ABSTRACT
Piscirickettsiosis is the most important bacterial disease in the Chilean salmon industry, which has sorted several efforts to its control, generating enormous economic losses. Epigenetic alterations, such as DNA methylation, can play a relevant role in the modulation of the metazoans response to pathogens. Bacterial disease may activate global and local immune responses generating intricate responses with significant biological impact in the host. However, it is scarcely understood how bacterial infections influence fish epigenetic alterations. In the present study, we utilized Pacific salmon and Piscirickettsiosis as model, to gain understanding into the dynamics of DNA methylation among fish-bacterial infection interactions. A genome-wide analysis of DNA methylation patterns in female spleen tissue of Pacific salmon was achieved by reduced representation bisulphite sequencing from a time course design. We determined 2,251, 1,918, and 2,516 differentially methylated regions DMRs among infected and control Pacific salmon in 1 dpi, 5 dpi, and 15 dpi, respectively. The mean methylation difference per DMR among control and infected groups was of ~35%, with an oscillatory pattern of hypo, hyper, and hypomethylation across the disease. DMCs, among the control and infected group, showed that they were statistically enriched in intergenic regions and depleted in exons. Functional annotation of the DMR genes demonstrated three KEGG principal categories, associated directly with the host response to pathogens infections. Our results provide the first evidence of epigenetic variation in fish provoked by bacterial infection and demonstrate that this variation can be modulated across the disease.
Introduction
Piscirickettsiosis is the most relevant bacterial disease in the Chilean salmon industry and liable for significant economic losses. Its causative agent is Piscirickettsia salmonis, a facultative gram-negative intracellular bacterium [Citation1]. Despite the details of the infection mechanism of P. salmonis are unknown, it is established that the pre-eminent host target cell, where this bacterium replicate and survive, are the macrophages [Citation2]. Albeit it has been developed several strategies against Piscirickettsiosis (e.g. vaccines, genetic selection, immunostimulants), its success in the field has not been as expected [Citation3]. That is coherent with the intricacy of managing intracellular bacterial infections that use phagocytic cells to replicate and survive (e.g. macrophages), which have developed advantageous ability to adjust host defensive immune cells for its benefit [Citation4]. Preceding transcriptomic salmonid studies have highlighted the modulation of the expression of key genes involved in innate immune response and oxidative process upon P. salmonis infection [Citation5–11]. Which generates a host response of intensified inflammation, alteration of type 1 T helper cells T (e.g., interferon-gamma) expression and constraint in the processing and presentation of antigen. The regulation of gene expression is a complex process, where different mechanisms or players can act in parallel or synergistically, considering internal or external stimuli, to determine the final level of expression of a gene [Citation12]. In this context, DNA methylation is one of the major epigenetic alteration mechanisms in metazoans, that performs a pivotal role in the regulation of gene expression [Citation13]. DNA cytosine methylation, at CpG dinucleotides, is normally considered as the most frequent epigenetic form of covalent alteration in metazoans and the most broadly analysed [Citation14]. In the last decades, the role of CG methylation in the regulation of gene expression has accumulated several works, focused mainly on biological aspects such as development, growth, ageing, immunological disorders, genomic imprinting, and cancer [Citation15]. However, in the last years, the role of CG methylation in host-bacterial infections interactions have summoned increased attention, as a potential modulation mechanism of the immune host response [Citation16–20]. For example, different studies have demonstrated the association among Helicobacter pylori and Porphyromonas gingivalis infections, both gram-negative bacteria, and abnormal hypermethylation of key genes in the gastric mucosa and gingival epithelial cells, respectively [Citation21,Citation22]. In contrast, another work has reported a predominant role of hypomethylation in numerous genes upon the infection with Mycobacterium tuberculosis [Citation23]. The great majority of these studies have been focalized in model organisms (e.g., human), and even though there are several genome-wide DNA methylation studies in fish, including salmonids, these have concentrated in ecological, productive aspects and parasite/viral infections [Citation24–32]. For example, Dhanasiri et al. [Citation24] have shown the effect of plant-based versus marine ingredients diets on the epigenome of zebrafish. Likewise, Berbel‐Filho et al. [Citation31] and Xiong et al. [Citation32] showed that exposure to parasite and viral pathogens can modify the methylome of mangrove killifish (Kryptolebias hermaphroditus) and of grass carp (Ctenopharyngodon idella).
In the present study, we speculate that there is a different CG methylation signature in salmonids infected with P. salmonis compared to control without infection, which may highlight the role of DNA methylation in the early stage of Piscirickettsiosis. Therefore, the objective of this study was to characterize, for the first time, the early temporal CG methylation signature from female post-smolt Pacific salmon (Oncorhynchus kisutch) spleen tissue infected with P. salmonis, using high-throughput sequencing technology. Pacific salmon, is a relevant species that play an important role in the social, ecological, and aquaculture sector of the Pacific coast [Citation33].
Results
To gain knowledge and identified methylation patterns in Pacific salmon challenged with P. salmonis, reduced representation bisulphite libraries from a time course design and spleen tissue, were constructed and sequenced on the Illumina Hiseq X Ten platform. In agreement with other works, the cumulative mortality began to increase rapidly after approximately 15 dpi [Citation34,Citation35] (). A mean depth (±SD) of 53.87 (±7.14) million reads were sequenced by sample, with an average mapping performance (±SD) of 89.96% (±4.28) (Supplementary Table S1).
Figure 1. Mortality curves of Pacific salmon infected with P. salmonis. The figure shows the cumulative mortality of the four replicates (tanks) (E1–E4)
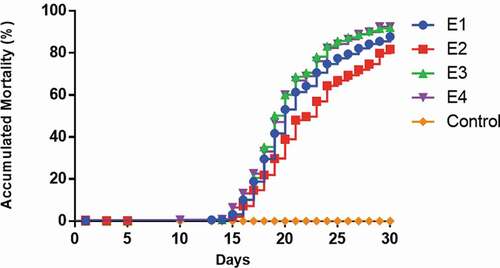
General patterns of differential methylation
To delineate general patterns of methylation response to bacterial infection in the Pacific salmon genome, we merged all infected (all the times) and control fish in two groups, and determine the respective general DMCs and DMRs. A total of 2,298,129 CG sites passed the minimum required filters, representing a mean of 4.7% of the total CGs available in the genome subsequently the alignment (Supplementary Table S1). A total of 53,503 DMCs (≥25, q < 0.01) were identified among control and infected group, with statistically significant more and fewer DMCs within intergenic regions and exons (G test; p < 0.05), respectively, that expected by chance. In the case of introns and promoters, not were detected significant difference (G test; p > 0.05) among the identified DMCs of each region and the expected by chance. Based on the DMCs identified, we established 718 DMRs (>25%, q < 0.01) among the control and infected group. A heatmap clustering of these 718 DMRs, showed that the totality of the samples was assorted according to the experimental group’s control and infected (). Of the total of DMRs identified, 345 were localized nearby or within of genes. The levels of absolute methylation differences among the control and infected group, of these DMRs, ranged from 25% to 90% per DMR (Supplementary Table S2). Likewise, both DMR and DMC presented a consistent pattern of higher levels of hyper that hypomethylation (454 hypermethylated and 264 hypomethylated DMRs, 33,019 hypermethylated, and 20,494 hypomethylated DMCs) among the experimental group’s control and infected. The total of DMRs obtained were mapped to 30 Pacific salmon chromosomes, with a range of 38 (LG8) to 11 (LG26) DMRs per chromosome.
Figure 2. (a) Comparative distribution (%) of DMCs genomic features (intergenic regions, promoters, introns and exons) and genomics features of the totality of CpG sites filtered. Outer and inner rings represent distribution of DMCs and filtered CpG sites, respectively. *: p < 0.05 (G-tets). (b) General DMR heatmap profile comparing pooled infected and control Pacific salmon organism
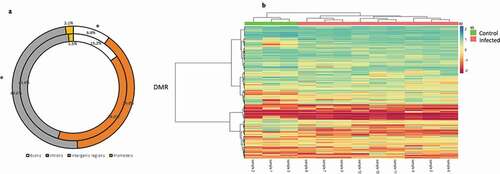
Time course patterns of differential methylation
To characterize specific epigenetic responses through the disease, DMR and DMC analyses were performed by time of infection. A total of 118,582, 107,346 and 127,143 DMCs were identified for 1 dpi, 5 dpi and 15 dpi, respectively, without an evident clustering on specific chromosomes or regions (–c)). An oscillatory pattern of DMC methylation was observed through the time course infection, exhibiting a higher number of DMCs hypomethylated in 1 dpi, hypermethylated in the 5 dpi and hypomethylated in 15 dpi ()). For all the experimental times we detected significantly (p < 0.05) lesser and enriched DMCs for exons and intergenic regions that expected by chance, respectively (). Based on the DMCs identified, we established 2,251, 1,918 and 2,516 DMRs to 1 dpi, 5 dpi and 15 dpi, respectively. Among a 43% to 46% of the DMRs identified (44% in 1dpi; 43% in 5dpi; 46% in 15 dpi) were localized nearby or within of genes. The levels of absolute methylation differences of these DMRs, among the control and infected times, ranged from 25% to 90% (1 dpi), from 25% to 80% (5 dpi) and from 25% to 92% (15 dpi) per DMR (Supplementary table S3). Likewise, 157 (7%) DMRs were shared across the time course infection and 561 (24.9%), 393 (17.4%) and 729 (32.4%) were unique for the infection time 1 dpi, 5 dpi and 15 dpi, respectively (). The GO enrichment analysis, of the DMRs mapped to genes, showed that most abundant significantly enriched categories in biological process, cellular component and molecular function were shared among the experimental times. Biological regulation, cellular processes, and regulation of biological process were the most abundant significantly enriched categories of biological process in 1, 5 and 15 dpi. To the cellular component category, the most abundant significantly enriched categories were cell, cell part, and organelle. In the case of the molecular function category, binding, catalytic activity, and transport activity were the most abundant categories (–c)). A total of 14 statistically enriched KEGG pathways were detected, with most of them (57%) being shared among the experimental times. The most significant shared categories corresponded to PI3K-Akt signalling pathway, focal adhesion, and ECM–receptor interaction ()).
Figure 3. (a–c) Manhattan plots of the chromosomal distribution of differentially methylated CpGs loci among infected and control Pacific salmon in (a) 1 dpi, (b) 5 dpi and (c) 15 dpi. Each point means an individual DMC with genomic location on the x-axis and DMC percentage of methylation on the y-axis. Dashed line represent limit among hypo and hypermethylated loci. (d) Frequency of hypo and hypermethylated DMCs along the Pacific salmon genome in 1 dpi – 15 dpi
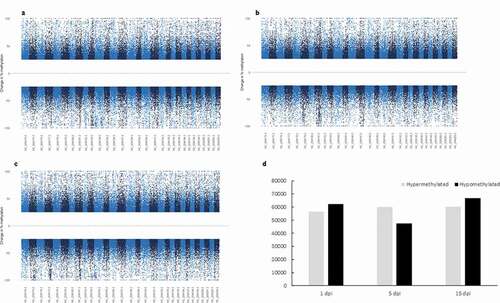
Figure 4. (a–c) Comparative distribution (%) of DMCs genomic features (intergenic regions, promoters, introns and exons) and genomics features of the totality of CpG sites filtered in (a) 1 dpi, (b) 5 dpi and (c) 15 dpi. Outer and inner rings represent distribution of DMCs and filtered CpG sites, respectively. *: p < 0.05 (G-tets). (d-f) DMR heatmap profile comparing infected and control Pacific salmon in (d) 1 dpi, (e) 5 dpi and (f) 15 dpi
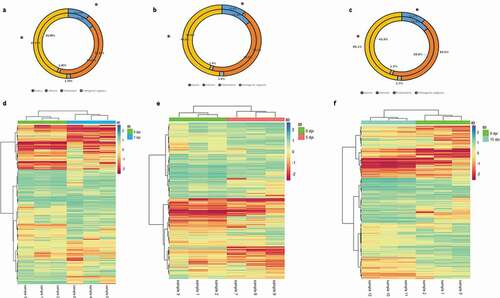
Discussion
In the last years, the epigenetics interactions of host-bacterial infections have concentrated an expanded interest. DNA methylation is the most investigated and best known functional epigenetics mechanism related to regulate gene expression [Citation36]. Previous in vitro and in vivo studies have demonstrated that the levels of DNA methylation may be modified in response to bacterial infections and that in turn these, can be associate with gene expression changes [Citation37–41]. However, these studies have been focalized mainly in model organism such, human and bovine. Therefore, exist scarce knowledge about the role of DNA methylation response of non-models animals, such as fish, to bacterial infections. In this study we used RRBS methodology, to evaluate DNA methylation in spleen tissue from female Pacific salmon during a time course infection by P. salmonis and uncovered both a general and specific epigenetics patterns across the disease.
From a general perspective, our results demonstrated that the pooled infected group showed a higher level of DMR and DMC hypermethylation in comparison with the control group. This is coherent with previous in vitro studies of Helicobacter pylori-infected human gastric mucosa cells and of several types of bovine cells challenged with lipopolysaccharides, where an increase in the level of DNA methylation was reported [Citation21,Citation38]. Furthermore, in vivo studies of Tongue sole (Cynoglossus semilaevis) infected with Vibrio harveyi, also have shown higher levels of DNA methylation upon infection [Citation42]. Moreover, a heatmap DMR-based cluster showed that the totality of the infected and control samples was clearly differentiated among both groups. Therefore, an increase in the levels of DNA methylation could correspond to a generalized response to bacterial infection in Pacific salmon. Besides, we also detected a moderated mean methylation of ~35% per DMR among the pooled infected and control groups, a value similar to the reported (~30%) among migratory and nonmigratory rainbow trout phenotypes and among control and ectoparasite infected Trinidadian guppies (Poecilia reticulata) [Citation29,Citation43]. Nonetheless, lower value of mean methylation (23% per DMR) have been reported among hatchery and stream-rearing environment conditions in rainbow trout organisms [Citation28].
The genomic distribution of DMCs, among the control and infected group of Pacific salmon shown that they were statistically enriched in intergenic regions and depleted in exons. Although canonically, the DNA methylation transcriptional suppression role has been mainly associated to promoters regions [Citation44], DNA methylation in intergenic regions can perform an important role in the silencing of transposable and viral elements, preventing in turn, potential gene interruptions, chromosome wreckage, and mutations, among others effect [Citation45,Citation46]. Interesting, stress conditions, including pathogens infection, can stimulate DNA transposons components in the genome [Citation47]. Consequently, a DMC increase in the intergenic regions of bacterial-infected Pacific salmon genome will contribute to manage its genome completeness, suppressing transposable elements. This is consistent with the elevated proportion of repeated elements found in the genome of salmonids [Citation48]. Exons-specific intragenic DNA methylation has been positively associated with gene expression levels and with the regulation of alternative splicing [Citation41,Citation49]. Also, human methylome and RNA-sequencing analysis have demonstrated that lower expression exons exhibit a reduced level of DNA methylation in comparison with higher expression exons [Citation50,Citation51]. Therefore, the depletion of DMC in exons, observed in this study, could contribute to decreasing the levels of expression in the transcriptome of bacterial-infected Pacific salmon.
From a time course perspective, we found a modulation of the levels of DMC and DMR, being both higher in the times 1 dpi and 15 dpi than in the time 5 dpi, with a consistent pattern of hypo-hyper-hypomethylation. This epigenetic modulation suggests that in the times 1 dpi and 15 dpi could exist a higher gene regulation response against P. salmonis. Besides, also we found a major trend to unique DMRs across the time’s infection, which could also mirror the effect of the progression of the infection in the levels of host methylation response. Nonetheless, several studies have established that pathogens can adjust the host response for their benefit, including epigenetic responses [Citation52–55]. In fact, we found several DMRs that mapped to genes (e.g., histone-arginine methyltransferase CARM1, N-lysine methyltransferase SMYD2-A, methyltransferase-like 26 and methyltransferase 1) implicated in the regulation of epigenetic changes [Citation56]. Methylation modifications in these genes may alter in a wide genomic range the levels of methylation. Moreover, the most relevant KEGG pathways categories of the mapped time course DMR genes (PI3K-Akt signalling pathway, focal adhesion, and ECM–receptor interaction), correspond to pathways related directly with the host response to pathogens infections. PI3K/Akt signalling is considered one relevant regulator pathway of several cellular process, including inflammatory host responses [Citation57]. The activation or attenuation of this pathway has been demonstrated that can be regulated by bacterial to their convenience, through for example of the control of the host process of apoptosis and cell survival [Citation58]. Focal adhesions correspond to an aggregation of proteins that generate a physical bridge of connection among integrins and the actin cytoskeleton [Citation59]. Several studies have demonstrated that bacterial pathogens may handle these proteins to facilitate their entry in host cells [Citation60]. In addition, the extracellular matrix (ECM), which is a dynamic cellular meshwork complex of macromolecules, perform key roles in cellular process such proliferation, migration, adhesion, and apoptosis, among others [Citation61]. Accumulative evidence has demonstrated that ECM components may be targeted or degraded by bacterial pathogens, enhancing the adhesion and/or propagation into the host [Citation62]. Interestingly, the categories described were shared across the disease, which could suggest that they are an important target of methylation changes upon P. salmonis infection in Pacific salmon.
Preceding salmonids transcriptomic studies have reported several coding genes differentially expressed among control and P. salmonis infected individuals [Citation5–11]. Although the functional annotation reported of these coding genes, in terms of GO categories and KEGG pathways, agree with the results obtained in the present study (e.g., GO:0050789, KO:04510) a one to one gene comparison showed that only a reduced number of these genes ((e.g., chitinase (cht), glutathione S-transferase (gst)) overlap with the DMR genes (epiloci) mapped in this study. This could imply that although both, genetic and epigenetic processes, can agree in the principal functional categories of the response to bacterial infection in salmonids, they may affect different specific genes.
In another hand, our experiment can have some potential drawbacks. Although RRBS is normally considered a robust genome-wide methylation approach, this will need a reference genome of adequate quality to effects of alignment and functional annotation aims. Albeit the assembled genome version of Pacific salmon, Oki_V2, available in the National Centre for Biotechnology Information, is an improved version with respect to Oki-V1, this is not finished. Therefore, the percentage of DMRs that not were placed within or near to know genes could be associated with an insufficient annotation of the Pacific salmon genome. Likewise, it is widely accepted that epigenetic changes are tissue-specific and that bacterial diseases may activate global and local immune responses. Therefore, the evaluating of other Pacific salmon tissues, including blood, could complement the results obtained in this study.
Conclusions
Congruent with preceding studies of bacterial diseases, in this study, we present the first proof that bacterial infection may have a relevant impact on genomic DNA methylation in fish. Our genome-wide methylation results disclose significant methylation alterations in Pacific salmon infected with P. salmonis and revealed that these alterations differ across the disease. Furthermore, our results showed a statistically significant up and down representation of methylation changes in intergenic regions and exons, respectively, implying a relevant performance of these genomic sectors in fish-bacterial interactions. Considering the in vivo challenge experiment results, the number of DMRs and metabolic pathway associated is reasonable propose that the epigenetic changes observed in the Pacific salmon genome could be possibly impelled by the bacterial pathogen, P. salmonis, to its proper benefit. Further research is warranted to evaluate if our results can leverage transcriptional changes and develop in this way a mechanistic model to explain resistant and susceptible Piscirickettsiosis phenotypes.
Material and methods
Experimental infection design and sampling
All animal experiments in this study were approved and conformed to the Institutional Ethics Committee, from Universidad de Santiago guidelines (USCH-1). Individuals utilized in the present study are part of a major experiment, including 1,700 animals from 35 different full-sub families from the genetic nucleic of Marine Farm S.A., Puerto Montt, Chile. The totalities of animals were not vaccinated and, were informed as pathogen-free by the national certified laboratory Fish Vet Group SpA. The challenge experiments were developed in the installations of Fundación Chile S.A. A virulent LF-89 strain of P. salmonis, isolated on 2010 from rainbow trout (Oncorhynchus mykiss), was utilized. Experimental individuals were infected by intraperitoneal injection (IP). Initially, a median lethal dose (LD50) was determined using 250 individuals (150 g mean), previously acclimated during 15 d, and five different dilutions (1:10, 1:100, 1:500, 1:1000, 1:10,000) plus a control. The LD50 challenge experiment had a duration of 30 d and a LD50 dilution of 1:700, which it was estimated at the end of the experiment and utilized for inoculation of the animals on the principal challenge. During the principal challenge, the experiment was developed in 1,450 individuals, all of it marked individually with Pit-Tags. Similar, to the LD50 experiment, individuals from the principal challenge were previously acclimated for 15 d, and then distributed randomly in four tanks (11 m3) (15°C, 32–33‰). Individuals were fed ad libitum with commercial feed pellet micro 100 and micro 200 (EWOS, Chile). Environmental parameters (e.g., temperature, salinity, and oxygen concentration) and cumulative mortalities were registered daily until the end of the experiment (30 d post-injection). In the course of the experiment, three individuals (females, same maturity level) were randomly sampled by tank at 0 (control non-infected time point, before inoculation), 1, 5 and 15 d post-injection (dpi). After sacrifice (blow head), spleen samples were surgically removed and collected from each individual and then stored immediately at −80°C. Spleen tissue was favoured because of its importance as an of the mayor lymphoid tissues in fishes and because is on the main organs affected by Piscirickettsiosis [Citation63].
DNA extraction and sequencing
Total DNA was isolated individually, using the DNeasy Blood and Tissue Kit (Qiagen, Holland) following manufacturer’s instructions. DNA concentration, purity, and integrity were determined by spectrophotometry (BioPhotometer, Eppendorf) and by Agilent Bioanalyser 2100, respectively. In order to survey genome-wide DNA methylation levels, reduced-representation bisulphite sequencing (RRBS) were developed [Citation64]. For this, 1ug of genomic DNA was digested with the MspI enzyme (New England Biolabs, USA), followed by end repair, A-base tailing and 5-methylcytosine-modified adapter ligation. Size selection was performed to obtain DNA fractions of MspI-digested products in the range of 150–350 bp. Subsequently, bisulphite treatment was conducted using the ZYMO EZ DNA Methylation-Gold Kit (Zymo Research, USA) following the manufacturer’s instructions. The converted DNAs were then amplified by 12 cycles of PCR, using 25 μl KAPA HiFi HotStart Uracil+ ReadyMix (2X) and 8-bp index primers (Roche, USA) with a final concentration of 1 μM each. The constructed RRBS libraries (12) were then analysed by Agilent 2100 Bioanalyzer and quantified by a Qubit fluorometer with Quant-iT dsDNA HS Assay Kit (Invitrogen, USA), and finally sequenced on Illumina Hiseq X Ten platform using a paired-end 150 bp strategy by CD Genomics.
Sequence trimming and mapping
Sequencing reads were quality and adapter trimmed using FastQC v 0.11.8 [Citation65] and TrimGalore! v 0.6.4 (http://www.bioinformatics.babraham.ac.uk/projects/trim_galore) with the specific RRBS option (–rrbs) enabled. Trimmed reads were aligned to the Pacific salmon genome reference, Okis_V2 (GenBank assembly accession: GCA_002021735.2) with BatMeth2 v 1.0 [Citation66]. Default settings, except for tolerating zero non-bisulphite mismatch number per read, were utilized. Only reads that mapped uniquely to the Pacific salmon genome reference, were considered in the next analysis. Due to the incapacity of bisulphite sequencing in distinguish among a real methylation variation and a C/T single nucleotide polymorphism (SNP), the package BS-SNPer [Citation67] was used to determine sites with presumptive CG SNP in the trimmed RRBS reads. The putative CG SNPs identified were detached from downstream analysis. Furthermore, only cytosine methylation in CpG dinucleotides were analysed, because CpG is the most frequent, most investigated and best known functional methylation in animal vertebrates [Citation68].
Identification of differentially methylated cytosines and regions
Individual differentially methylated cytosines (DMC) and differentially methylated regions (DMR), were identified using the R package MethylKit v-1.12.0 [Citation69], with read coverage normalized between samples. A minimum of 10 reads in all samples were needed at each CG site, for that site to be included in downstream analyses. Moreover, sites that were categorized with a coverage of the 99.9th percentile were filtered out in the next analysis [Citation70]. DMC were determined with a minimum false discovery rate (q-value) of 1%, correction for overdispersion and methylation difference of + (hypermethylation)/-(hypomethylation) ≥25% among control and infected times/fish. For identified DMR two criteria were required: i) three or more GC sites within a 1Kb region, with at least one site classified as DMC, and ii) absolute mean methylation difference greater than 25%, when comparing infected and control times/fish. For evaluate the common DMRs throughout the disease, the chromosomal names and coordinates of each DMR were extracted from the Pacific salmon genome reference, Okis_V2. To assort the Pacific salmon GC patterns and experimental times, a heatmap cluster analysis was conducted utilizing complete clustering on Euclidian distances, with Pheatmap v-1.0.12 (https://cran.r-project.org/web/packages/pheatmap/).
Gene annotation and functional analysis
DMRs and DMCs, were annotated to genes in the context of the genomic features promoters, exons, introns and intergenic regions, using the predicted gene models available for the Pacific salmon genome reference, Okis_V2 and BEDTools [Citation71]. Promoters regions were defined as within 1 kb up-and downstream of the transcription starting site [Citation72]. We used the G-test [74] and the package RVAideMemoire v 0.9–75 (https://cran.r-project.org/web/packages/RVAideMemoire/index.html), for compare the null distribution of the GC sites identified versus the distribution of the DMCs detected, in the context of its positions in the genomic features defined. When genomic features overlay, we gave preference to promoters > exons > introns > intergenic regions. To the functional annotation of DMRs also were considered the regions 5 kb up-and downstream of the matched positions [Citation25]. To recognize the functional roles of the associate DMR genes predicted, an enrichment analysis was performed on GO (Gene Ontology) terms and KEGG (Kyoto Encyclopaedia of Genes and Genomes) pathways with the packages in R topGo-v3.9 (http://bioconductor.org/packages/release/bioc/html/topGO.html) and KEGGprofile-v3.9 (https://bioconductor.org/packages/release/bioc/html/KEGGprofile.html), respectively. GO terms and KEGG pathways were considered significant with a Benjamini and Hochberg correction of FDR (False Discovery Rate) <0.05.
Author contributions
F.L., and R.V., designed research; S.B., R.V., and F.L., developed experimental protocols and bioinformatics pipelines; J.M., O.G., conducted the sampling; R.V., F.L., N.B., and K.K.G, wrote the paper. All authors reviewed the manuscript.
Accession number
The raw sequence has been deposited in the Sequence Read Archive (SRA), accession number National Center for Biotechnology Information (NCBI) SRA# PRJNA641939.
Supplemental Material
Download MS Excel (283.6 KB)Disclosure statement
The authors reported no potential conflicts of interest.
Supplementary material
Supplemental data for this article can be accessed here.
Additional information
Funding
References
- Fryer JL, Hedrick RP. Piscirickettsia salmonis: a gram-negative intracellular bacterial pathogen of fish. J Fish Dis. 2003;5:251–262.
- Ramírez R, Gómez FA, Marshall SH. The infection process of Piscirickettsia salmonis in fish macrophages is dependent upon interaction with host-cell clathrin and actin. FEMS Microbiol Lett. 2015;362:1–8.
- Evensen Ø. Immunization strategies against Piscirickettsia salmonis infections: review of vaccination approaches and modalities and their associated immune response profiles. Front Immunol. 2016;7:482.
- Das K, Garnica O, Dhandayuthapani S. Modulation of host miRNAs by intracellular bacterial pathogens. Front Cell Infect Microbio. 2016;6:79.
- Valenzuela-Miranda D, Valenzuela-Muñoz V, Farlora R, et al. MicroRNA-based transcriptomic responses of Atlantic salmon during infection by the intracellular bacterium Piscirickettsia salmonis. Dev Comp Immunol. 2017;77:287–296.
- Valenzuela-Miranda D, Gallardo-Escárate C. Novel insights into the response of Atlantic salmon (Salmo salar) to Piscirickettsia salmonis: interplay of coding genes and lncRNAs during bacterial infection. Fish Shellfish Immunol. 2016;59:427–438.
- Tacchi L, Bron JE, Taggart JB, et al. Multiple tissue transcriptomic responses to Piscirickettsia salmonis in Atlantic salmon (Salmo salar). Physiol Genomics. 2011;43:1241–1254.
- Pulgar R, Hödar C, Travisany D, et al. Transcriptional response of Atlantic salmon families to Piscirickettsia salmonis infection highlights the relevance of the iron-deprivation defence system. BMC Genomics. 2015;16:495.
- Rozas-Serri M, Peña A, Maldonado L. Transcriptomic profiles of post-smolt Atlantic salmon challenged with Piscirickettsia salmonis reveal a strategy to evade the adaptive immune response and modify cell-autonomous immunity. Dev Comp Immunol. 2018;81:348–362.
- Rozas-Serri M, Peña A, Maldonado L. Patterns of Piscirickettsia salmonis load in susceptible and resistant families of Salmo salar. Fish Shellfish Immunol. 2015;45:67–71.
- Rozas-Serri M, Peña A, Arriagada G, et al. Comparison of gene expression in post-smolt Atlantic salmon challenged by LF-89-like and EM-90-like Piscirickettsia salmonis isolates reveals differences in the immune response associated with pathogenicity. J Fish Dis. 2018;41:539–552.
- Emerson BM. Specificity of gene regulation. Cell. 2002;109:267–270.
- Gibney ER, Nolan CM. Epigenetics and gene expression. Heredity (Edinb). 2010;105:4–13.
- Robertson KD, Jones PA. DNA methylation: past, present and future directions. Carcinogensis. 2000;21:461–467.
- Wu H, Zhang Y. Reversing DNA methylation: mechanisms, genomics, and biological functions. Cell. 2014;156:45–68.
- Pacis A, Tailleux L, Morin AM, et al. Bacterial infection remodels the DNA methylation landscape of human dendritic cells. Genome Res. 2015;25:1801–1811.
- Bannister S, Messina NL, Novakovic B, et al. The emerging role of epigenetics in the immune response to vaccination and infection: a systematic review. Epigenetics. 2020;15:555–593.
- Grabiec AM, Potempa J. Epigenetic regulation in bacterial infections: targeting histone deacetylases. Crit Rev Microbiol. 2018;44:336–350.
- Marimani MAA, Duse A. The role of epigenetics, bacterial and host factors in progression of Mycobacterium tuberculosis infection. Tuberculosis. 2018;113:200–214.
- Crimi E, Benincasa G, Cirri S, et al. Clinical epigenetics and multidrug-resistant bacterial infections: host remodelling in critical illness. Epigenetics. 2020;14:1–13.
- Muhammad JS, Eladl MA, Khoder G. Helicobacter pylori-induced DNA Methylation as an epigenetic modulator of gastric Cancer: recent outcomes and future direction. Pathogens. 2019;8:23.
- Drury L, Chung WO. DNA methylation differentially regulates cytokine secretion in gingival epithelia in response to bacterial challenges. Pathog Dis. 2015;73:1–6.
- Gomez-Gonzalez PJ, Andreu N, Phelan JE, et al. An integrated whole genome analysis of Mycobacterium tuberculosis reveals insights into relationship between its genome, transcriptome and methylome. Sci Rep. 2019;9:5204.
- Dhanasiri AKS, Chen X, Dahle D, et al. Dietary inclusion of plant ingredients induces epigenetic changes in the intestine of zebrafish. Epigenetics. 2020;5:1–17.
- Le Luyer J, Laporte M, Beachamc TD, et al. Parallel epigenetic modifications induced by hatchery rearing in a Pacific salmon. Proc Natl Acad Sci USA. 2017;114:12964–12969.
- Fang X, Corrales J, Thornton C, et al. Global and gene specific DNA methylation changes during zebrafish development. Comp Biochem Phy B. 2013;166:99–108.
- Mackenzie RG, Nichols KM, Goetz GW, et al. Characterization of genetic and epigenetic variation in sperm and red blood cells from adult hatchery and natural-origin steelhead. Oncorhynchus mykiss. 2018;G3(8):3723–3736.
- Gavery MR, Nichols KM, Berejikian BA, et al. Temporal dynamics of DNA methylation patterns in response to rearing juvenile steelhead (Oncorhynchus mykiss) in a hatchery versus simulated stream environment. Genes (Basel). 2019;10:356.
- Baerwald MR, Meek MH, Stephens MR, et al. Migration-related phenotypic divergence is associated with epigenetic modifications in rainbow trout. Mol Ecol. 2016;25:1785–1800.
- Venney CJ, Johannsson MJ, Heath DD. Inbreeding effects on gene-specific DNA methylation among tissues of Chinook salmon. Mol Ecol. 2016;25:4521–4533.
- Berbel‐Filho WM, Garcia de Leaniz C, Morán P, et al. Local parasite pressures and host genotype modulate epigenetic diversity in a mixed‐mating fish. Ecol Evol. 2019;9:8736–8748.
- Xiong L, He L, Luo L, et al. Global and complement gene-specific DNA methylation in grass carp after grass carp reovirus (GCRV) infection. Int J Mol Sci. 2018;19:1110.
- Leiva F, Rojas-Herrera M, Reyes D, et al. Identification and characterization of miRNAs and lncRNAs of coho salmon (Oncorhynchus kisutch) in normal immune organs. Genomics. 2020;112:45–54.
- Lhorente JP, Gallardo JA, Villanueva B, et al. Disease resistance in Atlantic salmon (Salmo salar): coinfection of the intracellular bacterial pathogen Piscirickettsia salmonis and the sea louse caligus rogercresseyi. PLoS One. 2014;9:e95397.
- Barría A, Christensen KA, Yoshida GM, et al. Genomic predictions and genome-wide association study of resistance against Piscirickettsia salmonis in coho salmon (Oncorhynchus kisutch) using ddRAD sequencing. 2018;G3(8):1183–1194.
- Razin A, Kantor B. DNA Methylation in epigenetic control of gene expression in Epigenetics and Chromatin. (ed. Jeanteur, P.). Springer, Berlin, Heidelberg; Springer: 151–167. 2005.
- Chen J, Wu Y, Sun Y, et al. Bacterial lipopolysaccharide induced alterations of genome-wide DNA methylation and promoter methylation of lactation-related genes in bovine mammary epithelial cells. Toxins (Basel). 2019;11:298.
- Korkmaz FT, Kerr DE. Genome-wide methylation analysis reveals differentially methylated loci that are associated with an age-dependent increase in bovine fibroblast response to LPS. BMC Genomics. 2017;18:405.
- Jhamat N, Niazi A, Guo Y, et al. LPS-treatment of bovine endometrial epithelial cells causes differential DNA methylation of genes associated with inflammation and endometrial function. BMC Genomics. 2020;21:385.
- De Luca M, Pels K, Moleirinho S, et al. The epigenetic landscape of innate immunity. AIMS Mol Sci. 2017;4:110–139.
- de la Calle-fabregat C, Morante-Palacios O, Ballestar E. Understanding the relevance of DNA methylation changes in immune differentiation and disease. Genes (Basel). 2020;11:110.
- Xiu J, Shao C, Zhu Y, et. al. Differences in DNA methylation between disease-resistant and disease-susceptible chinese tongue sole (Cynoglossus semilaevis) families. Front Genet. 2019;13(10):847.
- Luo C, Hajkova P, Ecker JE. Dynamic DNA methylation: in the right place at the right time. Science. 2018;361:1336–1340.
- Montanini B, Chen P-Y, Morselli M, et al. Non-exhaustive DNA methylation-mediated transposon silencing in the black truffle genome, a complex fungal genome with massive repeat element content. Genome Biol. 2014;15:411.
- Slotkin RK, Martienssen R. Transposable elements and the epigenetic regulation of the genome. Nat Rev Genet. 2007;8:272–285.
- Capy P, Gasperi G, Biémont C, et al. Stress and transposable elements: co‐evolution or useful parasites? Heredity (Edinb). 2000;85:101–106.
- Lien S, Koop BF, Sandve SR, et al. The Atlantic salmon genome provides insights into rediploidization. Nature. 2016;533:200–205.
- Berthelot C, Brunet F, Chalopin D, et al. The rainbow trout genome provides novel insights into evolution after whole-genome duplication in vertebrates. Nat Commun. 2014;5:3657.
- Choi JK. Contrasting chromatin organization of CpG islands and exons in the human genome. Genome Biol. 2010;11:R70.
- Tan S, Wang W, Zhong X, et al. Increased alternative splicing as a host response to Edwardsiella ictaluri infection in catfish. Mar Biotechnol. 2018;20:729–738.
- Morandini AC, Santos CF, Yilmaz O. Role of epigenetics in modulation of immune response at the junction of host–pathogen interaction and danger molecule signaling. Pathog Dis. 2016;74:ftw082.
- Zhang Q, Cao X. Epigenetic regulation of the innate immune response to infection. Nat Rev Immunol. 2019;19:417–432.
- Gómez-Díaz E, Jordà M, Peinado MA, et al. Epigenetics of host-pathogen Interactions: the Road ahead and the road behind. PLoS Pathog. 2012;8:e1003007.
- Schmid-Hempel P. Evolutionary parasitology: the integrated study of infections, immunology, ecology, and genetics. England: Oxford University Press; 2011.
- Lyko F. The DNA methyltransferase family: a versatile toolkit for epigenetic regulation. Nat Rev Genet. 2018;19:81–92.
- Fayard E, Xue G, Parcellier A, et al. Protein kinase B (PKB/Akt), a key mediator of the PI3K signaling pathway. Curr Top Microbiol Immunol. 2010;346:31–56.
- Krachler AM, Woolery AR, Orth K. Manipulation of kinase signaling by bacterial pathogens. J Cell Biol. 2011;195:1083–1092.
- Lo SH. Focal adhesions: what’s new inside. Dev Biol. 2006;294:280–291.
- Shi J, Casanova JE. Invasion of host cells by Salmonella typhimurium requires focal adhesion kinase and p130Ca. Mol Biol Cell. 2006;17:4698–4708.
- Yue B. Biology of the extracellular matrix: an overview. J Glaucoma. 2014;S20–S23. DOI:https://doi.org/10.1097/IJG.0000000000000108
- Chagnot C, Listrat A, Astruc T, et al. Bacterial adhesion to animal tissues: protein determinants for recognition of extracellular matrix components. Cell Microbiol. 2012;14:1687–1696.
- Almendras F, Fuentealba IC. Salmonid rickettsial septicemia caused by Piscirickettsia salmonis: a review. Dis Aquat Org. 1997;29:137–144.
- Meer A, Gnirke A, Bell GW, et al. Reduced representation bisulfite sequencing for comparative high-resolution DNA methylation analysis. Nucleic Acids Res. 2005;33:5868–5877.
- Wingett SW, Andrews S. FastQ Screen: A tool for multi-genome mapping and quality control. F1000Res. 2018;7:1338.
- Zhou Q, Lim J-Q, Sung W-K, et al. An integrated package for bisulfite DNA methylation data analysis with Indel-sensitive mapping. BMC Bioinformatics. 2019;20:47.
- Gao S, Zou D, Mao L, et al. BS-SNPER: SNP calling in bisulfite-seq data. Bioinformatics. 2015;31:4006–4008.
- Varriale A. DNA methylation, epigenetics, and evolution in vertebrates: facts and challenges. Int J Evol Biol. 2014;475981:2014.
- Akalin A, Kormaksson M, Li S, et al. methylKit: a comprehensive R package for the analysis of genome-wide DNA methylation profiles. Genome Biol. 2012;13:R87.
- Warnecke PM, Stirzaker M, Melki JR, et al. Detection and measurement of PCR bias in quantitative methylation analysis of bisulphite-treated DNA. Nucleic Acids Res. 1997;25:4422–4426.
- Quinlan AR, Hall IM. BEDTools: a flexible suite of utilities for comparing genomic features. Bioinformatics. 2010;26:841–842.
- Akalin A, Franke V, Vlahoviček K, et al. Genomation: a toolkit to summarize, annotate and visualize genomic intervals. Bioinformatics. 2015;31:1127–1129.
- Smith G, Smith C, Kenny JG, et al. Genome-wide DNA methylation patterns in wild samples of two morphotypes of threespine stickleback (Gasterosteus aculeatus). Mol Biol Evol. 2015;32:888–895.