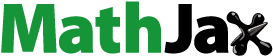
ABSTRACT
Target organ damage (TOD) manifests as vascular injuries in the body organ systems associated with long-standing hypertension. DNA methylation in peripheral blood leukocytes can capture inflammatory processes and gene expression changes underlying TOD. We investigated the association between epigenome-wide DNA methylation and five measures of TOD (estimated glomerular filtration rate (eGFR), urinary albumin-creatinine ratio (UACR), left ventricular mass index (LVMI), relative wall thickness (RWT), and white matter hyperintensity (WMH)) in 961 African Americans from hypertensive sibships. A multivariate (multi-trait) model of eGFR, UACR, LVMI, and RWT identified seven CpGs associated with at least one of the traits (cg21134922, cg04816311 near C7orf50, cg09155024, cg10254690 near OAT, cg07660512, cg12661888 near IFT43, and cg02264946 near CATSPERD) at FDR q < 0.1. Adjusting for blood pressure, body mass index, and type 2 diabetes attenuated the association for four CpGs. DNA methylation was associated with cis-gene expression for some CpGs, but no significant mediation by gene expression was detected. Mendelian randomization analyses suggested causality between three CpGs and eGFR (cg04816311, cg10254690, and cg07660512). We also assessed whether the identified CpGs were associated with TOD in 614 African Americans in the Hypertension Genetic Epidemiology Network (HyperGEN) study. Out of three CpGs available for replication, cg04816311 was significantly associated with eGFR (p = 0.0003), LVMI (p = 0.0003), and RWT (p = 0.002). This study found evidence of an association between DNA methylation and TOD in African Americans and highlights the utility of using a multivariate-based model that leverages information across related traits in epigenome-wide association studies.
Background
More than 40% of US adults have hypertension, with African Americans having higher prevalence than that of any other racial group [Citation1]. According to the 2011–2014 National Health and Nutrition Examination Survey, the prevalence of hypertension was 59% in African American men and 56% in African American women, compared to a prevalence of 47% and 41% in non-Hispanic White men and women [Citation1]. Due to long-standing hypertension, target organ damage (TOD) manifests as subclinical or clinical changes to the micro and macro vascular systems of the heart, brain, eyes, and kidneys [Citation2]. Oxidative stress, endothelial dysfunction, extracellular matrix formation, and innate and adaptive immune cell activation and invasion are some of the mechanisms recognized to play a role in TOD onset and progression [Citation2,Citation3]. Previous research indicates that measures of TOD are independent and strong predictors of cardiovascular morbidity and mortality and all-cause mortality [Citation4–7]. However, there are gaps in our knowledge about the progressive effects of hypertension over the life course and which risk factors contribute to the development of subclinical changes and symptomatic diseases related to TOD. African Americans are especially susceptible to manifestations of target organ damage from hypertension leading to a higher risk of adverse cardiovascular and renal outcomes and mortality [Citation8,Citation9]. Previous studies have shown that African Americans have greater left ventricular mass [Citation10,Citation11], higher incidence of chronic kidney disease, and end stage renal disease compared to non-Hispanic Whites [Citation12,Citation13]. Better understanding of the pathogenesis of TOD could elucidate and improve our prediction of related morbidities and mortality, especially in populations with the highest burden of hypertension.
A complex interplay of genetics and the environment contributes to the risk of TOD. Heritability studies point to a substantial genetic component, with heritability estimates ranging from 0.17 to 0.76 [Citation14–18]. Familial clustering patterns in Framingham Heart Study reveal that individuals who have at least one parent with TOD had an increase in the odds of any type of TOD, even after controlling for hypertension status [Citation19]. Epigenetic mechanisms may shed light on the ways that genetics, the environment, and traditional risk factors contribute to the progression of TOD [Citation20,Citation21]. To date, only a handful of well-powered epigenome-wide association studies (EWAS) have investigated the association between DNA methylation and blood pressure or related organ damage [Citation22–24].
In this study, we investigated the association between DNA methylation, interrogated in peripheral blood leukocytes, and 5 TOD measures in a cohort of older African Americans using longitudinally collected data. We hypothesized that biological pathways involved in the association between DNA methylation and TOD could be unique to each trait or could act in a pleiotropic manner. Hence, to gain a better understanding of these biological mechanisms, we employed both univariate (single-trait) and multivariate (multi-trait) models. Additionally, we assessed whether the effects of CpG sites on TOD were mediated by the expression of nearby genes and we used Mendelian randomization (MR) to investigate causality.
Patients and methods
Study sample
Genetic Epidemiology Network of Arteriopathy (GENOA) is a community-based study in Rochester, MN and Jackson, MS that aims to identify genes influencing blood pressure [Citation25]. In the first phase of GENOA (Phase I: 1996–2001), sibships with at least two adults with clinically diagnosed essential hypertension before age 60 were recruited, and all siblings in the sibship were invited to participate regardless of hypertension status [Citation20]. Exclusion criteria included secondary hypertension, alcoholism or drug abuse, pregnancy, insulin-dependent diabetes mellitus, or active malignancy. In Phase I, a total of 1,583 non-Hispanic whites (Rochester, MN) and 1,854 African Americans (Jackson, MS) were enrolled. In the second phase (Phase II: 2001–2005), all participants were invited for a second examination. Eighty per cent of African Americans (N = 1,482) and 75% of non-Hispanic whites (N = 1,213) from Phase 1 returned. Demographic information, medical history, clinical characteristics, lifestyle factors, and blood samples were collected in each phase. This study includes African American participants who had their DNA methylation profiles measured in whole blood samples collected at Phase I. Measures of TOD were collected at Phase II and/or in an ancillary study of brain magnetic resonance imaging (MRI) conducted shortly after Phase II. Gene expression profiles were measured from lymphoblastoid cell lines made from blood samples collected after Phase 1 (median time from Phase 1 was 5.8 years). A total of 961 participants who had DNA methylation measurements at Phase I and returned for Phase II were included in the current study. Written informed consent was obtained from all participants and approval was granted by participating institutional review boards (University of Michigan, University of Mississippi Medical Center, and Mayo Clinic).
Target organ damage measures
Five TOD measurements were selected for the current analyses. Two traits, estimated glomerular filtration rate (eGFR) and urinary-albumin-creatinine ratio (UACR), measured diminishing kidney function. Left ventricular mass index (LVMI) and relative wall thickness (RWT) captured structural remodelling of the heart. White matter hyperintensity (WMH) captured altered areas of white matter in the brain using MRI. eGFR, UACR, LVMI, and RWT were assessed at Phase II. WMH was assessed in an ancillary study shortly after Phase II.
Estimated glomerular filtration rate (eGFR) and urine albumin-to-creatinine ratio (UACR)
Blood was drawn on the morning of the study visit after an overnight fast of at least 8 hours. Serum creatinine was assessed with enzymatic assays on a Hitachi 911 Chemistry Analyzer (Roche Diagnostics, Indianapolis, IN). The CKD-EPI formula was used to calculate the estimated glomerular filtration rate (eGFR) [Citation26]. A first-morning sample urine was collected on the morning of the study visit. Urine creatinine and urine albumin were assessed with enzymatic assays on a Hitachi 911 Chemistry Analyzer (Roche Diagnostics, Indianapolis, IN).
Left ventricular mass index (LVMI) and relative wall thickness (RWT)
Left ventricular mass (LVM) was estimated as previously described [Citation27]. Briefly, Doppler, two-dimensional (2D) and M-mode (2D-guided) echocardiograms were performed following a standardized protocol [Citation28]. Measurements were made at the echocardiography reading centre using a computerized review station equipped with a digitizing tablet and monitor overlay used for calibration and quantification (Digisonics, Inc., Houston, Texas). LVM was calculated using end-diastolic dimensions by an anatomically validated formula and LVMI was derived by indexing LVM to the height raised to the power of 2.7 [Citation29]. RWT was calculated as twice the posterior wall thickness divided by the left ventricular internal dimension [Citation28].
White matter hyperintensity (WMH)
Brain magnetic resonance imaging was performed using Signa 1.5 T MRI scanners (GE Medical Systems, Waukesha, WI, USA) and images were processed at Mayo Clinic [Citation30]. Total brain and WMH volume in the corona-radiata and periventricular zone were determined from axial fluid-attenuated inversion recovery (FLAIR) images [Citation31]. Brain scans with cortical infarctions were excluded from the analyses because of the distortion of the WMH volume estimates that would be introduced in the automated segmentation algorithm. For additional details, see Smith et al. [Citation32]. Models assessing WMH were adjusted for total intracranial volume (TIV).
Methylation measures
Genomic DNA from 1,106 African American participants from Phase I was extracted from stored peripheral blood leukocytes using AutoGen FlexStar (AutoGen, Holliston, MA). Bisulphite conversion was done using with the EZ DNA Methylation Kit (Zymo Research, Irvine, CA) and then DNA methylation was measured using the Infinium MethylationEPIC BeadChip. IDAT files were imported using the Minfi R package [Citation33] and sex mismatches and outliers were excluded using the shinyMethyl R package [Citation34]. Probes with detection p-value <10−16 were considered to be successfully detected [Citation35] and both samples and probes that failed a detection rate of at least 10% were removed. Samples with incomplete bisulphite conversion identified using the QCinfo function in the ENmix R package [Citation36] were removed. Sample identity was checked using the 59 SNP probes included in the EPIC BeadChip and mismatched samples were removed. Afterwards, the Noob method was used for individual background and dye-bias normalization [Citation37]. Since two types of probes were present on the EPIC BeadChip (Infinium I and Infinium II), we used the Regression on Correlated Probes (RCP) method to adjust for the probe-type bias in the data [Citation38]. Cross-hybridizing probes and those on sex chromosomes were removed using DMRcate R package [Citation39]. Methylation beta values were changed to M-values using logit transformation ( [Citation40]. Sample plate, sentrix ID and sample row were identified as batch effect variables using principle variance component analysis. White blood cell type proportions within the blood sample were estimated using Houseman’s method [Citation41]. Methylation M-values were adjusted for white blood cell type counts and batch effects using linear mixed modelling and the residuals were added to the mean. After quality control, a total of 1100 participants remained for further analysis.
Gene expression measures
Gene expression levels in GENOA African American participants were measured in Epstein-Barr virus (EBV) transformed lymphoblastoid cell lines using the Affymetrix Human Transcriptome Array 2.0. The Affymetrix Expression Console provided by Affymetrix was used for array quality control and all array images passed visual inspection. Affymetrix CEL files were normalized using the Robust Multichip Average algorithm in the Affymetrix Power Tool software [Citation42]. The Brainarray custom CDF version 19 was used to map the probes to genes [Citation43] and Combat [Citation44] was used to adjust batch effects and other technical covariates. A total of 17,616 autosomal protein-coding genes were available for analysis. A total of 1,205 samples remained after quality control.
Genotyping and imputation information for MR analysis
GENOA African American samples were genotyped on the Affymetrix® Genome-Wide Human SNP Array 6.0 platform, Illumina® Human1M-Duo, or Human660W-Quad BeadChips. Participants were excluded if they had a missing SNP call rate ≥0.05 or were an outlier ≥6 standard deviations (SD) from the mean of the first 10 genome-wide principal components from genotype data. SNPs were excluded if they had unknown chromosomal location, <95% call rate, or minor allele frequency less than 0.01. Imputation was performed separately by chip (Affymetrix or Illumina) using the 1000 Genomes Phase3 v5 reference panel and post-imputation comparison between the two groups revealed no substantial differences in genotype and allele frequencies. SNPs with imputation quality <0.8 were removed before MR analysis.
Covariates
Height was measured by stadiometer and weight by electronic balance. Body mass index (BMI) was calculated as weight in kilograms divided by the square of height in metres. Resting systolic blood pressure (SBP) and diastolic blood pressure (DBP) were measured by a random zero sphygmomanometer and a cuff appropriate for arm size. The second and third of three readings, after the participant sat for at least 5 minutes, were averaged for analysis [Citation45]. Information on current anti-hypertensive medication use was collected. Smoking was categorized as current, former, or never smokers. Type 2 diabetes (T2D) was defined as fasting serum glucose concentration >126 mg/dl or self-reported physician-diagnosed diabetes and current anti-diabetes medication use (insulin or hypoglycaemic agents).
Statistical analyses
Epigenome-wide association analysis of TOD
TOD traits were rank-based inverse normalized to ensure they follow a Gaussian distribution and outliers at four standard deviations from the mean were removed. Analyses were performed using the multivariate linear mixed model implemented in the Genome-wide Efficient Mixed Model Association (GEMMA) software [Citation46]. Random effects in the linear mixed models were used to adjust for familial relationship by using the genetic relatedness matrix. SNP-based heritability (h2) of the TOD traits was additionally estimated using GEMMA [Citation47]. TOD traits were investigated individually using univariate (single-trait) linear mixed models and jointly using a multivariate (multi-trait) linear mixed model. The multivariate method tests a hypothesis of a given CpG being associated with at least one of the TOD traits. We hypothesized that given the correlation between the TOD traits and potentially similar underlying biological mechanisms, some CpG sites will have true pleiotropic effects on the different TOD traits and using this approach will provide a better model fit. Additionally, using a multivariate approach will increase our statistical power, even if the methylation sites are not associated with all of the TOD traits at the univariate level [Citation47]. The multivariate model included eGFR, UACR, LVMI and RWT as outcome variables. WMH was not included as the sample size is significantly smaller (N = 539). Following multivariate regression, we tested the association between the significant sites identified in the multivariate model and WMH.
Univariate and multivariate models were adjusted for age at trait measurement, sex, time between measurements, smoking status, and four genetic principal components (PCs) (model 1). The minimally adjusted multivariate model (model 1) was considered our main analysis model. For significant CpG sites identified in the main analysis model, we examined the scatterplots of the CpGs against TOD to identify and potentially remove any extreme outliers or leverage points. We investigated attenuation of effects for the CpG sites identified in the main analysis by adjusting for SBP, DBP and antihypertensive medication use (model 2) and T2D status and BMI (model 3). To account for multiple comparisons, the Benjamini-Hochberg procedure was applied to control the false discovery rate (FDR) at a threshold of q <10% [Citation48].
Illumina documentation was used to annotate significant CpG sites with functional and regulatory features that mapped their genomic location relative to CpG islands and nearby genes. Additionally, we examined any overlap with regulatory elements reported by the Encyclopaedia of DNA Elements (ENCODE [Citation49]) and the Functional ANnoTation Of the Mammalian genome (FANTOM [Citation50]).
Mediation of CpG-TOD associations by gene expression levels
For significant CpG sites identified in the main analysis model, we selected genes within a 250 kb range with expression levels associated with the corresponding CpG at p-value <0.1 for formal mediation analysis. Linear mixed models were adjusted for familial relationship, age, sex, time between the measures, and four genetic PCs. UACR was natural log transformed as ln(UACR+1). The mediation R package was then used to test for mediation by proximal gene expression levels between each CpG site and TOD association [Citation51,Citation52]. To account for multiple testing, an FDR q <0.1 was applied across all CpG-mediator-TOD associations.
Mendelian randomization analysis of CpG-TOD associations
To assess whether the significant CpG sites identified contributed causally to TOD measures, we performed one-sample Mendelian randomization (MR) analysis. We opted to use one-sample MR given the limited availability of public methylation quantitative trait locus (mQTL) databases and TOD genome wide association studies (GWAS) in participants of African ancestry. After excluding SNPs with minor allele frequency <0.05, instrumental variables (IV) were drawn from SNPs within ± 1 Mb of each corresponding significant CpG site. RVTEST was used to assess the CpG-SNP association and identify eligible SNPs (FDR q < 0.1) [Citation53]. Clumping for independence using the Phase 3, version 5 of the 1000 Genomes dataset for Africans (AFR), at r2 of 0.1 and physical distance threshold of 100 kb, was done using PLINK v1.07 [Citation54]. Causal estimates were obtained using the inverse-variance weighted (IVW) model in the MendelianRandomization R package [Citation55] for each TOD trait. UACR was natural log transformed as ln(UACR+1). Models were adjusted for familial relationship, age at baseline, sex, and four genetic PCs. A p-value <0.05 was used. Additionally, we applied the MR Egger test to examine pleiotropy because IVW MR is invalid in the presence of horizontal pleiotropic effects of IVs [Citation56]. Finally, since both the IVW and Egger methods rely on summary data, we also calculated the causal estimates for the significant results using two-stage least squares regression [Citation57].
Replication analysis
For the CpG sites identified in the multivariate model 1 in GENOA, we sought to test the association between each CpG site and each TOD trait univariately in 614 African Americans from the Hypertension Genetic Epidemiology Network (HyperGEN) study. Like GENOA, families in HyperGEN were selected if sibships had ≥2 siblings who had been diagnosed with hypertension before age 60. DNA methylation was assayed from peripheral blood leukocytes (buffy coat) using the Infinium HumanMethylation450 BeadChip (450 K). Further details about HyperGEN can be found in the Supplemental Text.
Results
Descriptive statistics
Sample characteristics are described in . The mean age of the participants was 57.7 years at baseline and the majority of the participants were females. The mean follow-up time between phases was approximately 5 years. Mean SBP and DBP were 137.8 mmHg and 79.3 mmHg, respectively. Of the sample, about 12% were current smokers and 60% were never smokers. TOD heritability estimates ranged between 0.21 for WMH and 0.52 for LVMI. TOD traits were significantly correlated at p-value <0.05 (r range: −0.21 to 0.36, Table S1) with the exception of WMH and UACR (r = 0.08).
Table 1. Characteristics of participants in GENOAa (N = 961)
Epigenome-wide association analysis of TOD
Results from the minimally adjusted univariate and multivariate (4-trait: eGFR, UACR, LVMI, and RWT) epigenome-wide association analyses are shown in . The corresponding QQ plots are shown in Figure S1. RWT, LVMI, and UACR models each identified a single CpG site at FDR q < 0.1, while no site reached the statistical significance threshold for eGFR. Our initial analysis identified eight significant CpG sites associated with TOD at FDR q < 0.1 for our main multivariate model. However, upon inspection of scatterplots, we identified one CpG site (cg07235511) with an outlier that drove the association. This site was excluded from our report of findings, leaving a total of seven CpG sites in the minimally adjusted model (model 1).
Table 2. Statistically significant CpG sites associated with TOD traits at FDR q < 0.1 using univariate and multivariate models in GENOA
We extended the multivariate model 1 by adjusting for SBP, DBP, antihypertensive medication use, BMI, and T2D status. shows the results of the adjusted models for the seven CpG sites previously identified in model 1 (q < 0.1). Adjusting for hypertension (Model 2) attenuated the association for one CpG site (cg04816311) while the remaining six CpG sites remained significant. Adjustment for BMI and T2D further attenuated the association for three more CpG sites (cg21134922, cg09155024, and cg10254690). In addition, one new CpG site was found significant at the same threshold (Model 3, cg02204965, p-value = 5.02 × 10−7, FDR q = 0.098).
Table 3. Adjusted multivariate models for statistically significant CpG sites from Model 1 in GENOA
We next performed follow-up analysis for the seven identified CpG sites from multivariate Model 1 to [Citation1]: assess their association with the individual TOD traits univariately [Citation2], identify which combination of traits was driving the multivariate association, and [Citation3] examine the association between the identified CpG sites and WMH. The results of the univariate models for each of these sites with each TOD trait are shown in Table S2. In model 1, 4 of the 7 identified sites were significantly associated with two TOD traits using a Bonferroni adjusted cut-off of p-value < 0.007, while the other 3 were associated with only LVMI. Both cg04816311 and cg12661888 had a consistent effect in which increased methylation was associated with worse TOD outcomes (higher UACR, lower eGFR and/or higher LVMI). To aid in the clinical interpretation of the results, equivalent linear mixed models were run for each significant CpG site identified in the main analysis model and each of the TOD traits without normalization (Table S3). Individual TOD values were calculated at the mean – 1SD and mean +1SD M-value methylation levels for each CpG site. Adjusting for SBP, DBP, and antihypertensive medication use did not substantively change the results from Model 1, except that cg02264946 became associated with RWT in addition to LVMI. Further adjustment for BMI and T2D attenuated the association between cg04816311 and LVMI but not UACR. None of the seven CpG sites identified were associated with WMH at p-value <0.05 (Table S4).
Bioinformatic characterization of CpG sites and mediation of CpG-TOD associations by gene expression levels
We characterized significant CpG sites bioinformatically and by examining their association with proximal gene expression. Five out of the seven sites identified in the multivariate model were located in DNase hypersensitivity sites (Table S5), but no overlap was found with any FANTOM sites. Gene expression was derived from cell lines created from blood samples that were taken a minimum of 1.9 years after methylation measurement. Within the identified range of ± 250 kb of the 7 CpG sites identified, a total of 14 genes were marginally associated with nearby CpG methylation levels at p-value <0.1 . These genes were selected for the mediation analysis. Methylation at the examined CpG sites was associated with decreased expression of the SAFB2, UNCX, MORN3, HPD, and OAT genes as well as a number of long noncoding RNA molecules. Formal mediation analyses did not identify mediation by gene expression for any of the CpG-TOD traits associations at FDR q < 0.1; however, post hoc power calculation showed that statistical power for the mediation analysis was low (<50%).
Table 4. Associations between statistically significant CpG sites from multivariate Model 1 and nearby gene expression (± 250 kb, p-value < 0.1) in GENOA
Mendelian randomization analysis of CpG-TOD associations
shows the results of inverse-variance weighted models to assess whether the identified CpG sites were casually associated with each of the TOD traits. For four of the seven CpG sites, we identified significant independent SNPs within ±1 Mb that had a CpG-SNP FDR q < 0.1 (Table S6). eGFR was inversely influenced by methylation at cg10254690 near OAT (effect estimate = −5.75 mL/min/1.73 m2 and p-value = 0.027) and cg07660512 (effect estimate = −14.91 mL/min/1.73 m2 and p-value = 0.042). Methylation at cg04816311 near C7orf50 positively influenced eGFR (effect estimate = 8.84 mL/min/1.73 m2 and p-value = 0.014). The effect estimates were consistent in direction, but of greater magnitude, than those estimated by our baseline association analysis at the univariate level. Figure S2 (A-C) shows the plots of these causal estimates using the IVW method, which shows an overall consistent effect of the SNPs. The MR Egger test suggested no evidence of horizontal pleiotropy for all CpGs with the exception of the effect of cg10254690 on eGFR, where the MR Egger test showed no evidence of causality. Effect estimates and standard errors using two stage least squares regression were slightly larger than with the summary methods, but the p-values were similar (Table S7). The associations were only significant at a p-value threshold of 0.05, and none would reach statistical significance if corrected for multiple testing.
Table 5. Mendelian randomization results showing the inverse-variance weighted effects of multiple SNPs used as instrumental variables in the association of CpG sites and TOD traits in GENOA
Replication analysis
Sample characteristics of the HyperGEN replication cohort are shown in Table S8. HyperGEN participants were younger than GENOA participants with a mean age of 48.4 (SD = 11.1) years. Mean BMI and antihypertensive medication use were similar in both cohorts. HyperGEN had a lower percentage of diabetic participants and a higher percentage of smokers. Both UACR and LVMI were higher in HyperGEN compared to GENOA. Out of 7 CpG sites significant at FDR q < 0.1 in GENOA, only 3 sites (cg04816311, cg09155024, and cg10254690) were present on the 450 K chip used in HyperGEN. Table S9 shows the univariate associations for each of the three CpG sites with each of the 4 TOD traits. In the minimally adjusted model (Model 1), cg04816311 was associated with eGFR, LVMI, and RWT at p-value <0.05. Effect directions were consistent in that increased methylation was associated with increased TOD: higher LVMI in both cohorts, higher RWT in HyperGEN, and higher UACR in GENOA. However, it was also associated with lower eGFR in HyperGEN (an indicator of less TOD). The second CpG, cg09155024 was not associated with any of the TOD measures in HyperGEN. The third CpG, cg10254690, which was associated with LVMI in GENOA, had the same beta coefficient direction in HyperGEN but did not reach statistical significance (p-value = 0.18).
Discussion
The study identified seven CpG sites associated with 4 TOD measures in a cohort of older African Americans using a multivariate approach. The multivariate model had more power to detect significant CpG sites than the univariate models which detected a single CpG site for three of the TOD traits. This is in line with evidence from the literature on the power gains associated with multivariate over standard univariate analyses in genetic studies [Citation46,Citation47,Citation58–60]. Testing the significant CpG sites identified in the multivariate model at the univariate level showed that not all of the sites reached statistical significance. While counterintuitive, this is expected as unassociated traits in the multivariate analysis increase power if they are correlated with the associated trait [Citation46,Citation47]. Our initial hypothesis was that DNA methylation would have the same direction of effect across the different TOD traits; however, our findings show that this may not be true for all CpG sites. None of the sites identified were associated with WMH, which may indicate a different underlying mechanism for that trait or may be due to the relatively low variability of WMH in this sample.
Five out of the seven sites identified in the multivariate model were located in DNase hypersensitivity sites, which are regions of chromatin that are not highly condensed, rendering the chromatin exposed and accessible for transcription. After adjusting for hypertension-related covariates, six of these associations remained significant, and three remained significant when BMI and T2D were included. Although there was evidence for an association between the identified CpG sites and cis-gene expression, including both protein coding and long non-coding RNA molecules, none were statistically significant in formal mediation analyses. MR analysis provided some evidence of causality between at least two CpG sites and eGFR.
The CpG site that was identified in GENOA and replicated in HyperGEN, cg04816311 near C7orf50, was previously reported to be associated with T2D in Mexican-Americans and sub-Saharan Africans [Citation61,Citation62]. cg04816311 was also associated with BMI in African Americans [Citation63]. The direction of effect reported in the study by Meeks et al. showed that hypermethylation was observed at cg04816311 among T2D cases compared to the controls [Citation62]. In our study, a change in M-value methylation (mean −1SD to mean +1SD) was associated with an approximately 13 mg/g increase in UACR and 2.6 g/height2.7 increase in LVMI in GENOA. In the model adjusting for BMI and T2D, the methylation effect was attenuated and was no longer significant. Genetic variants in this locus were also found to be associated with lipid levels [Citation64], blood pressure [Citation65,Citation66], and longevity [Citation67] in GWAS studies.
Methylation at the cg10254690 site, which maps near the OAT gene promoter region, was associated with significantly decreased expression of OAT. Additionally, MR analysis using IVW suggested a causal effect equivalent to an approximately 6 mL/min/1.73 m2 decrease in eGFR for each 1 unit M-value increase in DNA methylation. The OAT gene codes for ornithine aminotransferase, a key mitochondrial enzyme found in the liver, intestine, brain, and kidney that converts arginine and ornithine into glutamate and GABA [Citation68]. Ornithine is involved in the urea cycle and synthesis of nitric oxide (NO). It is an important messenger molecule that regulates blood vessel dilation and has other thrombotic and inflammatory effects [Citation69,Citation70]. Studies suggest that OAT is involved in controlling the proliferation of several cell lines, including vascular smooth muscle cells, and it acts as a modulator of collagen synthesis and of extracellular matrix formation [Citation68,Citation71]. In addition, there is evidence that OAT is involved in metabolic reprogramming in activated T-cells by providing ornithine and α-KG [Citation72]. One previous study found a nominal association between DNA methylation at cg10254690 and cortisol stress reactivity [Citation73], and genetic variants in OAT were also associated with diastolic blood pressure [Citation74].
Other genes identified in the multivariate model were IFT43 and CATSPERD. IFT43, near cg12661888, encodes a subunit of the intraflagellar transport complex A, a multi-protein complex involved in cilia assembly and maintenance. This subunit is essential in regulating the Sonic Hedgehog signalling pathway, which is involved in regulating the growth, differentiation, and patterning of cells, especially during embryonic development [Citation75]. CATSPERD, near cg02264946, encodes an auxiliary subunit of sperm calcium channel pore-forming proteins required for the motility of spermatozoa and male fertility [Citation76]. cg03042953 near SSBP3, a single-stranded DNA-binding protein 3, was only significant for the RWT trait model. Genetic loci in the SSBP3 locus were associated with P wave duration [Citation77], blood urea nitrogen [Citation78], and BMI [Citation79,Citation80]. The single trait model for UACR identified cg04816311 near C7orf50, which was also significant in the multivariate outcome model. cg21134922, found significant for LVMI, was not near a gene.
Changes in methylation may not affect the expression of the genes immediately proximal to the CpG site, and CpG regulatory effects could be more distal or in trans (i.e., affecting genes on different chromosomes) [Citation81]. When we evaluated whether CpG sites were associated with transcriptional changes, a number of genes within the range of 250 kb showed decreased expression levels with increasing methylation. Previous literature identified DNA sequence variants in one of these genes, UNCX near cg04816311, to be associated with eGFR and other kidney-related traits in GWAS studies [Citation82,Citation83]. However, our study did not identify any significant mediation effects using formal mediation analysis. This could be attributed to the lack of statistical power and/or the different cell types used for the methylation-gene expression measures (EBV-transformed lymphocyte cell line for gene expression versus peripheral blood leukocytes for DNA methylation). There is conflicting evidence on whether the methylation patterns of these cell lines are similar, whether the EBV transformation preserves the gene expression profile, and whether that translates to a similar gene expression profile in both [Citation84–87].
This study is among the very few studies that assessed the association between DNA methylation and TOD. To the best of our knowledge, it the first study to employ a pleiotropy-informed analysis to examine epigenome-wide DNA methylation sites associated with correlated TOD traits. However, this study has a number of limitations. First, although we employed a longitudinal design, it is difficult to rule out reverse causality, especially for TOD traits where disease onset and duration is difficult to detect. In addition, although MR analyses allowed us to characterize the direction of the association, findings did not reach statistical significance when accounting for multiple testing, which could be attributed to small sample size and low power. None of the sites identified in this study replicated epigenome-wide significant CpG sites from the limited epigenome-wide studies available for TOD traits [Citation22–24].
In conclusion, our study addresses an important gap in the literature on the role of DNA methylation in TOD in African Americans. CpG sites mapped to important genes that can further our understanding of biological mechanisms underlying these conditions. Future studies are needed to replicate these findings and further investigate gene expression and epigenetic profiles in more relevant tissue types such as the kidney and the heart, in addition to investigating the associations in different racial and ethnic groups. Findings from such research may direct future efforts that target early detection and intervention.
List of abbreviations
BMI: body mass index; CpG: cytosine-phosphate-guanine; DBP: diastolic blood pressure; EBV: Epstein-Barr virus; eGFR: estimated glomerular filtration rate; ENCODE: Encyclopedia of DNA Elements; EWAS: epigenome wide association study; FANTOM: Functional ANnoTation Of the Mammalian genome; FDR q: false discovery rate; GEMMA: Genome-wide Efficient Mixed Model Association; GENOA: Genetic Epidemiology Network of Arteriopathy; GWAS: genome wide association study; LVMI: left ventricular mass index; MR: Mendelian randomization; MRI: magnetic resonance imaging; PC: principal component; RWT: relative wall thickness; SBP: systolic blood pressure; SD: standard deviation; SE: standard error; SNP: single nucleotide polymorphism; T2D: type 2 diabetes; TOD: target organ damage; UACR: urinary albumin-creatinine ratio; WMH: white matter hyperintensity.
Authors’ contributions
FA and JAS conceived and designed the study and wrote and revised the manuscript. FA conducted analyses, with assistance from SMR, WZ, XZ, and MK. WZ cleaned the methylation data, and LS cleaned the gene expression data. THM led the collection of target organ damage phenotypes. SLRK and LFB oversaw data collection at all phases of GENOA and assisted with designing the study. ACJ, NSC, HKT, MRI, and DKA supervised and conducted replication analysis in HyperGEN. JAS supervised the study and provided funding for analysis. All authors read and approved the final manuscript.
Declarations
The authors have nothing to declare.
Ethics approval and consent to participate
Written informed consent was obtained from all participants, and Institutional Review Boards at the University of Michigan, University of Mississippi Medical Center, and Mayo Clinic approved this study.
Consent for publication
Not applicable.
Availability of data and materials
The datasets used and/or analyzed in the current study are available from the corresponding author on reasonable request.
Disclosure of interest
The authors report no conflict of interests.
Supplemental Material
Download MS Word (24.5 KB)Supplemental Material
Download MS Word (916.8 KB)Acknowledgments
The authors wish to thank the staff and participants of the GENOA and HyperGEN studies.
Disclosure statement
No potential conflict of interest was reported by the authors.
Supplemental material
Supplemental data for this article can be accessed here
Additional information
Funding
References
- Whelton PK, Carey, RM, Aronow WS, et al. 2017 ACC/AHA/AAPA/ABC/ACPM/AGS/APhA/ASH/ASPC/NMA/PCNA guideline for the prevention, detection, evaluation, and management of high blood pressure in adults: a report of the American college of cardiology/American heart association task force on clinical practice guidelines. J Am Coll Cardiol. 2018;71(19):e127–e248.
- Cohuet G, Struijker-Boudier H. Mechanisms of target organ damage caused by hypertension: therapeutic potential. Pharmacol Ther. 2006;111(1):81–98.
- Norlander AE, Madhur MS, Harrison DG. The immunology of hypertension. J Exp Med. 2018;215(1):21–33.
- Sehestedt T, Jeppesen J, Hansen TW, et al. Risk prediction is improved by adding markers of subclinical organ damage to SCORE. Eur Heart J. 2010;31(7):883–891.
- Harbaoui B, Courand P-Y, Defforges A, et al. Cumulative effects of several target organ damages in risk assessment in hypertension. Am J Hypertens. 2016;29(2):234–244.
- Fowkes, FG, Murray GD, Butcher I, et al. Ankle brachial index combined with Framingham Risk Score to predict cardiovascular events and mortality: a meta-analysis. JAMA. 2008;300(2):197–208.
- Sundstrom J, Lind L, Ärnlöv J, et al. Echocardiographic and electrocardiographic diagnoses of left ventricular hypertrophy predict mortality independently of each other in a population of elderly men. Circulation. 2001;103(19):2346–2351.
- Carnethon MR, Pu J, Howard G, et al. Cardiovascular health in African Americans: a scientific statement from the American heart association. Circulation. 2017;136(21):e393–e423.
- Virani SS, Alonso A, Benjamin EJ, et al. Heart disease and stroke statistics-2020 update: a report from the American heart association. Circulation. 2020;141(9):e139–e596.
- Fox E, Taylor H, Andrew M, et al. Body mass index and blood pressure influences on left ventricular mass and geometry in African Americans: the atherosclerotic risk in communities (ARIC) study. Hypertension. 2004;44(1):55–60.
- Kizer JR, Arnett DK, Bella JN, et al. Differences in left ventricular structure between black and white hypertensive adults: the hypertension genetic epidemiology network study. Hypertension. 2004;43(6):1182–1188.
- Tarver-Carr ME, Powe NR, Eberhardt MS, et al. Excess risk of chronic kidney disease among African-American versus white subjects in the United States: a population-based study of potential explanatory factors. J Am Soc Nephrol. 2002;13(9):2363–2370.
- Choi AI, Rodriguez RA, Bacchetti P, et al. White/black racial differences in risk of end-stage renal disease and death. Am J Med. 2009;122(7):672–678.
- Freedman BI, Beck SR, Rich SS, et al. A genome-wide scan for urinary albumin excretion in hypertensive families. Hypertension. 2003;42(3):291–296.
- Sachdev PS, Thalamuthu A, Mather KA, et al. White matter hyperintensities are under strong genetic influence. Stroke. 2016;47(6):1422–1428.
- Assimes TL, Narasimhan B, Seto TB, et al. Heritability of left ventricular mass in Japanese families living in Hawaii: the SAPPHIRe study. J Hypertens. 2007;25(5):985–992.
- Peterson VR, Norton GR, Redelinghuys M, et al. Intrafamilial aggregation and heritability of left ventricular geometric remodeling is independent of cardiac mass in families of African ancestry. Am J Hypertens. 2015;28(5):657–663.
- Fox CS, Qiong Y, Cupples LA, et al. Genomewide linkage analysis to serum creatinine, GFR, and creatinine clearance in a community-based population: the Framingham heart study. J Am Soc Nephrol. 2004;15(9):2457–2461.
- Niiranen TJ, Lin H, Larson MG, et al. Familial clustering of hypertensive target organ damage in the community. J Hypertens. 2018;36(5):1086–1093.
- Zhang W, Song M, Qu, J, et al. Epigenetic Modifications in Cardiovascular Aging and Diseases. Circ Res. 2018;123(7):773–786.
- Zhong J, Agha G, Baccarelli AA. The role of DNA methylation in cardiovascular risk and disease: methodological aspects, study design, and data analysis for epidemiological studies. Circ Res. 2016;118(1):119–131.
- Richard MA, Huan T, Ligthart S, et al. DNA methylation analysis identifies loci for blood pressure regulation. Am J Hum Genet. 2017;101(6):888–902.
- Chu AY, Tin A, Schlosser P, et al. Epigenome-wide association studies identify DNA methylation associated with kidney function. Nat Commun. 2017;8(1):1286.
- Chouliaras L, Pishva E, Haapakoski R, et al. Peripheral DNA methylation, cognitive decline and brain aging: pilot findings from the Whitehall II imaging study. Epigenomics. 2018;10(5):585–595.
- Daniels PR, Kardia SLR, Hanis CL, et al. Familial aggregation of hypertension treatment and control in the genetic epidemiology network of arteriopathy (GENOA) study. Am J Med. 2004;116(10):676–681.
- Levey AS, Stevens LA, Schmid CH, et al. A new equation to estimate glomerular filtration rate. Ann Intern Med. 2009;150(9):604–612.
- Arnett DK, Meyers KJ, Devereux RB, et al. Genetic variation in NCAM1 contributes to left ventricular wall thickness in hypertensive families. Circ Res. 2011;108(3):279–283.
- Devereux RB, Roman MJ, Ganau A, et al. Cardiac and arterial hypertrophy and atherosclerosis in hypertension. Hypertension. 1994;23(6 Pt 1):802–809.
- Lang RM, Badano LP, Mor-Avi V, et al. Recommendations for cardiac chamber quantification by echocardiography in adults: an update from the American society of echocardiography and the European association of cardiovascular imaging. J Am Soc Echocardiogr. 2015;28(1):1–39 e14.
- Jack CR Jr., Twomey CK, Zinsmeister AR, et al. Anterior temporal lobes and hippocampal formations: normative volumetric measurements from MR images in young adults. Radiology. 1989;172(2):549–554.
- Jack CR Jr., O’Brien PC, Rettman DW, et al. FLAIR histogram segmentation for measurement of leukoaraiosis volume. J Magn Reson Imaging. 2001;14(6):668–676.
- Smith JA, Turner ST, Sun YV, et al. Complexity in the genetic architecture of leukoaraiosis in hypertensive sibships from the GENOA Study. BMC Med Genomics. 2009;2(1):16.
- Aryee MJ, Jaffe AE, Corrada-Bravo H, et al. Minfi: a flexible and comprehensive Bioconductor package for the analysis of Infinium DNA methylation microarrays. Bioinformatics. 2014;30(10):1363–1369.
- Fortin JP, Fertig E, Hansen K. shinyMethyl: interactive quality control of Illumina 450k DNA methylation arrays in R. F1000Res. 2014;3:175.
- Lehne B, Drong AW, Loh M, et al. A coherent approach for analysis of the illumina humanmethylation450 beadChip improves data quality and performance in epigenome-wide association studies. Genome Biol. 2015;16(1):37.
- Xu Z, Niu L, Li L, et al. ENmix: a novel background correction method for illumina humanmethylation450 beadChip. Nucleic Acids Res. 2016;44(3):e20.
- Fortin JP, Triche TJ Jr., Hansen KD. Preprocessing, normalization and integration of the illumina humanmethylationEPIC array with minfi. Bioinformatics. 2017;33(4):558–560.
- Niu L, Xu Z, Taylor JA. RCP: a novel probe design bias correction method for illumina methylation beadChip. Bioinformatics. 2016;32(17):2659–2663.
- Peters TJ, Buckley MJ, Statham AL, et al. De novo identification of differentially methylated regions in the human genome. Epigenetics Chromatin. 2015;8(1):6.
- Du P, Zhang X, Huang -C-C, et al. Comparison of Beta-value and M-value methods for quantifying methylation levels by microarray analysis. BMC Bioinformatics. 2010;11(1):587.
- Houseman EA, Accomando WP, Koestler DC, et al. DNA methylation arrays as surrogate measures of cell mixture distribution. BMC Bioinformatics. 2012;13(1):86.
- Irizarry RA, Bolstad BM, Collin F, et al. Summaries of Affymetrix GeneChip probe level data. Nucleic Acids Res. 2003;31(4):e15.
- Dai M, Wang P, Boyd AD, et al. Evolving gene/transcript definitions significantly alter the interpretation of GeneChip data. Nucleic Acids Res. 2005;33(20):e175.
- Johnson WE, Li C, Rabinovic A. Adjusting batch effects in microarray expression data using empirical Bayes methods. Biostatistics. 2007;8(1):118–127.
- Turner ST, Kardia SLR, Mosley TH, et al. Influence of genomic loci on measures of chronic kidney disease in hypertensive sibships. J Am Soc Nephrol. 2006;17(7):2048–2055.
- Zhou X, Stephens M. Efficient multivariate linear mixed model algorithms for genome-wide association studies. Nat Methods. 2014;11(4):407–409.
- Stephens M, Emmert-Streib F. A unified framework for association analysis with multiple related phenotypes. PloS One. 2013;8(7):e65245.
- Benjamini Y, Hochberg Y. Controlling the false discovery rate: a practical and powerful approach to multiple testing. J R Stat Soc: Series B. 1995;57(1):289–300.
- Consortium EP. An integrated encyclopedia of DNA elements in the human genome. Nature. 2012;489(7414):57–74.
- Severin J, Waterhouse AM, Kawaji H, et al. FANTOM4 EdgeExpressDB: an integrated database of promoters, genes, microRNAs, expression dynamics and regulatory interactions. Genome Biol. 2009;10(4):R39.
- Tingley D, Yamamoto T, Hirose K, et al. mediation: R package for causal mediation analysis. J Stat Softw. 2014;59(5):38.
- Preacher KJ, Hayes AF. SPSS and SAS procedures for estimating indirect effects in simple mediation models. Behav Res Methods Instrum Comput. 2004;36(4):717–731.
- Zhan X, Hu Y, Li B, et al. RVTESTS: an efficient and comprehensive tool for rare variant association analysis using sequence data: table 1. Bioinformatics. 2016;32(9):1423–1426.
- Purcell S, Neale B, Todd-Brown K, et al. PLINK: a tool set for whole-genome association and population-based linkage analyses. Am J Hum Genet. 2007;81(3):559–575.
- Yavorska OO, Burgess S. MendelianRandomization: an R package for performing Mendelian randomization analyses using summarized data. Int J Epidemiol. 2017;46(6):1734–1739.
- Hemani G, Bowden J, Davey Smith G. Evaluating the potential role of pleiotropy in Mendelian randomization studies. Hum Mol Genet. 2018;27(R2):R195–R208.
- Kang H, Jiang Y, Zhao Q, et al. Ivmodel: an R package for inference and sensitivity analysis of instrumental variables models with one endogenous variable. arXiv e-prints. 2020. arXiv:2002.08457
- Korte A, Vilhjálmsson BJ, Segura V, et al. A mixed-model approach for genome-wide association studies of correlated traits in structured populations. Nat Genet. 2012;44(9):1066–1071.
- O’Reilly PF, Hoggart CJ, Pomyen Y, et al. MultiPhen: joint model of multiple phenotypes can increase discovery in GWAS. PLoS One. 2012;7(5):e34861.
- Ferreira MA, Purcell SM. A multivariate test of association. Bioinformatics. 2009;25(1):132–133.
- Kulkarni H, Kos MZ, Neary J, et al. Novel epigenetic determinants of type 2 diabetes in Mexican-American families. Hum Mol Genet. 2015;24(18):5330–5344.
- Meeks KAC, Henneman P, Venema A, et al. Epigenome-wide association study in whole blood on type 2 diabetes among sub-Saharan African individuals: findings from the RODAM study. Int J Epidemiol. 2019;48(1):58–70.
- Demerath EW, Guan W, Grove ML, et al. Epigenome-wide association study (EWAS) of BMI, BMI change and waist circumference in African American adults identifies multiple replicated loci. Hum Mol Genet. 2015;24(15):4464–4479.
- Ligthart S, Vaez A, Hsu Y-H, et al. Bivariate genome-wide association study identifies novel pleiotropic loci for lipids and inflammation. BMC Genomics. 2016;17(1):443.
- Evangelou E, Warren HR, Mosen-Ansorena D, et al. Genetic analysis of over 1 million people identifies 535 new loci associated with blood pressure traits. Nat Genet. 2018;50(10):1412–1425.
- Takeuchi F, Akiyama M, Matoba N, et al. Interethnic analyses of blood pressure loci in populations of East Asian and European descent. Nat Commun. 2018;9(1):5052.
- Yashin AI, Wu D, Arbeev KG, et al. Joint influence of small-effect genetic variants on human longevity. Aging (Albany NY). 2010;2(9):612–620.
- Ginguay A, Cynober L, Curis E, et al. Ornithine aminotransferase, an important glutamate-metabolizing enzyme at the crossroads of multiple metabolic pathways. Biology (Basel). 2017;6(1):18.
- Mori M, Gotoh T, Nagasaki A, et al. Regulation of the urea cycle enzyme genes in nitric oxide synthesis. J Inherit Metab Dis. 1998;21(Suppl 1):59–71.
- Huynh NN, Chin-Dusting J. Amino acids, arginase and nitric oxide in vascular health. Clin Exp Pharmacol Physiol. 2006;33(1–2):1–8.
- Durante W, Liao L, Reyna SV, et al. Transforming Growth Factor-β 1 Stimulatesl -Arginine Transport and Metabolism in Vascular Smooth Muscle Cells. Circulation. 2001;103(8):1121–1127.
- Wang R, Dillon C, Shi L, et al. The transcription factor Myc controls metabolic reprogramming upon T lymphocyte activation. Immunity. 2011;35(6):871–882.
- Houtepen LC, Vinkers CH, Carrillo-Roa T, et al. Genome-wide DNA methylation levels and altered cortisol stress reactivity following childhood trauma in humans. Nat Commun. 2016;7(1):10967.
- Parmar PG, Taal HR, Timpson NJ, et al. International genome-wide association study consortium identifies novel loci associated with blood pressure in children and adolescents. Circ Cardiovasc Genet. 2016;9(3):266–278.
- Liem KF Jr., Ashe A, He M, et al. The IFT-A complex regulates Shh signaling through cilia structure and membrane protein trafficking. J Cell Biol. 2012;197(6):789–800.
- Chung JJ, Navarro B, Krapivinsky G, et al. A novel gene required for male fertility and functional CATSPER channel formation in spermatozoa. Nat Commun. 2011;2:153.
- Christophersen IE, Magnani JW, Yin X, et al. Fifteen genetic loci associated with the electrocardiographic P wave. Circ Cardiovasc Genet. 2017;10(4). DOI:10.1161/CIRCGENETICS.116.001667.
- Wuttke M, Li Y, Li M, et al. A catalog of genetic loci associated with kidney function from analyses of a million individuals. Nat Genet. 2019;51(6):957–972.
- Locke AE, Kahali B, Berndt SI, et al. Genetic studies of body mass index yield new insights for obesity biology. Nature. 2015;518(7538):197–206.
- Pulit SL, Stoneman C, Morris AP, et al. Meta-analysis of genome-wide association studies for body fat distribution in 694 649 individuals of European ancestry. Hum Mol Genet. 2019;28(1):166–174.
- Kennedy EM, Goehring GN, Nichols MH, et al. An integrated -omics analysis of the epigenetic landscape of gene expression in human blood cells. BMC Genomics. 2018;19(1):476.
- Pattaro C, Teumer A, Gorski M, et al. Genetic associations at 53 loci highlight cell types and biological pathways relevant for kidney function. Nat Commun. 2016;7:10023.
- Hellwege JN, Velez Edwards DR, Giri A, et al. Mapping eGFR loci to the renal transcriptome and phenome in the VA Million Veteran Program. Nat Commun. 2019;10(1):3842.
- Sun YV, Turner ST, Smith JA, et al. Comparison of the DNA methylation profiles of human peripheral blood cells and transformed B-lymphocytes. Hum Genet. 2010;127(6):651–658.
- Aberg K, Khachane AN, Rudolf G, et al. Methylome-wide comparison of human genomic DNA extracted from whole blood and from EBV-transformed lymphocyte cell lines. Eur J Hum Genet. 2012;20(9):953–955.
- Hansen KD, Sabunciyan S, Langmead B, et al. Large-scale hypomethylated blocks associated with Epstein-Barr virus–induced B-cell immortalization. Genome Res. 2014;24(2):177–184.
- Çalışkan M, Cusanovich DA, Ober C, et al. The effects of EBV transformation on gene expression levels and methylation profiles. Hum Mol Genet. 2011;20(8):1643–1652.