ABSTRACT
DNA methylation (DNAm) age may reflect age-related variations in biological changes and abnormalities related to ageing. DNAm age acceleration measures have been associated with a number of cancers, but to our knowledge, have not been examined in relation to pancreatic cancer risk or survival. DNAm levels in leukocytes of prediagnostic blood samples of 393 pancreatic cancer cases and 431 matched controls, pooled from three large prospective cohort studies, were used to estimate DNAm age, epigenetic age acceleration (AA), and intrinsic epigenetic age acceleration (IEAA) metrics. Logistic regression and Cox proportional hazard regression models were used to examine the relationship between the various AA and IEAA metrics and pancreatic cancer risk and survival, respectively. The results showed that pancreatic cancer risk was significantly increased across all IEAA metrics, ranging from 83% to 95% increased risk when comparing the third and highest quartiles to the lowest quartile of IEAA. Consistent with these findings, the results from multivariate spline regression analyses showed non-linear relationships between all three IEAA metrics and pancreatic cancer risk with apparent threshold effect including two turning points at minimal and at maximal risks, respectively. There is no evidence of a significant association between pancreatic cancer survival and any of the epigenetic AA or IEAA metrics. Our results indicate DNAm age acceleration, measured in blood prior to cancer diagnosis, is associated with an increased risk of pancreatic cancer in a complex nonlinear, dose–response manner. Epigenetic IEAA metrics may be a useful addition to current methods for pancreatic cancer risk prediction.
Background
DNA methylation (DNAm) clocks are derived from epigenetic DNAm markers that are strongly correlated with chronological age [Citation1]. DNA methylation ageing clocks capture age-related epigenetic variations, which can be divided into intrinsic (or intra-cellular) and extrinsic (or broadly within-tissue and external) aspects of the ageing process [Citation2]. Intrinsic DNAm age is independent of age-related changes in blood-cell composition, while extrinsic DNAm age incorporates age-related changes in blood cell composition in the clocks’ algorithm.
Deviations between DNAm age and chronological age are commonly referred to as ‘age acceleration,’ or positive deviation between DNAm age and chronological age. Numerous cohort studies have reported that subjects with age acceleration were at an increased risk for all-cause mortality after controlling for known risk factors [Citation2–7]. Furthermore, a study showed that the offspring of semi-supercentenarians (individuals who reached an age of 105–109 y) have a lower epigenetic age than age-matched controls [Citation8]. Based on these findings, it has been hypothesized that epigenetic processes play a role in healthy ageing, and DNAm age captures some aspects of biological age. DNAm is thus thought to be a marker of susceptibility to disease and is associated with multiple health outcomes [Citation9].
DNAm age may reflect age-related variations in the biological changes and abnormalities related to cancer development. Observational studies have reported that age acceleration estimated using different DNAm clocks is associated with increased all cancer risk and shorter cancer survival independent of major health risk factors [Citation10,Citation11]. Consistent findings have been reported in studies that examined the association between DNAm age acceleration and specific types of cancer. In a pooled analysis of seven cohort studies, age acceleration had stronger associations with the risk of kidney cancer and B-cell lymphoma than colorectal, gastric, lung, prostate, and urothelial cancer [Citation10]. Intrinsic DNAm age acceleration estimated by Horvath’s clock (IEAA Horvath) was significantly associated with an increased risk of lung cancer in the Women’s Health Initiative (WHI) cohort [Citation12]. IEAA Horvath was also associated with an increased risk of breast cancer in a nested case–control study embedded in the European Prospective Investigation into Cancer and Nutrition (EPIC) cohort [Citation13]. Consistently, in a recent case–cohort study, significant positive associations were observed between age acceleration estimated by three different DNAm clocks (Hannum, Horvath, and PhenoAge) and risk of breast cancer [Citation14].
To our knowledge, no study has examined epigenetic clocks in relation to pancreatic cancer risk or survival. Prognosis of pancreatic cancer is poor, 91% of pancreatic cancer patients succumb to their disease within 5 y of diagnosis [Citation15], largely due to most cases being diagnosed at late stages. Identifying molecular biomarkers for pancreatic cancer risk prediction may improve early detection of this highly fatal disease. In this study, we assessed the associations of DNAm age acceleration and pancreatic cancer risk using incident pancreatic cancer cases and matched controls identified from three large cohort studies in the United States.
Methods
Study sample and blood collection
A pooled analysis using prediagnostic blood samples from pancreatic cancer cases and matched controls selected from the Nurses’ Health Study (NHS) [Citation16], the Physician’s Health Study (PHS) [Citation17], and the Health Professionals Follow-up Study (HPFS) [Citation18] was conducted. All blood specimens (for each cohort) were drawn by participants and mailed overnight, and upon receipt, the samples were aliquotted and frozen for future analyses.
All pancreatic cancer cases included in this nested case–control analysis were identified through self-reports, death records, or cancer registry searches. During follow-up (through 2018 for HPFS and NHS, and through 2010 for PHS), 403 incident cases were confirmed to have pancreatic cancer among the participants who provided blood samples. A control subject was 1:1 matched to each case on the cohort (which also matches on sex), age (±1 y), date of blood draw (±3 months), smoking (never, former, current), and race (White/other). Due to low DNA concentrations, some of the samples were removed after data processing of the arrays (see Supplementary Methods). As such, the initial 1:1 matching was not always conserved and resulted in some cases and controls with no matched pair. The final dataset consisted of 393 incidence pancreatic cases and 431 matched controls with blood samples collected from cases between 6 months and 26 y prior to cancer diagnosis.
DNA extraction and DNAm processing
DNA extracted from buffy coats was bisulphite-treated and DNA methylation was measured with the Illumina Infinium MethylationEPIC BeadChip [850 K] arrays (Illumina, Inc., CA, USA). Details concerning the preprocessing and quality control of the DNA methylation data are provided in the Supplementary Methods.
Methylation age and age acceleration estimation
Three DNAm clocks (Hannum [Citation19], Horvath [Citation20], and PhenoAge [Citation21]) were used to estimate subjects’ DNAm age and age acceleration. For each of the three DNAm clocks, DNAm age acceleration (AA) was defined by regressing DNAm age on chronologic age and calculating the difference between the observed chronological age and the fitted DNAm age (i.e., the residual). Additionally, intrinsic epigenetic age acceleration (IEAA) metrics were calculated using the residuals from the linear regression fit to DNAm age on chronologic age, adjusted for estimated blood cell composition [Citation22,Citation23]. Subjects with an absolute value of the age acceleration estimate greater than 3 standard deviations (SDs) from the mean were excluded (Supplementary Table 1).
Statistical analysis
gAll statistical analyses and plotting were performed in R 3.6.1 (R Core Team, 2018). Two-tailed P values ≤0.05 were considered statistically significant. Spearman’s rank correlation was used to calculate the correlations between age acceleration metrics. To examine the association with pancreatic cancer, a series of conditional logistic regression analyses were performed. All conditional logistic regression models (including all pairs of matched case and control) were further adjusted for body mass index (BMI). Quartiles were assigned according to the distribution of each DNAm age acceleration metric calculated among controls. Participants with missing covariate data were excluded from the analyses (<3.4% for all models). Similar results were observed when using unconditional logistic regression models, adjusting for all matching factors (chronological age, cohorts, race, date of blood draw, smoking status) and BMI (Supplementary Table 2).
Potential nonlinear relationships between each DNAm age acceleration metric (as a quantitative variable) and pancreatic cancer risk was evaluated using spline regression models, implemented in mgcv (the mixed GAM [generalized additive model] computation vehicle with automatic smoothness) package (v1.8–31; Simon Wood, 2019) [Citation24]. The degree of smoothness of model terms is estimated as part of fitting automatically based on maximal likelihood to determine a ‘best-fit’ model. This method uses a thin plate spline basis as the default basis for its smooth terms and does not require prespecified knots. When the degree of freedom is equal to 1, the model is the same as a least-squares linear regression model. All models adjusted for chronological age, sex, race, date of blood draw, smoking status, and BMI. Stratified analyses were also performed to examine whether the relationships differed across the following subgroups – sex (males or females), age groups (≤65 or >65 y), smoking status (current, former, or never smokers), and BMI categories (<25, ≥25 kg/m2) – by adding smooth-factor (subgroup) interaction terms to the spline regression models.
The association between survival time (i.e., time from diagnosis to death or censoring) and age acceleration among pancreatic cancer cases was examined using multivariable Cox proportional hazard models. Models were adjusted for cohort, chronological age at blood draw, race, smoking status, and BMI. Subjects with survival time equal to zero (n = 51) were excluded as these subjects had no medical records and were identified using the National Death Index.
Results
Study population
Participants in the nested case–control study were diagnosed with pancreatic cancer an average of 13 y (range 6 months to 26 y) after providing a blood sample. About 40% of the study participants were never smokers, 45% former smokers, and 15% current smokers. About half of the study participants were overweight or obese. For all three AA metrics, there were more pancreatic cancer cases with accelerated epigenetic age than the matched controls. Fewer female participants had accelerated epigenetic age than male participants ().
Table 1. Baseline characteristics of the study population, stratified by epigenetic age acceleration or decelerationa.
The correlations between the three DNAm age and chronological age were moderate for all three epigenetic clocks (r = 0.42, 0.40, and 0.32 for Hannum, Horvath, and PhenoAge, respectively) (Supplementary Figure 1). Correlations between each pair of age acceleration (AA) or intrinsic age acceleration (IEAA) metrics were high (r ranged from 0.65 to 0.98) in our study sample (Supplementary Figure 2). The distributions of epigenetic AA and IEAA metrics among pancreatic cancer cases and their matched controls are shown in Supplementary Figure 3. Consistently, the medians of all three epigenetic AA and three IEAA metrics were above zero (indicating accelerated age) among pancreatic cancer cases, while they were below zero (indicating decelerated age) among the matched controls.
DNAm age acceleration and pancreatic cancer risk
Logistic regression analyses were performed to examine the associations between epigenetic age acceleration and pancreatic cancer risk, adjusted for chronological age, cohorts, date of blood draw, race, smoking status, and BMI. Except for AA Horvath, all other age acceleration metrics (AA or IEAA Hannun, IEAA Horvath, and AA, or IEAA PhenoAge) showed significant associations with pancreatic cancer risk () The strongest associations were observed for all IEAA metrics when comparing participants in the third quartile to those in the lowest quartile (the cut-offs for these metrics varied); pancreatic cancer risk significantly increased, ranging from 89% increased risk for IEAA Horvath metric (OR = 1.89; 95% CI 1.23, 2.91; p = 0.004) to 95% increased risk for IEAA Hannum metric (OR = 1.95; 95% CI 1.24, 3.07; p = 0.004) and IEAA PhenoAge metric (OR = 1.95; 95% CI 1.26, 3.01; p = 0.003). Comparing the highest to the lowest quartile, pancreatic cancer risks were increased by 73% (OR = 1.73; 95% CI 1.11, 2.70; p = 0.015) and 83% (OR = 1.83; 95% CI 1.15, 2.90; p = 0.01) for AA and IEAA Hannum metrics, respectively, and increased by 75% for IEAA PhenoAge metric (OR = 1.75; 95% CI 1.11, 2.76; p = 0.016) (). Consistent with these findings, the multivariate spline regression results showed non-linear relationships between all three IEAA metrics and pancreatic cancer risk with apparent threshold effects with two turning points at minimal and at maximal risks, respectively. In general, the pancreatic cancer risk appeared to decrease with age acceleration to reach a minimal risk, and then the risks increased with age acceleration to reach a plateau or maximal risk at higher age acceleration (). Additionally, IEAA Horvath metric showed a possible downward trend after reaching a maximal risk. It should be noted that all risk estimates were less certain (i.e., larger standard errors) at both ends of the spline regression curve (i.e., larger decelerated and accelerated ages) due to few observations with values of IEAA less than −10 or greater than 10.
Figure 1. Forest plot of the associations between age acceleration metrics (per quintile) and pancreatic cancer risk
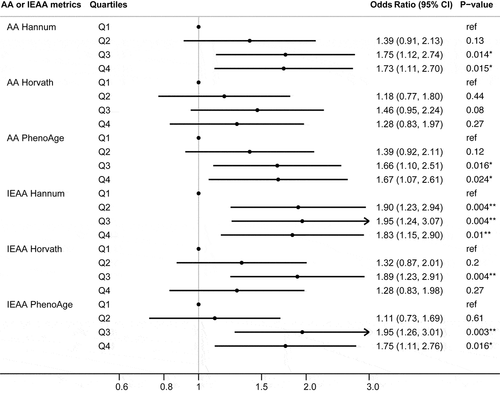
Figure 2. Results from the spline regression analyses of the associations between each IEAA metric and pancreatic cancer risks (panel A, IEAA Hannum; panel B, IEAA Horvath; panel C, IEAA PhenoAge)
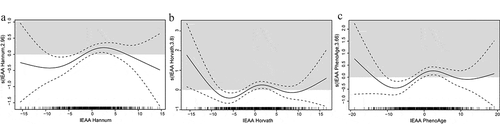
Stratified spline regression analyses
Stratified spline regression analyses were conducted to examine the associations between IEAA metrics and pancreatic cancer risk according to participants’ characteristics. There was a significant linear positive relationship between IEAA PhenoAge and pancreatic cancer risk among never smokers (p = 0.05), but the relationships were nonlinear among former and current smokers. For IEAA Hannum and IEAA Horvath metrics, their nonlinear relationships with pancreatic cancer risk were not significantly affected by smoking status (). For all three IEAA metrics, their relationships with pancreatic cancer risk differed by overweight status (BMI >25 kg/m2), in that the linear or nonlinear relationships were stronger, statistically, among overweight (IEAA Hannum: p = 0.05; IEAA Horvath: p = 0.02; IEAA PhenoAge: p = 0.09) than normal weight groups (). The relationships between age groups and pancreatic cancer risk were similar for IEAA Hannum and IEAA PhenoAge metrics, but a stronger nonlinear relationship was shown among the elderly group (age >65 y) for IEAA Horvath metric (p = 0.08) (Supplementary Figure 4). Both IEAA Horvath and IEAA PhenoAge metrics showed stronger nonlinear relationships with pancreatic cancer risk among men (IEAA Horvath: p = 0.01; IEAA PhenoAge: p = 0.05), while the relationships were similar by sex for IEAA Hannum metric (Supplementary Figure 5).
Figure 3. Stratified spline regression analyses of the associations between each IEAA metric and pancreatic cancer risks by smoking status (panel A, Never smokers; panel B, Former smokers; panel C, Current smokers)
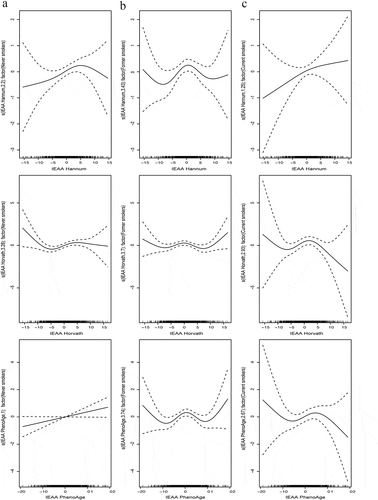
Figure 4. Stratified spline regression analyses of the associations between each IEAA metric and pancreatic cancer risks by BMI groups (panel A, BMI ≤ 25 kg/m2; panel B, BMI > 25 kg/m2)
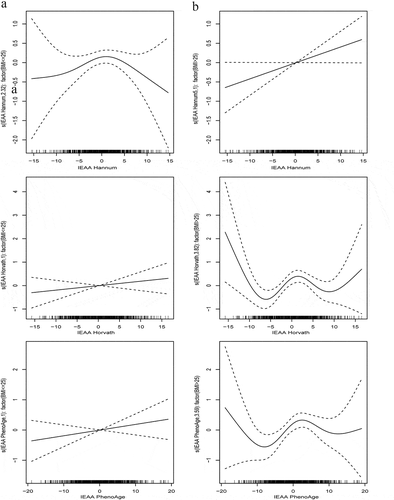
DNAm age acceleration and pancreatic cancer survival
We conducted case-only analyses to examine the associations between DNAm age acceleration and pancreatic cancer survival. Hazard ratios for pancreatic cancer survival were similar across all epigenetic AA and IEAA metrics; overall, we observed little evidence of any significant association between pancreatic cancer survival and any of the epigenetic AA or IEAA metrics (; Supplementary Figure 6).
Table 2. Associations between epigenetic age acceleration metrics (higher quintile vs. the lowest quintile) and pancreatic cancer survival in pancreatic cancer cases
Discussion
Our results indicate DNAm age acceleration is associated with an increased risk of pancreatic cancer that is independent of several key risk factors for cancer including chronological age. We found positive associations for all three epigenetic clocks (Hannum, Horvath, and PhenoAge), with stronger associations for intrinsic epigenetic age acceleration (IEAA) metrics suggesting that blood cell composition is a weak confounder in our analyses. Moreover, our findings suggest that the relation between DNAm age acceleration and pancreatic cancer risk occurs in a complex nonlinear dose–response manner.
While prior studies have examined the epigenetic clock associations with cancer risk [Citation10–14], only one study examined PhenoAge clock in relation to breast cancer risk [Citation14] and another assessed nonlinear relationships between Hannum or Horvath IEAA and all cancer incidence and mortality [Citation11]. Analysing multiple epigenetic age acceleration over time, the latter study found complex linear and nonlinear, dynamic time-dependent relationship with all cancer incidence and mortality [Citation11]. We did not find a significant association between age acceleration and pancreatic cancer survival. It should be noted that the prognosis of pancreatic cancer is poor (>90% of pancreatic cancer cases died within 2.5 y after diagnosis) so associations between age acceleration and pancreatic cancer survival would be hard to detect, if any exist (Supplementary Figure 6)
The three epigenetic clocks have only a few CpGs in common, but the AA or IEAA metrics were highly correlated with each other in our study sample. The correlations between these epigenetic AA or IEAA metrics were moderate to high (r ranged from 0.39 to 0.98) in a case–cohort study of breast cancer risk [Citation14]. Unlike previous studies (none of which included pancreatic cancer cases) [Citation10,Citation13,Citation14], the correlations between the epigenetic age estimated by the three epigenetic clocks and chronological age were only moderate in our study sample. The PhenoAge clock showed the smallest correlation with chronological age, which is not surprising because PhenoAge clock was trained on age-related and disease phenotypes in addition to chronological age [Citation21]. While different cancers are biologically distinct from each other, epigenetic age acceleration may not be cancer specific. The process of carcinogenesis is almost universally associated with both inflammation and activation of immune senescence pathways [Citation25,Citation26]. The fact that our findings also are consistent with those of the only prior study that examined the associations between age acceleration estimated by Hannum, Horvath, or PhenoAge clocks and risk of breast cancer [Citation14] may also suggest that age acceleration reflects a more general cancer-associated biological process.
We observed heterogeneous associations, varying by participants’ characteristics and IEAA metrics in the stratified analyses. These findings suggest that the three epigenetic clocks (Hannum, Horvath, and PhenoAge) capture different aspects of biological ageing and abnormal changes to cancer development, although the biological implications of the epigenetic clock are not well understood. Nonetheless, studies have consistently shown that BMI or obesity is positively associated with Hannum and Horvath age acceleration metrics [Citation27–30], which support our stratified analysis findings by overweight status. Since studies have identified many common genetic variants associated with BMI or obesity [Citation31], these findings point to a genetic component of these epigenetic clocks [Citation10]. Furthermore, our stratified analyses showed that associations between IEAA PhenoAge and pancreatic risk are modified by smoking status. These results suggest that the PhenoAge clock may be most sensitive to DNAm changes related to smoking than the Hannum and Horvath clocks. Several studies have shown that these epigenetic clocks are affected by lifestyle factors including smoking [Citation21,Citation27,Citation30,Citation32]. Additionally, compared to five different DNAm ageing clocks (including Hannum and Horvath), PhenoAge clock was shown to have stronger associations with all-cause mortality, smoking status, leukocyte telomere length, naive CD8 + T cells, and CD4 + T cells [Citation21].
Lastly, our results overall suggest that IEAA metrics may be more suitable for evaluating the associations with pancreatic cancer risks than AA metrics. Age-related DNAm signatures may be influenced by tissue’s cell composition, which is altered with age and may partially mask age acceleration associations to disease risk. IEAA metrics are not confounded by the blood cell compositions by definition.
Our study has several strengths. We employed a prospective study design by using prediagnostic bloods of incidence pancreatic cancer cases and matched controls from three large cohort studies in the United States. Our analyses minimize confounding through matching and statistical adjustments. Although we find evidence that age acceleration is associated with an increased risk of pancreatic cancer, our study has several limitations. First, our findings do not provide mechanistic insights into how these age-related changes influence pancreatic cancer risks. Second, our study population is comprised of mostly Caucasians so our results may not be generalizable to other races or ethnicity. Lastly, our stratified spline analyses may be underpowered due to small sample sizes in some subgroups and may have spurious findings due to multiple testing.
In sum, our study is first prospective study to examine Hannum, Horvath, and PhenoAge epigenetic clocks in relation to pancreatic cancer risk or survival, and we find that DNAm age acceleration is associated with an increased risk of pancreatic cancer in a complex nonlinear dose–response manner. Epigenetic IEAA metrics may be used to improve pancreatic cancer risk prediction when used in concert with known disease risk factors. Little is known regarding the trajectories of these epigenetic clock in relation to cancer risk. To build an evidence base to support epigenetic age as a biomarker for cancer early detection, future prospective studies should evaluate the changes in DNAm age (ideally at multiple time points) relating to cancer development.
Supplemental Material
Download Zip (1.9 MB)Disclosure statement
Dr Kelsey is a founder and scientific advisor for Celintec, which had no role in this work. All other authors (MC, MR, NZ, DK, IDV, and DSM) have no conflict of interest to disclose).
Supplementary material
Supplemental data for this article can be accessed here.
Data availability statement
All data from this study have been deposited in dbGAP [‘DNA Methylation Markers and Pancreatic Cancer Risk in 3 Cohort Studies (NHS, PHS, HPFS)’ phs001917.v1.p1]. https://www.ncbi.nlm.nih.gov/projects/gap/cgi-bin/study.cgi?study_id=phs001917.v1.p1
Additional information
Funding
References
- Bell CG, Lowe R, Adams PD, et al. DNA methylation aging clocks: challenges and recommendations. Genome Biol. 2019;20(1):249. Epub 2019/ 11/27. PubMed PMID: 31767039; PubMed Central PMCID: PMCPMC6876109.
- Chen BH, Marioni RE, Colicino E, et al. DNA methylation-based measures of biological age: meta-analysis predicting time to death. Aging (Albany NY). 2016;8(9):1844–1865. Epub 2016/ 10/01. PubMed PMID: 27690265; PubMed Central PMCID: PMCPMC5076441.
- Christiansen L, Lenart A, Tan Q, et al. DNA methylation age is associated with mortality in a longitudinal Danish twin study. Aging Cell. 2016;15(1):149–154. Epub 2015/ 11/26. PubMed PMID: 26594032; PubMed Central PMCID: PMCPMC4717264.
- Dugue PA, Bassett JK, Joo JE, et al. Association of DNA methylation-based biological age with health risk factors and overall and cause-specific mortality. Am J Epidemiol. 2018;187(3):529–538. Epub 2017/ 10/12. PubMed PMID: 29020168.
- Marioni RE, Shah S, McRae AF, et al. DNA methylation age of blood predicts all-cause mortality in later life. Genome Biol. 2015;16:25. Epub 2015/ 01/31. PubMed PMID: 25633388; PubMed Central PMCID: PMCPMC4350614
- Perna L, Zhang Y, Mons U, et al. Epigenetic age acceleration predicts cancer, cardiovascular, and all-cause mortality in a German case cohort. Clin Epigenetics. 2016;8:64. Epub 2016/ 06/09. PubMed PMID: 27274774; PubMed Central PMCID: PMCPMC4891876
- Murabito JM, Zhao Q, Larson MG, et al. Measures of biologic age in a community sample predict mortality and age-related disease: the framingham offspring study. J Gerontol A Biol Sci Med Sci. 2018;73(6):757–762. Epub 2017/ 10/05. PubMed PMID: 28977464; PubMed Central PMCID: PMCPMC5946832.
- Horvath S, Pirazzini C, Bacalini MG, et al. Decreased epigenetic age of PBMCs from Italian semi-supercentenarians and their offspring. Aging (Albany NY). 2015;7(12):1159–1170. Epub 2015/ 12/19. PubMed PMID: 26678252; PubMed Central PMCID: PMCPMC4712339.
- Dhingra R, Nwanaji-Enwerem JC, Samet M, et al. DNA methylation age-environmental influences, health impacts, and its role in environmental epidemiology. Curr Environ Health Rep. 2018;5(3):317–327. Epub 2018/ 07/27. PubMed PMID: 30047075
- Dugue PA, Bassett JK, Joo JE, et al. DNA methylation-based biological aging and cancer risk and survival: pooled analysis of seven prospective studies. Int J Cancer. 2018;142(8):1611–1619. Epub 2017/ 12/03. PubMed PMID: 29197076.
- Zheng Y, Joyce BT, Colicino E, et al. Blood epigenetic age may predict cancer incidence and mortality. EBioMedicine. 2016;5:68–73. Epub 2016/ 04/15. PubMed PMID: 27077113; PubMed Central PMCID: PMCPMC4816845
- Levine ME, Hosgood HD, Chen B, et al. DNA methylation age of blood predicts future onset of lung cancer in the women’s health initiative. Aging (Albany NY). 2015;7(9):690–700. Epub 2015/ 09/29. PubMed PMID: 26411804; PubMed Central PMCID: PMCPMC4600626
- Ambatipudi S, Horvath S, Perrier F, et al. DNA methylome analysis identifies accelerated epigenetic ageing associated with postmenopausal breast cancer susceptibility. Eur J Cancer. 2017;75:299–307. Epub 2017/ 03/05. PubMed PMID: 28259012; PubMed Central PMCID: PMCPMC5512160
- Kresovich JK, Xu Z, O’Brien KM, et al. Methylation-based biological age and breast cancer risk. J Natl Cancer Inst. 2019;111(10):1051–1058. Epub 2019/ 02/23. PubMed PMID: 30794318; PubMed Central PMCID: PMCPMC6792078
- American Cancer Society. Cancer facts & figures 2020. Atlanta: American Cancer Society, Inc; 2020.
- Belanger CF, Hennekens CH, Rosner B, et al. The nurses’ health study. Am J Nurs. 1978;78(6):1039–1040. Epub 1978/ 06/01. PubMed PMID: 248266
- Final report on the aspirin component of the on going Physicians' Health Study Steering Committee of the Physicians' Health Study Research Group N Engl J Med. 1989 Jul 20;321(3):129–35. PMID: 2664509
- Giovannucci E, Ascherio A, Rimm EB, et al. Physical activity, obesity, and risk for colon cancer and adenoma in men. Ann Intern Med. 1995;122(5):327–334. Epub 1995/ 03/01. PubMed PMID: 7847643
- Hannum G, Guinney J, Zhao L, et al. Genome-wide methylation profiles reveal quantitative views of human aging rates. Mol Cell. 2013;49(2):359–367. Epub 2012/ 11/28. PubMed PMID: 23177740; PubMed Central PMCID: PMCPMC3780611.
- Horvath S. DNA methylation age of human tissues and cell types. Genome Biol. 2013;14(10):R115. Epub 2013/ 10/22. PubMed PMID: 24138928; PubMed Central PMCID: PMCPMC4015143
- Levine ME, Lu AT, Quach A, et al. An epigenetic biomarker of aging for lifespan and healthspan. Aging (Albany NY). 2018;10(4):573–591. Epub 2018/ 04/21. PubMed PMID: 29676998; PubMed Central PMCID: PMCPMC5940111.
- Houseman EA, Accomando WP, Koestler DC, et al. DNA methylation arrays as surrogate measures of cell mixture distribution. BMC Bioinformatics. 2012;13:86. Epub 2012/ 05/10. PubMed PMID: 22568884; PubMed Central PMCID: PMC3532182
- Salas LA, Koestler DC, Butler RA, et al. An optimized library for reference-based deconvolution of whole-blood biospecimens assayed using the illumina humanMethylationEPIC BeadArray. Genome Biol. 2018;19(1):64. Epub 2018/ 05/31. PubMed PMID: 29843789; PubMed Central PMCID: PMCPMC5975716.
- Wood SN. Fast stable restricted maximum likelihood and marginal likelihood estimation of semiparametric generalized linear models. J R Stat Soc Ser B. 2011;73(1):3–36.
- Li L, Choi JY, Lee KM, et al. DNA methylation in peripheral blood: a potential biomarker for cancer molecular epidemiology. J Epidemiol. 2012;22(5):384–394. Epub 2012/ 08/07. PubMed PMID: 22863985; PubMed Central PMCID: PMCPMC3798632.
- Ponnappan S, Ponnappan U. Aging and immune function: molecular mechanisms to interventions. Antioxid Redox Signal. 2011;14(8):1551–1585. Epub 2010/ 09/04. PubMed PMID: 20812785; PubMed Central PMCID: PMCPMC3061194
- Fiorito G, Polidoro S, Dugue PA, et al. Social adversity and epigenetic aging: a multi-cohort study on socioeconomic differences in peripheral blood DNA methylation. Sci Rep. 2017;7(1):16266. Epub 2017/ 11/28. PubMed PMID: 29176660; PubMed Central PMCID: PMCPMC5701128.
- Horvath S, Erhart W, Brosch M, et al. Obesity accelerates epigenetic aging of human liver. Proc Natl Acad Sci U S A. 2014;111(43):15538–15543. Epub 2014/ 10/15. PubMed PMID: 25313081; PubMed Central PMCID: PMCPMC4217403.
- Nevalainen T, Kananen L, Marttila S, et al. Obesity accelerates epigenetic aging in middle-aged but not in elderly individuals. Clin Epigenetics. 2017;9:20. Epub 2017/ 03/16. PubMed PMID: 28289477; PubMed Central PMCID: PMCPMC5310016
- Quach A, Levine ME, Tanaka T, et al. Epigenetic clock analysis of diet, exercise, education, and lifestyle factors. Aging (Albany NY). 2017;9(2):419–446. Epub 2017/ 02/16. PubMed PMID: 28198702; PubMed Central PMCID: PMCPMC5361673.
- Locke AE, Kahali B, Berndt SI, et al. Genetic studies of body mass index yield new insights for obesity biology. Nature. 2015;518(7538):197–206. Epub 2015/ 02/13. PubMed PMID: 25673413; PubMed Central PMCID: PMCPMC4382211.
- Gao X, Zhang Y, Breitling LP, et al. Relationship of tobacco smoking and smoking-related DNA methylation with epigenetic age acceleration. Oncotarget. 2016;7(30):46878–46889. Epub 2016/ 06/09. PubMed PMID: 27276709; PubMed Central PMCID: PMCPMC5216910