ABSTRACT
Saliva and buccal samples are popular for epigenome wide association studies (EWAS) due to their ease of collection compared and their ability to sample a different cell lineage compared to blood. As these samples contain a mix of white blood cells and buccal epithelial cells that can vary within a population, this cellular heterogeneity may confound EWAS. This has been addressed by including cellular heterogeneity obtained through cytology at the time of collection or by using cellular deconvolution algorithms built on epigenetic data from specific cell types. However, to our knowledge, the two methods have not yet been compared. Here we show that the two methods are highly correlated in saliva and buccal samples (R = 0.84, P < 0.0001) by comparing data generated from cytological staining and Infinium MethylationEPIC arrays and the EpiDISH deconvolution algorithm from buccal and saliva samples collected from twenty adults. In addition, by using an expanded dataset from both sample types, we confirmed our previous finding that age has strong, non-linear negative correlation with epithelial cell proportion in both sample types. However, children and adults showed a large within-population variation in cellular heterogeneity. Our results validate the use of the EpiDISH algorithm in estimating the effect of cellular heterogeneity in EWAS and showed DNA methylation generally underestimates the epithelial cell content obtained from cytology.
Introduction
Cellular heterogeneity is a major potential confounder of epigenome-wide association studies (EWAS) due to the cell type-specific state of DNA methylation. This is particularly the case in oral samples, which are a mixture of epithelial cells from the ectoderm germ cell lineage and immune cells from the mesoderm lineage [Citation1–3]. We and others have found that cellular heterogeneity in oral samples is influenced by the method of sample collection, with buccal swabs containing a much higher proportion of epithelial cells than saliva [Citation2,Citation3]. We have also shown that epithelial cell proportion is also strongly influenced by age and oral health status [Citation3]. Deconvolution of cellular heterogeneity can be achieved by measuring the proportion of each cell type using cytology of collected cells or through algorithms that use DNA methylation data from specific cell types to generate estimates [Citation1,Citation2]. Such measures can then be used in EWAS models to correct for cellular heterogeneity. There are two studies comparing cytology estimates of tumour purity to DNA methylation-based estimates and mRNA expression-based [Citation4,Citation5]. However, to our knowledge, no study has compared these two methods for oral samples. We aimed to compare epithelial cell content of buccal samples via ORAcollect•DNA kits and saliva obtained via passive drool collected in Oragene•DNA kits, measured using cytology and estimated with the reference based EpiDISH algorithm [Citation2]. We hypothesized that estimations of epithelial cell content would be highly correlated between the two methods. In a sub-study, using customized ORAcollect•DNA collection instructions, we compared two similar methods of collection differing in collection site and duration.
Materials and methods
Participants
Twenty adult volunteers from Deakin University provided informed consent to collect one saliva sample and two buccal samples. Ethics approval was granted by the Human Research Ethics Committee of the Royal Children’s Hospital, Melbourne (#33,174) and Deakin University (2018–368). All methods were performed according to relevant protocols and regulations. Participants also completed an oral health questionnaire, which included questions about mouth injuries, oral infections, medications and smoking status (Supplementary Methods).
Oral sampling
Oral samples were obtained from participants under supervision of the research team. Participants were advised not to smoke, chew gum, or consume anything apart from water for the 30 minutes prior to providing samples. Ten minutes prior to sample collection, they were asked to rinse their mouth with water. Saliva samples were collected unstimulated via passive drool for three to five minutes to allow sufficient time to collect to the fill line (2 mL) of Oragene•DNA collection devices (OG; DNA Genotek Inc, Ottawa, Canada). One hundred microlitres of saliva were then smeared onto a microscope slide and immediately fixed with 95% ethanol for 10 minutes and left to dry at room temperature. Oragene DNA-stabilizing chemistry contained within the device was then released into the remaining sample. Following collection of saliva, two samples were collected from participants using ORAcollect•DNA (OC, DNA Genotek Inc, Ottawa, Canada), a sponge-tipped oral sample collection kit, sequentially using two collection methods. In the first (OCA) participants gently rubbed the sponge ten times in a back-and-forth motion in the furrow between their lower teeth and inner cheek on one side of their mouth. In the second (OCB), the sponge was rubbed up and down against the inside of the cheek twenty times then rubbed ten seconds in a back-and-forth motion in the furrows between their upper and lower right teeth and inner cheek on the opposite side of their mouth. Each sponge was wiped along the length of a standard size microscope slide and fixed as outlined for saliva. The sponge was then inserted into the ORAcollect•DNA tube containing DNA stabilizing chemistry, capped tightly and mixed by inversion 15 times.
Slide staining and microscopy
Slides were stained using Diff-Quik as detailed elsewhere [Citation6]. All slides were deidentified and analysed by two observers. Cell types were counted via bright field microscopy at 100x magnification in regions with adequate cell density. Counts were used if the discrepancy between observers was less than 10% of the total count of count for each cell type. Counts with a between observer discrepancy of greater than 10% were discarded and separate fields re-counted. A minimum of 50 epithelial cells and 100 cells total was counted. Cells were scored as epithelial cells or immune cells, the latter including segmented cells, lymphocytes and monocytes [Citation3].
DNA extraction
Genomic DNA was extracted from 0.5 mL of each oral sample using ethanol precipitation via prepIT•L2P kits (DNA Genotek Inc, Ottawa, Canada) following the manufacturer’s protocol. DNA concentration was measured using PicoGreen (Thermo Fisher Scientific, Canada) in a SpectraMax M2 plate-based fluorimeter (Molecular Devices, CA, USA). DNA quality was measured using a TapeStation (Agilent, Santa Clara, United States).
DNA methylation arrays
Following genomic DNA extraction from all the samples, these genomic DNA samples were treated with bisulphite to convert unmethylated cytosine into uracil and transformation of uracil into thymine by amplification. Genome-wide analysis of DNA methylation was assessed using Infinium MethylationEPIC arrays (Illumina, CA, USA) with probes of over 850,000 methylation sites at the GenoFIND Genomic Service Lab (DNA Genotek Inc, Ottawa, Canada). Hybridization and scanning were performed according to manufacturer’s instructions.
Pre-processing of illumina infinium array data
MethylationEPIC array analysis was performed using the R statistical programming language (www.R-project.org) and Bioconductor packages [Citation7]. Raw intensity data (IDAT) files were imported into R (3.6.3; http://cran.r-project.org/). Data quality was assessed using the minfi (v1.34.0) Bioconductor package [Citation7]. The MethylationEPIC probes were filtered by removing those with poor signal to noise ratio (mean detection p-value of >0.01), cross-reactivity to multiple genomic locations, containing a single nucleotide polymorphism at the CpG site, or map to sex chromosomes [Citation8]. Data was then subjected to subset-quantile within array normalization (SWAN) [Citation9], and between-array normalization (SQN) [Citation10]. The HEpiDISH/EpiDISH and Robust partial correlation (RPC) algorithms were applied to estimate proportions of epithelial, fibroblast and immune cells from MethylationEPIC array data [Citation11].
Data analysis
Descriptive statistical analyses were conducted on the age of the participant and proportions of epithelial and immune cells. The assumption of normality of the independent and dependent variables for each cell type was tested using the Shapiro-Wilk test. A Kruskal-Wallis ANOVA analysis was conducted to test for statistically significant differences in cell proportion and DNA yield between collection methods OCA, OCB and OG. In a post-hoc test, the Dunnett’s test with Bonferroni correction was applied to identify the relatively small but significant differences among collection methods. Variables collected from insufficient numbers of participants were not included in our statistical analysis.
Percentage of epithelial cells and estimated cell-type fractions from EpiDISH were graphed using box and whisker plots, which included information on interquartile range (boxes, 25th to 75th percentiles, boxes), median (horizontal lines), data within 5th-95th percentiles (whiskers), outliers (circles), and mean (crosses). The proportion of epithelial cells in oral samples estimated from cytology and DNA methylation was tested using a Pearson correlation coefficient. To investigate the age effect on epithelial cell content estimated using DNA methylation, the buccal and saliva sample data from this study was analysed along with eight of our other studies, three published [Citation3,Citation12,Citation13] and five unpublished, using a Spearman correlation coefficient due to the non-linearity of the relationship.
Results
Determination of epithelial and immune cell proportions using cytology
Slides from all twenty adults (mean age 26.9 years, range 21 to 48 years, 60% female) were analysable i.e., had sufficient cells for analysis. Seven individuals reported recent gum bleeding within the seven days preceding their collection day. Examples of microscopic fields of view are shown in . Epithelial cells were large, with low nuclear to cytoplasmic ratio and immune cells were much smaller with a high nuclear to cytoplasmic ratio. Immune cells included granulocytes with segmented nuclei, lymphocytes with round, dense nuclei surrounded by cytoplasm, monocytes with kidney-shaped nuclei. Between two and twenty fields of view at 100x magnification were required to score the minimum number of cells.
Figure 1. Examples of cellular morphology in oral samples. Representative fields of view from Diff-Quik staining of (a) saliva, 100x magnification and (b) OCA buccal sample, 400x magnification. Both samples contain large epithelial cells (Epi) with dense nuclei, and smaller immune cells, exemplified by lymphocytes (Lym), segmented cells (Seg) and monocytes (Mono).
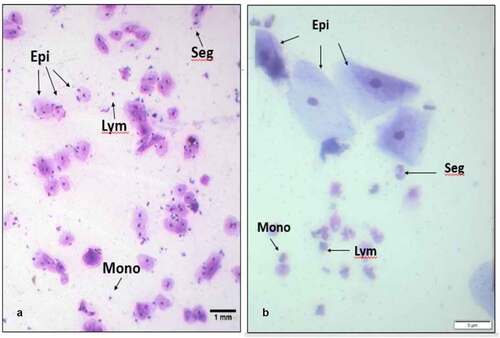
Results for estimations of epithelial cell proportions determined by cytology and DNA methylation analysis are shown in Supplementary Table 1 and . The mean proportion of epithelial cells in saliva (58%, SD 17.1%), was significantly lower than with sponge collection methods OCA (86.0%, SD 9.9%) and OCB (87.0%, SD 11.2%), p < 0.0001. There was a 28.5% mean difference (SD 6.5%) between saliva and cheek swab methods. There was no evidence for a difference in epithelial cell proportions between OCA and OCB (p = 0.6). There was also no evidence of an influence of recent gum bleeding (p value = 0.5) and sex (p value = 0.9) on epithelial cell proportion across all methods of sampling; results for individual oral collection methods were similar (data not shown).
Figure 2. Comparison of the percentage proportion of epithelial cells in oral samples, estimated using cytology and DNA methylation arrays, collected using three different methods (OCA, OCB and OG) estimated. Means are indicated with crosses. For both methods, the p-values of the difference between percentage of epithelial cells in OCA and OCB was > 0.05 and for the difference between percentage of epithelial cells of buccal sample collection (OCA and OCB) compared to saliva (OG) were < 0.0001.
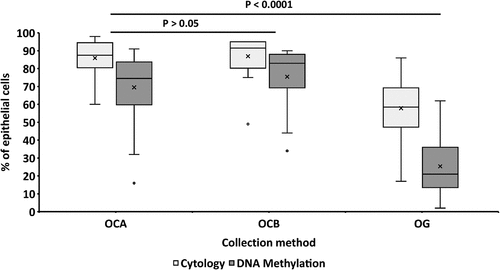
Determination of epithelial cell proportions using DNA methylation analysis
Saliva samples showed a significantly higher mean of total DNA yield per mL (33.7 µg, SD 24.2 µg) compared to oral sponge collection methods OCA (4.1 µg, SD 1.57 µg) and OCB (5.9 µg, SD 2.71 µg), p < 0.0001 for both comparisons (). Although DNA yield was approximately 1.7x higher in OCB compared to OCA, this difference was not significant (p = 0.083).
Figure 3. Range of DNA yields for each oral sample type. Box and whisker plots from saliva (OG) and the two methods of buccal sample collection (OCA and OCB). Means are indicated with crosses.
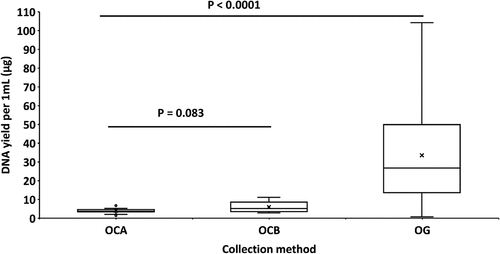
We next used the EpiDISH and robust partial correlation (RPC) algorithm on Infinium MethylationEPIC data to estimate cell type proportions. Although this method calculates proportion of epithelial, immune and fibroblast cell types, we found that the proportion of fibroblasts was negligible (mean = 0.4%) (Supplementary Table 1). As this meant that the proportion of immune and epithelial cells had a correlation of −1.0, we limited our analysis to the latter. As with cytology, the mean proportion of buccal epithelial cells determined by DNA methylation in saliva (25.4%, SD 17.1%), was significantly lower than cheek swab methods OCA (69.5%, SD 18.8%) and OCB (75.5%, SD 17.0%), p < 0.0001 (). A 47.1% mean difference with SD 0.7% in between saliva and cheek swab methods. There was no evidence for a difference in epithelial cell proportion between OCA and OCB (p = 0.11).
Comparison between epithelial cell proportions estimated using cytology and DNA methylation
To address our hypothesis that proportions of epithelial cells present in oral samples estimated using DNA methylation analysis represented the cell proportions as measured by cytology, we pooled all samples and compared both methods (). The two methods were strongly correlated (R = 0.84, P < 0.0001). However, the intercept of the line of best fit (methylation % = [1.32x cytology %] – 45%) on the x axis was 34%. A 20% mean difference of DNA methylation (SD 17.7%, IQR 63.7%) compared to cytology (SD 12.7%, IQR 50.8%) (Supplementary Table 1). Methods correlated similarly in buccals (R = 0.75, P < 0.0001) and saliva (R = 0.72, P < 0001) (Supplementary Figure 1).
An age effect on epithelial cell content in saliva
In our previous paper, we observed that epithelial cell content of buccal swabs and saliva was lower in adults compared to children [Citation3]. To investigate a possible age effect using epithelial cell content estimated using DNA methylation, we combined buccal swab and saliva data from this study with eight of our other Infinium array studies, including three published [Citation3,Citation12,Citation13] and five unpublished (, Supplementary Table 2). We found a strong, negative, non-linear, monotonic correlation between age and epithelial cell content estimated by DNA methylation (R = −0.77, p < 0.0001). We found a moderate relationship in both buccals (R = −0.61, P < 0.0001) and saliva (R = −0.64, P < 0.0001) (Supplementary Figure 2).
Figure 5. Comparison for epithelial cell content of oral samples. (a) The relationship between epithelial cell content of oral samples and age over eight studies. Studies are indicated by different colours. (b) Box and whisker plot from epithelial cell content of oral samples from eight studies. Numbers in brackets indicate the mean age of each study.
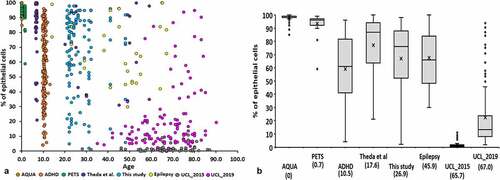
Discussion
Influences on epithelial content of oral samples
Buccal and saliva samples have a proven utility for epigenomics [Citation14,Citation15] and other cell-based omics [Citation16,Citation17]. As these samples are mixtures of epithelial and immune cells, deconvolution of these cellular mixtures is of utmost importance. Although cellular deconvolution algorithms based on reference sample types have been applied to epigenomic studies [Citation2], to our knowledge, the validity of such algorithms has not yet been tested using cytology of primary samples. We aimed to address this issue.
Our cytological analysis of adults with a mean age of 26 years showed that the epithelial content of ORAcollect•DNA (OC) samples was 86.5%, similar to the 83.4% we previously obtained using Copan flocked swabs in adults 16 years older [Citation3]. In the present study, epithelial cell content of saliva, but not buccal samples, was significantly higher than in our earlier study, which agrees with our previous finding that age has a much greater effect on saliva than on buccal samples. We also found that the epithelial content of ORAcollect•DNA collected samples was around 47% higher than that of saliva. This difference was 11% larger than that of our previous study, which may also reflect an age effect.
Our findings also suggest that the type of buccal collector has minimal influence on the proportion of epithelial cells collected and this may also be one reason why increasing collection time for OC sponge did not increase the proportion of epithelial cells collected, nor did it significantly increase DNA yield. However, future, larger studies are needed to further test our hypotheses. However, there may be a danger that longer collection times penetrate blood capillaries within the inner cheek, which would increase the proportion of immune cells, which may negate any rise in epithelial cell numbers.
We found no evidence that recent gum bleeding influenced the proportions of epithelial cells with either mode of sample collection. This disproved our hypothesis that gum bleeding would decrease proportions of epithelial cells, possibly because the severity and temporary nature of bleeding may be insufficient to cause a significant impact on immune cell numbers and possibly because of our relatively small sample size.
Comparison of epithelial cell proportions using cytology and DNA methylation
Using the EpiDISH algorithm [Citation2] on DNA methylation data generated by Infinium MethylEPIC arrays, we estimated that epithelial cell proportion was lower in saliva compared to OC-collected samples by an average of 47%, a larger magnitude than that shown using cytology. Across all samples, the correlation between the two methods of epithelial cell estimation was very high (R = 0.84). Taken together, these findings prove our primary hypothesis and imply that post hoc deconvolution accurately estimates cellular heterogeneity in oral samples. In a study comparing proportions of various blood cell types estimated using flow cytology and a blood-specific DNA methylation-based algorithm, a wide range of correlations, between 0.51 and 0.97 were observed [Citation18]. Our line of best fit showed that DNA methylation underestimated the epithelial cell content determined using cytology by 34% at 0% epithelial cells and by 13% at 87% epithelial cells (). This is larger than the over- or under-estimations of up to 10% observed in the previous study of blood [Citation18]. This discrepancy could be for a number of reasons. The reference dataset was derived from Illumina InfiniumHM450 array data from 11 different epithelial cell lines [Citation2] which may not accurately represent buccal epithelial cells. We also cannot rule out the possibility that buccal and immune cell types may have been differentially applied to slides prior to cytological examination. Nevertheless, the high correlation between epithelial cell proportions based on cytology and DNA methylation should still be sufficient to use the latter to generate estimations across a set of biosamples for adjustment within EWAS.
Investigating an age effect on the proportion of immune cells in buccal swabs
We found a strong, non-linear, negative correlation of epithelial content in buccal swabs and saliva with age (, Supplementary Figure 2). This agrees with our previous study that showed an effect in the same direction with buccal swabs and saliva in children and adults [Citation3]. In our earlier study, we showed that epithelial cell proportion was significantly lower in children with gingivitis. As gingivitis and other oral inflammatory pathologies such as periodontitis increase in prevalence from adolescence onwards [Citation19–21] and with long-term smoking [Citation22], this may result in an increase in immune cell content of oral samples and a corresponding decrease in epithelial cell content.
Strengths, limitations and future studies
To our knowledge, this study is the first to analyse the correlation between cell proportions in oral samples estimated using cytology and DNA methylation. Another strength is our longitudinal analysis showing a decline of epithelial content of buccal swabs and saliva with age. However, our sample size (n = 20 for both buccal samples and saliva; all studies, n = 753) is relatively small, although our sample size for the study of age effects (n = 753) was much larger. Future, larger-scale studies that compare estimates of cell proportion using both cytology and DNA methylation are required to validate our findings. Such studies should include a wider age-group and measures of oral health.
Supplemental Material
Download Zip (314.6 KB)Acknowledgments
The authors thank Ziad Marroushi and Christina Dillane for technical assistance and Elizabeth Firth for her comments on the manuscript. We thank Evie Muggli, Jane Halliday, Lata Vadlamudi and Tim Silk for permission to include unpublished data.
Disclosure statement
No potential conflict of interest was reported by the author(s).
Supplementary material
Supplemental data for this article can be accessed here.
References
- Teschendorff AE, Zheng SC. Cell-type deconvolution in epigenome-wide association studies: a review and recommendations. Epigenomics. 2017;9(5):757–768.
- Zheng SC, Breeze CE, Beck S, et al. EpiDISH web server: epigenetic dissection of intra-sample-heterogeneity with online GUI. Bioinformatics. 2019. DOI:10.1093/bioinformatics/btz833.
- Theda C, Hwang SH, Czajko A, et al. Quantitation of the cellular content of saliva and buccal swab samples. Sci Rep. 2018;8(1):6944.
- Teschendorff AE, Relton CL. Statistical and integrative system-level analysis of DNA methylation data. Nat Rev Genet. 2018;19(3):129–147.
- Zheng SC, Webster AP, Dong D, et al. A novel cell-type deconvolution algorithm reveals substantial contamination by immune cells in saliva, buccal and cervix. Epigenomics. 2018;10(7):925–940.
- Yang GC, Papellas J, Wu HC, et al. Application of ultrafast papanicolaou stain to body fluid cytology. Acta Cytol. 2001;45(2):180–185.
- Aryee MJ, Jaffe AE, Corrada-Bravo H, et al. Minfi: a flexible and comprehensive bioconductor package for the analysis of infinium DNA methylation microarrays. Bioinformatics. 2014;30(10):1363–1369.
- Chen Y-A, Lemire M, Choufani S, et al. Discovery of cross-reactive probes and polymorphic CpGs in the illumina infinium humanMethylation450 microarray. Epigenetics. 2013;8(2):203–209.
- Maksimovic J, Gordon L, Oshlack A. SWAN: subset-quantile within array normalization for illumina infinium HumanMethylation450 BeadChips. Genome Biol. 2012;13(6):R44.
- Touleimat N, Tost J. Complete pipeline for Infinium® Human Methylation 450K BeadChip data processing using subset quantile normalization for accurate DNA methylation estimation. Epigenomics. 2012;4(3):325–341.
- Teschendorff AE, Breeze CE, Zheng SC, et al. A comparison of reference-based algorithms for correcting cell-type heterogeneity in epigenome-wide association studies. BMC Bioinformatics. 2017;18(1):105.
- Martino D, Loke YJ, Gordon L, et al. Longitudinal, genome-scale analysis of DNA methylation in twins from birth to 18 months of age reveals rapid epigenetic change in early life and pair-specific effects of discordance. Genome Biol. 2013;14(5):R42.
- Mohandas N, Loke YJ, Hopkins S, et al. Evidence for type-specific DNA methylation patterns in epilepsy: a discordant monozygotic twin approach. Epigenomics. 2019;11(8):951–968.
- Langie SA, Moisse M, Declerck K, et al. Salivary DNA methylation profiling: aspects to consider for biomarker identification. Basic and Clinical Pharmacology and Toxicology. 2016;121:93–101.
- Farah R, Haraty H, Salame Z, et al. Salivary biomarkers for the diagnosis and monitoring of neurological diseases. Biomed J. 2018;41(2):63–87.
- Hassaneen M, Maron JL. Salivary diagnostics in pediatrics: applicability, translatability, and limitations. Front Public Health. 2017;5:83.
- Shah S. Salivaomics: the current scenario. J Oral Maxillofac Pathol. 2018;22(3):375–381.
- Gervin K, Page CM, Aass HC, et al. Cell type specific DNA methylation in cord blood: a 450K-reference data set and cell count-based validation of estimated cell type composition. Epigenetics. 2016;11(9):690–698.
- Huang S, He T, Yue F, et al. Longitudinal multi-omics and microbiome meta-analysis identify an asymptomatic gingival state that links gingivitis, periodontitis, and aging. mBio 2021;12(2). DOI:10.1128/mBio.03281-20.
- Lopez R, Smith PC, Gostemeyer G, et al. Ageing, dental caries and periodontal diseases. J Clin Periodontol. 2017;44(Suppl 18):S145–S52.
- Zainal Abidin Z, Zainuren ZA, Noor E, et al. Periodontal health status of children and adolescents with diabetes mellitus: a systematic review and meta-analysis. Aust Dent J. 2021. DOI:10.1111/adj.12845.
- Rad M, Kakoie S, Niliye Brojeni F, et al. Effect of long-term smoking on whole-mouth salivary flow rate and oral health. J Dent Res Dent Clin Dent Prospects. 2010;4(4):110–114.