ABSTRACT
Chronic kidney disease (CKD) related cardiovascular disease (CVD) is characterized by vascular remodelling with well-established structural and functional changes in the vascular wall such as arterial stiffness, matrix deposition, and calcification. These phenotypic changes resemble pathology seen in ageing, and are likely to be mediated by sustained alterations in gene expression, which may be caused by epigenetic changes such as tissue-specific DNA methylation. We aimed to investigate tissue specific changes in DNA methylation that occur in CKD-related CVD. Genome-wide DNA methylation changes were examined in bisulphite converted genomic DNA isolated from the vascular media of CKD and healthy arteries. Methylation-specific PCR was used to validate the array data, and the association between DNA methylation and gene and protein expression was examined. The DNA methylation age was compared to the chronological age in both cases and controls. Three hundred and nineteen differentially methylated regions (DMR) were identified spread across the genome. Pathway analysis revealed that DMRs associated with genes were involved in embryonic and vascular development, and signalling pathways such as TGFβ and FGF. Expression of top differentially methylated gene HOXA5 showed a significant negative correlation with DNA methylation. Interestingly, DNA methylation age and chronological age were highly correlated, but there was no evidence of accelerated age-related DNA methylation in the arteries of CKD patients. In conclusion, we demonstrated that differential DNA methylation in the arterial tissue of CKD patients represents a potential mediator of arterial pathology and may be used to uncover novel pathways in the genesis of CKD-associated complications.
Introduction
Chronic kidney disease (CKD) is the syndrome characterized by reduced eGFR and kidney damage following a wide range of different underlying diseases. Cardiovascular disease (CVD) is the primary cause of morbidity and mortality among patients with CKD [Citation1]. In CKD-related CVD, structural and morphological changes occur in the blood vessels leading to arterial stiffness, matrix deposition, and calcification [Citation2]. These changes are underpinned by the activation of vascular smooth muscle cells (VSMC), which alter the extracellular matrix (ECM) component causing vascular remodelling [Citation3]. Recently, it has been suggested that these vascular changes represent accelerated ageing [Citation4,Citation5]. Taken together, these phenotypic changes are likely to be a consequence of sustained alterations in gene expression. Inflammation, oxidative stress, and other consequences of uraemia that are evident in CKD induce epigenetic changes [Citation6], including DNA methylation, histone modifications and non-coding RNAs, hence epigenetic modifications are mechanisms through which these long-term phenotypic changes might occur.
In mammals, DNA methylation classically occurs either at cytosine bases of CpG sites that are found spread out across the genome or in or near CpG islands, which are located close to transcription start sites or other regulatory regions. Although DNA methylation is traditionally associated with repression of gene expression in both cases, there is increasing evidence of both down- and up-regulation of gene expression [Citation7]. While ageing is associated with an overall decrease in global DNA methylation, it can also increase or decrease at specific loci and such changes have been proposed to predispose to diseases of ageing including cancer and metabolic disease [Citation8].
A number of studies have demonstrated changes in DNA methylation in patients with CKD although these have been limited to interrogating leucocyte and/or kidney tissue DNA methylation [Citation9–14]. Furthermore, the largest of these studies with longitudinal follow-up suggests the majority of these changes were a consequence of impaired kidney function rather than a cause [Citation11], implying that DNA methylation might be a key mechanism mediating the relationship between CKD and CKD-associated complications, including CKD-related CVD. Finally, it is clear that DNA methylation is not only time dependent but also subject to substantial tissue-specific variation, meaning that understanding the contribution of epigenetic mechanisms to pathology requires the interrogation of the tissue of interest [Citation15].
We hypothesized that the changes observed in the arterial wall in CKD-related CVD are mediated through DNA methylation of regulatory elements of key genes implicated in the pathobiology of disease and that understanding which genes and pathways are subject to this epigenetic regulation might provide key insight into CKD-related CVD. We aimed to describe genome-wide differences in arterial wall DNA methylation in patients with CKD versus healthy controls. We then validated the observed differentially methylated regions using methylation-specific PCR and assessed the downstream impact on gene and protein expression. Finally, we explored the relationship between DNA methylation patterns observed in CKD and those that occur in otherwise healthy ageing.
Results
Participants
Thirty-two individuals contributed specimens for this study, of whom 25 were CKD patients and 7 were healthy donors. The study groups are summarized in . Mean age was 48.2 years in cases and 51.14 years in controls. The percentage of male and female participants was similar between groups. Half of the CKD patients (48%) were on dialysis.
Table 1. Demographic data. Mean age is given in years. CKD = chronic kidney disease patients, Control = healthy donors, CI = 95% confidence interval
Differentially methylated regions of arterial DNA potentially identify known and new disease-related pathways
We used the EPIC genome-wide methylation array to access the methylation status of DNA isolated from the VSMC of CKD patients and healthy subjects ()). Four thousand and five hundred (4.5 k) differentially methylated probes (DMP), hypermethylated (Δβ<0) or hypomethylated (Δβ>0) in CKD, reached the genome-wide significance p-value (cut-off p-value< 9 × 10−8) [Citation16] ()). The differentially methylated probes encompassed 319 differentially methylated regions (DMR). DMRs were found across the genome in all autosomal chromosomes ()), with 87% of them being directly associated with genes ()). Strong enrichment was seen near promoters, exons, introns, and intron/exon junctions ()). The remaining 13% were associated with CpG islands, island shores, and shelves (up to 2Kb and 4Kb from the CpG island respectively [Citation17]), and inter-island regions ()).
Figure 1. Annotation of differentially methylated probes and regions. (a) Cluster plot for CKD and control samples based on their methylation profiles. (b) Pie chart of 4.5 k DMPs that reached genome-wide significance (p-value <9x10−8) showing percentage of hypermethylated (52%) and hypomethylated (48%) probes. (c) Bar plot showing the position of the DMRs across the human genome in the autosomal chromosomes. (d) Basic annotation of the DMRs in relation to genomic regions. Y-axis: % enrichment normalized to background. (e) Gene set enrichment and gene ontology analysis showing lead gene ontology categories (biological processes), and representative genes per category. X-axis: -log10 of the adjusted p-values
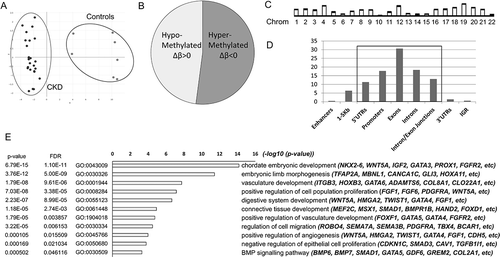
Gene set enrichment and gene ontology analysis revealed that genes associated with DMRs participate in pathways related to embryonic development and morphogenesis (NKX2-6, WNT5A,TWIST1, etc.), regulation of vascular development (GATA3/4/5, FGFR2, etc.), cell proliferation, and migration (SMAD3, CAV1, PDGFRA, etc.), as well as signalling pathways such as TGFβ, FGF, Rho signalling and others ()). Top hypermethylated and hypomethylated probes are shown in . Interestingly, among the top differentially methylated regions are found genes and homeobox containing transcription factors with established roles in embryonic development and maturation of the vascular system ().
Table 2. List of top differentially methylated probes (DMP). Hypo- and hyper- methylated probes are shown based on their adjusted p-values. Δβ value shows the methylation difference of CKD and control samples. DMP-associated genomic regions and/or genes are shown. Chrom: chromosome; IGR: intergenic region; UTR: untranslated region. TSS1500: 1500bp from the transcription start site
Table 3. Lead differentially methylated regions (DMR). Lead DMRs based on their adjusted p-values (Bumphunter p-value area). The name of the genes associated with each DMR is given, as well as the chromosomal location, the genomic region, and the number of probes (DMPs) found in each DMR
Validation of the methylation array data using MSP
To validate the methylation array data, methylation-specific PCR (MSP) was used. Primers for randomly selected DMRs were specifically designed to amplify methylated and unmethylated DNA ()). Methylation levels in MSP were consistent with the array data and showed a strong positive correlation in most cases studied (DMR 8: Spearman r = 0.75, p-value = 0.0007, DMR 9: Spearman r = 0.71, p-value = 0.0019) (), Supplementary Figure 1). DMRs 8 and 9 were both found hypermethylated in CKD cases compared to healthy individuals in the EPIC array (DMR 8: adjusted p-value = 1.48E-05, DMR 9 adjusted p-value = 4.43E-05) (Supplementary Figure 1(a)). DMR 8 spans a 1.5 kb long genomic region containing a CpG island that crosses intron/exon junctions of the overlapping C5orf66 and PITX1 genes, while DMR 9 is found at the 3’ UTR of PITX1 gene. These data confirm that the methylation array reproducibly captures the quantitative methylation status of loci across the human genome in the arterial tissue using our sample pipeline.
Figure 2. Methylation specific PCR (MSP). (a) Primers were designed to specifically amplify methylated and unmethylated DNA. Control 0% (100% u) and 100% (100% m) methylated DNA samples were used individually or at a ratio of 50:50 to assess the dose-response across methylation levels. (b,c) correlation analysis of methylation using MSP (y-axis) and EPIC array methylation (x-axis). r: Spearman correlation coefficient
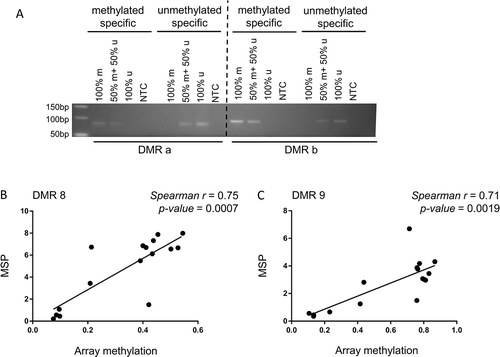
Association of DNA methylation and gene and protein expression
To further look into the biological relevance of DNA methylation, gene-expression levels of differentially methylated genes were examined. Genes were selected from the top 60 DMRs based on the differential methylation levels, the location of each DMR, and the annotated gene function and properties. Gene expression was tested for the HOXA5, HLX, HAND2, SLC41A3, SVIL, and FMOD genes, and correlated with the EPIC array methylation values of the corresponding DMRs ( and Supplementary Figure 2).
Figure 3. DNA methylation associates with HOXA5 gene and protein expression. Correlation of (a) HOXA5 gene expression in VSMC, normalized to housekeeping genes and (b) HOXA5 protein expression, assessed by immunohistochemistry with the EPIC methylation array values of the corresponding DMR 17. r: Spearman correlation coefficient
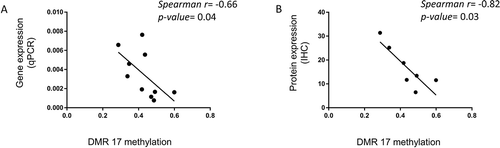
HOXA5 gene and protein expression (qPCR and immunohistochemistry, respectively) was strongly negatively correlated with DMR 17 methylation values (p-value = 0.043, p-value = 0.03, respectively) (), suggesting a direct effect of DNA methylation on gene expression. DMR 17 is located at the promoter of HOXA5 gene and was found hypermethylated in CKD in the EPIC array. When HOXA5 gene expression was examined, it was found significantly downregulated in CKD (Supplementary Figure 3). There was a suggestion for downregulation of HOXA5 protein in CKD, but the difference in expression did not reach statistical significance (Supplementary Figure 3).
The changes in DNA methylation in the arterial wall of patients with CKD do not parallel those observed in ageing
Using the methylation values of the EPIC array and the ‘Horvath clock’, a tissue-independent age predictor [Citation18], we calculated the estimated methylation age of the samples. The predicted methylation age was correlated well with the actual chronological age of the subjects in both cases and controls () (Spearman correlation r = 0.92, p-value <0.0001). However, no evidence of a higher DNA methylation age was found in CKD patients, suggesting that DNA methylation does not mediate a process of accelerating ageing in the arteries of CKD patients.
Discussion
Although the exploration of epigenetic modifications in CKD has grown in the recent years, this is the first time that the methylation status of arterial wall DNA of CKD patients and healthy individuals have been quantified. Here, we examined the methylation status of VSMC at genome-wide level and we showed that there are approximately 319 large genomic regions and 4.5 k probes that are differentially methylated in the arteries of patients with CKD. The regions are spread across the genome, and mostly found in or near genes, promoters, and regulatory regions. The most significantly differentially methylated regions are associated with genes, processes, and signalling pathways with roles in development, vasculature, and fibrotic diseases.
In detail, differentially methylated genes are involved in the production and deposition of the extracellular matrix, encoding for collagenous and non-collagenous proteins (COL16A1, COL26A1, CLO6A3, COL7A1, COL9A3, COMP), as well as proteins involved in the matrix deposition and degradation such as metalloproteinases and metallopeptidases (ADAMTS8/9/12, MMP2, LOXL1). Members of TGF-β, FGF, PDGF, and Rho signalling pathways were also found to be differentially methylated in our dataset. In particular, signalling molecules of the TGFβ (TGFβ1, TGFβ1l1, SMAD3) and FGF (FGF1, FGF6, FGFBP2) pathways were all found hypomethylated in CKD. Interestingly, although CpG probes in or near GATA5 were found significantly hypomethylated (e.g., cg08583859, Δβ = 0.189, p-value = 3.81E-11), probes near GATA4 were found both hypermethylated and hypomethylated in CKD, indicating a putative functional role for GATA binding site-dependent regulation. RHOH and RHOJ genes as well as other Rho/Ras family members (e.g., ARHGAP25, ARHGEF25) were also found differentially methylated, contributing to the published evidence about the role of Rho signalling in the development and progression of vascular disease [Citation19,Citation20]. Interestingly, although recent evidence highlight the involvement of the immune system in the development of vascular disease [Citation21], we confirmed that the effects seen in this study were driven by changes in the vascular media and not by infiltration of immune cells populations (Supplementary Figure 5) [Citation22].
In contrast to a number of similar studies, we confirmed the accuracy of our array results at lead loci, demonstrating consistent changes in DNA-methylation using a MSP approach. Validation of the direction of array methylation using the MSP method might vary dependent on the locus of the methylated region in relation to the closest gene, with DMRs located in open sea/intragenic regions exhibiting indirect associations (Supplementary Figure 1(a,b)). We also showed that DNA methylation associates well with gene and protein expression at our lead locus, with HOXA5 gene and protein expression being downregulated (Supplementary Figure 3) in those samples, following hypermethylation of the gene promoter. HOXA5 encodes a homeobox transcription factor with important roles not only in embryonic development but also as a morphoregulatory gene in tissue remodelling, angiogenesis, and wound healing [Citation23]. A recent study speculated the role of HOXA5 as an anti-atherogenic gene and showed that disturbed blood flow-dependent DNA methylation caused hypermethylation of HOXA5 promoter and therefore silencing of gene expression [Citation24]. Hence, the data presented in this study in accordance with other published data support the role of HOXA5 as a mechanosensitive gene and the concept that DNA methylation-dependent HOXA5 repression contributes to pathologic tissue remodelling seen in CKD-related CVD leading to abnormal vasculature.
Similar analysis at other lead loci demonstrated unexpected results. DMR 7 is located 1–5Kb upstream of the HAND2 gene transcription start site, and was found hypermethylated in CKD. However, hypermethylation at DMR 7 was associated with an increase in gene expression of the HAND2 gene (Supplementary Figure 2). Interestingly, DMR 7 is also in close proximity to the HAND2-antisense 1 gene, which shares a bidirectional promoter with HAND2. Although we could not demonstrate a clear association between methylation levels and expression of the anti-sense gene, these findings underline the complex-relationship between CpG methylation and expression of proximate genes and reinforce the importance of examining the impact of DNA-methylation on downstream biological pathways. In fact, the analysis of association between the location of the methylated DNA sites and gene expression has identified new patterns of methylation-mediated gene regulation including examples of methylation activating gene expression [Citation25,Citation26]. Studying expression of HAND2 gene is a good example as there is accumulating evidence suggesting that gene expression is strongly regulated by DNA methylation in the context of other diseases too [Citation27]. Other loci examined demonstrated quantitative trends towards inverse associations between gene-expression and methylation but not reaching thresholds for statistical significance (Supplementary Figure 2).
Furthermore, we aimed to address whether methylation changes in arterial DNA reflect epigenetic changes occurring in premature ageing. We used the ‘Horvath’ clock [Citation18] to calculate the ‘DNA-methylation age’ for each sample, and as predicted, there was a strong correlation between methylation and chronological age across cases and controls. Interestingly, CKD-case status was not associated with an increase in ‘DNA-methylation age’ over healthy controls suggesting that CKD-associated arterial pathology is not a simplistic process of accelerated ageing at least at the DNA methylation level. However, the ‘Horvath’ clock is built as a pan-tissue clock programmed on differing tissue training sets, which reduces the possibilities of identifying ageing-related changes associated with organ-specific pathologies [Citation28]. Therefore, using other disease-focused epigenetic clocks might capture the changes in accelerating ageing that occur due to CKD-cardiovascular pathology [Citation28].
The great majority of the published studies examining the epigenetic changes in CKD have been focused on the methylation status of DNA derived from whole blood, and to a lesser extent kidney tissue. In our study, we were interested in the association between methylation and CKD-related vascular complications, and therefore we aimed to assess the effect of DNA-methylation on gene and protein expression in VSMC. Our data are partially comparable with other published reports of differential DNA methylation in CKD in other tissues. In common with a previous report on DNA methylation of proximal tubule epithelial cells by Ko et al [Citation10], our study confirmed that differentially methylated genes in CKD are associated with known players and regulators of cell adhesion, development-related functions and signalling pathways involved in fibrosis, apoptosis and vasculature, such as collagen, fibronectin and other structural components, the TGFβ signalling pathway and SMAD proteins.
Another study by Smyth and colleagues was performed in leukocyte DNA to estimate the epigenetic features of CKD in which they identified 23 differentially methylated genes with a strong biological and functional relevance to CKD pathogenesis [Citation9]. Evidence of differential DNA methylation of the STK24 gene was replicated in our study, where the associated DMR 260 was also significantly hypermethylated in CKD. DMR 260 is located within an intron of the STK24 gene, which encodes for a serine/threonine protein kinase mediating phosphorylation of MAP kinases. Furthermore, evidence of hypermethylation of cg19942083 in CKD, which was reported by Chu and colleagues [Citation11], was also replicated in our study (Δβ = −0.0185). Association of increased methylation of this probe with prevalent CKD and estimated glomerular filtration rate (eGFR) has been replicated in two independent cohort studies and in a meta-analysis (corrected p-value = 2.8E-10) [Citation11]. Probe cg19942083 is located within an active enhancer between PTPN6 and PHB2 genes, and Chu et al. showed that increased methylation at the locus is associated with increased eGFR as well as decreased expression of PTPN6 gene and renal fibrosis [Citation11]. Interestingly, association of PTPN6 differential methylation with eGFR was again recently replicated [Citation29] supporting further the role of PTPN6 gene as a promising candidate gene in kidney disease. Future studies are required to elucidate the role of these genes in the development and progression of cardiovascular pathology in CKD.
Key strengths of this study include the use of the EPIC array, which assesses the DNA methylation status at epigenome scale examining over 850 k probes spread across the genome. Our key advantage compared to similar studies is the use of a specific tissue of interest that enabled us to directly associate successfully the effect of methylation with gene and protein expression in the arterial wall. In addition, this approach facilitated our comprehension of the epigenetic phenomena and their relation to gene function and role in the CKD-related pathobiology.
The limited number of samples and the heterogeneous DNA material obtained from the healthy donors are some key downsides of this study. Nevertheless, the two groups of the study were clustered in two clear and very distinct populations based on their methylation profile. Another limitation is the lack of any hard clinical outcomes associated with CKD-related cardiovascular phenotypes among the CKD cases. Although this study provided evidence that DNA methylation status is a valuable tool to identify genes and mechanisms implicated in CKD-related CVD, further studies are required to determine whether the effect of DNA methylation retains a causal role or is an epiphenomenon of alternative underlying mechanisms and genome-epigenome crosstalk. Investigation of probes that exhibit clustered distribution in DNA methylation, known as ‘gap probes’, will also aid this cause [Citation30]. DNA methylation signals of gap probes, which are usually associated with adjacent SNPs, have a strong genetic basis, and they can be used as reliable surrogates for underlying genetic structure that is biologically relevant to the phenotype. In our study, we have identified [Citation30] a number of gap probes associated with differentially methylated loci, including probes in DMR 17, highlighting that potential genetic effects are present and need to be further investigated. Animal models of CKD-related CVD and mechanisms that block methylation, as well as further genetic annotation and analysis of the methylated loci could enlighten the role of DNA methylation in the cardiovascular component of CKD.
In summary, we have demonstrated differential DNA methylation at a range of loci in arterial tissue from patients with CKD as compared to healthy controls. These epigenetic changes represent a potential mediator of arterial pathology in those with renal failure, which in turn may be a result of the uraemic milieu or other consequences of advanced CKD. Our findings also suggest that CKD-associated CVD does not reflect epigenetic changes seen in ageing and alternative paradigms should be sorted. We established the use of profiling the epigenome-wide methylation status in CKD-derived arterial DNA as a proof of concept to uncover novel pathways in the genesis of CKD-associated complications.
Participants, methods and materials
Study participants and donation of arterial material
The study protocol was approved by the London – Queen Square Research Ethics Committee (ref: 16/LO/0269) and all participants provided written informed consent.
All cases were patients with end-stage renal disease undergoing live or cadaveric-donor single organ renal transplantation at the Royal Free London NHS Foundation Trust. At the time of surgery, a small portion (1–2 cm) of inferior epigastric artery (IEA) was retrieved. Control samples were obtained from patients undergoing live kidney donation operations as well as tissue from the main renal artery of donated live and cadaveric donor kidneys (the latter not transplanted and donated for research). At surgery (at the discretion of the operating surgeon dependent on whether substantial additional dissection was required) either the IEA was divided, and portion retrieved (~1 cm) or alternatively, excess material from the renal artery of the explanted kidney was collected for research. All specimens were placed in ice-cold saline before further processing. Basic demographic and clinical data were collected on all participants.
Tissue specimens
Within 1 h of retrieval, endothelial and adventitial layers were dissected off the arterial specimen. The remaining arterial media was either stabilized, in RNA later (Thermo Fisher, Cat. no: AM7020) or All Protect tissue reagent (Qiagen, Cat. no: 76405) for future isolation of nuclei acids, or fixed in formalin before transfer to ethanol for histological processing.
Nucleic acid isolation from human vessels
For the isolation of DNA and RNA the AllPrep DNA/RNA Mini kit (Qiagen, Cat. no: 80204) was used. DNA and RNA were isolated from the arterial media (vascular smooth muscle layer) of 25 recipients (CKD patients; IEA) and 7 donors (controls; IEA or renal artery) during kidney transplantation procedures. 0.3–0.6 mg of prepared vascular tissue was homogenized in 600 µl lysis buffer with stainless steel beads (Qiagen, Cat. no: 69989) using a conventional tissue-lyser. The samples were kept ice-cold during homogenization. DNA and RNA were then isolated following the manufacturer’s instructions. DNA was purified and concentrated by ethanol precipitation.
Bisulphite conversion of DNA
250ng DNA/sample was bisulphite-converted (EZ DNA Methylation kit, Zymo, Cat. no: D5001) and methylation status was assessed by the Illumina EPIC array (UCL Genomics Institute). For the methylation-specific PCRs DNA was bisulphite-converted following the same protocol.
Illumina methylation array analysis
The Illumina EPIC methylation array assessed over 850.000 methylation sites across the genome at single-nucleotide resolution. Methylation sites outside CpG islands, as well as probes that span enhancers and promoters identified by ENCODE and FANTOM, open chromatin regions and DNase hypersensitivity sites are also included [Citation31].
For the methylation data analysis and the identification of differentially methylated probes and regions, the ChAMP package was used exclusively in R 4.0 [Citation32,Citation33]. Failed probes (detection p-value <0.01), SNP-related probes [Citation34], multi-hit probes [Citation35], and probes located on the XY chromosomes were filtered out from the dataset. The methylation is measured using the beta (β) value, which ranges from 0 (no methylation) to 1 (100% methylation), and offers an intuitive biological interpretation. The type 2 probe bias was normalized using the BMIQ method [Citation36]. For the principal component analysis and the detection of batch effects, singular value decomposition (SVD) method was used [Citation37], and the technical variation was corrected using ComBat [Citation38] on the normalized values (Supplementary Figure 4). ComBat was performed once to correct the ‘array’ batch variation only, as this was the batch with the biggest effect on the dataset. To prevent correction of batch effects that would be influenced by group differences in case of unbalanced group design, sample group was not included in the model matrix during ComBat analysis.
For the identification of the differentially methylated probes between CKD patients and healthy controls, the Limma package [Citation39] was used implemented through ChAMP. Differentially methylated regions were identified using the Bumphunter algorithm [Citation40], with each region containing a minimum of seven DMPs. The p-values were corrected using the ‘bootstrap’ method through ChAMP. Gene enrichment analysis was performed using the ‘gometh’ method of ‘missMethyl’ package [Citation41] through ChAMP. This method reduces significantly the bias during gene set analysis by adjusting the number of CpGs attributed to a gene or locus. The list of DMRs was used as input for the Annotatr package [Citation42] to dissect genomic annotations.
Methylation-specific PCR (MSP)
Methylation-specific PCRs were performed using the Epitect MSP kit (Qiagen, Cat. no: 59305). Two different sets of primers (Supplementary Table 1) were designed for each region, specific either to methylated or unmethylated DNA using the MethPrimer program (www.urogene.org). Most of the primers spanned at least one CpG to increase the primer specificity and efficiency to distinguish between methylated and unmethylated DNA. 2 µl of bisulphite-converted DNA (~10 ng) was used per MSP reaction. One hundred percent methylated DNA and unmethylated DNA (0% methylation) were used as positive and negative control, respectively (Qiagen, Cat. no: 59568). MSP products were run in a 2% agarose gel, visualized and photographed using a UVP gel documentation system (BioSpectrum). Densitometry analysis was performed in ImageJ to infer the relative methylation of the locus per sample in relation to the positive (methylation value = 1) and negative control (methylation value = 0).
Immune cell population involvement
A cell-type fraction analysis was performed with EpiDISH [Citation22] using the beta values of our dataset.
Gene expression analysis- Quantitative PCR
500 ng RNA/sample was reverse transcribed following a standard protocol (Thermo Fisher, Cat. no: 4368814) in a total reaction volume of 100 µl. 2 µl of cDNA (1:10 dilution) was used per qPCR reaction (QuantiFast SYBR Green, Qiagen, Cat. no: 204054). Relative gene expression was normalized against the mean Ct of three housekeeping genes: RPL13, HPRT, and ACTB. Primer sequences and annealing temperature for each primer pair are given in the Supplementary Table 2.
Immunohistochemistry
Immunohistochemistry was performed on formalin fixed paraffin-embedded epigastric arteries isolated during the kidney transplantation procedures. After antigen retrieval with 1 mM EDTA pH 8.0, sections were immunodecorated with optimally diluted antibodies (HOXA5: sc-515309, Santa-Cruz, 0.5 µg/ml in TBS). Labelled Polymer – Dako REAL EnVision-HRP (Dako, K4000) was used prior to developing with 3,3’-Diaminobenzidine (DAB) reagent (Dako, K3467). Specificity of staining was confirmed using isotype-matched IgG control antibodies (0.5 µg/ml).
Statistical analysis
Tests for differences in CpG methylation at specific loci (as determined by MSP), gene and protein expression, between groups were performed using Student’s t-test. The association between CpG methylation (assessed by EPIC array) and age, CpG methylation (by MSP), gene or protein expression was tested using Pearson correlation coefficient. The analysis was performed in GraphPad Prism 5. Post-hoc power calculations were performed using pwrEWAS [Citation43].
Author contributions
BC conceived the study with assistance from JN, SB, DCW and MP. BL led the surgical aspects of the work. AD, MK and AO collected the samples and performed the laboratory procedures. AD performed the analysis with support from APW, TO and SB. AD and BC drafted the manuscript with support from SB and TO. All authors read and approved the final submission.
Supplemental Material
Download MS Word (1,023.7 KB)Acknowledgments
The authors thank all the participants as well as Royal Free London Renal Transplant Surgical team for their help collecting samples.
Disclosure statement
No potential conflict of interest was reported by the authors.
Supplementary material
Supplemental data for this article can be accessed here.
Additional information
Funding
References
- Go AS, Chertow GM, Fan D, et al. Chronic kidney disease and the risks of death, cardiovascular events, and hospitalization. N Engl J Med. 2004;351(13):1296–1305.
- Blacher J, Guerin AP, Pannier B, et al. Arterial calcifications, arterial stiffness, and cardiovascular risk in end-stage renal disease. Hypertension. 2001;38(4):938–942.
- Briet M, Burns KD. Chronic kidney disease and vascular remodelling: molecular mechanisms and clinical implications. Clin Sci (Lond). 2012;123(7):399–416.
- London GM, Safar ME, Pannier B. Aortic aging in ESRD: structural, hemodynamic, and mortality implications. J Am Soc Nephrol. 2016;27(6):1837–1846.
- Hamczyk MR, Nevado RM, Barettino A, et al. Biological versus chronological aging: JACC focus seminar. J Am Coll Cardiol. 2020;75(8):919–930.
- Zawada AM, Rogacev KS, Heine GH. Clinical relevance of epigenetic dysregulation in chronic kidney disease-associated cardiovascular disease. Nephrol Dialysis Transplantation. 2013;28(7):1663–1671.
- Schübeler D. Function and information content of DNA methylation. Nature. 2015;517(7534):321–326.
- Bell CG, Beck S. The epigenomic interface between genome and environment in common complex diseases. Brief Funct Genomics. 2010;9(5–6):477–485.
- Smyth LJ, McKay GJ, Maxwell AP, et al. DNA hypermethylation and DNA hypomethylation is present at different loci in chronic kidney disease. Epigenetics. 2014;9(3):366–376.
- Ko YA, Mohtat D, Suzuki M, et al. Cytosine methylation changes in enhancer regions of core pro-fibrotic genes characterize kidney fibrosis development. Genome Biol. 2013;14(10):R108.
- Chu AY, Tin A, Schlosser P, et al. Epigenome-wide association studies identify DNA methylation associated with kidney function. Nat Commun. 2017;8(1):1286.
- Wing MR, Devaney JM, Joffe MM, et al. DNA methylation profile associated with rapid decline in kidney function: findings from the CRIC study. Nephrol Dial Transplant. 2014;29(4):864–872.
- Sapienza C, Lee J, Powell J, et al. DNA methylation profiling identifies epigenetic differences between diabetes patients with ESRD and diabetes patients without nephropathy. Epigenetics. 2011;6(1):20–28.
- Lecamwasam A, Sexton-Oates A, Carmody J, et al. DNA methylation profiling of genomic DNA isolated from urine in diabetic chronic kidney disease: a pilot study. PLoS One. 2018;13(2):e0190280.
- Schultz MD, He Y, Whitaker JW, et al. Human body epigenome maps reveal noncanonical DNA methylation variation. Nature. 2015;523(7559):212–216.
- Mansell G, Gorrie-Stone TJ, Bao Y, et al. Guidance for DNA methylation studies: statistical insights from the Illumina EPIC array. BMC Genomics. 2019;20(1):366.
- Irizarry RA, Ladd-Acosta C, Wen B, et al. The human colon cancer methylome shows similar hypo- and hypermethylation at conserved tissue-specific CpG island shores. Nat Genet. 2009;41(2):178–186.
- Horvath S. DNA methylation age of human tissues and cell types. Genome Biol. 2013;14(10):R115.
- Zhou Q, Liao JK. Rho kinase: an important mediator of atherosclerosis and vascular disease. Curr Pharm Des. 2009;15(27):3108–3115.
- Duluc L, Wojciak-Stothard B. Rho GTPases in the regulation of pulmonary vascular barrier function. Cell Tissue Res. 2014;355(3):675–685.
- Fani L, van der Willik KD, Bos D, et al. The association of innate and adaptive immunity, subclinical atherosclerosis, and cardiovascular disease in the rotterdam study: a prospective cohort study. PLoS Med. 2020;17(5):e1003115.
- Zheng SC, Webster AP, Dong D, et al. A novel cell-type deconvolution algorithm reveals substantial contamination by immune cells in saliva, buccal and cervix. Epigenomics. 2018;10(7):925–940.
- Kachgal S, Mace KA, Boudreau NJ. The dual roles of homeobox genes in vascularization and wound healing. Cell Adh Migr. 2012;6(6):457–470.
- Dunn J, Qiu H, Kim S, et al. Flow-dependent epigenetic DNA methylation regulates endothelial gene expression and atherosclerosis. J Clin Invest. 2014;124(7):3187–3199.
- Kulis M, Heath S, Bibikova M, et al. Epigenomic analysis detects widespread gene-body DNA hypomethylation in chronic lymphocytic leukemia. Nat Genet. 2012;44(11):1236–1242.
- Spainhour JC, Lim HS, Yi SV, et al. Correlation patterns between DNA methylation and gene expression in the cancer genome atlas. Cancer Inform. 2019;18:1176935119828776.
- Jones A, Teschendorff AE, Li Q, et al. Role of DNA methylation and epigenetic silencing of HAND2 in endometrial cancer development. PLoS Med. 2013;10(11):e1001551.
- Bell CG, Lowe R, Adams PD, et al. DNA methylation aging clocks: challenges and recommendations. Genome Biol. 2019;20(1):249.
- Chen J, Huang Y, Hui Q, et al. Epigenetic associations with estimated glomerular filtration rate (eGFR) among men with HIV infection. Clin Infect Dis. 2020;70(4):667–673.
- Andrews SV, Ladd-Acosta C, Feinberg AP, et al. “Gap hunting” to characterize clustered probe signals in Illumina methylation array data. Epigenetics Chromatin. 2016;9(1):56.
- Pidsley R, Zotenko E, Peters TJ, et al. Critical evaluation of the illumina methylationEPIC beadchip microarray for whole-genome DNA methylation profiling. Genome Biol. 2016;17(1):208.
- Morris TJ, Butcher LM, Feber A, et al. ChAMP: 450k chip analysis methylation pipeline. Bioinformatics. 2014;30(3):428–430.
- Aryee MJ, Jaffe AE, Corrada-Bravo H, et al. Minfi: a flexible and comprehensive bioconductor package for the analysis of Infinium DNA methylationmicroarrays. Bioinformatics. 2014;30(10):1363–1369.
- Zhou W, Laird PW, Shen H. Comprehensive characterization, annotation and innovative use of infinium DNA methylation BeadChip probes. Nucleic Acids Res. 2017;45(4):e22.
- Nordlund J, Backlin CL, Wahlberg P, et al. Genome-wide signatures of differential DNA methylation in pediatric acute lymphoblastic leukemia. Genome Biol. 2013;14(9):r105.
- Teschendorff AE, Marabita F, Lechner M, et al. A beta-mixture quantile normalization method for correcting probe design bias in illumina infinium 450 k DNA methylation data. Bioinformatics. 2013;29(2):189–196.
- Teschendorff AE, Menon U, Gentry-Maharaj A, et al. An epigenetic signature in peripheral blood predicts active ovarian cancer. PLoS One. 2009;4(12):e8274.
- Johnson WE, Li C, Rabinovic A. Adjusting batch effects in microarray expression data using empirical Bayes methods. Biostatistics. 2007;8(1):118–127.
- Ritchie ME, Phipson B, Wu D, et al. limma powers differential expression analyses for RNA-sequencing and microarray studies. Nucleic Acids Res. 2015;43(7):e47.
- Jaffe AE, Murakami P, Lee H, et al. Bump hunting to identify differentially methylated regions in epigenetic epidemiology studies. Int J Epidemiol. 2012;41(1):200–209.
- Phipson B, Maksimovic J, Oshlack A. missMethyl: an R package for analyzing data from Illumina’s HumanMethylation450 platform. Bioinformatics. 2016;32(2):286–288.
- Cavalcante RG, Sartor MA. annotatr: genomic regions in context. Bioinformatics. 2017;33(15):2381–2383.
- Graw S, Henn R, Thompson JA, et al. pwrEWAS: a user-friendly tool for comprehensive power estimation for epigenome wide association studies (EWAS). BMC Bioinformatics. 2019;20(1):218.