ABSTRACT
Temperature is a key environmental parameter affecting both the phenotypes and distributions of organisms, particularly ectotherms. Rapid organismal responses to thermal environmental changes have been described for several ectotherms; however, the underlying molecular mechanisms often remain unclear. Here, we studied whole genome cytosine methylation patterns of European grayling (Thymallus thymallus) embryos from five populations with contemporary adaptations of early life history traits at either ‘colder’ or ‘warmer’ spawning grounds. We reared fish embryos in a common garden experiment using two temperatures that resembled the ‘colder’ and ‘warmer’ conditions of the natal natural environments. Genome-wide methylation patterns were similar in populations originating from colder thermal origin subpopulations, whereas single nucleotide polymorphisms uncovered from the same data identified strong population structure among isolated populations, but limited structure among interconnected populations. This was surprising because the previously studied gene expression response among populations was mostly plastic, and mainly influenced by the developmental temperature. These findings support the hypothesis of the magnified role of epigenetic mechanisms in modulating plasticity. The abundance of consistently changing methylation loci between two warmer-to-colder thermal origin population pairs suggests that local adaptation has shaped the observed methylation patterns. The dynamic nature of the methylomes was further highlighted by genome-wide and site-specific plastic responses. Our findings support both the presence of a plastic response in a subset of CpG loci, and the evolutionary role of methylation divergence between populations adapting to contrasting thermal environments.
Introduction
Adaptation to changing environments is a fundamental process for the survival of populations and species, especially during fast-paced environmental changes. Such rapid changes are a predicted consequence of the global warming, which may cause large-scale changes in the environments of natural populations in the near future [Citation1]. Rapid phenotypic responses to climate change have been reported in several studies [Citation2–4]. However, it remains unclear whether such rapid responses are a result of natural selection on the standing genetic variation within populations resulting in genetic adaptation [Citation5] and, if so, whether the pace and strength of such microevolution are sufficient to counteract global warming [Citation6,Citation7].
Phenotypic plasticity, the phenomenon of a genotype producing different phenotypes in response to different environmental conditions [Citation8], is an alternative mechanism for responding to environmental changes. Plasticity may buy time for populations in the initial stages of adaptation, essential during, e.g., climate change and other very intense phenomena such as the colonization of novel environments, or following the introduction of new predators [Citation5,Citation9,Citation10]. Plasticity may be favourable especially in situations when the environment is temporally heterogeneous, and when there are reliable environmental cues to predict future environmental changes [Citation11,Citation12]. Examples of the interplay between genetic adaptation and plasticity leading to climate change responses are currently limited, and the need to further study these responses has been highlighted [Citation5,Citation9,Citation10].
Within the lifespan of an individual, phenotypic variability is modulated by non-genetic mechanisms rather than by genetic mutations. Thus, epigenetic mechanisms may be important for modulating plasticity by playing a role as an interface between the genome and environment [Citation13]. Theoretical and modelling approaches show that, over relatively short ecological time scales, epigenetic modifications can contribute to the persistence of populations by increasing plasticity [Citation12,Citation14]. Over longer evolutionary time scales, such modifications are predicted to have permanent evolutionary effects, altering the pace and outcome of the adaptation process [Citation12,Citation14,Citation15]. For instance, epigenetic modifications may slow down adaptation due to their instability, decrease the final fitness outcome by decreasing the strength of natural selection, aid genetic adaptation by assimilation or facilitate the whole adaptation process by allowing the non-adapted populations to initially persist [Citation12,Citation14,Citation15]. Epigenetic markers, including various types of functional groups that can be added to the DNA molecule or the associated histones, are a relatively dynamic group of DNA modifications with frequently reversible states in comparison to the more stable nucleotide sequence polymorphisms. The attachment of a methyl group to a cytosine nucleotide in the DNA, referred to as cytosine methylation [Citation16], is an evolutionarily ancient, conserved, and abundant epigenetic mechanism. In most vertebrates including teleost fishes, cytosine methylation predominantly occurs in the CpG sequence context (sequences in a genome containing cytosine followed by guanine) [Citation17], where the methylation machinery typically maintains methylation as the default state, particularly during the embryonic and early life stages [Citation16,Citation18]. In upstream regulatory regions of genes, CpG methylation levels may play transcriptionally instructive roles, particularly in CpG-rich promoters with CpG islands [Citation16,Citation19]. In gene bodies, CpG methylation has been suggested to regulate the alternative splicing machinery between tissues, prevent spurious transcription initiation or protect chromatin structure from RNA polymerase during gene expression [Citation16,Citation19,Citation20]. Epigenetic regulation may be important during development and the early life of individuals [Citation21]. For example, a link between globally increased cytosine methylation in response to changes in environmental temperature in early life stages has been observed in multiple teleost fishes, such as the threespine stickleback (Gasterosteus aculeatus) and Atlantic cod (Gadus morhua) [Citation22,Citation23]. More targeted changes have been reported, including methylation and gene expression alterations in specific genes such as myogenin, encoding a major muscle protein, in the larvae of Senegalese sole (Solea senegalensis) and Atlantic salmon (Salmo salar) [Citation24,Citation25], and dnmt genes, that regulate the overall methylation levels, in Atlantic cod [Citation23]. Such epigenetic responses to internal or external stimuli may serve as underlying mechanism of developmental plasticity.
European grayling (Thymallus thymallus) provides a good model system for studying the early stages of ongoing local adaptation. European grayling (hereafter referred to as ‘grayling’) is a salmonid fish that is commonly found in freshwater habitats across a large part of Europe. The species inhabits fragmented and heterogeneous freshwater environments [Citation26]. Such spatially and temporally variable freshwater habitats predict a potential role of environmental plasticity in adaptive processes, especially in species with relatively long life span, which can exacerbate the pressures caused by climate change in grayling. Further, the spawning and the subsequent embryonic development of grayling takes place in the early summer, when the water temperature is considerably more variable than during the spawning and developmental season of many other salmonids, which often spawn during the autumn. However, the level of genetic variation within grayling populations has been shown to be low, which may restrict the capacity for genetic adaptation [Citation27]. Our study system consists of multiple recently founded populations in Norway [Citation28]. The populations are closely located both geographically and genetically, but they experience systematic differences in the water temperature both during spawning and larval development [Citation29,Citation30]. Previous studies have provided indications of multiple rapidly evolved phenotypic traits in these grayling populations under circumstances that are expected to hinder adaptation, such as the relatively short adaptation period since population foundation and the limited genetic diversity [Citation29,Citation31]. Differences between populations have been reported in traits such as embryonic development time, larval survival and growth rate [Citation29,Citation32]. Some traits seem to have evolved in a parallel fashion among populations experiencing similar spawning temperatures, suggesting that adaptive evolution, rather than neutral genetic drift, is the main driving force for these changes [Citation27,Citation33]. For example, increased growth rate of muscle mass combined with delayed skeletal development in populations spawning in relatively colder water may posit an adaptive trade-off to maximize larval body mass, which is a key factor affecting later over-winter survival in colder-watered environment [Citation33]. However, plasticity explains much of the observed embryonic gene expression patterns among populations and may thus have an important role affecting the adaptation process [Citation30].
Research on adaptive responses to changing environmental temperatures at different levels of molecular variation is still scarce, particularly for organisms with relatively long generation times. In the grayling system specifically, despite considerable previous research, the potential role of epigenetics remains unstudied. Here, we first describe the genome-wide embryonic methylation variation in grayling and hypothesize that during the short adaptation time period to changes in environmental temperatures, the role of epigenetic mechanisms is magnified, and thus displaying more molecular variation, in comparison to the role of genetic mechanisms. We expect this magnification to be detectable for previously reported divergent phenotypes either between populations from different thermal origins (evolutionary change) or between different developmental temperatures (plastic response). We test this hypothesis by first identifying patterns in the genome-wide methylation variation within and between populations with potential relevance for thermal adaptation. We compare the evolutionary and plastic components shaping methylation level variation to the underlying single nucleotide polymorphisms (SNPs) and resulting transcription levels. We then assess the effect of karyotype and sequence functionality on the methylation patterns. Finally, we quantify the site-specific methylation plasticity and report on candidate genes that may be under epigenetic developmental regulation and, thus, contribute to the phenotypic plastic response to developmental temperature variation.
Materials and methods
Grayling samples
We sampled five grayling populations in the study system with variable water temperatures during spawning and the early development period (Supplementary Figure 1). The ancestral population (sampled at Otta in River Gudbrandsdalslågen, downstream from Lesjaskogsvatnet) is isolated from the other populations by a partly impassable waterfall for an unknown number of generations. The other four of these populations share a common ancestor that inhabited River Gudbrandsdalslågen in the 1880s, approximately 22 grayling generations before sampling [Citation26]. Since then, human activities that are traceable from historical records [Citation26,Citation33] have led to the sequential colonization of several nearby lakes and streams (referred to subsequently as ‘divergence order’; ). The typical spawning temperatures in River Gudbrandsdalslågen at Otta (hereafter Otta), as well as River Steinbekken, flowing into Lake Lesjaskogsvatnet, and Lake Hårrtjønn, can be described as relatively warmer in comparison to the colder conditions of the spawning populations in Rivers Valåe and Kvita, flowing into Lake Lesjaskogsvatnet and Lake Aursjøen, respectively [Citation29,Citation30], with the average difference between warmer and colder conditions estimated at 3.7°C in 2013 (Supplementary Figure 1, Supplementary Figure 2). The populations spawn in relatively colder and warmer waters, i.e., their ‘thermal origin’, herein referred to as the colder- and warmer-origin populations, respectively. Details of the common garden experiment are outlined in [Citation30] and summarized in and in Supplementary Table 1. Briefly, mature fish were collected from each of the five sampling locations during the spawning period in spring 2013. Eggs and sperm were extracted under anaesthesia at the natural sampling locations, stored on ice and transported to the experimental facility located at the University of Oslo. For each population, a mixture of eggs from four to five females was pooled and fertilized with a pool of sperm from four to six males from the corresponding population. Eggs were reared at mean developmental temperatures of 7.0°C and 10.2°C, a range similar to the natural variation during early development of the grayling in the water system. At the average predicted age of 205 degree days after fertilization, matching the eyed-egg embryonic stage, embryos from each population were sampled. We sampled pre-hatching embryos because by then, a typical teleost embryo has established sperm-like methylation blueprint and the tissue-specific methylation patterns have already differentiated, while the young age still minimizes the noise caused by further methylome modifications in response to time and internal or external stimuli [Citation34,Citation35]. The samples were immediately frozen on dry ice and stored at -80°C until DNA extraction for individual sequencing of four or six embryos from each population, including two to three individuals per population reared at both warmer and colder developmental temperatures.
Figure 1. Schematic summary of the experimental design used in the study. Spawning adults were collected from the wild, and gametes stripped, and fertilizations conducted for pools of males and females from each study population. Then, the embryos were reared in a common-garden environment until sampling during the eyed-egg stage
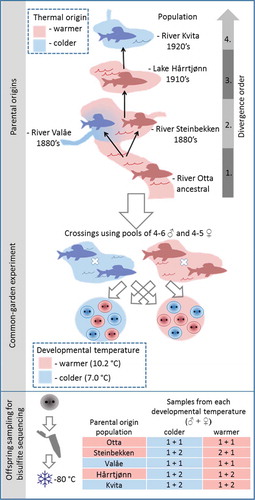
Methylation dataset
Altogether, 26 embryos were processed for bisulphite sequencing. DNA from each embryo was extracted using a salt extraction protocol [Citation36]. Sample concentrations were measured using Qubit Fluorometric Quantitation (Life Technologies) and quality controlled before and after library preparation using Advanced Analytical Fragment Analyser. The ordering of the samples was randomized to avoid lane effects. Library preparation protocol was adapted from [Citation37] for samples diluted to contain 1,000 ng of genomic DNA at The Finnish Functional Genomics Centre. During the library preparation, genomic DNA was first fragmented with Covaris focused-ultrasonicator using target peak size 200 of base pairs, purified and size-selected (100–600 base pairs) with AMPure magnetic beads. Then, the adapter ligation step included poly(A) tail repair using End-It DNA end repair kit (Epicentre) and Klenow fragment (3ʹ-5ʹ exo), a second round of purification and size selection (>100 base pair) of the DNA with AMPure magnetic beads, and the ligation of unique Illumina TruSeq indexing adapter (1:10 dilution) for each sample. After two rounds of bead SPRI clean-ups, Invitrogen MethylCode Bisulphite Conversion Kit was used to convert unmethylated cytosines in the DNA fragments to uracils. Six cycles of PCR were performed with KAPA HiFi Uracil+ Polymerase and the final libraries were extracted using SPRI bead clean-up. Finally, the samples were pooled and sequenced using the Illumina HiSeq3000 platform and TruSeq v3 chemistry to produce 75 base paired-end reads at the average estimated amount of 21.3 (19.4–24.5) gigabase pairs of sequence for each sample, resulting in the average of 12.3x per-sample coverage (10.7x-13.4x) in the genome of the estimated size of 1.5 gigabase pairs (Supplementary Table 1) [Citation38].
The sequenced reads were quality trimmed using ConDeTri software [Citation39] with a minimum trimmed read length of 30 base pairs, followed by reference-based assembly of the reads against the recently published chromosome-level genome assembly [Citation38] with Bismark bisulphite mapper v. 0.16.1 [Citation40]. Following assembly, CpG methylation information was collected for each sample using the bismark_methylation_extractor script included in the Bismark package in paired-end mode and filtered so that each CpG locus used in the subsequent analysis had information from at least 16 samples with 8–30 read coverage after combining the methylation levels from each strand of the symmetrical CpG sites. The sex of each sampled individual was determined by extracting the read coverage in a region including the sexually dimorphic Y-chromosome gene and 100,000 base pair flanking sequences with BEDTools coverageBed v. 2.26.0 [Citation38,Citation41]. Individuals without coverage at the sdY locus were assumed to be females.
Messenger RNA dataset
We utilized previously sequenced mRNA reads (NCBI BioProject PRJNA419685) originating from the same common garden experiment [Citation30], which included 34 embryos from four of the five study populations used here (excluding the Steinbekken population) that had been raised at similar warmer and colder developmental temperatures. The mRNA samples had been collected at 140 degree days post fertilization and sequenced using the Illumina HiSeq 2000 platform with 100 base paired-end reads, resulting in an average of 78.7 million read pairs per sample. We complemented the previously reported de novo assembly of the mRNA reads [Citation30] with a reference-based assembly against the genome sequence [Citation38] using TopHat assembler v. 2.1.1 [Citation42], followed by quantification of transcription levels using HTSEQ-count v. 0.9.0 [Citation43]. The transcription levels were normalized using the remove unwanted variation (RUV) method RUVr [Citation44] implemented in the R package RUVseq v. 1.16.0 [Citation44], which uses residuals from a generalized linear regression model of counts taking into account the covariates of interest, which were the population of origin, resembling evolutionary natal temperature, and experimental developmental temperature in this case.
Single nucleotide polymorphism dataset
We identified SNPs in the methylation sequence assembly using BS-SNPer [Citation45] that excludes the SNPs resulting from the underlying methylation differences [Citation46]. The SNP filtering steps excluded triallelic loci, polymorphisms in only one sample, loci where the methylation-corrected BS-SNPer genotypes disagreed with those extracted using the regular SNP calling pipeline, and C/T polymorphisms. We then re-extracted the genotypes with the regular SAMtools SNP calling procedure including the commands mpileup and bcftools call to verify the homozygous genotypes that could be called but were not extracted during the BS-SNPer analysis. Finally, we excluded the cytosine loci at which nucleotide polymorphism was detected from further methylation analysis.
Annotating the CpG loci
To categorize the CpG loci based on functional genomic regions, including promoter, 5’UTR, coding and 3’UTR sequences, we overlapped the CG dinucleotide positions in the genome assembly [Citation38] with the associated gene predictions. For simplicity, we allowed each CpG locus to have one grayling transcript annotation for each functional region type. For example, we allowed only one promoter annotation for each CpG locus, but simultaneously the locus could have one 3’UTR annotation. Promoter intervals were determined as the 500 base pair flanking sequences upstream from each annotated mRNA region. We used this relatively short interval to reduce the possibility of misannotations to unrelated genes. We also predicted the locations of CpG islands with cpgplot implemented in the EMBOSS package (v. 6.5.7.0) with a window size of 200. Finally, we defined genomic intervals outside functional genomic regions as intergenic.
Genome-wide methylation variation in comparison to nucleotide and gene transcription variation
To investigate the molecular variation between individuals without any prior assumptions about the effects of the variables, we performed principal component analysis of the methylation level estimates, SNPs and z-score normalized gene transcription levels including observations without any missing data. To compare the relevant patterns in the molecular variation between populations, we calculated the mean pairwise Euclidean distances between populations along the two first principal components of each level of molecular variation. To further explore the contributions of multiple explanatory variables at the different levels of molecular variation, we performed distance-based redundancy analysis [Citation47] of the pairwise Euclidean distances between individuals. This nonparametric method is tolerant of zero-inflated datasets, which is often the case in methylation data. The following explanatory variables were included: (1) Divergence order was used to describe the effect of neutral evolutionary processes, such as genetic drift, that would separate the most distantly related populations most strongly from the common ancestor. Divergence order was assigned for each sample based on the historical records of the colonization times of each water region (, Supplementary Figure 1). It was described using a rank scale ranging from the ancestral population with rank one, and populations inhabiting Lesjaskogsvatnet with rank two, to Lake Hårrtjønn and River Kvita populations with ranks three and four, respectively; (2) thermal origin was assumed to originate from non-neutral selection processes that would result in parallel evolution of the populations inhabiting environments with similar developmental temperatures; (3) experimental developmental temperature and (4) sex of each embryo. We repeated the analysis for the methylation, SNP, and normalized transcription dataset. The significance of the explanatory variables was verified using ANOVA-like permutation tests. R functions dist, dbrda, and anova.cca in the stats v. 3.4.0 and vegan v. 2.4.6 packages were used in the analysis.
To quantify the changes in the overall chromosomal methylation levels linking to several evolutionary, plastic and chromosomal architecture variables, we calculated chromosome-specific mean methylation levels for each individual and used them as the dependent variable in a linear mixed-effects model per-chromosome methylation mean ~ developmental temperature + sex + (1 | population) + (1 | chromosome) + (1 | homeolog) + (population | developmental temperature), where individual developmental temperature and sex were used as independent fixed effect variables, supplemented with random intercepts for the five categories of sampling populations, chromosome identities and ancestral identities of homeologous chromosome pairs originating from the salmonid-specific whole-genome duplication event 80–100 million years ago [Citation48-49]. The model was implemented with the lmer function of the lmerTest package v. 3.1.0 in R. We also estimated random slopes for population-by-developmental temperatures (°C). The significance of random terms was estimated by likelihood ratio tests between models with and without random terms fitted under residual maximum likelihood. The observed differences between population-temperature combinations were further studied with pairwise t-tests of per-chromosome estimates for the population-temperature groups of individuals after removing the per-chromosome variation by taking residuals from a linear model that fitted methylation means for each chromosome identity.
Site-specific analysis to detect developmental plasticity and differentiation between populations
We compared the abundance of the CpG loci where the methylation levels changed consistently according to the thermal origin when a warmer-origin population colonized a colder environment to the abundance of the inconsistently changed CpG loci. The methylation level changes were detected between the two neighbouring (based on divergence order) warmer-to-colder-origin population pairs (Otta-Valåe and Hårrtjønn-Kvita) inhabiting separate water regions. We counted the number of CpG loci where the mean methylation response was estimated to increase or decrease consistently by at least 50% in the two warmer-to-colder-origin population pairs. The number of consistently changed loci was then compared to the number of loci showing at least a 50% inconsistent change between the population pairs. The higher abundance of consistently than inconsistently changed loci was verified with the Chi-squared test. For comparison, we also tested for a possible enrichment of consistent plastic methylation changes of at least 50% within the two warmer- or colder-origin populations; and repeated the analysis with adding the third possible population pair from the populations inhabiting Lake Lesjaskogsvatnet.
To reveal the specific chromosomal regions with a plastic response to the developmental temperature or the sex of the embryo, we used an approach similar to an epigenome-wide association study (EWAS). We tested the effects of several variables on CpG methylation status in promoters, 5’UTR and 3’UTR sequences, and coding regions. We fitted a mixed logistic regression model (methylated read counts, unmethylated read counts) ~ 1+ temperature + sex + (1 | population) + (population | temperature) where, like above, we included fixed effects of temperature and sex, random intercept for the five population categories and random slopes for the population-by-developmental temperatures [Citation50]. The model was fit with a logit link function under Laplace approximation using the bobyqa optimizer implemented with the glmer function in the R package lme4 v. 4.1.1. Detecting variation for the random population term can be interpreted as the presence of differences among populations, whereas detecting variation for the population-by-developmental temperature interaction term indicates the presence of differences in how populations respond to developmental temperature, i.e., developmental plasticity. To reduce type I error caused by overdispersion, we estimated the dispersion factors for each model by dividing the estimated sum of the squared Pearson residuals with the residual degrees of freedom and added observation-level random factors for models with a dispersion factor >1 [Citation51]. Like above, we also estimated the significances of random variables using likelihood ratio tests and included random terms only if significantly improving the model (P < 0.1 for the population term and P < 0.05 for the population-by-developmental temperature interaction term) [Citation52]. If neither of the random terms was significant, we used a logistic regression model without random terms, implemented with the glm function of the R stats package. Finally, to link the underlying nucleotide sequence properties (upstream CpG richness) to the site-specific developmental plasticity, we compared the observed mean CpG abundance in the upstream regulatory sequences associated with temperature-plastic CpG loci to the distribution of the corresponding upstream CpG abundancies associated with random upstream regulatory sequences based on one hundred permutations.
Describing methylation patterns in functional regions
We described the abundancies of low- and high-methylated loci in different functional genomic regions by calculating the overall methylation state of each CpG locus as completely unmethylated (0% methylated), hypomethylated (<20% methylated), intermediately methylated (≥20% and ≤80% methylated) or hypermethylated (>80% methylated) based on the mean methylation levels across all samples, and compared the frequencies of loci with different methylation states between functional genomic regions. We visualized the distributions with kernel density estimates obtained from the density function in R stats package v. 3.5.2 using Gaussian kernel smoothing function.
Gene list analyses
In order to annotate the grayling transcripts, we associated them with well-annotated genes of the model species, zebrafish (Danio rerio). We matched the predicted grayling proteins to the best matching zebrafish proteins (v. GRCz11) from the Ensembl database [Citation53] with Blastp+ v. 2.6.0 [Citation54], resulting in zebrafish matches with an e-value <0.0001 and score >45.8.
To study the typical functions of the genes with consistently hypo- or hypermethylated upstream regulatory sequences across all samples and low or high CpG content, we generated four subsets of zebrafish orthologous genes with CpG-poor or CpG-rich upstream regulatory regions (including promoters and 5’UTR regions) and a hypo- or hypermethylated methylation status. The median number of upstream CpG loci associated with each zebrafish orthologue was used as a threshold for defining the CpG abundance category. To include equally sized groups of orthologous genes with a low or high methylation status observed repeatedly (here, in five CpG loci), we selected genes for which all of the analysed CpG loci were hypomethylated (excluding intermediately methylated loci) and equal number of the genes with the largest proportions of hypermethylated loci. We compared the four test categories against a background list including the combination of all four gene lists.
Gene ontology enrichments for genes with a plastic response detected in the site-specific analysis were identified for the temperature- and sex-sensitive grayling transcripts for which multiple significantly plastic (FDR < 0.05) CpG loci were detected. To test for genotype-by-environment interaction, we used the genes associated with multiple CpG loci and best fit using models including the population-by-temperature interaction. All genes associated with multiple CpG loci included in the site-specific analysis were used as the background in the gene list analyses.
Each gene list comparison was performed with standard hypergeometric models implemented in the gene ontology enrichment analysis and visualization tool [Citation55] with the database version updated 29 June 2019.
Data availability
The bisulphite sequencing reads were deposited at NCBI SRA under BioProject ID PRJNA588748.
Results
A total of 9,663,307 variable and 290,705 completely unmethylated CpG loci remained in the analysis after the exclusion of loci exhibiting low sample coverage or potential nucleotide variation. Of those, 207,380 loci were located in promoter sequences, 87,283 in 5’UTRs, 604,596 in coding sequences, 20,158 in 3’UTRs, 639,631 in CpG islands and 8,440,454 were intergenic. The estimated overall mean methylation level was 76.8%, including 8.2% hypomethylated and 72.1% hypermethylated loci. 3,465,289 loci did not contain any missing observations. Similarly, the final SNP dataset consisted of 78,012 complete observations. The transcription levels of 22,526 mRNA transcripts were included in the mRNA data set.
Genome-wide methylation variation in comparison to SNP and transcription variation
Based on the methylation dataset, the average Euclidean distance between the individuals from the colder-origin populations along the two most important principal components was smaller than the mean pairwise distances between any of the other populations, indicating that colder-origin population individuals have very similar genome-wide methylation profiles. A one-way multivariate analysis of variance (MANOVA) for the two first principal components verified the between-population differences from zero in the principal coordinates (Pillai’s Trace = 1.5152, F8,42 = 16.41, P < 0.0001; ), and Tukey’s post hoc tests for PC1 and PC2 detailed that the colder-origin populations were the only population pair with the difference not deviating from zero (Tukey’s adjusted P > 0.05, ). Also in the SNP dataset, individuals from the colder-origin populations clustered together more tightly along the principal components (), but the mean distance between the colder-origin individuals was not different from the pairwise distances between individuals from other populations (). Instead, based on the SNP dataset, the individuals inhabiting Rivers Valåe and Steinbekken in Lake Lesjaskogsvatnet were the only population pair without significant differentiation between the principal components, verified by MANOVA (Pillai’s Trace = 1.843, F8,42 = 61.619, P < 0.0001; ) and subsequent Tukey’s post-hoc tests for PC1 and PC2 (Tukey’s adjusted P > 0.05, ). In contrast, the principal components derived from the gene transcription estimates did not reveal such differences between pairwise population distances (, ). Based on the distance-based redundancy analysis and verified by ANOVA-like permutation tests, divergence order and thermal origin explained 4.5% and 4.3% of the variation in the methylation dataset (Supplementary Table 2, ) and, similarly, 6.1% and 5.5% in the SNP dataset (). In contrast, for the transcription dataset, 32.0% of the variation was explained by developmental temperature, along with 4.6% of the marginally significant effect (P < 0.1) of thermal origin ().
Figure 2. The two first principal components of the methylation (a), nucleotide (b) and transcription level (c) analysis, and the corresponding results from distance-based redundancy analysis (d-f), including the percentages of variation explained by the most important axes. We used four (for Otta and Valåe) or six (for Steinbekken, Hårrtjønn and Kvita) individuals, including individuals from both developmental temperatures as indicated with symbols, in analyses A, B, D and E. Similarly, we used eight (for Otta and Valåe) or nine (for Hårrtjønn and Kvita) individuals in analyses C and F. Arrows in figures D-F represent the effects of the explanatory variables with significance levels indicated as follows: ‘***’ for P < 0.001, ‘**’ for P < 0.01, ‘.’ for P < 0.1. The symbols used for developmental temperatures and populations are listed below the figure. Red and blue symbols distinguish between the warmer and colder thermal origin
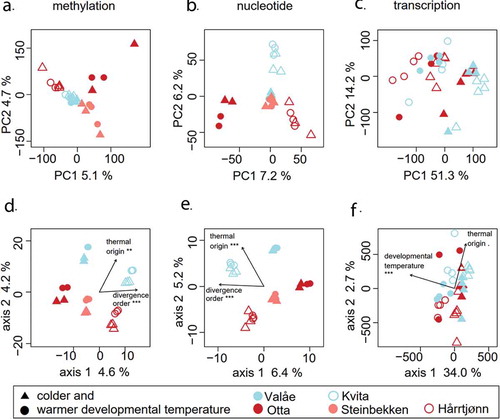
Table 1. Mean pairwise Euclidean distances between methylation, SNP, and gene expression signatures of grayling embryos, measured within (given on the diagonal) and between populations from the two most explanatory principal components of each data set. We used four (for Otta and Valåe) or six (for Steinbekken, Hårrtjønn, and Kvita) individuals, regardless of the developmental temperature, to calculate the average distances at the methylation and SNP level. Similarly, we used eight (Otta and Valåe) or nine (Hårrtjønn and Kvita) individuals to calculate the average distances at the gene transcription level. The distances between populations with similar thermal origins are marked with [Citation1] and [Citation2] for warmer and colder thermal origin, respectively, the comparisons between populations inhabiting Lake Lesjaskogsvatnet are marked with [Citation3], and the comparisons within population with [Citation4]. The between-population distances, which significantly deviate from zero along PC1 or PC2, are highlighted with bold font for Tukey’s corrected P < 0.05
To estimate genome-wide differences in the methylation levels, we chose, based on likelihood ratio tests, the model per-chromosome methylation mean ~ sex + (1 | population) + (1 | chromosome) + (1 | homeolog) + (population | temperature) (Supplementary Table 3). Further inspection of the homeologous chromosomes revealed that the chromosomal methylation levels averaged over all samples exhibited a correlation of 0.92 between the homeologous chromosome duplicates (t23 = 11.18, P < 0.0001, Supplementary Figure 3). The pairwise t-tests revealed distinct methylation levels (with P < 0.05) with an average of 0.9% absolute methylation difference found in 44 of the 45 pairwise population-specific developmental temperature comparisons (Supplementary Table 4). Among the comparisons, genome-wide hypomethylation was present at the lower developmental temperature in the Otta, Valåe, Hårrtjønn, and Kvita populations ().
Figure 3. Estimated differences in the mean methylation levels of the study populations when reared in colder- in comparison to warmer developmental temperature. We used two (for Otta and Valåe) or three (for Steinbekken, Hårrtjønn and Kvita) individuals from each developmental temperature and population to calculate the mean differences. The differences are estimates from pairwise t-tests, reported with 95% confidence intervals and the significance levels of comparisons indicated with ‘***’ (P < 0.0001)
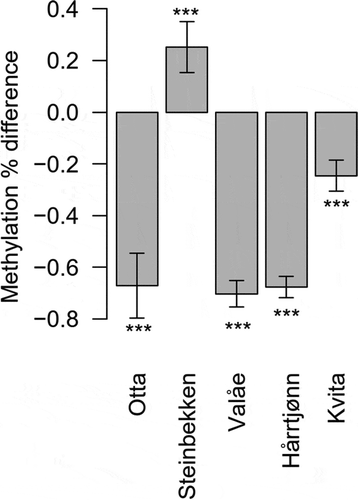
Site-specific analysis to detect plastic and evolutionary changes
We identified 1.8-fold abundance (χ21 = 82.3, P < 0.0001) in the 715 CpG loci with consistently changed methylation levels between the two population pairs including warmer and colder origin, in comparison to 408 inconsistently changed loci (). The observed consistent changes were enriched in coding sequences and 3ʹUTR sequences, and depleted from the upstream regulatory regions (χ23 = 22.4, P < 0.0001; ). When adding the third possible population pair from Lake Lesjaskogsvatnet, the results were similar (Supplementary Figure 4A). In contrast to the consistency with thermal origin at the methylation level, there was no such enrichment of the developmentally plastic changes within populations, with 212 and 183 consistently changed plastic loci being not different in abundance from the 222 and 164 loci that were inconsistently changed within the warmer or colder thermal origin populations, respectively (χ21 = 1.0, P = 0.308). Based on principal components of the three pair comparison of the consistently changed loci without missing observations, the first principal component now explained the majority (67.1%) of the variation and separated the populations by thermal origin, while the colder-origin populations remained as the most tightly clustered populations (t8 = 7.47, P < 0.0001) in comparison to the three warmer-origin populations (Supplementary Figure 4B, Supplementary Table 5). None of the loci were consistently responding to developmental temperatures within all populations. Based on separate analyses for warmer and colder thermal origin individuals, the first principal components based on consistently plastic loci within thermal origins explained 44.7% and 42.0% of the variation and separated the samples of the corresponding thermal origin by developmental temperature (Supplementary Figure 4 C-D). Interestingly, the loci identified in the colder-origin comparison also grouped the warmer-origin samples by developmental temperature and by population.
Figure 4. Consistently and inconsistently changed methylation levels in the CpG loci in two pairs of grayling populations with subsequent colonization events in the grayling study system (red = warmer-origin, blue = colder-origin populations). Of the total of 1,094 CpG loci with ≥ 50% change observed in the population means of the methylation levels, we here report the number of consistently and inconsistently changed CpG loci. The population means were calculated over four individuals from Otta and Valåe populations, each, and over six individuals from Hårrtjønn and Kvita, each, regardless of the rearing temperature. The arrow describes the relative divergence time using the colonization order of the population pairs as the unit
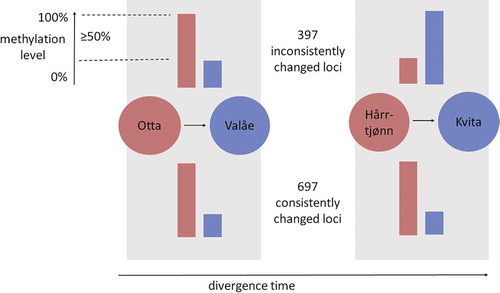
Figure 5. The observed occurrences of temperature-plastic and sex-plastic CpG loci from the EWAS-like analysis, and of the consistently changed CpG loci between populations in different functional gene regions, in comparison to the expected frequencies based on the numbers of non-plastic and inconsistently changed loci. Two (Otta and Valåe) and three (Steinbekken, Hårrtjønn and Kvita) individuals from each population and developmental temperature were used in the EWAS-like analysis. The consistent changes were based on the population means of four (Otta and Valåe) or six (Hårrtjønn and Kvita) individuals
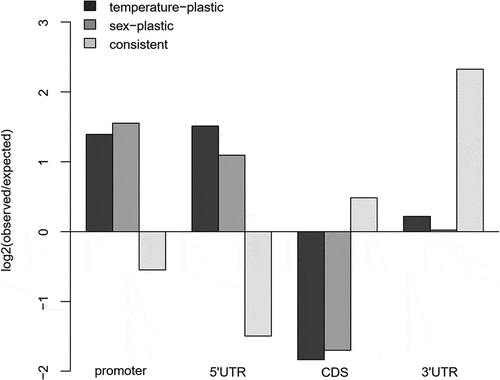
The EWAS-like site-specific analysis involved a total of 882,756 loci that were best described with models without any random effects. 21,566, 25,980 and 72 loci were best described including a random term for population, the population-by-temperature interaction, or both, respectively. Plastic loci were enriched in the upstream regulatory regions and depleted from the coding sequences (). A total of 1,806 and 2,271 loci in 1,059 and 1,393 orthologous zebrafish genes were found to be plastic between the developmental temperatures, and between sexes, respectively (Supplementary Figure 5 A and B, Supplementary Table 6). Among these, 116 loci, associated with 68 zebrafish genes, were detected as responsive for both temperature and sex. The developmental temperature-plastic CpG loci were often located in genes with CpG-poor promoters, whereby the observed mean number of 5.7 CpG loci was smaller (P < 0.001) than the mean number of 6.4 CpGs obtained from permutations of random promoters.
Describing methylation patterns in functional regions
We observed distinct patterns of CpG methylation among the functional genomic regions. In contrast to the overall state of hypermethylation in the genomes, methylation of the upstream regulatory regions exhibited a bimodal distribution, with only 43% of promoter and 39% of 5’UTR loci being hypermethylated while the abundance of hypermethylated loci in the other functional genomic regions was 72–81% (Supplementary Figure 6). Furthermore, completely unmethylated loci were concentrated in upstream regulatory regions in comparison to the corresponding abundance in other regions (χ24 = 72.2, P < 0.0001).
Gene list analyses
Among the transcripts with low CpG content in the nucleotide sequences, we found a total of eight and 15 enriched gene ontology terms among the 2,094 and 2,324 transcripts with hypo- or hypermethylated upstream regulatory regions, respectively. Among these gene ontology terms, hypermethylated upstream regions were associated with terms such as cytokine receptor activity, myosin complex and signalling functions, located in membranes, whereas hypomethylated upstream regions were associated with terms localized to the intracellular parts, including organelles such as the mitochondrion but excluding the plasma membrane. The number of enriched gene ontology terms in the transcripts with a high upstream CpG content was greater, with 68 and 58 terms being associated with the 1,709 hypo- and 1,477 hypermethylated upstream sequences (Supplementary Figure 7 A-B, Supplementary Table 7). Among these terms, upstream hypomethylation was related to the development of the central nervous system and cell fate, and to numerous terms related to the regulation of transcription and gene expression or nucleic acid binding within the nucleus. In contrast, genes with hypermethylated upstream sequences were associated with cell adhesion and signalling receptors, especially in membranes.
The CpG loci for which the methylation changes were best explained (FDR < 0.05) by the models including the population-by-temperature-interaction term were associated with genes that were enriched for nine biological processes, 33 molecular functions and one cellular component (Supplementary Figure 7 C, Supplementary Table 7), including the myosin complex, motor activity, signal sequence binding, regulation of protein depolymerization and multiple terms related to Rho GTPases. The most overrepresented term was membrane depolarization during action potential. This term was, however, non-significant after multiple testing correction (FDR = 0.234), likely because of the small category size (only eight genes, among which seven were best explained with models including the gene-by-environment interaction) (Supplementary Table 7). No gene set with significant main effect of developmental temperature or sex showed any gene ontology enrichment.
Discussion
Methylation has often been proposed as a key regulator of gene expression in vertebrates, and the addition of methyl groups in the upstream regulatory regions has been suggested to dynamically switch off gene expression [Citation16]. The global methylation signatures revealed genome-wide changes at the evolutionary time scale, which may provide potential for the evolution of mechanisms behind phenotypic response. We confirmed that the global methylation levels were dynamic in grayling during development and that temperature-responsive CpG loci were often detected in the upstream regulatory regions in the site-specific analysis. In contrast, the abundance of the loci with evolutionary signal in coding sequences and downstream regulatory regions rather than in upstream regulatory regions suggest that functionally important cytosine methylation may also be frequent outside the promoter regions. Thus, we were able to find support for both the plastic response in a subset of CpG loci, and the evolutionary role of methylation divergence between populations adapting to contrasting thermal environments.
When evaluating the patterns in the genome-wide molecular variation based on principal components and distance-based redundancy analysis axes, we found both methylation and nucleotide variation between populations affected by the divergence order and the thermal origin, confirming that both neutral evolution and local adaptation may have shaped the molecular variation. As expected, the most similar nucleotide variation was found between populations sampled from Lesjaskogsvatnet, which is explained by ongoing gene flow between these population [Citation31]. Supporting the hypothesis of the magnified role of epigenetic mechanisms in comparison to nucleotide variation at the initial stages of adaptation, we found high similarity between the colder thermal origin populations, but not between the warmer thermal origin populations. Although understanding the underlying reason behind the high similarity between populations from colder thermal origin is clearly out of the scope of this study, it is tempting to hypothesize that since the ancestral population naturally spawns in relatively warmer temperatures, beneficial genetic variation may have been more abundant among the founder individuals of the newly established warmer-origin populations, making thermal adaptation requirements less extreme. In contrast, in the absence of suitable nucleotide variation, epigenetic mechanisms altering the patterns of cytosine methylation and, possibly, other epigenetic markers such as histone modifications or microRNA dynamics may have been invoked in the founders of colder-origin populations [Citation30,Citation35]. The relatively low amount of variation explained by the first principal components in comparison to the residual variation at the nucleotide and CpG level (13.4% and 9.8%, respectively) may be explained by factors such as the heterogeneity of divergence and differential natural selection among chromosomal regions at these levels of molecular variation. Particularly at the methylation level this may mean that the methylation state may be relatively constant in any population. Also, the portion of epigenetic variation influenced by the environment or other stochastic events might be lower than the portion tightly linked to the nucleotide sequence itself because some of the CpG methylation loci are affected by nucleotide sequence variation either in cis (physically associated with the CpG locus) or in trans (located away from the CpG locus). In the most extreme sense, this may occur when obligatory methylation variation is directly determined by nucleotide polymorphisms [Citation56].
In contrast to other levels of molecular variation, we detected high plasticity and only a marginal effect of thermal origin in the global patterns of transcription variation, providing only limited evidence that populations from different thermal origins have diverged at this biological level. Favourable genetic or, particularly, epigenetic modifications may shape the gene expression response only during specific developmental time points, in specific tissues or post-transcriptionally. However, evolution may have been constrained by natural selection to produce an overall canalized response during complex developmental processes, resulting in steady transcription response between populations [Citation57]. This may also be reflected by the observation that the majority (65.5%) of the total transcription level variance could be explained with the two first principal components. As we could not assess tissue- or time point-specific responses due to our whole embryo (and thus mixed tissue) samples, further research on specific tissues or a time-series experiment might reveal more details of the transcriptional response, whether evolutionary or plastic. Whole-embryo analyses also place limitations on interpreting methylation data. Therefore, alternative approaches were not feasible due to the small size of the embryos. However, sampling the methylomes just after the environmentally sensitive period of early development may have compensating benefits. Early sampling reduces the amount of noise in the information content of the methylation levels, which would otherwise have accumulated with age and environmental exposure. Furthermore, studying embryonic mixed tissue methylation levels may provide sensitivity for detecting the trans-generational methylation patterns inherited from the parental generation [Citation35] and present since fertilization. In addition, early life history stages have been early shown to be a critical time point for phenotypic adaptation in this system (Koskinen et al. 2002), therefore further justifying the chosen approach.
A portion of the variation in the methylation levels was explained by grayling chromosome identity. Interestingly, the strong correlation observed between the methylation levels of homeologous chromosome duplicates suggests that some of the epigenetic patterns have originated prior to the salmonid-specific genome duplication [Citation58] and have been conserved over 80 million years. Alternatively, the homeolog-specific methylation patterns may participate in the regulation of transcription of the homeologous gene duplicates [Citation35]. After controlling for the variation explained by the grayling chromosome identity, we were able to detect global plastic responses to developmental temperature in the methylomes.
The temperature-plasticity of the embryonic teleost methylation machinery has been reported for the DNA methylatransferase gene family dnmt3 in whole-embryo samples [Citation59]. However, the global hypomethylation observed here in the colder developmental temperature in four of the five grayling populations studied contradicts the expected negative relationship between temperature and methylation levels, based on an among-species comparison of various fish species inhabiting colder or warmer environments [Citation60]. Methylation levels may be altered by stochastic erosion processes caused by oxidative stress, which results from ageing and various unfavourable conditions such as hypoxia, glucocorticoid exposure, toxicant or nutritional challenges and sub-optimal temperatures, and may ultimately result in the embryonic origin of adult disease [Citation18,Citation61]. In cold-water fish species, such as grayling, oxidative stress may be induced in response to relatively small deviations from the optimal temperature, particularly during early developmental stages when the antioxidant defence may not function efficiently [Citation62]. The reports of increasing methylation levels in response to temperature changes in fish [Citation22,Citation23] may raise the question of whether the global upregulation of the methylation levels under thermal stress is stochastic or adaptive. The regulation of global methylation levels may be necessary in order to maintain equilibrated reactions when variable temperatures change the pace of reactions in the cell. Alternatively, the underlying reason may be found from altered tissue-specific methylation patterns in highly abundant tissues such as muscle.
Further evidence supporting the importance of methylation differentiation in the adaptation process was provided by the observation that a subset of loci with consistent methylation level changes between populations adapted towards different thermal origins. This observation may also link phenotypic responses to methylation changes in some loci, as the consistent methylation changes were mainly located in three genes with well-annotated physiological and developmental effects affecting traits such as the regulation of phototransduction [Citation63], pigmentation [Citation64] and ciliogenesis [Citation65]. Although the underlying causality behind the observed epigenetic patterns in the grayling system remains speculative, such epigenetic adaptation in the same direction in the replicated populations may provide examples of facilitated epigenetic variation, which are variable only in specific genotype contexts (Supplementary Figure 4B) [Citation56]. Unexpectedly, the consistently changed loci were depleted from upstream regulatory regions, and enriched in coding sequences and downstream regulatory regions of genes. This may highlight the importance of considering also other regulatory roles for methylation besides transcriptional intensity adjustment, such as the regulation of the splicing of alternative transcript isoforms.
Whereas the emergence of consistent methylation changes may include adaptive processes resulting in fixed changes in the methylation levels of populations, consistent plastic changes between developmental temperatures within each thermal origin may be used to study the evolution of plasticity. Although partly limited by sample size and population replicates, the loci with consistent epigenetic plasticity in the novel environment (colder thermal origin) within the grayling system were also plastic in the populations from the ancestral environmental condition (warmer-origin populations). Further research may reveal if the epigenetic plasticity maintained in the novel environmental conditions consists of a core subset, selected from ancestral thermal plasticity.
The site-specific comparisons between the methylation levels of individual CpG loci among samples revealed 3,961 temperature- or sex-responsive plastic CpG sites in transcripts corresponding to 2,387 orthologous zebrafish genes. Either a mixed tissue effect caused by whole-embryo sampling or studying a less temperature-responsive developmental stage may explain why we did not observe any enrichments in the gene sets overlapping plastic CpG loci. It has been acknowledged that the study of such developmentally variable effects in teleosts is lacking [Citation35,Citation66]. For example, sex-biased expression was mainly observed in the hatching-stage larvae and not in the embryonic stage in grayling [Citation67]. Studies comparing the molecular mechanisms of thermal plasticity during multiple embryonic developmental points in teleosts are missing, but thermal plasticity likely is more pronounced at some developmental stages than others. The temperature-plastic CpG loci were preferentially associated with CpG-poor upstream regulatory regions, which we previously estimated to be less functionally enriched than the CpG-rich upstream sequences. We selected the grayling transcripts with multiple top developmental temperature-responsive outlier loci, based on P-values, as the strongest candidates for temperature-plastic genes (Supplementary Table 6, Supplementary Figure 5A). Among the most extreme outliers, we found a transcripts best matching to Atlantic salmon dyrk4 among salmonids (LOC106609440; score = 1,010; e-value < 0.0001) which is a gene with well-reported roles in multiple key signalling pathways, important during developmental processes and cell homoeostasis [Citation68] and possibly in phosphorylating voltage-dependent L-type calcium channels [Citation69]. Most of the dyrk4-associated temperature-plastic loci were found in the CpG island-containing promoter region of the longer isoform. Among the top outliers, we also found a transcript matching a salmonid voltage-dependent L-type calcium channel subunit cacna1d (LOC106583449; score = 1,712; e-value < 0.0001) [Citation69], required for transmitting signals in excitable cells, for example, to initiate muscle contraction, or to regulate teleost heart contraction [Citation70,Citation71]. As expected based on previous reports of sex-biased methylation patterns in many vertebrates such as rats, birds and fish [Citation22,Citation72,Citation73] we found loci with sex-related plasticity. Some of the grayling transcripts associated with multiple top sex-biased methylation outlier loci matched to genes associated with reproduction [Citation74–76] or reported with testes-biased expression in the Expression Atlas (accessed 24 May 2019) [Citation77]. Such transcripts were matching to genes such as dyrk4, rangap1 (LOC111981245; e-value < 0.0001; score = 20,556), and fut9 (Fucosyltransferase 9) (LOC106596297; e-value < 0.0001; score 1,260).
Methylation variation was best explained by site-specific models including the population-by-developmental-temperature interaction term in 26,052 CpG loci (2.8% of the loci analysed), indicating the presence of differences in how populations respond to developmental temperature, i.e., gene-by-environment interaction. Many of the gene ontology terms that were enriched among genes with a potential gene-by-environment interaction were related to myosin and motor activity and, possibly, membrane depolarization during an action potential, although this result was non-significant (Supplementary Table 7). Such functions may also be linked to some of the annotations of the top population-by-developmental temperature outliers (Supplementary Table 6). Among these, we found annotations for a giant muscle protein titin [Citation78]; a synthetase of uridine monophosphate (UMP), which may promote muscle endurance [Citation79]; and a gene encoding lipoxygenase homology domains 1b (Loxhd1b), which may cause effects similar to those of the myosin variant myo3a when mutated [Citation80]. Both functional plasticity of cardiac muscle and plasticity affecting muscle growth are key parameters altered by environmental temperature in teleosts [Citation81], including grayling.
The key features of the embryonic grayling methylomes closely resembled those of many vertebrates, including the overall high genome-wide methylation levels [Citation18], contrasted by the more variable upstream regulatory regions. While the low frequency of CpG loci in promoters was related to the abundance of plastic CpG loci, as we observed in site-specific analysis, high upstream CpG abundance associated with functional gene ontology enrichments (Supplementary Table 7, Supplementary Figure 7 A-B). This may highlight the importance of reproducible methylation dynamics during processes such as the development of nervous system and muscle tissue, and developmental growth [Citation82–85]. Such processes were related to hypomethylated upstream sequences along with within-cell functions such as DNA binding, gene expression within organelles and the regulation of cellular and metabolic processes, which may be regularly expressed within cells (Supplementary Table 7, Supplementary Figure 7A). Similar hypomethylation patterns have previously been observed in zebrafish embryos but not necessarily in adults [Citation82,Citation84,Citation85]. In contrast, we were able to link upstream hypermethylation to a set of genes enriched with cell communication functions, such as cell adhesion and transmembrane signalling, which may require more variable expression (Supplementary Table 7, Supplementary Figure 7 B). Hypermethylation related to G-protein signalling, as found in our grayling samples, has also been reported in zebrafish embryos at various stages [Citation82]. In contrast, although genes related to cell adhesion were hypermethylated in grayling embryos during eyed stage, the opposite has been reported during the very early stages of development in zebrafish [Citation85]. Together, these observations may be used as examples of the temporally variable epigenetic regulation of signalling.
Conclusions
Epigenetic regulation has been proposed as an important level of molecular variation in animals. Beyond the observed embryonic grayling methylation patterns, which generally resembled those of a typical vertebrate, the observed methylation- and also nucleotide-level molecular variation was most strongly affected by both neutral evolution and thermal origin. Supporting the hypothesis of a magnified role of methylation in rapid adaptation in this grayling system, the colder thermal origin populations were very similar at the methylation level, whereas at the nucleotide level, patterns were affected by gene flow. Contrastingly, the resulting gene transcription response was mostly plastic, suggesting that epigenetic regulation may affect certain developmental points or tissues. Epigenetic regulation may also affect factors not related to the transcriptional intensity, such as alternative splicing, as suggested by the enrichment of coding sequences and downstream functional regions among the consistently changed methylation loci between population pairs with warmer-to-colder transition in the environmental temperatures. The differences in the plastic cytosine methylation patterns in colder thermal origin populations experiencing a novel environmental condition in comparison to the warmer thermal origin, which resembles the ancestral condition in the grayling system, may provide further support for the importance of methylation in rapid adaptation. Although less obvious, we also detected genome-wide plasticity at the methylation levels as embryos raised in the colder developmental environment were hypomethylated in comparison to individuals raised in warmer developmental environment. Moreover, we found almost 2,000 independent cytosine loci, abundant in (often CpG-poor) upstream regulatory sequences, with a plastic response to developmental temperature. The identified candidate genes for thermal adaptation and plasticity may be interesting subjects for future thermal adaptation studies in other species.
Supplemental Material
Download MS Excel (73.1 KB)Supplemental Material
Download MS Word (2.5 MB)Acknowledgments
We thank the Finnish Centre for Scientific Computing for providing computational resources. We thank Ane Kvinge for assistance with field sampling and the common garden experiment. We thank the editor for their smooth handling of the manuscript. We thank anonymous reviewers for constructive comments and suggestions which greatly improved the manuscript.
Disclosure statement
The authors report no conflict of interest.
Supplementary material
Supplemental data for this article can be accessed here.
Additional information
Funding
References
- IPCC Working group II. Climate change 2014 - Impacts, adaptation, and vulnerability, Part B: regional aspects. Geneva: Cambridge University Press; 2014.
- Cavalheri HB, Symons CC, Schulhof M, et al. Rapid evolution of thermal plasticity in mountain lake Daphnia populations. Oikos. 2018;128:692–700.
- Lustenhouwer N, Wilschut RA, Williams JL, et al. Rapid evolution of phenology during range expansion with recent climate change. Glob Chang Biol. 2018;24:e534–44.
- Parmesan C, Williams-Anderson A, Moskwik M, et al. Endangered Quino checkerspot butterfly and climate change: short-term success but long-term vulnerability? J Insect Conserv. 2015;19:185–204.
- Merilä J, Hendry AP. Climate change, adaptation, and phenotypic plasticity: the problem and the evidence. Evol Appl. 2013;7:1–14.
- Geerts AN, Vanoverbeke J, Vanschoenwinkel B, et al. Rapid evolution of thermal tolerance in the water flea Daphnia. Nat Clim Chang. 2015;5:956.
- Bradshaw WE, Holzapfel CM. Genetic response to rapid climate change: it’s seasonal timing that matters. Mol Ecol. 2008;17:157–166.
- Ghalambor CK, McKay JK, Carroll SP, et al. Adaptive versus non-adaptive phenotypic plasticity and the potential for contemporary adaptation in new environments. Funct Ecol. 2007;21:394–407.
- Lande R. Evolution of phenotypic plasticity in colonizing species. Mol Ecol. 2015;24:2038–2045.
- Fox RJ, Donelson JM, Schunter C, et al. Beyond buying time: the role of plasticity in phenotypic adaptation to rapid environmental change. Philos Trans R Soc B Biol Sci. 2019;374:20180174.
- Reed TE, Robin SW, Schindler DE, et al. Phenotypic plasticity and population viability: the importance of environmental predictability. Proc R Soc B Biol Sci. 2010;277:3391–3400.
- Hendry AP. Key questions on the role of phenotypic plasticity in eco-evolutionary dynamics. J Hered. 2016;107:25–41.
- Ecker S, Pancaldi V, Valencia A, et al. Epigenetic and transcriptional variability shape phenotypic plasticity. BioEssays. 2018;40:1–11.
- Gienapp P, Teplitsky C, Alho JS, et al. Climate change and evolution: disentangling environmental and genetic responses. Mol Ecol. 2008;17:167–178.
- Kronholm I, Collins S. Epigenetic mutations can both help and hinder adaptive evolution. Mol Ecol. 2016;25:1856–1868.
- Schübeler D. Function and information content of DNA methylation. Nature. 2015;517:321–326.
- Peat JR, Ortega-Recalde O, Kardailsky O, et al. The elephant shark methylome reveals conservation of epigenetic regulation across jawed vertebrates. F1000Res. 2017;6:526.
- De Paoli-Iseppi R, Deagle BE, McMahon CR, et al. Measuring animal age with DNA methylation: from humans to wild animals. Front Genet. 2017;8:2010–2017.
- Maunakea AK, Nagarajan RP, Bilenky M, et al. Conserved role of intragenic DNA methylation in regulating alternative promoters. Nature. 2010;466:253–257.
- Neri F, Rapelli S, Krepelova A, et al. Intragenic DNA methylation prevents spurious transcription initiation. Nature. 2017;543:72–77.
- Bogdanović O, Smits AH, De La Calle Mustienes E, et al. Active DNA demethylation at enhancers during the vertebrate phylotypic period. Nat Genet. 2016;48:417–426.
- Metzger DCH, Schulte PM. Persistent and plastic effects of temperature on dna methylation across the genome of threespine stickleback (gasterosteus aculeatus). Proc R Soc B Biol Sci. 2017;284:20171667.
- Skjærven KH, Hamre K, Penglase S, et al. Thermal stress alters expression of genes involved in one carbon and DNA methylation pathways in Atlantic cod embryos. Comp Biochem Physiol - A Mol Integr Physiol. 2014;173:17–27.
- Campos C, Valente LMP, Conceição LEC, et al. Temperature affects methylation of the myogenin putative promoter, its expression and muscle cellularity in Senegalese sole larvae. Epigenetics. 2013;8:389-397.
- Burgerhout E, Mommens M, Johnsen H, et al. Genetic background and embryonic temperature affect DNA methylation and expression of myogenin and muscle development in Atlantic salmon (Salmo salar). PLoS One. 2017;12:1–15.
- Haugen TO, Vøllestad LA. A century of life history evolution in grayling. Genetica. 2001;112–113:475–491.
- Koskinen MT, Nilsson J, Veselov AJ, et al. Microsatellite data resolve phylogeographic patterns in European grayling, Thymallus thymallus, Salmonidae. Heredity (Edinb). 2002;88:391–401.
- Vøllestad LA, Primmer CR. Understanding local adaptation in a freshwater salmonid fish: evolution of a research programme. ICES J Mar Sci. 2019;76:1404–1414.
- Haugen TO. Early survival and growth in populations of grayling with recent common ancestors - Field experiments. J Fish Biol. 2000;56:1173–1191.
- Mäkinen H, Sävilammi T, Papakostas S, et al. Modularity facilitates flexible tuning of plastic and evolutionary gene expression responses during early divergence. Genome Biol Evol. 2018;10:77–93.
- Junge C, Vøllestad LA, Barson NJ, et al. Strong gene flow and lack of stable population structure in the face of rapid adaptation to local temperature in a spring-spawning salmonid, the European grayling (Thymallus thymallus). Heredity (Edinb). 2011;106:460–471.
- Haugen TO, Vøllestad LA. A century of life-history evolution in grayling. Genetica. 2001;112–113:475–491.
- Kavanagh KD, Haugen TO, Gregersen F, et al. Contemporary temperature-driven divergence in a Nordic freshwater fish under conditions commonly thought to hinder adaptation. BMC Evol Biol. 2010;10:350.
- Jiang L, Zhang J, Wang JJ, et al. Sperm, but not oocyte, DNA methylome is inherited by zebrafish early embryos. Cell. 2013;153:773–784.
- Best C, Ikert H, Kostyniuk DJ, et al. Epigenetics in teleost fish: from molecular mechanisms to physiological phenotypes. Comp Biochem Physiol Part - B Biochem Mol Biol. 2018;224:210–244.
- Aljanabi SM, Martinez I, Rural S, et al. Universal and rapid salt-extraction of high quality genomic DNA for PCR-based techniques. Nucleic Acids Res. 1997;25:4692–4693.
- Urich MA, Nery JR, Lister R, et al. MethylC-seq library preparation for base-resolution whole-genome bisulfite sequencing. Nat Protoc. 2015;10:475–483.
- Sävilammi T, Primmer CR, Varadharajan S, et al. The chromosome-level genome assembly of european grayling reveals aspects of a unique genome evolution process within salmonids. G3 Genes. Genomes, Genet. 2019;9:1283–1294.
- Smeds L, Künstner A. ConDeTri - A content dependent read trimmer for illumina. PLoS One. 2011;6:e26314.
- Krueger F, Andrews SR. Bismark: A flexible aligner and methylation caller for Bisulfite-Seq applications. Bioinformatics. 2011;27:1571–1572.
- Quinlan AR, Hall IM. BEDTools: a flexible suite of utilities for comparing genomic features. Bioinformatics [Internet] 2010; 26:841–842.
- Langmead B, Trapnell C, Pop M, et al. Ultrafast and memory-efficient alignment of short DNA sequences to the human genome. Genome Biol. 2009;10:R25.
- Anders S, Pyl PT, Huber W. HTSeq-A Python framework to work with high-throughput sequencing data. Bioinformatics. 2015;31:166–169.
- Risso D, Ngai J, Speed TP, et al. Normalization of RNA-seq data using factor analysis of control genes or samples. Nat Biotechnol. 2014;32:896–902.
- Gao S, Zou D, Mao L, et al. BS-SNPer: SNP calling in bisulfite-seq data. Bioinformatics. 2015;31:4006–4008.
- Tomso DJ, Bell DA. Sequence context at human single nucleotide polymorphisms: overrepresentation of CpG dinucleotide at polymorphic sites and suppression of variation in CpG islands. J Mol Biol. 2003;327:303–308.
- Legendre P, Andersson MJ. Distance-based redundancy analysis: testing multispecies responses in multifactorial ecological experiments. Ecol Monogr. 1999;69:1–24.
- Allendorf FW, Thorgaard GH. Tetraploidy and the evolution of salmonid fishes. In: Turner BJ, editor. Evolutionary genetics of fishes. Boston, MA: Springer US; 1984. p. 1–53.
- Macqueen DJ, Johnston IA. A well-constrained estimate for the timing of the salmonid whole genome duplication reveals major decoupling from species diversification. Proc R Soc B Biol Sci. 2014;281:20132881.
- Schielzeth H, Forstmeier W. Conclusions beyond support: overconfident estimates in mixed models. Behav Ecol. 2009;20:416–420.
- Harrison XA. Using observation-level random effects to model overdispersion in count data in ecology and evolution. PeerJ. 2014;2:e616.
- Stram D, Lee JW. Variance components testing in the longitudinal mixed effects model. Biometrics. 1994;50:1171–1177.
- Frankish A, Vullo A, Zadissa A, et al. Ensembl 2018. Nucleic Acids Res. 2017;46:D754–61.
- Altschul SF, Madden TL, Schäffer AA, et al. Gapped BLAST and PSI-BLAST: A new generation of protein database search programs. Nucleic Acids Res. 1997;25:3389–3402.
- Eden E, Navon R, Steinfeld I, et al. GOrilla: a tool for discovery and visualization of enriched GO terms in ranked gene lists. BMC Bioinformatics. 2009;10:48.
- Richards EJ. Inherited epigenetic variation - revisiting soft inheritance. Nat Rev Genet. 2006;7:395–402.
- Siegal ML, Bergman A. Waddington’s canalization revisited: developmental stability and evolution. Proc Natl Acad Sci. 2002;99:10528–10532.
- Lien S, Koop BF, Sandve SR, et al. The Atlantic salmon genome provides insights into rediploidization. Nature [Internet]. 2016;533:200–205. .
- Campos C, Valente LMP, Fernandes JMO. Molecular evolution of zebrafish dnmt3 genes and thermal plasticity of their expression during embryonic development. Gene. 2012;500:93–100.
- Varriale A. DNA methylation, epigenetics, and evolution in vertebrates: facts and challenges. Int J Evol Biol. 2014;2014:475981.
- Gupta B, Hawkins RD. Epigenomics of autoimmune diseases. Immunol Cell Biol. 2015;93:271–276.
- Simčič T, Jesenšek D, Brancelj A. Effects of increased temperature on metabolic activity and oxidative stress in the first life stages of marble trout (Salmo marmoratus). Fish Physiol Biochem. 2015;41:1005–1014.
- Stearns G, Evangelista M, Fadool JM, et al. A mutation in the cone-specific pde6 gene causes rapid cone photoreceptor degeneration in zebrafish. J Neurosci. 2007;27:13866–13874.
- Braasch I, Schartl M, Volff JN. Evolution of pigment synthesis pathways by gene and genome duplication in fish. BMC Evol Biol. 2007;7:1–18.
- Bontems F, Fish RJ, Borlat I, et al. C2orf62 and TTC17 are involved in actin organization and ciliogenesis in zebrafish and human. PLoS One. 2014;9:e86476.
- Oomen RA, Hutchings JA. Transcriptomic responses to environmental change in fishes: insights from RNA sequencing. Facets. 2017;2:610–641.
- Maitre D, Selmoni OM, Uppal A, et al. Sex differentiation in grayling (Salmonidae) goes through an all-male stage and is delayed in genetic males who instead grow faster. Sci Rep. 2017;7:1–11.
- Aranda S, Laguna A. de la Luna S. DYRK family of protein kinases: evolutionary relationships, biochemical properties, and functional roles. Faseb J. 2010;25:449–462.
- Papadopoulos C, Arato K, Lilienthal E, et al. Splice variants of the dual specificity tyrosine phosphorylation-regulated kinase 4 (DYRK4) differ in their subcellular localization and catalytic activity. J Biol Chem. 2011;286:5494–5505.
- Vornanen M, Shiels HA, Farrell AP. Plasticity of excitation-contraction coupling in fish cardiac myocytes. Comp Biochem Physiol - A Mol Integr Physiol. 2002;132:827–846.
- GW Z, Striessnig J, Koschak A, et al. The physiology, pathology, and pharmacology of voltage-gated calcium channels and their future therapeutic potential. Pharmacol Rev. 2015;67:821–870.
- Nugent BM, Wright CL, Shetty AC, et al. Re: brain feminization requires active repression of masculinization via DNA methylation. Nat Neurosci. 2015;18:690–701.
- Rubenstein DR, Skolnik H, Berrio A, et al. Sex-specific fitness effects of unpredictable early life conditions are associated with DNA methylation in the avian glucocorticoid receptor. Mol. Ecol. 2016;25:1714–1728.
- Rockett JC, Patrizio P, Schmid JE, et al. Gene expression patterns associated with infertility in humans and rodent models. Mutat Res - Fundam Mol Mech Mutagen. 2004;549:225–240.
- Wang CM, Hu SG, Ru YF, et al. Different effects of androgen on the expression of Fut1, Fut2, Fut4 and Fut9 in male mouse reproductive tract. Int J Mol Sci. 2013;14:23188–23202.
- Chunmei W, Huang C, Gu Y, et al. Biosynthesis and distribution of lewis x- And lewis y-containing glycoproteins in the murine male reproductive system. Glycobiology. 2011;21:225–234.
- Petryszak R, Keays M, Tang YA, et al. Expression Atlas update - An integrated database of gene and protein expression in humans, animals and plants. Nucleic Acids Res. 2016;44:D746–52.
- Lange S, Xiang F, Yakovenko A, et al. The Kinase Domain of Titin controls muscle gene expression and protein turnover. Science. 2005;308:1599–1603.
- Gella A, Ponce J, Cusso R, et al. Effect of the nucleotides CMP and UMP on exhaustion in exercise rats. J Physiol Biochem. 2008;64:9–17.
- Grillet N, Schwander M, Hildebrand MS, et al. Mutations in LOXHD1, an Evolutionarily Conserved Stereociliary Protein, Disrupt Hair Cell Function in Mice and Cause Progressive Hearing Loss in Humans. Am J Hum Genet. 2009;85:328–337.
- Johnston IA. Environment and plasticity of myogenesis in teleost fish. J Exp Biol. 2006;209:2249–2264.
- Andersen IS, Reiner AH, Aanes H, et al. Developmental features of DNA methylation during activation of the embryonic zebrafish genome. Genome Biol. 2012;13:R65.
- Borgel J, Guibert S, Li Y, et al. Targets and dynamics of promoter DNA methylation during early mouse development. Nat Genet. 2010;42:1093–1100.
- Potok ME, Nix DA, Parnell TJ, et al. Reprogramming the maternal zebrafish genome after fertilization to match the paternal methylation pattern. Cell. 2013;153:759–772.
- Skvortsova K, Tarbashevich K, Stehling M, et al. Retention of paternal DNA methylome in the developing zebrafish germline. Nat Commun. 2019;10:3054.