentially methylated in the meta-analysis are located in the promoter region, making them better candidates to be the causal variant [Citation2].
The stratification analysis by stroke subtypes revealed distinct methylation patterns that could provide further mechanistic insights; however, replication is needed. The LAA subtype showed enrichment for genes involved in plasma HDL-C levels and adipogenesis pathway which correlates to LAA pathophysiology. Its top MVP, in the GPS2 locus, has recently been associated with obesity and diabetes mellitus, with the authors suggesting that this gene may contribute to atherogenesis [Citation21]. Additionally, cg17218495 is in the SMARCA4 locus, previously associated with stroke and lipid metabolism) [Citation22,Citation23]. The CE analysis was enriched by genes involved in the insulin signalling pathway and adipogenous hallmark genes, while SVD analysis was enriched by genes involved in lipid metabolisms. Moreover, two MVPs were located in CNNM2, a known variant associated with blood pressure, and TRIM47, associated with white-matter hyperintensities which correlate to SVD pathophysiology [Citation19,Citation24].
DNAm can respond to changes in the environment and might mediate gene expression and phenotypes induced by prior exposure, such as glycaemic exposure, a phenomenon called metabolic memory [Citation25–Citation27]. Based on those studies, DNAm may be one of the mechanisms involved in the metabolic memory. We only reported an association with IS traits, but cannot infer causality of these methylation changes; however, these MVPs could be causal variants, biomarkers of exposure, or stochastic changes. Further investigation is needed.
In this study, we analysed one of the largest available series of IS patients with genome-wide methylation data. We replicated our results in two independent samples. Moreover, we identified 22 CpG sites related to IS, two of them located in the ZFHX3 locus, harbouring known genetic variants associated with stroke. Stratification by stroke subtype revealed distinct methylation patterns associated with different vascular risk factors; however, these results must be replicated.
EWAS are prone to significant inflation and bias of test statistics. Neither GWAS-based methodology nor confounder adjustment methods completely remove bias and inflation [Citation28]. Epigenome data differ from genetic data: they are quantitative measures (whereas genotypes are discrete) that are subject to major confounding effects of batch analysis, whether technical, such as DNA extraction protocols; biological, including cellular heterogeneity; or epigenetic changes caused by the disease process itself [Citation28–Citation31]. In our study, blood samples were collected in the acute phase of IS; although we adjusted for stroke severity using the NIH scale in an effort to correct the residual confounding, the inflation is evident. Nonetheless, some signals were identified and others require replication.
Some limitations of the study should be considered. First, we measured DNAm in peripheral blood cells. Some authors have suggested that the methylation levels of some CpGs/regions are tissue-specific [Citation32], and we might have lost some signals by not choosing specific tissues that could have a higher impact in DNAm. However, other authors consider methylation patterns of whole blood a good proxy for the methylation levels from a specific site of action. Second, the cross-sectional study design precluded any inference of causality in the reported association between IS and DNAm levels. Third, there is a lack of replication in the meta-analysis. Fourth, we cannot draw conclusions about the effect of these methylation changes in gene expression because we lack gene expression data.
DNAm changes in 22 CpGs play a role in IS pathophysiology. They may orchestrate gene expression that contributes to IS and/or offer a biomarker of IS and vascular risk factors. Further investigation is required to assess the functional effect of DNAm changes and establish causality.
Supplemental Material
Download MS Excel (870.9 KB)Supplemental Material
Download PDF (1.3 MB)Acknowledgments
We are grateful to all neurologists, nurses, and residents from the stroke unit and to technical staff from the IMIM who helped in performing this study. Elaine M. Lilly, PhD, provided English language assistance.
Disclosure statement
No potential conflict of interest was reported by the authors.
Supplementary material
Supplemental data for this article can be accessed here.
Additional information
Funding
References
- WHO. Global status report on noncommunicable diseases 2014. [cited 2015 Dec 2]. Available from: http://www.who.int/nmh/publications/ncd-status-report-2014/en/
- Malik R, Chauhan G, Traylor M, et al. Multiancestry genome-wide association study of 520,000 subjects identifies 32 loci associated with stroke and stroke subtypes. Nat Genet. 2018;50:524–537.
- Bevan S, Traylor M, Adib-Samii P, et al. Genetic heritability of ischemic stroke and the contribution of previously reported candidate gene and genomewide associations. Stroke. 2012;43:3161–3167.
- Dichgans M, Pulit SL, Rosand J. Stroke genetics: discovery, biology, and clinical applications. Lancet Neurol. 2019;18:587–599.
- Malik R, Dau T, Gonik M, et al. Common coding variant in SERPINA1 increases the risk for large artery stroke. Proc Natl Acad Sci U S A. 2017;114:3613–3618.
- Nikpay M, Goel A, Won -H-H, et al. A comprehensive 1000 Genomes–based genome-wide association meta-analysis of coronary artery disease. Nat Genet. 2015;47:1121–1130.
- Holliday EG, Maguire JM, Evans T-J, et al. Common variants at 6p21.1 are associated with large artery atherosclerotic stroke. Nat Genet. 2012;44:1147–1151.
- Kilarski LL, Achterberg S, Devan WJ, et al. Meta-analysis in more than 17,900 cases of ischemic stroke reveals a novel association at 12q24.12. Neurology. 2014;83:678–685.
- Portela A, Esteller M. Epigenetic modifications and human disease. Nat Biotechnol. 2010;28(10):1057–1068.
- Soriano-Tárraga C, Jiménez-Conde J, Giralt-Steinhauer E, et al. Global DNA methylation of ischemic stroke subtypes. PLoS ONE. 2014;9(4):e96543.
- Soriano-Tárraga C, Giralt-Steinhauer E, Mola-Caminal M, et al. Ischemic stroke patients are biologically older than their chronological age. Aging (Albany NY). 2016;8:2655–2666.
- Soriano-Tárraga C, Mola-Caminal M, Giralt-Steinhauer E, et al. Biological age is better than chronological as predictor of 3-month outcome in ischemic stroke. Neurology. 2017;89:830–836.
- Soriano-Tárraga C, Giralt-Steinhauer E, Mola-Caminal M, et al. Biological Age is a predictor of mortality in Ischemic Stroke. Sci Rep. 2018;8. DOI:10.1038/s41598-018-22579-0
- NINDS Stroke Genetics Network (Sign) SL, International Stroke Genetics Consortium (ISGC) PF, Wong Q, et al. Loci associated with ischaemic stroke and its subtypes (SiGN): a genome-wide association study. Lancet Neurol. 2016;15:174–184.
- Ikram MA, Seshadri S, Bis JC, et al. Genomewide association studies of stroke. N Engl J Med. 2009;360:1718–1728.
- Jones GT, Tromp G, Kuivaniemi H, et al. Meta-analysis of genome-wide association studies for abdominal aortic aneurysm identifies four new disease-specific risk loci. Circ Res. 2017;120:341–353.
- Evangelou E, Warren HR, Mosen-Ansorena D, et al. Genetic analysis of over 1 million people identifies 535 new loci associated with blood pressure traits. Nat Genet. 2018;50:1412–1425.
- Dupuis J, Langenberg C, Prokopenko I, et al. New genetic loci implicated in fasting glucose homeostasis and their impact on type 2 diabetes risk. Nat Genet. 2010;42:105–116.
- Verhaaren BFJ, Debette S, Bis JC, et al. Multiethnic genome-wide association study of cerebral white matter hyperintensities on MRI. Circulation. 2015;8:398–409.
- Jian X, Satizabal CL, Smith AV, et al. Exome chip analysis identifies low-frequency and rare variants in MRPL38 for white matter hyperintensities on brain magnetic resonance imaging. Stroke. 2018;49:1812–1819.
- Drareni K, Ballaire R, Barilla S, et al. GPS2 deficiency triggers maladaptive white adipose tissue expansion in obesity via HIF1A activation. Cell Rep. 2018;24:2957–2971.e6.
- Surakka I, Horikoshi M, Mägi R, et al. The impact of low-frequency and rare variants on lipid levels. Nat Genet. 2015;47:589–597.
- Dichgans M, Malik R, König IR, et al. Shared genetic susceptibility to ischemic stroke and coronary artery disease: a genome-wide analysis of common variants. Stroke. 2014;45:24–36.
- Ehret GB, Munroe PB, Rice KM, et al. Genetic variants in novel pathways influence blood pressure and cardiovascular disease risk. Nature. 2011;478:103–109.
- Soriano-Tárraga C, Jiménez-Conde J, Giralt-Steinhauer E, et al. Epigenome-wide association study identifies TXNIP gene associated with type 2 diabetes mellitus and sustained hyperglycemia. Hum Mol Genet. 2016;25:609–619.
- Ceriello A. The emerging challenge in diabetes: the “metabolic memory”. Vascul Pharmacol. 2012;57:133–138.
- Waki H, Yamauchi T, Kadowaki T. The epigenome and its role in diabetes. Curr Diab Rep. 2012;12:673–685.
- van Iterson M, van Zwet EW, Heijmans BT, et al. Controlling bias and inflation in epigenome- and transcriptome-wide association studies using the empirical null distribution. Genome Biol. 2017;18. DOI:10.1186/s13059-016-1131-9
- Relton CL, Davey Smith G. Two-step epigenetic Mendelian randomization: a strategy for establishing the causal role of epigenetic processes in pathways to disease. Int J Epidemiol. 2012;41(1):161–176.
- Soriano-Tárraga C, Jiménez-Conde J, Giralt-Steinhauer E, et al. DNA isolation method is a source of global DNA methylation variability measured with LUMA. Experimental analysis and a systematic review. PLoS ONE. 2013;8. DOI:10.1371/journal.pone.0060750
- Leek JT, Scharpf RB, Bravo HC, et al. Tackling the widespread and critical impact of batch effects in high-throughput data. Nat Rev Genet. 2010;11:733–739.
- Lowe R, Slodkowicz G, Goldman N, et al. The human blood DNA methylome displays a highly distinctive profile compared with other somatic tissues. Epigenetics. 2015;10:274–281.