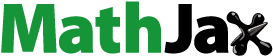
ABSTRACT
Hexavalent chromium compounds are well-established respiratory carcinogens to which humans are commonly exposed in industrial and occupational settings. In addition, natural and anthropogenic sources of these compounds contribute to the exposure of global populations through multiple routes, including dermal, ingestion and inhalation that elevate the risk of cancer by largely unresolved mechanisms. Cr(VI) has genotoxic properties that include ternary adduct formation with DNA, increases in DNA damage, mostly by double-strand break formation, and altered transcriptional responses. Our previous work using ATAC-seq showed that CTCF motifs were enriched in Cr(VI)-dependent differentially accessible chromatin, suggesting that CTCF, a key transcription factor responsible for the regulation of the transcriptome, might be a chromium target. To test this hypothesis, we investigated the effect of Cr(VI) treatment on the binding of CTCF to its cognate sites and ensuing changes in transcription-related histone modifications. Differentially bound CTCF sites were enriched by Cr(VI) treatment within transcription-related regions, specifically transcription start sites and upstream genic regions. Functional annotation of the affected genes highlighted biological processes previously associated with Cr(VI) exposure. Notably, we found that differentially bound CTCF sites proximal to the promoters of this subset of genes were frequently associated with the active histone marks H3K27ac, H3K4me3, and H3K36me3, in agreement with the concept that Cr(VI) targets CTCF in euchromatic regions of the genome. Our results support the conclusion that Cr(VI) treatment promotes the differential binding of CTCF to its cognate sites in genes near transcription-active boundaries, targeting these genes for dysregulation.
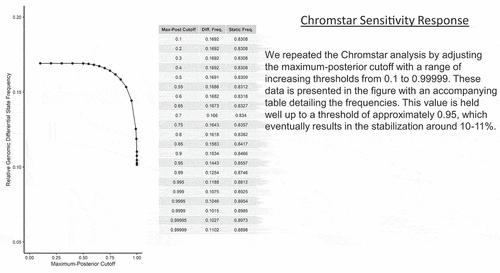
Introduction
Compounds containing hexavalent chromium (Cr(VI)), collectively known as chromates, are well-established respiratory carcinogens used in industries associated with metallurgical and refractory processes [Citation1]. Epidemiological and meta-analyses of published studies have demonstrated a positive association between Cr(VI) exposure and increased mortality, with an increased cancer risk in tissues of direct or early contact, such as the respiratory tract, head and neck, and the gastrointestinal tract [Citation2]. In 2006, OSHA estimated that there were close to 560,000 workers occupationally exposed to Cr(VI) through inhalation and dermal routes, with most exposures occurring in steel-related welding, painting, electroplating, steel mills, foundries, and textile dyes [Citation3]. However, both anthropogenic and natural sources of Cr(VI) promote environmental contamination of air, soil, and water, resulting in much large populations of individuals being exposed to low levels of the metal over long periods of time. In humans, gastrointestinal effects have been observed epidemiologically both in occupational and in general populations, with symptoms of abdominal pain, ulceration, indigestion, and vomiting [Citation1]. Animal studies in our lab and others have corroborated these adverse health effects in the gastrointestinal tract following exposure to Cr(VI) in drinking water, including duodenal histiocytic infiltration, epithelial hyperplasia, enterocyte hypertrophy, and chronic villous wounding [Citation1,Citation4–6]. These findings highlight the need to investigate potential adverse health outcomes that may be associated with chronic exposure to low levels of Cr(VI).
Despite the wealth of evidence at the molecular level suggesting that Cr(VI) has carcinogenic properties, the mechanisms that promote tumorigenesis are not fully understood. Cr(VI) is largely reduced extracellularly to trivalent chromium (Cr(III)) within the gastrointestinal system following ingestion, with gastric juice acting as the primary reducing agent in the stomach [Citation7,Citation8]. Extracellular trivalent chromium does not permeate efficiently across cellular membranes and is primarily excreted in urine [Citation1]. In contrast, hexavalent chromium is readily transported across membranes by anion transporters. Approximately 95% of the intracellular load is reduced through 1- or 2- electron steps to Cr(III) by antioxidants such as ascorbate, glutathione, and cysteine [Citation9,Citation10]. As a consequence of Cr(VI) reduction, cells retain elevated levels of Cr(III), which cannot be secreted across the cellular membrane, but can react with DNA to form both binary (Cr-DNA) and ternary (ligand-Cr-DNA) adducts. These adducts elicit genotoxicity through multiple routes, including oxidative stress and radical-mediated DNA damage. DNA adduction leads to double strand DNA break formation and persistent γH2A.X foci accumulation, as well as to larger biological aberrations such as micronucleus formation and aneuploidy [Citation7,Citation10–14]. The reduction of Cr(VI) creates a redox-sensitive state within the cell that activates response pathways associated with oxidative stress and, through the generation of free radicals, induces ERK1 and ERK2 MAP kinase and NFkB pathways [Citation12,Citation13,Citation15–17]. In addition, Cr(VI) promotes the silencing of tumour suppressor genes, alters the transcriptional responses to co-toxicants, and promotes DNA damage in euchromatic regions of the genome [Citation18–22]. In this context, our earlier work examining the mechanisms that result in Cr-mediated gene repression found that Cr(VI) crosslinked a complex of HDAC1-DNMT1 to the promoter of several xenobiotic-inducible genes, maintaining a closed chromatin state and repressing transcription despite the presence of a transcription activating signal [Citation23].
Chromatin architecture plays an important role in the regulation of gene expression. Histone modifications are key regulators of the genome and serve as important marks to identify both active and inactive genome domains. In previous work, we used FAIRE-, ATAC- and MNase-seq to assess the extent of changes in chromatin accessibility that occur following treatment with Cr(VI) and identified a dose-dependent association between transcriptional changes and differentially-accessible chromatin [Citation24,Citation25]. Noteworthy, annotation of the enriched regions returned two transcription factors, AP-1 and CTCF, that demonstrated a significant differential accessibility under treatment conditions. Considered a ‘master weaver of the genome’, CTCF is a crucial component of chromatin organization, serving a multifaceted set of roles that include the mediation of chromatin-chromatin interactions, transcriptional activation and suppression, and insulation of histone signals, among others [Citation26–29]. Studies targeting its binding sites have demonstrated that inversions, mutations, and deletions can promote changes in local gene expression, while other studies have suggested that these sites are targets of mutagenesis in cancer progression [Citation30–32]. Furthermore, comparisons between normal and cancer cell lines have identified that chromatin topology is frequently restructured within Topologically Associated Domains (TADs), forming new contacts and disrupting established loops, which may be responsible for changes in transcriptional programming [Citation33].
Disruption of chromatin structure and changes in transcriptomic pathways suggest that Cr(VI) may promote tumorigenesis by disrupting the mechanisms that regulate gene expression at both the pathway and structural levels. In the current study, we tested the hypothesis that Cr(VI) disrupts the regulation of transcription by affecting CTCF’s capacity to recognize and bind its cognate sites. We used ChIP-seq to identify differentially bound sites following 1 µM chromium treatment for 48 hours, as well as its impact on histone profiles associated with transcription. Our findings support the notion that Cr(VI) targets euchromatic regions of the genome and suggests that differential binding of CTCF in the proximity of active genes may be an indication of regions with increased susceptibility to Cr(VI)-mediated disruption.
Results
Cr(VI) promotes differential CTCF binding near transcription start sites
We used ChIP-seq to examine the effect of Cr(VI) on CTCF binding in Hepa-1c1c7 cells following a 48-hour treatment with 1 µM potassium chromate. Samples were processed using the ENCODE ChIP-seq pipeline [Citation34,Citation35] with additional filtering criteria set to provide high-confidence CTCF peaks. To limit the inclusion of false positives [Citation36], we selected for domains that contained a CTCF motif and had a FDR value less than 0.01. After implementation of the exclusion criteria, we identified 46,394 regions that were used to test for differential binding. We found 365 enriched and 450 depleted sites with a significance of p-value 0.05 (). Examples of differential binding can be seen in Fig. S1. Though the number of differentially-bound peaks was similar, the strength of the signal appeared to be an important factor in the determination of increased or reduced binding. Differentially-bound sites with high levels of CTCF binding in controls trended towards reduction after Cr(VI) treatment, while weakly bound sites were the preferred target of Cr-enrichment (/b). Overall, the global population of sites tested exhibited a high degree of uniformity, suggesting that subsets of sites are affected, with the relative size of disruption being linked to the strength of the site’s binding capacity.
Figure 1. CTCF sites are differentially bound at TSS promoter regions of Cr(VI)-treated cells. CTCF ChIP-seq data for control and 1 µM Cr(VI)-treated Hepa-1 cells were processed using the ENCODE ChIP-seq pipeline [Citation34,Citation35] to provide a foundation for downstream analyses. Approximately 80,000 peaks were called for both conditions when compared to total input; this was further optimized for high confidence regions by selecting for peaks with an FDR 0.01 in the optimal set called by SPP [Citation61,Citation62], then using Homer [Citation64] to identify peaks that contained the canonical CTCF motif. (a) Following peak selection, DiffBind [Citation54,Citation55] was used to identify differentially-bound sites between Cr-treated and control samples. Points density was calculated by binning peaks into 200 segments, represented by their frequency. Sites with statistical significance threshold of p-value
0.05 are highlighted in blue and red. (b) DeepTools265 was used to assess the signal of differentially-bound sites in each subset. Signal values were computed for each replicate bigwig (1X normalized) with an extension of ± 1 Kb on the flanking regions. The mean value of the replicates for each bin is represented. (c) Homer [Citation64] was used to provide annotation statistics for the subsets of regions. Sites were progressively binned by their distance to the nearest TSS. The asterisks represent the mean of 500 repeated subsampling iterations with the standard error (selecting n = 365 for Cr-Enriched and n = 450 for Cr-Depleted) within the global peak population (n = 46,394) to provide an accurate representation of expected peak profiles. (d) Log2 ratios were calculated using Homer’s [Citation64] ‘annStats’ flag with default parameters. Red and blue values represent significant Cr-Enriched and Cr-Depleted peaks respectively; numbers next to each bar indicate the number of peaks in each category and annotations with 0 occurrences were excluded. Promoter regions are considered to be within −1 Kb to +100 bp of the TSS
![Figure 1. CTCF sites are differentially bound at TSS promoter regions of Cr(VI)-treated cells. CTCF ChIP-seq data for control and 1 µM Cr(VI)-treated Hepa-1 cells were processed using the ENCODE ChIP-seq pipeline [Citation34,Citation35] to provide a foundation for downstream analyses. Approximately 80,000 peaks were called for both conditions when compared to total input; this was further optimized for high confidence regions by selecting for peaks with an FDR ≤ 0.01 in the optimal set called by SPP [Citation61,Citation62], then using Homer [Citation64] to identify peaks that contained the canonical CTCF motif. (a) Following peak selection, DiffBind [Citation54,Citation55] was used to identify differentially-bound sites between Cr-treated and control samples. Points density was calculated by binning peaks into 200 segments, represented by their frequency. Sites with statistical significance threshold of p-value ≤ 0.05 are highlighted in blue and red. (b) DeepTools265 was used to assess the signal of differentially-bound sites in each subset. Signal values were computed for each replicate bigwig (1X normalized) with an extension of ± 1 Kb on the flanking regions. The mean value of the replicates for each bin is represented. (c) Homer [Citation64] was used to provide annotation statistics for the subsets of regions. Sites were progressively binned by their distance to the nearest TSS. The asterisks represent the mean of 500 repeated subsampling iterations with the standard error (selecting n = 365 for Cr-Enriched and n = 450 for Cr-Depleted) within the global peak population (n = 46,394) to provide an accurate representation of expected peak profiles. (d) Log2 ratios were calculated using Homer’s [Citation64] ‘annStats’ flag with default parameters. Red and blue values represent significant Cr-Enriched and Cr-Depleted peaks respectively; numbers next to each bar indicate the number of peaks in each category and annotations with 0 occurrences were excluded. Promoter regions are considered to be within −1 Kb to +100 bp of the TSS](/cms/asset/b622133b-8f56-472c-8b2d-a4eef07cf1db/kepi_a_1864168_f0001_oc.jpg)
Next, we examined the genomic context of these sites using multiple metrics. Each site was annotated for the closest transcription start site and the relative distances were progressively binned. To account for drastically different numbers between the overall population and statistically significant sites, the global population was separated into two categories based on the gain or loss of binding, then repeatedly subsampled (500 iterations, selecting n = 365 for Cr-Enriched and n = 450 for Cr-Depleted) to match the number of significant, differentially-bound sites matching the relative criteria (). Sites that were associated with a gain in binding tended to follow the global population; notably, sites with reduced binding exhibited a substantial increase in frequency around the proximal region of the TSS, specifically within ±500 base pairs (), suggesting the likelihood of a potential association between the loss of CTCF binding and the regulation of proximal genes. This was further corroborated through annotation statistics of the pooled, statistically significant peaks (n = 365 for Cr-Enriched and n = 450 for Cr-Depleted), which demonstrated enrichment in coding-related regions, specifically promoters for Cr-Depleted regions ().
There are several possible reasons that could cause binding reduction. To assess whether the changes found resulted from functional alterations or global depression/enrichment of the protein, we tested the mRNA and protein expression levels of CTCF and cohesin components. Expression of CTCF remained unchanged at both transcription and translation levels following 48 hours of 1 µM Cr(VI) treatment (Fig. S2). Given that this is a relatively short window of time for protein expression levels to change, we also tested cells that had been treated with varying concentrations of Cr(VI) for 5 and 15 passages to examine whether chronic exposure had an impact on expression. At both time points, there were no discernible differences in the level of protein or mRNA expression, indicating that the overall levels of CTCF remain relatively stable with long-term treatment of Cr(VI) (Fig. S2). Cohesin components (RAD21, STAG1, SMC1/3) exhibited specific patterns similar to those of CTCF; gene expression of several cohesin components noted no significant change at 48 hours when compared to the untreated condition and protein expression was not appreciably affected at any of the time points (Fig. S2). Together, these findings suggest that binding variability is the result of functional interactions following Cr(VI) treatment, which result in changes of CTCF binding.
Genes near differentially-bound CTCF sites have functional roles in the response to Cr(VI) exposure
Early studies hypothesized that CTCF serves as a regulator of transcription through multiple mechanisms, including activator and suppressor roles [Citation37,Citation38], insulation of epigenetic marks [Citation39], and orchestration of three-dimensional chromatin organization [Citation40–42]. While its role in the organization of chromatin has been further supported, new evidence suggests that its influence on gene expression may be the result of facilitating enhancer-promoter chromatin interactions as well as shielding promoters from the activity of neighbouring enhancers [Citation43]. Accordingly, we hypothesized that differential CTCF binding could lead to changes in the transcriptional regulation of adjacent genes. Following annotation, we identified 206 unique genes that had a differentially-bound CTCF site within ±5 Kb of their TSS and cross-referenced them with expression data from our previously published RNA-seq ()[Citation44]. While the mean log2 fold-change is slightly higher than 0, or no change, 43 genes showed an absolute log2 fold change of 0.5 or greater, with 16 being statistically significant (adjusted p-value 0.05) and skewed towards upregulation. Notably, one of the most significant genes identified in the Cr-depleted subset was a subunit of the glutamate-cysteine ligase, Glcm, which may be implicated in Cr(VI)-mediated toxicity through its role in remediation of oxidative stress [Citation22]. Interestingly, the mean profile of the active and suppressive marks, H3K36me3 and H3K27me3 respectively, suggests that the genes were generally activated () in controls with a mean signal similar to genes in the 50% to 60% ranked expression percentile and remained so after treatment (Fig. S3). To better understand whether there may be functional associations attached to these changes, we used Enrichr [Citation45,Citation46] to annotate biological processes that each subset of genes may be involved in (). Both the enriched and depleted subsets were found to be involved in pathways associated with Cr(VI) exposure; in particular, pathways with CTCF-depleted genes in the proximal-promoter regions were involved in chromatin-related functions such as double-strand break repair and EGFR signalling, while enriched pathways included the regulation of IGF-LR signalling, DNA replication, and regulation of membrane potential [Citation10,Citation21,Citation47–51]. Taken together, these results suggest that differential binding of CTCF occurs near active genes, specifically in proximity to genes with functional roles in the cell’s response to Cr(VI) exposure.
Figure 2. Genes near affected CTCF sites are functionally important and exhibit transcriptional change. Following the annotation of differentially-bound CTCF sites, a subset of genes with an affected site was selected using a cut-off of TSS ± 5 Kb. (a) The log2 fold change in transcription for this subset of genes was mapped using previously published RNA-seq data from our lab (GSE49571, Table S5)[Citation44]. Genes are coloured by the differential-binding status of the CTCF site with select labelling. The dotted line represents the threshold of an adjusted p-value 0.05. (b) Histone modification profiles across genes in each subset were quantified using DeepTools265 and the mean signal from each sample subset is represented. (c) Enrichr [Citation45,Citation46] was used to provide functional annotations for same gene subsets. The top 10 biological processes, as ranked by the -log10 p-value, are represented
![Figure 2. Genes near affected CTCF sites are functionally important and exhibit transcriptional change. Following the annotation of differentially-bound CTCF sites, a subset of genes with an affected site was selected using a cut-off of TSS ± 5 Kb. (a) The log2 fold change in transcription for this subset of genes was mapped using previously published RNA-seq data from our lab (GSE49571, Table S5)[Citation44]. Genes are coloured by the differential-binding status of the CTCF site with select labelling. The dotted line represents the threshold of an adjusted p-value ≤ 0.05. (b) Histone modification profiles across genes in each subset were quantified using DeepTools265 and the mean signal from each sample subset is represented. (c) Enrichr [Citation45,Citation46] was used to provide functional annotations for same gene subsets. The top 10 biological processes, as ranked by the -log10 p-value, are represented](/cms/asset/f73eb989-16c4-4149-99d2-25fbb288e9f5/kepi_a_1864168_f0002_oc.jpg)
Chromium-induced changes in chromatin states are localized effects
Histone modifications are key components in the regulation of gene transcription, serving as dynamic signalling mechanisms that can modulate chromatin accessibility and activity through epigenetic writers, readers, and erasers. CTCF often serves as an insulator of these signals through genomic compartmentalization and the formation of insulated neighbourhoods. While CTCF is typically found at the demarcating locations of TADs, new evidence suggests that its depletion does not promote the spread of heterochromatin domains directly [Citation43]. Our previous findings suggest that Cr(VI) elicits broad changes in chromatin architecture and that CTCF disruption may cause changes in epigenetic profiles to promote or suppress gene transcription [Citation24,Citation25,Citation44]. To test this hypothesis, we used ChIP-seq analyses of multiple histone modifications to assess how Cr(VI) treatment affected the strength and propagation of activation, suppression, and enhancer marks throughout the genome. Genome-wide correlation using 1-Kb windows showed no substantial deviations in the behaviour of the signal between control and Cr(VI) (), indicating that differences are potentially the result of targeted effects rather than a systemic change in histone modification activity. To better assess these fluctuations, we used the ChromstaR [Citation52] package to map combinatorial chromatin states for both control and treated samples (an example region is provided in Fig. S4). In agreement with the global correlation, approximately 83% of the genome retained the same state classification, while 16% was estimated to have a transition (). We therefore quantified the number of genomic regions within each combination in controls and compared them to treated samples to try and infer what types of transitions are most common following treatment (uncategorized regions were not included). Certain histone marks, such as H3K4me3, were far more prominent than others and exhibited higher degrees of variability (Fig. S5). Importantly, this is not necessarily a reflection of the proportion of the affected genome, as evidenced by the H3K27me3 mark; while the number of domains called appears to be fewer than the H3K4me3 classification, the size of the region included in each domain is larger.
Figure 3. Changes in chromatin states are localized effects. DeepTools265 and ChromstaR [Citation52] were used to assess global signal patterns and characterize chromatin states. (a) Each biological replicate was used to measure the 1x normalized signal across the genome in 1 Kb bins. Spearman’s rank correlation was performed to determine the degree of similarity compared with all other samples and conditions. (b) Chromatin states were classified using ChromstaR [Citation52] with the mode set to ‘combinatorial’, a bin size 1 Kb bins, and a step size of 500 bp. States were considered ‘Static’ if there was no change in the classification between the control and Cr(VI)-treated samples, and ‘Differential’ (‘Diff’) if they were distinct. Following this categorization, the proportion of the genome that falls under each classification was summarized. (c) The global and differentially-bound set of CTCF peaks identified using DiffBind [Citation54,Citation55] were intersected with the subset of differential chromatin state transitions. To account for substantial differences in region numbers, the global population was repeatedly subsampled (n = 846, iterations = 500) and the mean is reported with the standard error
![Figure 3. Changes in chromatin states are localized effects. DeepTools265 and ChromstaR [Citation52] were used to assess global signal patterns and characterize chromatin states. (a) Each biological replicate was used to measure the 1x normalized signal across the genome in 1 Kb bins. Spearman’s rank correlation was performed to determine the degree of similarity compared with all other samples and conditions. (b) Chromatin states were classified using ChromstaR [Citation52] with the mode set to ‘combinatorial’, a bin size 1 Kb bins, and a step size of 500 bp. States were considered ‘Static’ if there was no change in the classification between the control and Cr(VI)-treated samples, and ‘Differential’ (‘Diff’) if they were distinct. Following this categorization, the proportion of the genome that falls under each classification was summarized. (c) The global and differentially-bound set of CTCF peaks identified using DiffBind [Citation54,Citation55] were intersected with the subset of differential chromatin state transitions. To account for substantial differences in region numbers, the global population was repeatedly subsampled (n = 846, iterations = 500) and the mean is reported with the standard error](/cms/asset/18d091e2-489a-4576-b0ba-38a3e8fc14bd/kepi_a_1864168_f0003_oc.jpg)
Following the general characterization of combinatorial chromatin states between control and Cr-treated conditions, we investigated whether differential CTCF-binding events occur in regions demonstrating changes in their state classification. An intersection of these targeted sites revealed that the majority of the differentially bound CTCF sites are located in static states, while approximately 39% are found in regions with varied state classifications (). While a small number of these sites were found in regions defined as treatment-specific, most were defined as other; hence, we collectively considered these groups as differential transitions. The process was repeated for the global set of peaks, which was repeatedly subsampled (n = 846, iterations = 500) to estimate whether differential binding of CTCF results in an increased rate of state transition following chromium treatment. Altogether, differentially-bound CTCF sites were slightly enriched over the global mean estimate, though the results suggest that alterations in CTCF binding affinity at these sites due to Cr(VI) treatment do not preferentially induce proximal chromatin state transitions when compared to the global population of sites.
Cr(VI) restructures intra-TAD chromatin loops
CTCF and cohesin are well-established as key components of chromatin architecture and organization, frequently found together at TAD domain boundaries and intra-TAD loops. These connections are important in the regulation of transcription, and their disruption may be a driver of carcinogenesis. In recent years, algorithms have been developed which predict chromatin-chromatin contacts based on ChIP-seq data and the characterization of known interactions. Consequently, we generated sets of predicted intra-TAD loops for control and Cr-treated samples by using the algorithm proposed by Matthews and Waxman [Citation53].
Processing control and Cr-treated samples identified 5,189 and 5,528 predicted intra-TAD loops, respectively (). Overall, the sizes of the loops were very similar with a median of approximately 140 Kb in the control and 146 Kb in the treated samples, indicating that any changes are likely to be localized effects rather than a complete shift in the segmentation of chromatin loops (). This is further supported by similar signal profiles for each histone modification per treatment across its set of loops, though the strength of enrichment is different between treatments (). Specifically, H3K27ac and H3K36me3 exhibit a generalized increase in signal across looping regions, while H3K4me3 shows a decrease in signal at each of the anchor sites. To identify whether unique looping interactions were reflective of the signal changes, we used an intersection analysis to identify three sets of regions based on their overlapping status. Loops were considered common, when loop pairs overlapped by a reciprocal fraction of 0.99 or more, and treatment-specific, otherwise. A total of 2,268 common loops were identified in both sets of samples, representing slightly less than half of each set (Fig. S6). Cross comparison between control and treated signals in each of the respective sets suggests that this may be the result of certain biases in peak ranking or selection, as both are nearly identical regardless of the loop classification (Fig. S7).
Figure 4. IntraTAD chromatin loop predictions suggest subtle differences in enrichment of histone modification signals. The algorithm proposed by Matthews and Waxman was implemented independently for control and treated conditions with minor modifications to capture as many potential loops as possible prior to merging [Citation53]. (a) The number of distinct, predicted intra-TAD interactions for each condition were quantified. (b) Loop size was then calculated as the distance between each candidate pair’s start and end. (c) Loop candidates were passed to DeepTools265 to measure histone modification signals across candidate loop regions which were normalized to a common length and then extended by 5 Kb in either direction to quantify signal activity outside of the looping interactions using a bin size of 100 bp. The mean of each bin is presented. The symbols A(l)/A(r) represent the left and right anchors of the loop, respectively
![Figure 4. IntraTAD chromatin loop predictions suggest subtle differences in enrichment of histone modification signals. The algorithm proposed by Matthews and Waxman was implemented independently for control and treated conditions with minor modifications to capture as many potential loops as possible prior to merging [Citation53]. (a) The number of distinct, predicted intra-TAD interactions for each condition were quantified. (b) Loop size was then calculated as the distance between each candidate pair’s start and end. (c) Loop candidates were passed to DeepTools265 to measure histone modification signals across candidate loop regions which were normalized to a common length and then extended by 5 Kb in either direction to quantify signal activity outside of the looping interactions using a bin size of 100 bp. The mean of each bin is presented. The symbols A(l)/A(r) represent the left and right anchors of the loop, respectively](/cms/asset/d8cee5bb-74fe-495c-b462-976c0ee2dc18/kepi_a_1864168_f0004_oc.jpg)
We took a separate approach to examine potential changes in chromatin looping by investigating the sites that serve as anchors. A list of unique anchor sites was generated by pooling the predicted loops from each condition and selecting unique CTCF sites found at either the start or end locations (original sites used to predict the loop). Out of the total number of peaks considered by DiffBind [Citation54,Citation55] for the CTCF samples, 7,548 were mapped to predicted anchor sites. The mean signal profiles of each histone modification were calculated for the predicted anchors as well as all CTCF sites (), with possible anchors showing markedly different profiles when compared to the total peak set. Specifically, CTCF enrichment was increased in the anchor subset whereas the H3K27ac, H3K27me3, and H3K4me3 marks were depressed at the peak centre suggesting that these sites serve important regulatory roles. To determine whether peaks in the anchor subset were potential targets of Cr-related fluctuations, we applied the exclusion criteria mentioned earlier () to identify 157 significant, differentially-bound peaks from the predicted anchor set (7,548), with 138 of the sites exhibiting a reduction in CTCF binding. As noted earlier (), CTCF anchors that were reduced (‘Cr-Depleted’) tended to have stronger levels of initial binding, while those that showed an increase (‘Cr-Enriched’) were lower than average (). Though the number of sites in each set are very different, the signal profiles of the histone modifications suggest that the site susceptibility of CTCF may be driven by its epigenetic patterning. Of the marks tested, H3K27ac and H3K4me3 showed strong distinctions between each anchor group that appear to have a measurable impact. Specifically, while the mean anchor signals of these marks were relatively minimal compared with the general peak population (), sites with significantly reduced CTCF binding had signal profiles that were nearly as strong as the global signal across all peaks, but also showed a clear distinction at the centre of the peak (). Additionally, Cr(VI) treatment increased the signal of H3K27ac and decreased H3K4me3 at sites with reduced CTCF binding, although the H3K4me3 change was minimal. In contrast, the signal profile of H3K27ac across differentially-bound sites that were in the ‘Cr-Enriched’ group was much more consistent with the mean signal of all predicted anchors whereas H3K4me3 showed an intermediate signal increase. The suppressive marks H3K27me3 and H3K9me3, as well as the active mark H3K36me3, displayed enriched signals in the ‘Cr-Enriched’ set compared to the other groups. However, when comparing the strength of these signals () to that of each mark’s respective global peak signal (), they still appear to be minimally enriched. Hence, while these profiles are very different, the marks that appear to be the major drivers between each of the groups and treatments are H3K27ac and H3K4me3. In conclusion, the implementation of intra-TAD looping prediction algorithms provides an important meta-contextual layer to help identify that significantly reduced CTCF sites are frequently marked as anchors for chromatin-chromatin interactions, susceptible to aberrant shifts in transcription regulation (Fig. S8).
Figure 5. Differentially-bound anchors display altered histone modification profiles. Predicted loop regions were intersected with the population of CTCF sites tested in DiffBind [Citation54,Citation55] to identify peaks that are predicted to be anchors. (a) Regions were considered as anchors if the start or end value was found within a peak. A total of 7,548 peaks were classified as potential anchors prior to thresholding (also termed ‘Predicted Anchors’). Signals for each histone modification were calculated using DeepTools265 computeMatrix in reference-point mode. (b) Anchor peaks were then filtered to select significant sites (pval 0.05) and classified as either ‘Cr-Enriched’ or ‘Cr-Depleted’ based on the log2 fold change. Data is represented as the mean signal per bin for each group and the number of observations are provided in parentheses
![Figure 5. Differentially-bound anchors display altered histone modification profiles. Predicted loop regions were intersected with the population of CTCF sites tested in DiffBind [Citation54,Citation55] to identify peaks that are predicted to be anchors. (a) Regions were considered as anchors if the start or end value was found within a peak. A total of 7,548 peaks were classified as potential anchors prior to thresholding (also termed ‘Predicted Anchors’). Signals for each histone modification were calculated using DeepTools265 computeMatrix in reference-point mode. (b) Anchor peaks were then filtered to select significant sites (pval ≤ 0.05) and classified as either ‘Cr-Enriched’ or ‘Cr-Depleted’ based on the log2 fold change. Data is represented as the mean signal per bin for each group and the number of observations are provided in parentheses](/cms/asset/8dbb10f9-58b5-418f-b122-982cd0278b35/kepi_a_1864168_f0005_oc.jpg)
Discussion
Our prior work using ATAC-seq found that the CTCF sites that were enriched in differentially-accessible chromatin by Cr(VI) treatment were located near the proximal promoter domains of genes[Citation25]. Here, we provide additional context to these findings by measuring the effect of Cr(VI) on both CTCF binding and histone modifications that are established marks of transcriptional activation or suppression. We identify subsets of CTCF that show differential binding affinities following Cr(VI) treatment and describe the characteristics that may determine the direction of change. Significantly reduced binding sites are overwhelmingly comprised of regions that have strong binding affinities. These regions are in proximity to coding domains, specifically, transcription start sites, suggesting that these cognate sites are functionally important in the regulation of local genes. To establish whether differential binding results in transcriptional changes, we referenced previous RNA-seq to map gene activity. To test whether gene activity was consistent between the RNA-seq and our current study, we ranked the mean RPKM scores of each gene in the RNA-seq controls and measured the histone modification signals that are associated with active (H3K36me3) and suppressed (H3K27me3) transcription within each bin, finding a high degree of correlation (Fig. S3). Using the mean signals of each rank, we could estimate that the genes in the current study were moderately activated, with the mean signal of histone modifications being similar between control and treated conditions, though a marginal increase in H3K36me3 was reported. In agreement with previous findings [Citation24], the RNA-seq data for the genes closest to differentially bound CTCF sites suggest that disruption promotes a general trend towards increased transcription. These profiles provide indirect support for the hypothesis proposed by DeLoughery et al. that Cr-mediated DNA double-strand breaks are localized to euchromatic regions [Citation56]. While we are unable to determine whether these regions are enriched in DNA break formation, we can conclude that all three, changes in binding, proximity to differentially-expressed genes, and histone profiles, point at the conclusion that the CTCF sites disrupted by Cr(VI) are enriched in regions of accessible chromatin.
To develop a comprehensive, contextual understanding of the epigenomic environment after Cr(VI) treatment, we used ChromstaR [Citation52] to map combinatorial chromatin states across the genome and integrate genomic datasets. This approach allowed us to identify regions susceptible to changes in histone modification patterning and estimate the proportion of the genome that is affected by treatment. Overlap analysis of differentially-bound CTCF sites with regions exhibiting shifts in chromatin states found that approximately 30% of the CTCF sites were altered by treatment. It remains to be determined whether this is a shift occurring as a result of Cr(VI) disrupting CTCF directly, leading to a change in signal profile, or of Cr(VI) promoting enrichment of these particular signals leading to a change in CTCF binding. Interestingly, the histone marks which seem to be most affected are H3K4me3 and H3K27ac, highlighting the possibility that enhancer and promoter regions of active genes may be regions targeted by Cr(VI). Indeed, Chen et al. previously reported a global increase in H3K4me3 levels in human A549 following treatment with Cr(VI) [Citation57]. While our findings indicate that H3K4me3 was slightly decreased around the CTCF sites that we examined, it should be noted that differential binding analysis of control and Cr(VI)-treated samples also showed a generalized increase in the global signal, exhibiting a positive correlation between log2 fold change and binding strength (Fig. S9).
To assess the effects of altered CTCF binding on architectural integrity, we used the algorithm proposed by Matthews and Waxman, which predicts intra-TAD chromatin loops using CTCF and cohesin ChIP-seq data [Citation53]. Intra-TAD loops further compartmentalize topologically associated domains, providing an additional layer of control in the regulation of gene expression, and numerous studies have found that carcinogenesis can rewire these conformations and functionally impact the activity of the transcriptome [Citation58]. Therefore, we used our data to study whether changes in CTCF binding promoted transcriptome dysregulation through the disruption of anchor sites key to architectural integrity. Similar numbers of predicted loops were observed for control and treated samples and their characteristics were similar overall, suggesting that any disruption in loop formation was likely to be a localized effect and not necessarily systemic in nature. Following classification of each loop as either common or treatment specific, it was surprising to find that loops specific to a condition showed similar signal profiles between control and treated samples for CTCF and histone modifications (Fig. S7). Several explanations could account for this finding. On the one hand, despite having set relaxed criteria for inclusion, the volume of peaks called for one CTCF condition might have led to a truncation of all but the top sites when passed through the pipeline. As a result, these sites may indeed be present in the other condition, but their rank might have been adjusted and led to the exclusion in later steps of the analysis, resulting in a technical bias. On the other hand, changes in binding affinity of CTCF at specific sites due to Cr(VI) treatment may alter the sites that pass through the truncation. To distinguish between these alternatives, we used a different approach focusing on the anchor predictions through the integration of the DiffBind [Citation54,Citation55] analysis. When comparing the signal of histone modifications across differentially bound anchors, it became evident that H3K27ac and H3K4me3 played a substantial role in the determination of sites that result in a significant gain or loss in CTCF binding. In an analysis of architectural disorganization in the cancer genome, Taberlay et al. observed that cancer-specific chromatin-interactions were associated with changes in transcription and enriched at enhancers, promoters, and insulators [Citation59]. Consistent with these findings, we have observed that Cr(VI) affects gene expression in areas that are differentially accessible [Citation24]. Given that H3K27ac is elevated in these regions following Cr(VI) treatment, it is plausible that differentially bound anchors may be the result of changes that affect enhancer activity or enhancer-promoter interactions. Importantly, these findings require validation in targeted studies, as current computational approaches suffer from a high rate of false positives [Citation60]. Nevertheless, that should not detract from the value that these types of predictions have: regions identified by integrated and predictive analyses can be further studied in a targeted manner.
In summary, in the present study we have investigated the effect of Cr(VI) on CTCF binding and characterized the local impact on transcription regulation. While it remains to be determined whether changes in binding affinity are the result of direct interactions between chromium and CTCF, our results suggest that disruption of CTCF in the presence of Cr(VI) affects regions with distinct, functionally important characteristics. Further studies are needed to investigate the degree to which these outcomes affect targeted transcription.
Materials and Methods
Cell Culture
Hepa-1c1c7 cells (mouse hepatoma, ATCC) were cultured in alpha-minimum essential medium (Gibco, 12,000–022) supplemented with 5% foetal bovine serum (Sigma, F2442) and 1% penicillin-streptomycin (Gibco, 15,140–122) at 37ºC under 5% CO2 conditions. Cells were passaged, treated, and collected between 80–90% confluency.
Reagents
Potassium chromate (Fisher Chemical, P220-500), hereinafter referred to as Cr(VI) or simply chromium, was freshly prepared in double-distilled H2O and filtered through a 0.22 µm filter prior to use.
ChIP-seq
Samples were prepared in biological duplicate, with each obtained by pooling four independently treated 15 cm dishes to achieve a high volume of chromatin. ChIP-seq was performed with slight modifications using methods detailed previously [Citation25]. In brief, approximately 50 million cells per treatment were fixed in 1% formaldehyde for 10 minutes at room temperature and subsequently quenched with 0.125 M glycine for 5 minutes. Fixed cells were rinsed and collected in ice-cold PBS supplemented with a protease inhibitor cocktail (Roche, 05–056-489-001). Following collection, cells were lysed to isolate nuclei which were then resuspended in nuclei lysis buffer to a concentration of 10,000 nuclei/µl. Ten rounds of 6 minute sonication cycles (30 seconds on, 30 seconds off) were performed using a Diagenode BioRuptor (Diagenode, UCD-200) to shear chromatin to a range between 150–600 bp. Lysates were clarified and the sheared chromatin was diluted in IP dilution buffer prior to aliquoting. Antibodies were applied to aliquots and incubated overnight at 4°C, followed by antigen-antibody complex retrieval using MagnaChIP A/G beads (EMD Millipore, 16–663). Samples were washed three times in low-salt, high-salt, and LiCl buffers before eluting at 37°C in elution buffer. Eluates were de-crosslinked and treated with RNase A and Proteinase K before purifying DNA using the ChIP DNA Clean and Concentrator Kit (Zymo, D5205). DNA yield was measured by Qubit Fluorometric Quantitation using the dsDNA HS Assay Kit (Thermofisher, Q32851). Pulldowns were validated for successful enrichment prior to sequencing (data not shown) using the primers (IDTDNA) listed in Table S4. Information regarding antibodies used and application details can be found in Table S1.
NEBNext Ultra II DNA Library Prep Kit (NEB, Ipswich, MA) was used for library preparation with the following type of input: Immunoprecipitated DNA, fragmented DNA without immunoprecipitation as negative control, and non-template control. The DNA input was quantified by Qubit assay and a total of 1–10 ng DNA was used. The final PCR for library indexing and amplification was set to 7. Next, the cleaned library was analysed by Bioanalyzer (Agilent, Santa Clara, CA) using DNA high sensitivity chip to check its quality. On average an expected library insert size of 250–300 bp was shown with no amplification identified in the non-template control. Finally, to accurately quantify the library for the clustering, the library concentration was qPCR measured by NEBNext Library Quant Kit (NEB) using QuantStudio 5 Real-Time PCR Systems (Thermo Fisher). Finally, individually indexed and compatible libraries were pooled and sequenced using HiSeq 1000 sequencer (Illumina, San Diego, CA). Under the sequencing setting of single read 1 × 51 bp, about 50 million pass filter reads per sample were generated for sequencing analysis.
Sequencing Analysis
Fastq files were processed using the ENCODE ChIP-seq pipeline [Citation34,Citation35]. Unless otherwise noted, SPP’s [Citation61,Citation62] optimal IDR-thresholded peaks were used for downstream transcription factor analyses and MACS2 [Citation63] optimal IDR-thresholded peaks were used for histone modifications. Differential binding analysis was performed on peaks with a q-value 0.01 using the DiffBind [Citation54,Citation55] package and regions with less than 10 reads were filtered. Additionally, Homer [Citation64] was used to annotate CTCF peaks prior to differential binding analysis to select for sites which contain the CTCF motif. General peak annotations and were performed using Homer’s [Citation64] annotatePeaks.pl tool. DeepTools2 [Citation65] was used to generate the 1X RPGC-normalized bigwig files from combined bam files and calculate signal values. Gene set enrichment analysis was performed using Enrichr [Citation45,Citation46] and information from the ‘GO Biological Process 2018’ database was reported. Overlapping regions were calculated using the intersectBed command in the BEDTools suite [Citation66]. Combinatorial chromatin state maps were calculated and analysed using the ChromstaR [Citation52] package in combinatorial mode with default settings, a bin size of 1,000 bp, and a step size of 500 bp. Intra-TAD loop predictions were generated using the scripts provided by Matthews and Waxman in their publication Citation[53]; we used MEME Suite v4.12.0 [Citation67] and made minor modifications to their pipeline for compatibility. General data processing, analysis, and visualization was performed using R [Citation68] and packages in the Tidyverse library [Citation69]. A list of relevant utilities and versions can be found in Table S2.
RT-qPCR
Cells were lysed using TRIzol (Invitrogen, 15,596,026) and the aqueous phase was retained following phase separation with chloroform. RNA was then precipitated overnight at −80°C using isopropanol, washed with ethanol, and resuspended in RNase-free water (IDTDNA, 11–05-01-04). Yields were quantified using a Nanodrop 1000 (ThermoFisher Scientific). RNA was converted to cDNA using Superscript III reverse transcriptase (ThermoFisher Scientific, 18,080,093) and random primers (ThermoFisher Scientific, 48,190,011). Gene expression was measured on an Mx3000P qPCR System (Agilent) using the primers (IDTDNA) listed in Table S4 and the ddCt method.
Western blotting
Protein expression was quantified using methods previously described [Citation5]. Briefly, cells were collected and lysed in NETN buffer, followed by brief sonication using a Diagenode BioRuptor (Diagenode, UCD-200). Protein lysates were clarified using centrifugation and concentrations were quantified using the Pierce BCA assay (Pierce, 23,227) on Wallac 1420 Victor2 microplate reader (Perkin Elmer, 8381–30-1005). 15 µg aliquots were boiled in Laemmli loading buffer (CSH) prior to protein separation using SDS-PAGE. Following separation, proteins were transferred to PVDF membranes overnight at 4°C. Membranes were blocked with 5% (w/v) non-fat milk in TBST for 1 hour at room temperature, followed by antibody incubation. Blots were washed with TBST and probed for 1 hour at room temperature using HRP-conjugated secondary antibodies. Blots were subjected to further TBST washes and rinsed in TBS before developing the signal on a C-DiGit Blot Scanner (Li-Cor) using SuperSignal West Pico (Thermo Scientific, PI34080). Information regarding antibodies used and application details can be found in Table S3.
Data availability
Sequencing data has been uploaded to Geo Omnibus and is available with the accession number GSE154387. Details for previously published datasets are provided in Table S5.
List of abbreviations
Supplemental Material
Download PDF (3.3 MB)Acknowledgments
We thank Dr. Ying Xia for a critical reading of the manuscript. This research was supported by NIEHS grants R01 ES010807, and by the NIEHS Center for Environmental Genetics grant P30 ES06096. A.V.H. is supported by the NIEHS Training Grant T32 ES007250.
Disclosure statement
The authors declare no conflict of interest.
Supplementary material
Supplemental data for this article can be accessed here.
Additional information
Funding
References
- Wilbur S, Abadin H, Fay M, et al. Toxicological profile for chromium. 2012.
- Deng Y, Wang M, Tian T, et al. The effect of hexavalent chromium on the incidence and mortality of human cancers: a meta-analysis based on published epidemiological cohort studies. Front Oncol. 2019;9:24.
- Howard J Criteria for a recommended standard occupational exposure to hexavalent chromium DHHS (NIOSH) publication No. 2013–128. 2013.
- Thompson CM, Proctor DM, Haes LC, et al. Investigation of the mode of action underlying the tumorigenic response induced in B6C3F1 mice exposed orally to hexavalent chromium. Toxicol Sci. 2011;123:58–70.
- Sanchez-Martin FJ, Fan Y, Carreira V, et al. Long-term coexposure to hexavalent chromium and B[a]P causes tissue-specific differential biological effects in liver and gastrointestinal tract of mice. Toxicol Sci. 2015;146:52–64.
- Thompson CM, Seiter J, Chappell MA, et al. Synchrotron-based imaging of chromium and γ -H2AX immunostaining in the duodenum following repeated exposure to Cr(VI) in drinking water. Toxicol Sci. 2015;143:16–25.
- Sun H, Brocato J, Costa M. Oral chromium exposure and toxicity. Curr Environ Health Rep. 2015;2:295–303.
- De Flora S, Camoirano A, Bagnasco M, et al. Estimates of the chromium(VI) reducing capacity in human body compartments as a mechanism for attenuating its potential toxicity and carcinogenicity. Carcinogenesis. 1997;18:531–537.
- Zhitkovich A. Chromium in drinking water: sources, metabolism, and cancer risks. Chem Res Toxicol. 2011;24:1617–1629.
- Zhitkovich A. Importance of chromium-DNA adducts in mutagenicity and toxicity of chromium(VI). Chem Res Toxicol. 2005;18:3–11.
- Reynolds, M, Stoddard L, Bespalov I, et al. Ascorbate acts as a highly potent inducer of chromate mutagenesis and clastogenesis: linkage to DNA breaks in G2 phase by mismatch repair. Nucleic Acids Res. 2006;35(2):465–476.
- Ha L, Ceryak S, Patierno SR. Chromium(VI) activates ataxia telangiectasia mutated (ATM) protein requirement of ATM for both apoptosis and recovery from terminal growth arrest. J Biol Chem. 2003;278:17885–17894.
- Ha L, Ceryak S, Patierno SR. Generation of S phase-dependent DNA double-strand breaks by Cr(VI) exposure: involvement of ATM in Cr(VI) induction of γ -H2AX. Carcinogenesis. 2004;25:2265–2274.
- Holmes AL, Wise SS, Sandwick SJ, et al. Chronic exposure to lead chromate causes centrosome abnormalities and aneuploidy in human lung cells. Cancer Res. 2006;66:4041–4048.
- Chen L, Ovesen JL, Puga A, et al. Distinct contributions of JNK and p38 to chromium cytotoxicity and inhibition of murine embryonic stem cell differentiation. Environ Health Perspect. 2009;117:1124–1130.
- Kim G, Yurkow EJ. Chromium induces a persistent activation of mitogen-activated protein kinases by a redox-sensitive mechanism in H4 rat hepatoma cells. Cancer Res. 1996;56:2045–2051.
- Chen F, Ye J, Zhang X, et al. One-electron reduction of chromium(VI) by γ -lipoic acid and related hydroxyl radical generation, dG hydroxylation and nuclear transcription factor- ĸ B activation. Arch Biochem Biophys. 1997;338:165–172.
- Holmes AL, Wise SS, Pelsue SC, et al. Chronic exposure to zinc chromate induces centrosome amplification and spindle assembly checkpoint bypass in human lung fibroblasts. Chem Res Toxicol. 2010;23:386–395.
- Qin Q, Xie H, Wise SS, et al. Homologous recombination repair signaling in chemical carcinogenesis: prolonged particulate hexavalent chromium exposure suppresses the Rad51 response in human lung cells. Toxicol Sci. 2014;142:117–125.
- Wise SS, Holmes AL, Wise Sr JP. Hexavalent chromium-induced DNA damage and repair mechanisms. Rev Environ Health. 2008;23:39–58.
- Xie H, Holmes AL, Young JL, et al. Zinc chromate induces chromosome instability and DNA double strand breaks in human lung cells. Toxicol Appl Pharmacol. 2009;234:293–299.
- Fan Y, Ovesen JL, Puga A. Long-term exposure to hexavalent chromium inhibits expression of tumor suppressor genes in cultured cells and in mice. J Trace Elem Med Biol. 2012;26:188–191.
- Schnekenburger M, Talaska G, Puga A. Chromium cross-links HDAC1 DNMT1 complexes to chromatin inhibiting histone remodeling marks critical for transcriptional activation. Mol Cell Biol. 2007. DOI:https://doi.org/10.1128/MCB.00838-07
- Ovesen JL, Fan Y, Zhang X, et al. Formaldehyde-assisted isolation of regulatory elements (FAIRE) analysis uncovers broad changes in chromatin structure resulting from hexavalent chromium exposure. PloS One. 2014;9. DOI:https://doi.org/10.1371/journal.pone.0097849.
- VonHandorf A, Sanchez-Martin FJ, Biesiada J, et al. Chromium disrupts chromatin organization and CTCF access to its cognate sites in promoters of differentially expressed genes. Epigenetics. 2018;13:363–375.
- Lutz M, Burke LJ, Barreto G, et al. Transcriptional repression by the insulator protein CTCF involves histone deacetylases. Nucleic Acids Res. 2000;28:1707–1713.
- Ong C-T, Corces VG. CTCF: an architectural protein bridging genome topology and function. Nat Rev Genet. 2014;15:234–246.
- Phillips JE, Corces VG. CTCF: master weaver of the genome. Cell. 2009;137:1194–1211.
- Splinter E, Heath H, Kooren J, et al. CTCF mediates long-range chromatin looping and local histone modification in the β-globin locus. Genes Dev. 2006;20:2349–2354.
- Filippova GN, Qi CF, Ulmer JE, et al. Tumor-associated zinc finger mutations in the CTCF transcription factor selectively alter its DNA-binding specificity. Cancer Res. 2002;62:48–52.
- Klenova EM, Morse III HC, Ohlsson R, et al. The novel BORIS + CTCF gene family is uniquely involved in the epigenetics of normal biology and cancer. In: Seminars in cancer biology. vol. 12. Elsevier, 2002:399–414.
- Katainen R, Dave K, Pitkänen E, et al. CTCF/cohesin-binding sites are frequently mutated in cancer. Nat Genet. 2015;47:818–821.
- Tang Z, Luo OJ, Li X, et al. CTCF-mediated human 3D genome architecture reveals chromatin topology for transcription. Cell. 2015;163:1611–1627.
- Consortium EP, others. An integrated encyclopedia of DNA elements in the human genome. Nature. 2012;489:57–74.
- Davis CA, Hitz BC, Sloan CA, et al. The Encyclopedia of DNA elements (ENCODE): data portal update. Nucleic Acids Res. 2018;46:D794–D801.
- Pickrell JK, Gaffney DJ, Gilad Y, et al. False positive peaks in ChIP-seq and other sequencing-based functional assays caused by unannotated high copy number regions. Bioinformatics. 2011;27:2144–2146.
- Zuin J, Dixon JR, van der Reijden MI, et al. Cohesin and CTCF differentially affect chromatin architecture and gene expression in human cells. Proc Nat Acad Sci. 2014;111:996–1001.
- Ohlsson R, Renkawitz R, Lobanenkov V. CTCF is a uniquely versatile transcription regulator linked to epigenetics and disease. Trends Genet. 2001;17:520–527.
- Cuddapah S, Jothi R, Schones DE, et al. Global analysis of the insulator binding protein CTCF in chromatin barrier regions reveals demarcation of active and repressive domains. Genome Res. 2009;19:24–32.
- Rubio ED, Reis DJ, Welcsh PL, et al. CTCF physically links cohesin to chromatin. Proc Nat Acad Sci. 2008;105:8309–8314.
- Rudan MV, Barrington C, Henderson S, et al. Comparative Hi-C reveals that CTCF underlies evolution of chromosomal domain architecture. Cell Rep. 2015;10:1297–1309.
- deWit E, Vos ES, Holwerda SJ, et al. CTCF binding polarity determines chromatin looping. Mol Cell. 2015;60:676–684.
- Nora E, Goloborodko A, Valton A, et al. Targeted degradation of ctcf decouples local insulation of chromosome domains from genomic compartmentalization. Cell. 2017;169:930–944. e22
- Ovesen JL, Fan Y, Chen J, et al. Long-term exposure to low-concentrations of Cr(VI) induce DNA damage and disrupt the transcriptional response to benzo[a]pyrene. Toxicology. 2014;316:14–24.
- Chen EY, Tan CM, Kou Y, et al. Enrichr: interactive and collaborative HTML5 gene list enrichment analysis tool. BMC Bioinformatics. 2013;14:128.
- Kuleshov MV, Jones MR, Rouillard AD, et al. Enrichr: a comprehensive gene set enrichment analysis web server 2016 update. Nucleic Acids Res. 2016;44:W90–W97.
- He J, Qian X, Carpentier R, et al. Repression of miR-143 Mediates Cr(VI)-Induced Tumor Angiogenesis via IGF-IR/IRS1/ERK/IL-8 Pathway. Toxicol Sci. 2013;134:26–38.
- Kim D, Dai J, Fai LY, et al. Constitutive activation of epidermal growth factor receptor promotes tumorigenesis of Cr(VI)-transformed cells through decreased reactive oxygen species and apoptosis resistance development. J Biol Chem. 2015;290:2213–2224.
- Reynolds MF, Peterson-Roth EC, Bespaloc IA, et al. Rapid DNA double-strand breaks resulting from processing of Cr-DNA cross-links by both MutS dimers. Cancer Res. 2009;69:1071–1079.
- Xie Y, Zhuang Z. Chromium(VI)-induced production of reactive oxygen species, change of plasma membrane potential and dissipation of mitochondria membrane potential in Chinese hamster lung cell cultures. Biomed Environ Sci. 2001;14:199–206.
- Zhitkovich A, Peterson-Roth E, Reynolds M. Killing of chromium-damaged cells by mismatch repair and its relevance to carcinogenesis. Cell Cycle. 2005;4:4050–4052.
- Taudt A, Nguyen MA, Heinig M, et al. chromstaR: tracking combinatorial chromatin state dynamics in space and time. bioRxiv. 2016;038612.
- Matthews BJ, Waxman DJ. Computational prediction of CTCF/cohesin-based intra-TAD loops that insulate chromatin contacts and gene expression in mouse liver. Elife. 2018;7:e34077.
- Stark R, Brown G, others. DiffBind: differential binding analysis of ChIP-Seq peak data. R Package Version. 2011;100:3–4.
- Ross-Innes CS, Stark R, Teschendorff AE, et al. Differential oestrogen receptor binding is associated with clinical outcome in breast cancer. Nature. 2012;481:389–393.
- DeLoughery Z, Luczak MW, Ortega-Atienza S, et al. DNA double-strand breaks by Cr(VI) are targeted to euchromatin and cause ATR-dependent phosphorylation of histone H2AX and its ubiquitination. Toxicol Sci. 2015;143:54–63.
- Chen D, Kluz T, Fang L, et al. Hexavalent chromium (Cr(VI)) down-regulates acetylation of histone H4 at lysine 16 through induction of stressor protein Nupr1. PloS One. 2016;11-17.
- Jia R, Chai P, Zhang H, et al. Novel insights into chromosomal conformations in cancer. Mol Cancer. 2017;16:173.
- Taberlay PC, Achinger-Kawecka J, Lun ATL, et al. Three-dimensional disorganization of the cancer genome occurs coincident with long-range genetic and epigenetic alterations. Genome Res. 2016;26(6):719–731.
- Al Bkhetan Z, Plewczynski D. Three-dimensional epigenome statistical model: genome-wide chromatin looping prediction. Sci Rep. 2018;8:1–11.
- Kharchenko PV, Tolstorukov MY, Park PJ. Design and analysis of ChIP-seq experiments for DNA-binding proteins. Nat Biotechnol. 2008;26:1351–1359.
- Landt SG, Marinov CK, Kundaje A, et al. ChIP-seq guidelines and practices of the ENCODE and modENCODE consortia. Genome Res. 2012;22:1813–1831.
- Zhang Y, Liu T, Meyer CA, et al. Model-based analysis of chip-seq (macs). Genome Biol. 2008;9:R137.
- Heinz S, Benner C, Spann N, et al. Simple combinations of lineage-determining transcription factors prime cis-regulatory elements required for macrophage and B cell identities. Mol Cell. 2010;38:576–589.
- Ramirez F, Ryan DP, Grüning B, et al. deepTools2: a next generation web server for deep-sequencing data analysis. Nucleic Acids Res. 2016;44:W160–W165.
- Quinlan AR, Hall IM. BEDTools: a flexible suite of utilities for comparing genomic features. Bioinformatics. 2010;26:841–842.
- Bailey TL, Boden M, Buske FA, et al. MEME SUITE: tools for motif discovery and searching. Nucleic Acids Res. 2009;37:W202–W208.
- Core Team R. R: A language and environment for statistical computing. R Foundation for Statistical Computing, 2020.
- Wickham H, Averick M, Bryan J, et al. Welcome to the tidyverse. J Open Source Software. 2019;4:1686.