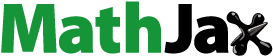
ABSTRACT
Cystic fibrosis (CF) is a monogenic disease, characterized by massive chronic lung inflammation. The observed variability in clinical phenotypes in monozygotic CF twins is likely associated with the extent of inflammation. This study sought to investigate inflammation-related aberrant DNA methylation in CF twins and to determine to what extent acquired methylation changes may be associated with lung cancer.
Blood-based genome-wide DNA methylation analysis was performed to compare the DNA methylomes of monozygotic twins, from the European CF Twin and Sibling Study with various degrees of disease severity. Putatively inflammation-related and differentially methylated positions were selected from a large lung cancer case-control study and investigated in blood by targeted bisulphite next-generation-sequencing. An inflammation-related locus located in the Plakophilin-3 (PKP3) gene was functionally analysed regarding promoter and enhancer activity in presence and absence of methylation using luciferase reporter assays.
We confirmed in a unique cohort that monozygotic twins, even if clinically discordant, have only minor differences in global DNA methylation patterns and blood cell composition. Further, we determined the most differentially methylated positions, a high proportion of which are blood cell-type-specific, whereas others may be acquired and thus have potential relevance in the context of inflammation as lung cancer risk factors. We identified a sequence in the gene body of PKP3 which is hypermethylated in blood from CF twins with severe phenotype and highly variably methylated in lung cancer patients and controls, independent of known clinical parameters, and showed that this region exhibits methylation-dependent promoter activity in lung epithelial cells.
Introduction
Cystic fibrosis (CF) is an autosomal recessive monogenic disease caused by mutations in the cystic fibrosis transmembrane conductance regulator (CFTR) gene which is located on chromosome 7q31.2 and was discovered in 1989 [Citation1]. It affects approximately over 1:2000 new-borns in the Caucasian population [Citation2].
More than 2000 different genetic variants in CFTR, including missense, frameshift, splicing and nonsense mutations as well as in-frame deletions and insertions, are known to be causative for various CF phenotypes [Citation3]. CFTR protein deficiency causes excessive inflammatory processes in the affected organs, mainly the lung, pancreas and intestines [Citation4,Citation5]. Major contributors to the severity of this condition are respiratory malfunctions and malnutrition. These symptoms are reflected by the clinical parameters forced expiratory volume in 1 second percent (FEV1%) and weight expected for height percent (wfh%), both referring to the non-CF population and representative for pulmonary and nutritional status, respectively, which together are considered to define the disease severity and subsequently intra-pair discordance [Citation6]. Both parameters are associated with the chronic inflammation of the affected organs [Citation7,Citation8].
Considering that CF is a monogenic disease, the severity of the lung condition is remarkably variable in different individuals. The most evident cause for this phenomenon is the high number of distinct CFTR mutation types, leading to different CFTR protein defects and impaired functions [Citation3]. Additionally, a number of genetic modifiers affecting the CF phenotype have been identified in various genetic association studies and have been shown to account for more than 50% of variation in the lung disease phenotype [Citation9–12]. Interestingly, clinical discordance has been observed even in monozygotic twins with CF. This implies a role of environment and epigenetics in determining disease severity in addition to the genetic factors [Citation13,Citation14]. Phenotypical discordance in monozygotic twins is plausibly associated with epigenetic effects, as the respective twins are genetically identical and typically share early-life environmental exposure. Due to the reduced confounders and cohort effects in twin studies, even small sample groups are appropriate for identification of phenotype-associated epigenetic markers and mechanisms [Citation15,Citation16].
Several twin studies have been successfully performed to investigate epigenetic patterns, such as DNA methylation, in the context of lung function, inflammatory diseases and cancer [Citation13,Citation17–21]. Differentially methylated loci associated with diseases such as cancer are considered promising as biomarkers for risk, development and progression of diseases [Citation20,Citation22,Citation23, Citation24]. DNA methylation patterns, although cell-type and tissue specific can be reflected in clinically accessible surrogate tissues, such as whole blood [Citation25–29]. In concert with other epigenetic mechanisms, DNA methylation decreases the accessibility of genomic regions and therefore has physiological functional relevance for genomic stability, chromatin structure, imprinting, transcription factor binding, splicing, silencing of retro-transposal, repetitive elements and gene expression (e.g., oncogenes) as well as for activation of gene expression (e.g., tumour suppressor genes) and transcription of antisense transcripts [Citation30–33].
Consequently, aberrant DNA methylation or demethylation is also functionally associated with a variety of diseases, among them inflammatory diseases and cancer [Citation31,Citation34–37].
It has been shown that chronic inflammation in the lung and other tissues alters DNA methylation patterns via distinct mechanisms involving inflammatory mediators and infectious agents [Citation38–41]. Chronic inflammation and infections are strongly connected with cancer risk, initiation and progression and have been described as causative and consequential events in cancer development [Citation42,Citation43].
On the one hand, infectious agents generate metabolites, on the other hand, the host´s immune system produces cytokines, chemokines, growth factors and free radicals. If unresolved, chronic inflammation causes a constant disordered environment, finally leading to genetic and epigenetic aberrations promoting neoplasia [Citation44,Citation45]. Hence, infection and inflammation can both increase cancer risk via multiple mechanisms of which many have yet to be clarified. A better understanding of these mechanisms is essential in order to improve cancer intervention strategies.
Altered DNA methylation is one mechanism promoting cancer development in an inflammatory environment, as has been shown for infections with the Human Papilloma virus, Helicobacter pylori, Epstein–Barr Virus and Hepatitis B and C viruses [Citation46, Citation47–52]. Chronic inflammatory lung diseases such as chronic obstructive pulmonary disease (COPD) are associated with an increased risk for lung cancer [Citation53, Citation54, Citation55]. Furthermore, chronic inflammation of the lung has been shown to be implicated in lung cancer development also via epigenetic mechanisms [Citation56,Citation57].
Although CF has not been demonstrated to be associated with lung cancer risk, we consider CF an adequate model for investigating the impact of massive chronic inflammation of the lung and its implications in other inflammation-related lung diseases such as lung cancer [Citation58]. It has been shown that the well-known modifier of CF lung disease, Mucin 4 (MUC4) and Mucin 5AC (MUC5AC) are associated with lung cancer risk and prognosis, respectively [Citation11,Citation59,Citation60]. Further CF lung modifier genes, such as ETS homologous factor (EHF), (Epithelial splicing regulatory protein 2) ESRP2 and macrophage migration inhibitory factor (MIF) have functional roles in epithelial to mesenchymal transition, a process that is also epigenetically regulated and plays a major role in lung cancer development [Citation61–65].
Glutathione S-transferase M 1 (GSTM1), involved in detoxification pathways, is related to lung cancer risk in an environment-dependent manner and modifies lung disease severity in CF as well [Citation11,Citation66,Citation67]. Transforming growth factor beta 1 (TGFβ1), associated with inflammation and lung cancer risk and the smoking-related differentially methylated gene Aryl-hydrocarbon receptor repressor (AHRR) are likewise modifiers of CF lung disease [Citation11,Citation65,Citation68,Citation69].
In the present study we aim to identify novel mechanisms that may play a role in inflammation-related lung carcinogenesis. We investigated aberrant DNA methylation patterns putatively acquired by chronic inflammation of the lung, their appearance in the lung cancer context and their functional implications in lung diseases, particularly lung cancer. For this purpose, we examined blood-based genome-wide DNA methylation patterns in monozygotic CF twin pairs with distinct phenotypical discordance from the European CF Twin and Sibling Study [Citation6]. In order to study inflammation-related epigenetic alterations, genome-wide DNA methylation data were obtained for 22 monozygotic twin pairs with CF. The methylomes were characterized with respect to the clinical parameters. Discordance-dependent differentially methylated loci were identified by an unbiased selection process. Differentially methylated loci, potentially interesting in the context of chronic lung inflammation and lung cancer, were tested in whole blood samples of a large lung cancer case-control cohort to discover epigenetic alterations with possible functional implications in inflammation-related lung cancer. For the most interesting locus, functional analysis has been conducted in lung epithelial cells.
Material and methods
Subjects and samples
Illumina 450 K methylation analysis was conducted for 22 monozygotic twin pairs with Cystic Fibrosis from the European CF Twin and Sibling Study. Spirometry-based lung function measurements were available and the clinical severity (DfO: distance from origin) and the phenotypical intra-pair discordance (Delta) had been calculated based on lung function, reflected by FEV1% (forced expiratory volume in 1 second percent) and wfh% (weight expected for height percent), representative for nutritional status as described previously [Citation6]. With respect to their intra-pair discordance, the twin pairs were assigned to the categories concordant (n = 6), intermediate (n = 6), discordant (n = 6) and NA (unknown discordance) (n = 4) ().
Table 1. Clinical characteristics of the CF twins.
For targeted bisulphite sequencing-based methylation analysis 109 lung cancer patients and 109 healthy controls from the HD lung cancer case–control cohort [Citation70,Citation71] were pair-wise matched by sex, age (± 0 years), packyears (±0 years) and smoking status (current, ex and never smokers), in a nested case-control study design ().
Table 2. Clinical characteristics of lung cancer cases and healthy controls individually matched by age, gender, smoking status and packyears.
Epigenome-wide DNA methylation analysis
From all subjects, frozen peripheral blood samples were used to extract genomic DNA (gDNA). The gDNA was bisulphite-converted with the EZ DNA Methylation™ Kit (Zymo Research). DNA methylation was quantified with the Illumina 450 K BeadChip Array (Illumina). Bioinformatical analysis and quality control of 450 K methylation data were performed with the R package RnBeads [Citation72].
iDMP selection process
For selection of disease-related differentially methylated positions, probes with missing values were removed. Further, positions located in repetitive sequence according to Price et al. (n_bp_repetitive 1) were ignored [Citation73]. Intra-pair differential methylation was calculated as DM = βsevere – βmild, where β is the methylation value corresponding to % methylation of the more or less severely affected twin.
For discordance-dependent differentially methylated probes the following assumptions were made: (1) they are strongly differentially methylated, arbitrarily defined as (10%) only in discordant twin pairs and twin pairs with unknown discordance, (2) they are not differentially methylated, defined as
(5%) in concordant twin pairs. Methylation differences below 5% at single CpG nucleotides may not be reliably detectable with different methods, such as pyrosequencing [Citation74].
Probes were considered one-directionally differentially methylated if (1) the correlation between methylation values and individual severity (DfO), (2) the correlation between methylation differences and intra-pair discordance (Delta) and (3) the mean methylation differences () consistently indicate a hyper- or hypo-methylation (i.e., positive DfO value, negative Delta value and negative
value or vice versa).
Correlations were calculated according to:
where is the severity or discordance matrix,
the methylation value or methylation difference matrix and
is one severity or discordance value corresponding to one methylation value or methylation difference, respectively.
Identification of positions significantly differentially methylated between different blood-cell-types, was done with an F-test (described in statistical analyses), using a publicly available dataset. Briefly, Reinius et al. sorted and performed cell-type specific genome-wide DNA methylation analysis with different blood cell-types obtained from six healthy individuals [Citation75]. Calculations in our study are based on granulocytes, monocytes, CD4+ and CD8+ T cells, B cells and natural killer cells.
TCGA data analysis
The cancer genome atlas (TCGA) research network DNA methylation data (https://www.cancer.gov/tcga) obtained with the Illumina 450K BeadChip, were downloaded from the TCGA Wanderer for lung adenocarcinoma (LUAD) and lung squamous cell carcinoma (LUSC) and corresponding normal adjacent tissues [Citation76]. Probes with a methylation difference of ≥5% between LUAD (32 normal samples, 463 tumour samples) and LUSC (43 normal samples, 361 tumour samples) and normal lung tissue, in the same direction as in the CF twin cohort, were considered to be differentially methylated.
ENCODE data analysis
Chromatin immunoprecipitation DNA-sequencing (ChIP-seq) data from nine different cell lines (NHLF, NHEK, K562, HUVEC, HSMM, HMEC, HepG2, H1-hESC and GM12878) from the Broad institute were considered using the UCSC genome browser [Citation77–80].
Assay design for targeted bisulphite next-generation sequencing (NGS)
Twenty-one targeted assays, corresponding to 20 genomic regions were designed using the PyroMark Assay Design Software 2.0. Primer sequences are provided in Supplementary . The assays were designed based on the UCSC Genome Browser Human Feb. 2009 (GRCh37/hg19) Assembly (http://genome.ucsc.edu/cgi-bin/hgGateway?db=hg19).
Targeted bisulphite next-generation sequencing
Whole blood was obtained from the study subjects and gDNA was isolated. Further processing involved multiple kits which were always used according to the manufacturer’s standard instructions, with exceptions detailed below. Concentrations were determined with the Qubit 2.0. (Thermo Fisher Scientific) using the Qubit dsDNA Broad Range Assay (Thermo Fisher Scientific). Five hundred ng gDNA from each donor was bisulphite-converted with the EZ DNA Methylation™ Kit (Zymo Research) using the alternative incubation conditions for the Illumina Infinium® Methylation assay and eluted in 2 × 30 µl instead of 1 × 10 µl. For the amplification of the target regions, the HotStarTaq DNA polymerase (Qiagen) was used. Ten multiplex PCR reactions were performed for 21 regions as shown in Supplementary . The amplification products were cleaned up with AMPureXP Beads (Agencourt) to remove primer dimers in a sample:beads ratio of 1:0.8 prior to determination of concentration with the Qubit dsDNA High Sensitivity Kit (Thermo Fisher Scientific). Amplicons were pooled so that each amplicon was represented in an equimolar amount within a given sample. Eight cycles of index PCRs were performed with the NexteraXT Index Kit v2 Set A and B (Illumina) at 55°C annealing temperature and 30 sec elongation time using the KAPA HiFi HotStart Mix (Roche). The resulting indexed amplicons were purified with AMPureXP Beads (Agencourt) to remove primer dimers in a sample:beads ratio of 1:0.8 prior to determination of concentration with the Qubit dsDNA Broad Range Kit (Thermo Fisher Scientific). All samples were equimolarly pooled and again cleaned up with AMPureXP Beads (Agencourt) to remove primer dimers in a sample:beads ratio of 1:0.8 prior to determination of concentration with the Qubit dsDNA Broad Range Kit (Thermo Fisher Scientific). The quality and size of the library was verified using the Fragment Analyser Standard Sensitivity NGS Fragment 1–6000bp Kit (Agilent) on the Fragment Analyser (Agilent). The final library was denatured and diluted according to the MiSeq System Denature and Dilute Libraries Guide (Illumina) and library concentration was determined with the Qubit dsDNA High Sensitivity Kit (Thermo Fisher Scientific). The final libraries were spiked with 25% PhiX Control v3 (Illumina), diluted to 8pM and 300bp paired-end sequenced on a MiSeqDx instrument (Illumina) with the MiSeq Reagent Kit V3 600 cycles (Illumina). Samples from cases and controls were sequenced on the same flow cell.
Bioinformatical analyses of targeted bisulphite NGS data
Demultiplexed FASTQ files from two runs on the MiSeq (Illumina) were unzipped and merged sample-wise. Prior to alignment, reads were quality trimmed with Trim Galore! (Babraham Bioinformatics), (https://github.com/FelixKrueger/TrimGalore) using Cutadapt version 1.12 for paired-end reads. The quality encoding type was set ASCII+33 (phred 33). Low-quality ends were removed with a threshold of phred score 20. The Nextera Transposase was assumed as adaptor sequence. The maximum trimming error rate was set to 0.1, the minimum required adapter overlap (stringency) was specified as 2 bp and the minimum required sequence length for both reads, before a sequence pair gets removed, was set to 20 bp. Trimmed reads were aligned with the methylation analysis pipeline ‘bicycle’ in the paired-end mode with a maximum valid read length of 1000 and phred33 as quality settings for bowtie2 [Citation81]. The UCSC Genome Browser on Human Feb. 2009 (GRCh37/hg19) Assembly was used (http://hgdownload.soe.ucsc.edu/goldenPath/hg19/bigZips/hg19.2bit) as reference genome. The obtained file was converted to a FASTA file, which can be used by bicycle with twoBitToFa (http://hgdownload.soe.ucsc.edu/admin/exe/linux.x86_64/twoBitToFa). Methylation analysis with ‘bicycle’ was performed with a fixed error rate computation mode (Watson error rate = 0.01, Crick error rate = 0.01). Non-correctly bisulphite-converted reads, reads aligned to both Watson (+) and Crick (-) strands and reads with more than one possible alignment were ignored. Mean coverage represents the mean read depth across all cytosines in a region.
Statistical analyses
Calculation of 95% confidence interval was performed with the CI function from the ‘Rmisc’ R-package. All statistical analyses were performed with R and RStudio software if not stated differently.
The cell-type contribution was estimated with the R package RnBeads applying the algorithm by Houseman et al. [Citation72,Citation82]. As a reference, data from Reinius et al. were used [Citation75].
An F-test was done with GEO2R (https://www.ncbi.nlm.nih.gov/geo/geo2r/) using the dataset of Reinius et al. to identify cell-type specific differentially methylated positions. For this purpose, the methylation values of granulocytes, CD4+ T cells, CD 8+ T cells, CD14+ monocytes, CD19+ B cells and CD56+ natural killer cells were taken into account [Citation75]. P-values were false discovery rate (FDR) corrected (Bonferroni) and values below 0.05 were considered statistically significant.
The non-parametric Wilcoxon rank sum test was performed one-sided and unpaired with a confidence interval of 0.95. The exact p-value was computed and then rounded to the second digit. The function wilcox.test was used.
Enrichment analysis was executed with one-sided Fisher´s exact test using the fisher.test function. Gene association, CGI relation and regulatory region were characterized according to the Illumina HumanMethylation450 v1.2 Manifest file. Gene association corresponds to functional regions with UCSC genes that a probe is associated with. CGI relation is equivalent to the location of the CpG relative to the CpG island. Enhancers were defined as predicted enhancer elements, DHS as experimentally determined DNase I Hypersensitivity Sites which correspond to open chromatin (ENCODE project). Promoters and cell-type specific promoters are promoter-associated and cell-type-dependent promoter-associated probes, respectively, provided by the Methylation Consortium. Telomeric and subtelomeric regions were defined as the distal 500 kb of each chromosome arm [Citation83]. Imprinted regions were specified according to the Illumina HumanMethylation450 BeadChip (v1.2, extended annotation) file. Probes that overlap with the stretch of 1500 bp around the transcription start site of any of the transcripts of known imprinted genes were defined as imprinted.
The power-analysis was done with the power.t.test function using standard deviations of the candidate positions in the whole blood of 796 healthy individuals from case-control pairs from the NOWAC, MCCS, NSHDS and EPIC HD studies [Citation22] (Supplementary Table 3). The power to detect methylation differences ≥5% with a two-sided paired Student´s t-test with a number of 109 observations per group and a Type I error probability of 5% was calculated (Supplementary Table 4).
The paired Student´s t-test was performed two-sided with a confidence interval of 0.95 with the function t.test. The two variances have been treated as being equal and NA values were omitted. P-values were corrected for false discovery rate with the R package q-value.
The principal component analysis was executed with the methylation values obtained from 218 samples using the default settings of the prcomp function from the stats package.
The Pearson correlation between numerical clinical parameters (age and packyears) and mean methylation values per region was calculated using the cor function. The NAs were removed.
The Pearson correlation between the global mean methylation difference and discordance (Delta) and age was calculated using the cor function. The NAs were removed.
The coefficient of determination, corresponding to in the mean methylation per region explained by the respective nominal parameters (sex, cancer histology, cancer occurrence, and smoking status) was calculated by performing a multiple regression using the lm function. The NAs were removed.
Gene-ontology enrichment analysis was performed for genes with inflammation-related differentially methylated positions in their gene body or promoter for the terms biological process, molecular function and cellular component with a Fisher´s Exact test, FDR was calculated.
Welch Two Sample t-test was performed with the relative luciferase activity fold-change values. Luciferase activity values were normalized for firefly activity. The fold-change was calculated and compared to the empty vector as a reference.
Luciferase reporter assay
To investigate the functional role of the putatively inflammation-related differentially methylated region (DMR) located in the Plakophilin 3 (PKP3) gene (chr11:396,135–397,671, 1537bp; hg19), it was cloned into the pCpGfree basic vector (Invivogen) for testing promoter activity and into the pCpGfree promoter vector (Invivogen) for testing enhancer activity (Supplementary Figure 8). To determine the effect of methylation on the activity of the region, in vitro methylation of the constructs was performed with M.SssI (NEB). In short, DNA isolated from the blood of a healthy donor was amplified using the Phusion HF polymerase (Thermo Scientific) with primers (Sigma, Supplementary Table 8) containing the restriction enzyme sites for NsiI and BamHI at the 5´ends of the forward and reverse primer, respectively. The PCR product clean-up was performed with the ReliaPrep DNA Clean-Up and Concentration kit (Promega). One thousand ng of the amplicon and the plasmid were double digested with 20 U of BamHI (NEB) and 20 U NsiI (NEB) at 37°C for 20 min. The digests were cleaned-up with 0.5x volumes SPRI beads. The ligation was performed with a 1:3 plasmid-to-insert ratio with T4 DNA ligase (NEB) at 16°C overnight. Chemically competent E. coli GT115 (pirmut strain, Invivogen) cells were transformed by performing a heat shock with 5 µl ligation reaction and were spread on LB-zeocin (50 µl/ml; Invivogen) plates. After successful growth in larger volumes, the presence of the insert was verified via colony PCR with Phusion HF polymerase (Thermo Scientific) and short primers targeting the insert region and Sanger sequencing (LGC genomics; Supplementary Table 8). The plasmids containing desired inserts were extracted with the PureYield Plasmid Midiprep System (Promega). In vitro methylation of 2 µg constructs was performed with 8 U M.SssI (NEB) at 37°C for 3 h. The enzyme was inactivated at 65°C for 20 min, followed by purification with the ReliaPrep DNA Clean-Up and Concentration kit (Promega).
A549 and H1299 lung epithelial cells were seeded into 24 well plates (1.5×105 cells/well) with Ham F-12 K + 10% foetal bovine serum medium for A549 and RPMI + 10% FBS for H1299 without antibiotics. The next day, 500 ng plasmid and 25 ng pGL4 SV40 control plasmid were co-transfected with Lipofectamine 3000 (Thermo Scientific) and incubated overnight. After cell lysis, the Lucia and Firefly read-out was generated with a Tecan Spark plate reader (Tecan). The Lucia luciferase activity was normalized to the firefly activity. To calculate the fold increase in gene expression, the empty vector was used as a control. Each experiment was performed in triplicates and repeated at least three times. Cells were regularly tested for mycoplasma.
Results
Independently of their clinical disease severity and discordance, monozygotic twins with CF are epigenetically highly similar
The phenotype of CF exhibits extensive inflammation, particularly in the lung [Citation9]. These disease-related processes may have an impact on DNA methylation patterns. In order to investigate CF phenotype- and discordance-related epigenetic alterations in whole blood, 22 clinically well-characterized monozygotic twin pairs with CF from the European CF Twin and Sibling Study were studied [Citation6]. In total, the CF twins comprise eleven different homo- and heterozygous CFTR genotypes of which the most abundant is p.Phe508del/p.Phe508del (p.Phe508del homozygous) representing the expected distribution in Europe [Citation84]. From the 44 individuals, peripheral blood DNA was obtained for epigenome-wide DNA methylation analysis of more than 400,000 selected loci in the human genome ().
The overall methylation patterns in all twins, irrespective of clinical discordance, demonstrate a bimodal distribution, representing the normally observed patterns of DNA methylation values in healthy tissues, with the majority of CpG dinucleotides being either very highly (>85%) or very slightly (<10%) methylated [Citation85]. These results suggest, that even strong inflammatory processes do not shift global DNA methylation patterns in whole blood.
To determine the global effect of clinical severity and discordance on the DNA methylome, the total and pair-wise methylation differences across all loci covered by the array were assessed. Cumulated, all twin pairs revealed a very low mean global intra-pair methylation difference of −0.1% (standard deviation 2.7%) with an upper and lower 95% confidence interval of 0.029% and −0.256%, respectively. Thus, a significant difference cannot be assumed. The pair-wise mean global methylation differences were between −0.6% and 0.4%, the absolute differences were between 1.1% and 2.0% and their extent correlates weakly positively and significantly with clinical intra-pair discordance (Delta) (r2 = 0.378, p-value = 0.023) and age (r2 = 0.391, p-value = 0.009). This confirms other studies demonstrating, that monozygotic twins are epigenetically highly similar and reveals that this high similarity remains even in individuals clinically discordant for a severe inflammatory disease such as CF [Citation21,Citation86,Citation87].
Differences in blood cell composition in CF twins are minor and occur mainly in discordant pairs
Blood cell-type composition is known to change upon inflammation [Citation88]. Hence, the blood cell contribution of six different blood cells (granulocytes, CD4+ T cells, CD8+ T cells, B cells, monocytes and natural killer cells) has been estimated for all samples based on the obtained DNA methylation values using six reference methylomes and an algorithm developed by Houseman et al. [Citation75,Citation82]. Irrespective of the individual phenotypical severity and the intra-pair clinical discordance, blood cell compositions within one twin pair are noticeably similar. The minor intra-pair cell-type contribution differences occur mainly in discordant twin pairs and apparently display a shift from natural killer cells to granulocytes in the more severely affected individuals (a, Supplementarya, b). This is in concordance with the fact, that a high abundance of neutrophils is frequently observed in individuals with cystic fibrosis and points out that despite clinical discordance, the blood cell-type composition differences in monozygotic CF twins are surprisingly small [Citation88].
Figure 1. Blood cell-type contribution in CF twins based on whole blood DNA methylation. (a) Estimated cell-type distribution (%) of six different cell-types in peripheral blood according to DNA methylation values in the 44 subjects representing 22 twin pairs. Heatmap of the contributions of six different cell-types in peripheral blood. Twin pairs are separated by black vertical lines. The twin with the milder phenotype is displayed on the left, whereas the one with the more severe phenotype is shown on the right. (b) Scatterplot of intra-pair cell-type contribution differences for six different cell-types. Clinical intra-pair discordance is indicated in colour.
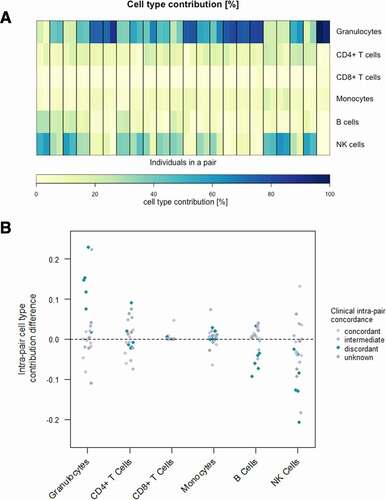
Monozygotic CF twin pairs exhibit only a few differentially methylated positions, correlating with disease severity and intra-pair discordance
In order to investigate disease-related methylation alterations, a selection process was developed and applied to identify CpG sites which are differentially methylated in an intra-pair comparison, taking into account individual severity and intra-pair discordance. 75.2% of all investigated positions covered passed a strict quality control with removal of unreliable probes, probes with missing values and probes located in repetitive sequences. Only 0.86% of all positions investigated, present discordance-dependent differential methylation according to the defined requirements. Of these, 25.6% are consistently differentially methylated in one direction in a pair-wise comparison (e.g., either consistently hyper- or hypo-methylated in all of the more severely affected individuals of a twin pair) resulting in 802 disease-related differentially methylated positions (DMPs). Due to the strongly inflammatory component of CF, these positions are referred to as putative inflammation-related DMPs (iDMPs) (, Supplementary table 5).
Figure 2. Selection process applied for identification of acquired inflammation-related differentially methylated positions (iDMPs). 75.2% of all probes measured passed a strict quality control (QC) including removal of cross-reactive and unreliable probes, probes with missing values and probes located in repetitive sequences. 0.2% of all retained probes are considered disease-related differentially methylated according to the assumptions described in the methods. Given that 87% of iDMPs are significantly differentially methylated in different blood cell-types, 106 probes are presumed to be acquired iDMPs.
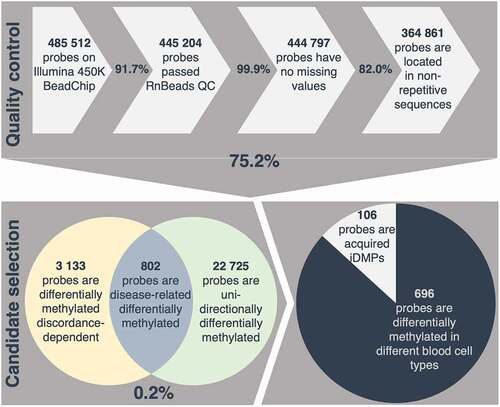
A high proportion of disease-related differentially methylated positions may reflect blood cell-type composition effects
Since DNA methylation is known to be tissue- and cell-type specific, the list of candidate iDMPs was examined for known blood cell-type specific differentially methylated positions from a publicly available dataset [Citation75,Citation89]. Interestingly, 86.8% of iDMPs have been shown to be significantly differentially methylated between different blood cell-types and therefore could reflect differential blood cell counts between the twin pairs. This would be in concordance with CF being a disease characterized by massive chronic inflammation, especially in the lung with consequent alterations in the immune cell composition and underscores the applied selection process for inflammation-related differentially methylated positions [Citation90].
The remaining 106 iDMPs are considered acquired in relation with the CF disease severity, which may be environmentally promoted and accordingly of particular interest for diseases [Citation91] (, Supplementary table 6).
Disease-related methylation differences in monozygotic twins are of a small magnitude
To validate the applied selection process, and thus the relation of the 106 selected candidate positions with the clinical discordance of the twin pairs, the extent of differential methylation was assessed. A trend from lower methylation differences to higher ones with increasing discordance can be observed for the selected iDMPs. Interestingly, twin pairs with unknown discordance exhibit the same mean of absolute sums of intra-pair methylation differences as twin pairs with intermediate discordance. Furthermore, concordant twin pairs display significantly (p = 0.004, one-sided Wilcoxon test) lower sums of intra-pair methylation differences than discordant twin pairs (). This confirms that the putative iDMPs are differentially methylated in a discordance-dependent manner as expected due to this type of selection process. However, when investigating the DNA methylation values in the twins, there are small differences between the individuals and the pairs clearly cluster together (Euclidean distance and complete hierarchical clustering), emphasizing the epigenetic similarity of twins even in the event of clinical discordance ().
Figure 3. Methylation and intra-pair methylation differences in CF twins. (a) Absolute intra-pair methylation differences of putative acquired iDMPs. Clinical discordance is indicated in colour. Methylation differences are significantly higher (p = 0.004, one-sided Wilcoxon test) in discordant than in concordant twin pairs. The means and the medians of the absolute sums of the differences increase with the extent of clinical discordance. (b) Heatmap of methylation [%] in candidate iDMPs in 44 individuals. Rows and columns are clustered by complete hierarchical clustering and Euclidean distance.
![Figure 3. Methylation and intra-pair methylation differences in CF twins. (a) Absolute intra-pair methylation differences of putative acquired iDMPs. Clinical discordance is indicated in colour. Methylation differences are significantly higher (p = 0.004, one-sided Wilcoxon test) in discordant than in concordant twin pairs. The means and the medians of the absolute sums of the differences increase with the extent of clinical discordance. (b) Heatmap of methylation [%] in candidate iDMPs in 44 individuals. Rows and columns are clustered by complete hierarchical clustering and Euclidean distance.](/cms/asset/11b1fc26-8fa0-4d44-bb03-b5c5999c07f3/kepi_a_1959976_f0003_oc.jpg)
Acquired inflammation-related differentially methylated positions are not enriched in GO-terms and do not cluster at genomic regions
In order to determine whether alterations in DNA methylation which are related to the disease phenotype of CF may accumulate in certain genomic or functional regions, enrichment analysis of the candidate iDMPs was performed. Acquired iDMPs occur to be relatively evenly distributed across the chromosomes. In comparison to all CG dinucleotides measured, they are not enriched in imprinted or (sub)telomeric regions. They do not accumulate in CG-rich or regulatory regions such as enhancers, DNA hypersensitivity sites or promoters. Also, no accumulation at gene bodies, transcription start sites or untranslated regions was observed. Of 106 iDMPs, 35 are located in a gene promoter and 47 in a gene body (including pseudogenes and antisense transcripts) and 21, 19 and 10 are located in a CpG island, CpG shore and CpG self, respectively (Supplementary). These 82 gene-associated iDMPs correspond to 81 inflammation-related differentially methylated regions (iDMRs) and 79 different genes.
Gene ontology (GO) enrichment analysis was performed with gene-associated iDMPs, however it did not deliver significant (Fisher´s Exact test, FDR) enrichment for the biological processes, the molecular function or the cellular component of the respective genes. In summary, no positional or functional hotspots or patterns were observed for acquired iDMPs; however, due to the stringent selection process, only few observations could be included into the analyses, which makes it difficult to notice genome-wide patterns.
An acquired iDMR in a CpG island in the PKP3 gene body is highly variably methylated in blood from lung cancer patients compared to healthy controls
It has been shown that environmental factors, such as cigarette smoking and inflammation can change DNA methylation patterns. These alterations can have an impact on the risk, the development and the prognosis of diseases such as cancer, which is known to be strongly associated with environmental factors (i.e., smoking) [Citation22, Citation23, Citation92, Citation93, L. Citation24].
Since chronic inflammation is a major risk factor for cancer in general, putative inflammation-related variations in DNA methylation may be relevant for lung cancer. To identify positions which are of particular interest in the context of inflammation and lung cancer, the list of putative acquired iDMPs was inspected a) for the loci most prominently differentially methylated in the CF twin cohort and b) for loci for which methylation differences were also observed in TCGA in lung cancer tissues compared to normal adjacent tissues. Of 106 iDMPs, 11 were strongly (more than once ≥10%) or frequently (more than four times ≥5%) differentially methylated in the CF twin data and 21 were differentially methylated (≥5%) in LUAD and LUSC compared to the corresponding normal tissue in TCGA data, with an overlap of three iDMPs in these two groups. Two of the candidates are located in the same region (13) directly adjacent to each other in the gene body of Plakophilin 3 (PKP3) (Supplementary).
DNA methylation of these selected acquired iDMPs and flanking regions was assessed in 218 whole blood samples from a lung cancer case-control study (). Of these 29 most interesting acquired iDMPs with regard to lung cancer, 21, corresponding to 20 genomic regions could be tested with bisulphite targeted-NGS in whole blood from lung cancer patients and healthy controls. The power to detect differences in mean methylation levels of at least 5% in the lung cancer case-control cohort of 109 pairs for all positions tested is above 99% (Supplementary table 4).
To cover all samples, two flow cells (MiSeq, Illumina) were loaded. A mean cluster density of 776 K/mm2 could be achieved of which 94.66% (36.19 million reads of 38.27 million reads) passed the filter. On average, 80.62% of the bases hold a quality score above Q30. In order to obtain only high-quality sequences, on average 98.01% and 88.29% of all reads were trimmed from the forward and reverse reads, respectively. No reads were removed. Forward reads had a better quality than reverse reads which is generally observed in paired-end sequencing with Illumina (Supplementary). Of all reads, 62% could be assigned to the samples. We assume that approximately 25% of the library is PhiX spike-in. Of all reads obtained for the 218 samples, 94.65% passed the filter and could be processed with the methylation analysis pipeline ‘bicycle.’ 84.55% of all reads which passed the filter could be uniquely aligned to the human genome. 0.86% were mapped to both Watson (+) and Crick (-) strands, 2.74% were aligned to multiple regions in the genome and 5.57% of the reads were removed due to possibly failed bisulphite-conversion (Supplementary). 98.55% of the uniquely aligned high-quality reads could be aligned on target (i.e., at the targeted regions) (Supplementary). For all samples, a mean coverage of 1694x could be achieved across all regions of interest. No region had a mean coverage below 100x with an average of 1634x (range: 124x – 4841x) (Supplementary).
Figure 4. DNA methylation differences in lung cancer cases compared to healthy controls. (a) Heatmap of methylation differences in CG dinucleotides of 20 genomic regions (numbers), corresponding to 21 iDMPs (rows separated by black lines) in 209 pairs (columns). NAs are shown in black, clinical parameters are colour coded (b) Bar plot of the means of all absolute intra-pair methylation differences [%] per CG dinucleotide of the 20 numbered regions. Median values across the regions are indicated. The width of the boxes represents the number of observations (i.e., the number of CG dinucleotides per region).
![Figure 4. DNA methylation differences in lung cancer cases compared to healthy controls. (a) Heatmap of methylation differences in CG dinucleotides of 20 genomic regions (numbers), corresponding to 21 iDMPs (rows separated by black lines) in 209 pairs (columns). NAs are shown in black, clinical parameters are colour coded (b) Bar plot of the means of all absolute intra-pair methylation differences [%] per CG dinucleotide of the 20 numbered regions. Median values across the regions are indicated. The width of the boxes represents the number of observations (i.e., the number of CG dinucleotides per region).](/cms/asset/35741e0e-da13-4234-a7e5-dbc4a8d13277/kepi_a_1959976_f0004_oc.jpg)
Methylation differences between lung cancer case-control pairs are small in most regions. If differential methylation occurs, then it is region-specific with respect to extent and direction, implying that in one pair, the whole region is consistently differentially methylated (). Applying a two-sided, paired Student´s t-test, no significant differences of the tested iDMPs in lung cancer compared to controls could be observed after FDR correction of p-values. Principal component analysis did not reveal any clustering according to known clinical parameters (Supplementary ). Further, correlation and multiple regression analysis of mean methylation values per region and known clinical parameters of the samples did not disclose any relations (correlation and coefficient of determination values below 0.18) between methylation in the investigated regions and known clinical parameters (sex, cancer occurrence, cancer histology, age, packyears and smoking status). However, of 20 regions analysed, one region (#13) comprising two adjacent candidate iDMPs (cg17840408, cg25258098) appears to be highly variably methylated across the lung cancer-case control cohort in both directions (i.e., hyper- and hypo-methylated) irrespective of known clinical parameters (, Supplementary Figure 6). The iDMR of interest is located on a CpG island in the gene body of PKP3, encoding for the desmosomal protein PKP3, known to play a role in carcinogenesis [Citation94]. It overlaps with a putative regulatory region (Supplementary Figure 7). CpG islands are often regulated via DNA methylation [Citation83,Citation95–98]. In contrast to promoter methylation, gene body methylation is more frequently, associated with active gene expression and may prevent cryptic transcription initiation [Citation99,Citation100]. Upregulation of gene expression by intragenic CpG island hypermethylation has been demonstrated to be associated with cancer [Citation101]. Also, variably methylated regions have been shown to be enriched for enhancer histone modifications and transcription factor-binding sites and may exhibit important regulatory functions especially regarding cell- and tissue-type [Citation102]. It is therefore suggested that this iDMR, hypermethylated in CF and highly variably methylated in lung cancer and healthy subjects, may have functional relevance in the context of lung inflammation and cancer.
Figure 5. Promoter and enhancer activity of the methylated and unmethylated inflammation-related DMR in lung epithelial cells. Fold change relative luciferase activity in A549 and H1299 cells. The empty vector corresponds to a construct without promoter sequence. For the putative promoter vector, the sequence of interest has been cloned into the empty vector upstream of the luciferase gene. For the putative enhancer construct, the region of interest was cloned upstream of a minimal promoter for the luciferase gene. Both vectors were compared to their in silico methylated counterparts.
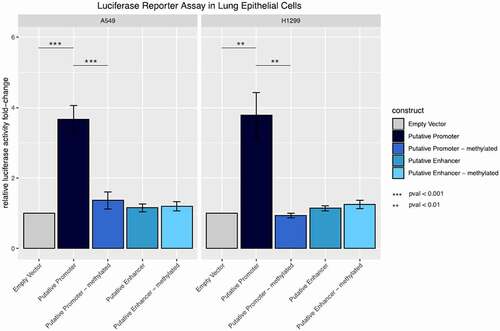
The candidate iDMR in a CpG island in the PKP3 gene body exhibits a methylation-dependent promoter activity in lung epithelial cells
Evaluation of publicly available chromatin immunoprecipitation DNA-sequencing (ChIP-seq) data from the Broad institute and ENCODE revealed that 5´-adjacent to the candidate region in the PKP3 gene body is a CTCF binding site and that the iDMR may be located in an insulator in NHLF, HUVEC and H1-hESC cells; however, it could function also as a promoter for instance in HepG2 cells [Citation77–80]. CTCF binding facilitates inter- and intra-chromosomal chromatin looping and thus enables physical proximity of regulatory regions. Further, CTCF binding sites colocalizes with promoters and enhancers and attracts or repels transcription factors and subsequently activates or represses transcription. CTCF preferentially binds to unmethylated CTCF binding sites and, in turn, binding of CTCF to insulator regions prevents its methylation [Citation103–105].
DNA methylation of cis and trans regulatory functional elements, such as CTCF binding sites, impacts their function and subsequently results in activation or inactivation of gene expression in a context- and cell-type specific manner [Citation32,Citation106]. To evaluate the functional relevance of the putative regulatory region, associated with a CTCF binding site in the tissue of interest, luciferase reporter assays were performed in the lung epithelial cells A549 and H1299. Therefore, the region of interest was cloned into a CpG-free vector upstream of the luciferase gene with and without minimal promoter to determine functional activity as enhancer and promoter, respectively (Supplementary Figure 8). In both cell lines a highly significant increase in the relative luciferase activity (Welch Two Sample t-test, p-value = 0.0008513) could be shown for constructs containing the region of interest 5´ to the luciferase gene compared to vectors without the respective region. This indicates that the putative regulatory region functions as a promoter in lung epithelial cells. However, the insertion of the same regulatory element together with a minimal promoter did not result in enhanced luciferase activity in comparison to the empty plasmid, suggesting that it does not function as an enhancer in the tested cell lines. To determine the effect of DNA methylation of the regulatory region on luciferase expression, both constructs were in vitro methylated. Since the vectors are CpG free, only the inserted sequence is methylated. While no change in luciferase activity was shown for the methylated (A549: 1.20 ± 0.129; H1299 :1.25 ± 0.117) and unmethylated (A549: 1.15 ± 0.109; H1299 :1.14 ± 0.072) enhancer constructs, methylation of the promoter construct resulted in significantly decreased luciferase activity (A549: −3.66 ± 0.400; H1299: −3.78 ± 0.0644) compared to the unmethylated region (A549: −1.36 ± 0.0239; H1299: −0.94 ± 0.064) suggesting that the promoter function of the region of interest is depleted upon its methylation ().
Taken together, these results show that the iDMR located in the PKP3 gene body can function as a promoter in lung epithelial cells and that this function is eliminated via DNA methylation.
Discussion
In order to investigate inflammation-related aberrant DNA methylation, genome-wide methylation was analysed in twins with CF, as a chronic inflammatory lung disease. CF disease severity is highly associated with the degree and the duration of inflammatory processes, particularly in the lung [Citation4].
In the lung, the neutrophilic inflammation leads to release of neutrophil elastases, matrix-metalloproteinases, nitric oxide and reactive oxygen species (ROS), which cannot be efficiently resolved and cause massive tissue injury. Therefore, the CF lung is flooded with proinflammatory mediators such as tumour necrosis factor alpha (TNF-α), interleukin (IL)-1β, IL-6, IL-8 and others. On the other hand, anti-inflammatory cytokines for instance, IL-10, are decreased, resulting in impaired resolution of inflammation and an extensive and chronic inflammatory state including defective apoptosis and phagocytosis [Citation5,Citation107]. Like CF, COPD is characterized by mucus hypersecretion, oxidative stress, neutrophilic inflammation and the involvement of macrophages. TNF‐α, IL‐1β, IL‐6 and IL‐8 are contributing to inflammation in COPD as well [Citation108]. COPD is an inflammatory disease of the lung highly associated with lung cancer [Citation55,Citation109]. COPD and other chronic inflammatory disorders of the lung are associated with an increased risk for lung cancer development [Citation53, J. Citation54, Citation55]. Also, the major risk factor for lung cancer, smoking, is known to trigger lung inflammation [Citation110–112].
CF, although a disease characterized by massive chronic lung inflammation, is not associated with lung cancer risk [Citation58]. This may be due to the fact that the early and strong impairment of the lung is fatal, or lung transplantation is indicated and performed prior to the development of lung cancer [Citation113,Citation114]. It has been even suggested that the p.Phe508del deletion has a protective role in lung cancer [Citation115]. However, due to its severe phenotype, we consider CF an appropriate model for investigating excessive and persistent chronic inflammation of the lung with potential implications for lung cancer. Since the CFTR genotype, the genomic background, sex, age and socioeconomic factors can be neglected in this study exploring monozygotic twins, we assume that clinical intra-pair discordance is at least to some extent due to a different degree of inflammation [Citation84,Citation116,Citation117].
Global alterations of DNA methylation patterns have been described in cancer and ageing [Citation118,Citation119]. Investigating the global methylome of monozygotic twins, no overall alterations in DNA methylation were observed in intra-pair comparisons. These observations were independent of clinical discordance of the respective twin pair and are in accordance with previous studies, showing that monozygotic twins are epigenetically highly similar and emphasize the importance of age, sex, genetic background and environment for the shape of the methylome [Citation86,Citation87,Citation120–126].
Global and locus-specific DNA methylation differences as well as phenotypic discordance in monozygotic twins are known to increase with age [Citation127,Citation128]. In this study, no relation between the absolute global methylation difference and the age of the twin pairs could be observed. However, the twins evaluated in this study are relatively young with a mean age of 14.4 years.
DNA methylation is known to be cell-type-specific. Therefore, DNA methylation values obtained from an epigenome-wide analysis can be applied to estimate the cell-type proportion in a sample with a mixed cell population [Citation75,Citation82]. Our data indicate high similarities in blood cell proportion in intra-pair comparisons. Cell-type composition differences were mainly observed in clinically discordant twins which is in accordance with the fact that inflammatory diseases alter the abundance of immunological cells in blood [Citation129]. Relative differential cell-type counts are mainly attributed to granulocytes and natural killer cells in an inverse manner. A larger fraction of granulocytes in blood from more severely diseased individuals, compared to their less severely affected twin counterparts, may point to neutrophilia, the high abundance of neutrophils (granulocytes, basophils and eosinophils), in the blood and subsequently the lung which in turn is a well-known symptom in CF [Citation88].
To discover whether chronic lung inflammation may cause locus-specific differential methylation in the blood, positions differentially methylated in a severity- and discordance-specific manner were selected. Thus, a subset of putative iDMPs could be identified. Our data indicate that a large proportion of these iDMPs have been shown to be differentially methylated in a blood cell-type-specific manner and hence may be attributed to cell-type composition effects [Citation75,Citation89]. Given that the abundance of immune cells in the blood is likely to be altered in an inflammatory setting, this emphasizes that the selected loci may be related to inflammation [Citation129].
All other iDMPs are considered to be acquired due to exposure to the inflammatory environment. Acquired alteration of DNA methylation induced by environmental factors is of particular interest in the context of diseases [Citation91,Citation130,Citation131]. The 106 iDMPs we observed in our study are not enriched in genomic location and in relation to particular genes or to functional regions. Seventy-seven percent of the iDMPs are considered gene-associated; however, their respective genes are not enriched in biological process, molecular function or cellular components. However, due to the stringent selection process, the number of observed iDMPs may have been too small for these analyses.
Among the 21 tested loci, none was differentially methylated in blood from lung cancer cases compared to healthy controls. However, one DMR including two iDMPs, cg17840408 and cg25258098, directly adjacent to each other and hyper-methylated in blood of the CF twins, was found to be highly variably methylated in whole blood of lung cancer cases compared to healthy controls, irrespective of known clinical parameters (sex, age, packyears, current smoking status and lung cancer histology in cases) which are known to be related to DNA methylation [Citation24,Citation121,Citation132,Citation133]. This variable methylation is extended also to the 5´- and 3´-flanking regions. The iDMR is located on chromosome 11p15.5, a subtelomeric region, in a CpG island indicating a regulatory function of DNA methylation in this region since these CpG dense regions are often prone to regulation by DNA methylation [Citation83,Citation95–98].
In non-related individuals, one major cause of interindividual methylation variability are genetic differences, whereas in monozygotic twins it is determined either by stochastic effects, which result in an epigenetic drift or by environmental exposure, such as chronic inflammation [Citation122,Citation128]. It has been shown that DNA methylation at certain CpG sites differs between distinct tissues, whereas others are consistently methylated in any tissue of an individual but display interindividual differences. The latter may be particularly valuable in the context of risk biomarkers in peripheral blood independently of functional relevance as they may offer information regarding epigenetic regulation in less accessible tissues [Citation134].
ChIP-seq data from the Broad institute and ENCODE revealed that in lung cells the putatively inflammation-related differentially methylated region may function as an insulator, whereas in other cell-types it may have promoter activity [Citation77–80]. CTCF binding sites regulate inter- and intra-chromosomal interactions between functional elements, enable three-dimensional chromatin structure, separate topological regions and regulate transcription factor accessibility. Therefore, CTCF binding can dynamically control gene transcription and alternative splicing events and thus contributes to dynamic processes such as cell differentiation and generation of immune cell diversity [Citation103,Citation105]. Our results indicate, that the iDMR functions as a promoter in lung epithelial cells, which is inactivated upon methylation.
The putatively regulatory iDMR, overlapping with a CTCF-binding site is located in the gene body of PKP3 (NM_007183). PKP3 encodes for the 81.081kD PKP3 protein which is expressed in the desmosomes and the nucleus of the simple and the most stratified epithelia and plays a role in linking cadherins and intermediate filaments in the cytoskeleton, in cell–cell communication by enhancing the desmosomal strength, in the RNA metabolism and as transcription factor associated with cancer and metastasis [Citation135–139]. Desmosomal proteins such as PKP3 have implications in epithelial integrity and tissue remodelling, both hallmarks of inflammatory lung diseases, such as CF [Citation140,Citation141]. They further appear to have tumour-promoting as well as tumour-suppressing properties in distinct cancer entities. They are essential for epithelial homoeostasis, tissue integrity and regulation of cell proliferation, differentiation, migration, invasion and apoptosis and are regulated by growth factors [Citation94,Citation142].
Altered expression of PKP3 transcripts has already been described in connection with lung cancer and other cancer types. Depending on the type of cancer, over-expression of PKP3 protein and PKP3 mRNA have been shown to have oncogenic and tumour suppressing effects [Citation94,Citation143–146]. In non-small cell lung tumours, which include lung adenocarcinomas and squamous cell carcinomas, PKP3 is described as an oncogene, which has the potential as a prognostic marker but also in the therapeutic area [Citation147]. Desmosomal loss and loss of haematopoietic PKP3 is associated with increased inflammation [Citation136,Citation148,Citation149]. Overexpression of adhesion molecules such as PKP3 could be demonstrated to be associated with differential infiltration of various immune cells in human lung cancer tissue, making the demosome status a potential biomarker in the context of immunotherapies [Citation150].
Also, in the airway epithelia of CF lungs, epithelial junctions and integrity may have major roles in permeability to ions and pathogen products and subsequently lung inflammation [Citation151].
Interestingly, aberrant methylation and expression of PKP3 has been associated with endometriosis, which is an inflammatory disease of the endometrium and has been observed in the mucosa of ulcerative colitis patients with and without cancer [Citation152–154].
Directly adjacent to PKP3 is the single immunoglobin interleukin-1 (IL-1)-Related Receptor (SIGIRR) gene, which is also known as IL-1 receptor 8 (IL-1R8). IL-1R8 is anti-inflammatory and shows its effect against inflammatory diseases, also in the lung [L. Citation155].
It is interesting to note that frequent polymorphisms in the region comprising PKP3, SIGIRR and another gene, TMEM16J, have been linked to susceptibility to tuberculosis, another inflammatory lung disease [Citation156].
At present it is not yet possible to say for certain which genes in which cell-types are regulated by the methylation-dependent promoter iDMR. However, due to the functional studies performed, it can be hypothesized that this iDMR acts as an alternative promoter for the PKP3 gene.
In summary, a unique cohort of monozygotic CF twins has been clinically and epigenetically characterized in this study. The remarkable epigenetic intra-pair similarity observed even in clinically discordant twins, indicates that besides genetic and epigenetic phenotype-contribution further factors, such as the microbiome, that is likewise related to CF disease severity and progression, have a major impact on the individual development of cystic fibrosis [Citation157,Citation158]. Selected positions could be shown to be differentially methylated in CF twins in a discordance-dependent manner. These could reflect a consequence of chronic lung inflammation for the DNA methylome in the blood or may have a functional effect on the phenotype. Additionally, we observed one inflammation-related differentially methylated region to be highly variably methylated in the whole blood of individuals with and without lung cancer. Functional assays revealed that this region functions as a methylation-dependent promoter in lung epithelial cells. This suggests that the region in the PKP3 gene body is a functional promoter in lung tissues which is hypermethylated upon inflammation and variably methylated in the context of lung cancer. Whether this regulatory region may act as an alternative promoter for PKP3 gene or has insulatory or chromatin remodelling function, thus influencing nearby genes such as SIGIRR and TMEM16J in situ, may be elucidated in further research.
A better understanding of the effect of chronic inflammation on DNA methylation and the presentation of inflammation-related epigenetic changes in lung cancer may improve existing strategies in risk assessment of inflammatory lung diseases and lung cancer. Thus, it can provide further insights into the mechanisms of carcinogenesis promoted by inflammation, such as epithelial to mesenchymal transition and epithelial tissue integrity. These insights have the potential to pave the way for advances in risk stratification, prognosis and treatment of inflammation-related diseases and cancer.
Authors’ contributions
AR, BT, CP and ES conceived and planned the study. ES conducted the CF twin data analysis and performed and analysed the lung cancer NGS data. YA carried out initial bioinformatics analysis and provided support regarding further analysis of the CF twin data. WH was responsible for the Illumina 450K Methylation Analysis of the CF twins. AS and NZ assisted with the library preparation and sequencing. AH performed and analysed the luciferase reporter assays. MW assisted with the bioinformatics analysis of the sequencing data and provided the computing machines. ES drafted the manuscript, AR and BT made critical revisions. All authors approved the final manuscript.
Ethics approval and consent to participate
Cystic fibrosis cohort
The study was approved by the ethics committee of Hannover Medical School (No. 3739, last update 8 May 2014 and No. 6790, 22 April, 2015).
Lung cancer case-control cohort
All participants gave their informed consent. The study was approved by the ethical committee of the Medical Faculty of the University of Heidelberg (Nr. 182/96, 201/98 and 199/2001).
Supplemental Material
Download Zip (4.5 MB)Acknowledgments
The authors would like to thank the DKFZ core facility for performing the Illumina 450K analyses.
Further, the authors would like to thank Christian Faltus, Katharina Elsensohn, Florian Wolff, Jutta Horeijs-Höck and Josef Thalhamer for their scientific and personal support.
Disclosure statement
No potential conflict of interest was reported by the author(s).
Supplementary material
Supplemental data for this article can be accessed here.
Additional information
Funding
References
- Riordan JR, Rommens JM, Kerem B, et al. Identification of the cystic fibrosis gene: cloning and characterization of complementary DNA. Science. 1989;245(4922):1066–1073.
- Warwick WJ. The incidence of cystic fibrosis in Caucasian populations. Helv Paediatr Acta. 1978;33(2):117–125.
- Stanke F, Tümmler B. Classification of CFTR mutation classes. Lancet Respir Med. 2016;4(8):e36.
- Castellani C, Assael BM. Cystic fibrosis: a clinical view. Cell Mol Life Sci. 2017;74(1):129–140.
- Roesch EA, Nichols DP, Chmiel JF. Inflammation in cystic fibrosis: an update. Pediatr Pulmonol. 2018;53(S3):S30–S50.
- Mekus F, Ballmann M, Bronsveld I, et al. Categories of ΔF508 homozygous cystic fibrosis twin and sibling pairs with distinct phenotypic characteristics. Twin Res. 2001;3(4):277–293.
- Brownell JN, Bashaw H, Stallings VA. Growth and Nutrition in Cystic Fibrosis. Semin Respir Crit Care Med. 2019;40(6):775–791.
- Cantin AM, Hartl D, Konstan MW, et al. Inflammation in cystic fibrosis lung disease: pathogenesis and therapy. J Cyst Fibros. 2015;14(4):419–430.
- Cutting GR. Cystic fibrosis genetics: from molecular understanding to clinical application. Nat Rev Genet. 2015;16(1):45–56.
- Gong J, Wang F, Xiao B, et al. Genetic association and transcriptome integration identify contributing genes and tissues at cystic fibrosis modifier loci. PLoS Genet. 2019;15(2):e1008007.
- Shanthikumar S, Neeland MN, Saffery R, et al. Gene modifiers of cystic fibrosis lung disease: a systematic review. Pediatr Pulmonol. 2019;54(9):1356–1366.
- Stanke F, Hector A, Hedtfeld S, et al. An informative intragenic microsatellite marker suggests the IL-1 receptor as a genetic modifier in cystic fibrosis. Eur Respir J. 2017;50(6):6.
- Bolund ACS, Starnawska A, Miller MR, et al. Lung function discordance in monozygotic twins and associated differences in blood DNA methylation. Clin Epigenetics. 2017;9(1):132.
- Collaco JM, Blackman SM, McGready J, et al. Quantification of the relative contribution of environmental and genetic factors to variation in cystic fibrosis lung function. J Pediatr. 2010;157(5):802–807.e801-803.
- Tan Q, Christiansen L, von Bornemann Hjelmborg J, et al. Twin methodology in epigenetic studies. J Exp Biol. 2015;218(Pt 1):134–139.
- Xiang Z, Yang Y, Chang C, et al. The epigenetic mechanism for discordance of autoimmunity in monozygotic twins. J Autoimmun. 2017;83:43–50.
- Generali E, Ceribelli A, Stazi MA, et al. Lessons learned from twins in autoimmune and chronic inflammatory diseases. J Autoimmun. 2017;83:51–61.
- Gomez-Cabrero D, Almgren M, Sjöholm LK, et al. High-specificity bioinformatics framework for epigenomic profiling of discordant twins reveals specific and shared markers for ACPA and ACPA-positive rheumatoid arthritis. Genome Med. 2016;8(1):124.
- Hwang JY, Lee HJ, Go MJ, et al. Genome-wide methylation analysis identifies ELOVL5 as an epigenetic biomarker for the risk of type 2 diabetes mellitus. Sci Rep. 2018;8(1):14862.
- Roos L, van Dongen J, Bell CG, et al. Integrative DNA methylome analysis of pan-cancer biomarkers in cancer discordant monozygotic twin-pairs. Clin Epigenetics. 2016;8(1):7.
- Schamschula E, Hagmann W, Assenov Y, et al. Immunotyping of clinically divergent p.Phe508del homozygous monozygous cystic fibrosis twins. J Cyst Fibros. 2021;20(1):149–153.
- Fasanelli F, Baglietto L, Ponzi E, et al. Hypomethylation of smoking-related genes is associated with future lung cancer in four prospective cohorts. Nat Commun. 2015;6(1):10192.
- Wrage M, Hagmann W, Kemming D, et al. Identification of HERC5 and its potential role in NSCLC progression. Int J Cancer. 2015;136(10):2264–2272.
- Baglietto L, Ponzi E, Haycock P, et al. DNA methylation changes measured in pre-diagnostic peripheral blood samples are associated with smoking and lung cancer risk. Int J Cancer. 2017;140(1):50–61.
- Bergougnoux A, Claustres M, De Sario A. Nasal epithelial cells: a tool to study DNA methylation in airway diseases. Epigenomics. 2015;7(1):119–126.
- Farré P, Jones MJ, Meaney MJ, et al. Concordant and discordant DNA methylation signatures of aging in human blood and brain. Epigenetics Chromatin. 2015;8(1):19.
- Houseman EA, Kim S, Kelsey KT, et al. DNA Methylation in Whole Blood: uses and Challenges. Curr Environ Health Rep. 2015;2(2):145–154.
- Huang Y-T, Chu S, Loucks EB, et al. Epigenome-wide profiling of DNA methylation in paired samples of adipose tissue and blood. Epigenetics. 2016;11(3):227–236.
- Um SW, Kim Y, Lee BB, et al. Genome-wide analysis of DNA methylation in bronchial washings. Clin Epigenetics. 2018;10(1):65.
- Choi J, Lyons DB, Kim MY, et al. DNA Methylation and Histone H1 Jointly Repress Transposable Elements and Aberrant Intragenic Transcripts. Mol Cell. 2020;77(2):310–323.e317.
- Pfeifer GP. Defining Driver DNA Methylation Changes in Human Cancer. Int J Mol Sci. 2018;19(4):1166.
- Tirado-Magallanes R, Rebbani K, Lim R, et al. Whole genome DNA methylation: beyond genes silencing. Oncotarget. 2017;8(3):5629–5637.
- Wolff F, Leisch M, Greil R, et al. The double-edged sword of (re)expression of genes by hypomethylating agents: from viral mimicry to exploitation as priming agents for targeted immune checkpoint modulation. Cell Commun Signal. 2017;15(1):13.
- Calle-Fabregat CDL, Morante-Palacios O, Ballestar E. Understanding the Relevance of DNA Methylation Changes in Immune Differentiation and Disease. Genes (Basel). 2020;11(1):110.
- Ciechomska M, Roszkowski L, Maslinski W. DNA Methylation as a Future Therapeutic and Diagnostic Target in Rheumatoid Arthritis. Cells. 2019;8(9):953.
- Hedrich CM, Mäbert K, Rauen T, et al. DNA methylation in systemic lupus erythematosus. Epigenomics. 2017;9(4):505–525.
- Saradna A, Do DC, Kumar S, et al. Macrophage polarization and allergic asthma. Transl Res. 2018;191:1–14.
- Hattori N, Ushijima T. Analysis of DNA Methylation in Tissues Exposed to Inflammation. Methods Mol Biol. 2018;1725:185–199.
- Scott M, De Sario A. DNA methylation changes in cystic fibrosis: cause or consequence? Clin Genet. 2020;98(1):3–9.
- Somineni HK, Venkateswaran S, Kilaru V, et al. Blood-Derived DNA Methylation Signatures of Crohn’s Disease and Severity of Intestinal Inflammation. Gastroenterology. 2019;156(8):2254–2265.e2253.
- Sun S, Barreiro LB. The epigenetically-encoded memory of the innate immune system. Curr Opin Immunol. 2020;65:7–13.
- Bray F, Ferlay J, Soerjomataram I, et al. Global cancer statistics 2018: GLOBOCAN estimates of incidence and mortality worldwide for 36 cancers in 185 countries. CA Cancer J Clin. 2018;68(6):394–424.
- Hanahan D, Weinberg RA. Hallmarks of cancer: the next generation. Cell. 2011;144(5):646–674.
- Chen L, Deng H, Cui H, et al. Inflammatory responses and inflammation-associated diseases in organs. Oncotarget. 2017;9(6):7204–7218.
- Poetsch AR. The genomics of oxidative DNA damage, repair, and resulting mutagenesis. Comput Struct Biotechnol J. 2020;18:207–219.
- Bouchard MJ, Navas-Martin S. Hepatitis B and C virus hepatocarcinogenesis: lessons learned and future challenges. Cancer Lett. 2011;305(2):123–143.
- Hattori N, Ushijima T. Epigenetic impact of infection on carcinogenesis: mechanisms and applications. Genome Med. 2016;8(1). DOI:10.1186/s13073-016-0267-2
- Mitra A, MacIntyre DA, Marchesi JR, et al. The vaginal microbiota, human papillomavirus infection and cervical intraepithelial neoplasia: what do we know and where are we going next? Microbiome. 2016;4(1):58.
- Maeda M, Moro H, Ushijima T. Mechanisms for the induction of gastric cancer by Helicobacter pylori infection: aberrant DNA methylation pathway. Gastric Cancer. 2017;20(Suppl 1):8–15.
- Takeshima H, Ushijima T. Accumulation of genetic and epigenetic alterations in normal cells and cancer risk. NPJ Precis Oncol. 2019;3(1):7.
- Eyvazi S, Vostakolaei MA, Dilmaghani A, et al. The oncogenic roles of bacterial infections in development of cancer. Microb Pathog. 2020;141:104019.
- Nakagawa T, Matsusaka K, Misawa K, et al. Stratification of HPV-associated and HPV-negative oropharyngeal squamous cell carcinomas based on DNA methylation epigenotypes. Int J Cancer. 2020;146(9):2460–2474.
- Mur LA, Huws SA, Cameron SJ, et al. Lung cancer: a new frontier for microbiome research and clinical translation. Ecancermedicalscience. 2018;12:866.
- Dong J, Ma Q. Integration of inflammation, fibrosis, and cancer induced by carbon nanotubes. Nanotoxicology. 2019;13(9):1244–1274.
- Husebø GR, Nielsen R, Hardie J, et al. Risk factors for lung cancer in COPD - results from the Bergen COPD cohort study. Respir Med. 2019;152:81–88.
- Hata A, Nakajima T, Matsusaka K, et al. A low DNA methylation epigenotype in lung squamous cell carcinoma and its association with idiopathic pulmonary fibrosis and poorer prognosis. Int J Cancer. 2020;146(2):388–399.
- Parris BA, O’Farrell HE, Fong KM, et al. Chronic obstructive pulmonary disease (COPD) and lung cancer: common pathways for pathogenesis. J Thorac Dis. 2019;11(Suppl 17):S2155–s2172.
- Neglia JP, FitzSimmons SC, Maisonneuve P, et al. The risk of cancer among patients with cystic fibrosis. Cystic Fibrosis and Cancer Study Group. N Engl J Med. 1995;332(8):494–499.
- Zhang Z, Wang J, He J, et al. Genetic variants in MUC4 gene are associated with lung cancer risk in a Chinese population. PLoS ONE. 2013;8(10):e77723.
- Dong Y, Zhou L, Zhao D, et al. MUC5AC enhances tumor heterogeneity in lung adenocarcinoma with mucin production and is associated with poor prognosis. Jpn J Clin Oncol. 2020;50(6):701–711.
- Albino D, Longoni N, Curti L, et al. ESE3/EHF controls epithelial cell differentiation and its loss leads to prostate tumors with mesenchymal and stem-like features. Cancer Res. 2012;72(11):2889–2900.
- Jäger B, Klatt D, Plappert L, et al. CXCR4/MIF axis amplifies tumor growth and epithelial-mesenchymal interaction in non-small cell lung cancer. Cell Signal. 2020;73:109672.
- O’Leary K, Shia A, Schmid P. Epigenetic Regulation of EMT in Non-Small Cell Lung Cancer. Curr Cancer Drug Targets. 2018;18(1):89–96.
- Warzecha CC, Carstens RP. Complex changes in alternative pre-mRNA splicing play a central role in the epithelial-to-mesenchymal transition (EMT). Semin Cancer Biol. 2012;22(5–6):417–427.
- Wright FA, Strug LJ, Doshi VK, et al. Genome-wide association and linkage identify modifier loci of lung disease severity in cystic fibrosis at 11p13 and 20q13.2. Nat Genet. 2011;43(6):539–546.
- He Q, Wang L, Zhang J, et al. CYP2E1 and GSTM1 gene polymorphisms, environmental factors, and the susceptibility to lung cancer. J Clin Lab Anal. 2018;32(6):e22403.
- Yu P, Kusuma JD, Suarez MAR, et al. Lung cancer susceptibility from GSTM1 deletion and air pollution with smoking status: a meta-prediction of worldwide populations. Oncotarget. 2018;9(57):31120–31132.
- Grieshober L, Graw S, Barnett MJ, et al. AHRR methylation in heavy smokers: associations with smoking, lung cancer risk, and lung cancer mortality. BMC Cancer. 2020;20(1):905.
- Park KH, Lo Han SG, Whang YM, et al. Single nucleotide polymorphisms of the TGFB1 gene and lung cancer risk in a Korean population. Cancer Genet Cytogenet. 2006;169(1):39–44.
- Risch A, Wikman H, Thiel S, et al. Glutathione-S-transferase M1, M3, T1 and P1 polymorphisms and susceptibility to non-small-cell lung cancer subtypes and hamartomas. Pharmacogenetics. 2001;11(9):757–764.
- Timofeeva MN, Hung RJ, Rafnar T, et al. Influence of common genetic variation on lung cancer risk: meta-analysis of 14 900 cases and 29 485 controls. Hum Mol Genet. 2012;21(22):4980–4995.
- Assenov Y, Müller F, Lutsik P, et al. Comprehensive Analysis of DNA Methylation Data with RnBeads. Nat Methods. 2014;11(11):1138–1140.
- Price ME, Cotton AM, Lam LL, et al. Additional annotation enhances potential for biologically-relevant analysis of the Illumina Infinium HumanMethylation450 BeadChip array. Epigenetics Chromatin. 2013;6(1):4.
- Kurdyukov S, Bullock M. DNA Methylation Analysis: choosing the Right Method. Biology (Basel). 2016;5(1):3.
- Reinius LE, Acevedo N, Joerink M, et al. Differential DNA Methylation in Purified Human Blood Cells: implications for Cell Lineage and Studies on Disease Susceptibility. PLoS ONE. 2012;7(7):e41361.
- Díez-Villanueva A, Mallona I, Peinado MA. Wanderer, an interactive viewer to explore DNA methylation and gene expression data in human cancer. Epigenetics Chromatin. 2015;8(1):22.
- Ernst J, Kellis M. Discovery and characterization of chromatin states for systematic annotation of the human genome. Nat Biotechnol. 2010;28(8):817–825.
- Ernst J, Kheradpour P, Mikkelsen TS, et al. Mapping and analysis of chromatin state dynamics in nine human cell types. Nature. 2011;473(7345):43–49.
- Euskirchen GM, Rozowsky JS, Wei CL, et al. Mapping of transcription factor binding regions in mammalian cells by ChIP: comparison of array- and sequencing-based technologies. Genome Res. 2007;17(6):898–909.
- Hudson ME, Snyder M. High-throughput methods of regulatory element discovery. Biotechniques. 2006;41(6): 673, 675, 677 passim.
- Grana O, Lopez-Fernandez H, Fdez-Riverola F, et al. Bicycle: a bioinformatics pipeline to analyze bisulfite sequencing data. Bioinformatics. 2018;34(8):1414–1415.
- Houseman EA, Accomando WP, Koestler DC, et al. DNA methylation arrays as surrogate measures of cell mixture distribution. BMC Bioinformatics. 2012;13(1):86.
- Hu H, Li B, Duan S. The Alteration of Subtelomeric DNA Methylation in Aging-Related Diseases. Front Genet. 2019;9:697.
- Elborn JS. Cystic fibrosis. Lancet. 2016;388(10059):2519–2531.
- Bergman Y, Cedar H. DNA methylation dynamics in health and disease. Nat Struct Mol Biol. 2013;20(3):274–281.
- Kaminsky ZA, Tang T, Wang S-C, et al. DNA methylation profiles in monozygotic and dizygotic twins. Nat Genet. 2009;41(2):240–245.
- Van Baak TE, Coarfa C, Dugué P-A, et al. Epigenetic supersimilarity of monozygotic twin pairs. Genome Biol. 2018;19(1):2.
- Downey DG, Bell SC, Elborn JS. Neutrophils in cystic fibrosis. Thorax. 2009;64(1):81–88.
- Holliday R, Pugh J. DNA modification mechanisms and gene activity during development. Science. 1975;187(4173):226–232.
- Levy H, Kalish LA, Huntington I, et al. Inflammatory markers of lung disease in adult patients with cystic fibrosis. Pediatr Pulmonol. 2007;42(3):256–262.
- Terry MB, Delgado-Cruzata L, Vin-Raviv N, et al. DNA methylation in white blood cells: association with risk factors in epidemiologic studies. Epigenetics. 2011;6(7):828–837.
- Guida F, Sandanger TM, Castagné R, et al. Dynamics of smoking-induced genome-wide methylation changes with time since smoking cessation. Hum Mol Genet. 2015;24(8):2349–2359.
- Teschendorff AE, Yang Z, Wong A, et al. Correlation of Smoking-Associated DNA Methylation Changes in Buccal Cells With DNA Methylation Changes in Epithelial Cancer. JAMA Oncol. 2015;1(4):476–485.
- Zhou G, Yang L, Gray A, et al. The role of desmosomes in carcinogenesis. Onco Targets Ther. 2017;10:4059–4063.
- Bibikova M, Barnes B, Tsan C, et al. High density DNA methylation array with single CpG site resolution. Genomics. 2011;98(4):288–295.
- Doi A, Park IH, Wen B, et al. Differential methylation of tissue- and cancer-specific CpG island shores distinguishes human induced pluripotent stem cells, embryonic stem cells and fibroblasts. Nat Genet. 2009;41(12):1350–1353.
- Irizarry RA, Ladd-Acosta C, Wen B, et al. The human colon cancer methylome shows similar hypo- and hypermethylation at conserved tissue-specific CpG island shores. Nat Genet. 2009;41(2):178–186.
- Ziller MJ, Gu H, Müller F, et al. Charting a dynamic DNA methylation landscape of the human genome. Nature. 2013;500(7463):477–481.
- Neri F, Rapelli S, Krepelova A, et al. Intragenic DNA methylation prevents spurious transcription initiation. Nature. 2017;543(7643):72–77.
- Yang X, Han H, De Carvalho DD, et al. Gene body methylation can alter gene expression and is a therapeutic target in cancer. Cancer Cell. 2014;26(4):577–590.
- Arechederra M, Daian F, Yim A, et al. Hypermethylation of gene body CpG islands predicts high dosage of functional oncogenes in liver cancer. Nat Commun. 2018;9(1):3164.
- Gu J, Stevens M, Xing X, et al. Mapping of Variable DNA Methylation Across Multiple Cell Types Defines a Dynamic Regulatory Landscape of the Human Genome. G3 (Bethesda). 2016;6(4):973–986.
- Arzate-Mejía RG, Recillas-Targa F, Corces VG. Developing in 3D: the role of CTCF in cell differentiation. Development. 2018;145(6):dev137729.
- Herold M, Bartkuhn M, Renkawitz R. CTCF: insights into insulator function during development. Development. 2012;139(6):1045–1057.
- Ruiz-Velasco M, Kumar M, Lai MC, et al. CTCF-Mediated Chromatin Loops between Promoter and Gene Body Regulate Alternative Splicing across Individuals. Cell Syst. 2017;5(6):628–637.e626.
- Ambrosi C, Manzo M, Baubec T. Dynamics and Context-Dependent Roles of DNA Methylation. J Mol Biol. 2017;429(10):1459–1475.
- Lévêque M, Le Trionnaire S, Del Porto P, et al. The impact of impaired macrophage functions in cystic fibrosis disease progression. J Cyst Fibros. 2017;16(4):443–453.
- Barnes PJ. Cellular and molecular mechanisms of asthma and COPD. Clin Sci. 2017;131(13):1541–1558.
- Houghton AM. Mechanistic links between COPD and lung cancer. Nat Rev Cancer. 2013;13(4):233–245.
- Shields PG, Berman M, Brasky TM, et al. A Review of Pulmonary Toxicity of Electronic Cigarettes in the Context of Smoking: a Focus on Inflammation. Cancer Epidemiol Biomarkers Prev. 2017;26(8):1175–1191.
- Siegel RL, Miller KD, Jemal A. Cancer statistics, 2019. CA Cancer J Clin. 2019;69(1):7–34.
- Strzelak A, Ratajczak A, Adamiec A, et al. Tobacco Smoke Induces and Alters Immune Responses in the Lung Triggering Inflammation, Allergy, Asthma and Other Lung Diseases: a Mechanistic Review. Int J Environ Res Public Health. 2018;15(5):1033.
- Chaparro C, Keshavjee S. Lung transplantation for cystic fibrosis: an update. Expert Rev Respir Med. 2016;10(12):1269–1280.
- Ranganathan SC, Hall GL, Sly PD, et al., & Australian Respiratory Early Surveillance Team for Cystic, F. Early Lung Disease in Infants and Preschool Children with Cystic Fibrosis. What Have We Learned and What Should We Do about It? Am J Respir Crit Care Med. 2017;195(12):1567–1575. .
- Li Y, Sun Z, Wu Y, et al. Cystic fibrosis transmembrane conductance regulator gene mutation and lung cancer risk. Lung Cancer. 2010;70(1):14–21.
- Bronsveld I, Mekus F, Bijman J, et al. Chloride conductance and genetic background modulate the cystic fibrosis phenotype of ΔF508 homozygous twins and siblings. J Clin Investig. 2001;108(11):1705–1715.
- MacGregor AJ, Snieder H, Schork NJ, et al. Twins: novel uses to study complex traits and genetic diseases. Trends Genet. 2000;16(3):131–134.
- Gentilini D, Garagnani P, Pisoni S, et al. Stochastic epigenetic mutations (DNA methylation) increase exponentially in human aging and correlate with X chromosome inactivation skewing in females. Aging (Albany NY). 2015;7(8):568–578.
- Jones PA, Baylin SB. The epigenomics of cancer. Cell. 2007;128(4):683–692.
- Bell JT, Tsai PC, Yang TP, et al. Epigenome-wide scans identify differentially methylated regions for age and age-related phenotypes in a healthy ageing population. PLoS Genet. 2012;8(4):e1002629.
- Boks MP, Derks EM, Weisenberger DJ, et al. The relationship of DNA methylation with age, gender and genotype in twins and healthy controls. PLoS ONE. 2009;4(8):e6767.
- Hannon E, Knox O, Sugden K, et al. Characterizing genetic and environmental influences on variable DNA methylation using monozygotic and dizygotic twins. PLoS Genet. 2018;14(8):e1007544.
- Horvath S. DNA methylation age of human tissues and cell types. Genome Biol. 2013;14(10):R115.
- Liu J, Morgan M, Hutchison K, et al. A study of the influence of sex on genome wide methylation. PLoS ONE. 2010;5(4):e10028.
- Teschendorff AE, Menon U, Gentry-Maharaj A, et al. Age-dependent DNA methylation of genes that are suppressed in stem cells is a hallmark of cancer. Genome Res. 2010;20(4):440–446.
- Yet I, Tsai P-C, Castillo-Fernandez JE, et al. Genetic and environmental impacts on DNA methylation levels in twins. Epigenomics. 2016;8(1):105–117.
- Fraga MF, Ballestar E, Paz MF, et al. Epigenetic differences arise during the lifetime of monozygotic twins. Proc Natl Acad Sci U S A. 2005;102(30):10604–10609.
- Talens RP, Christensen K, Putter H, et al. Epigenetic variation during the adult lifespan: cross-sectional and longitudinal data on monozygotic twin pairs. Aging Cell. 2012;11(4):694–703.
- Artis D, Spits H. The biology of innate lymphoid cells. Nature. 2015;517(7534):293–301.
- Brown TA, Lee JW, Holian A, et al. Alterations in DNA methylation corresponding with lung inflammation and as a biomarker for disease development after MWCNT exposure. Nanotoxicology. 2016;10(4):453–461.
- Ehrlich M. DNA hypermethylation in disease: mechanisms and clinical relevance. Epigenetics. 2019;14(12):1141–1163.
- Issa J-P. Aging and epigenetic drift: a vicious cycle. J Clin Invest. 2014;124(1):24–29.
- Nunes SP, Diniz F, Moreira-Barbosa C, et al. Subtyping Lung Cancer Using DNA Methylation in Liquid Biopsies. J Clin Med. 2019;8(9):1500.
- Gunasekara CJ, Scott CA, Laritsky E, et al. A genomic atlas of systemic interindividual epigenetic variation in humans. Genome Biol. 2019;20(1):105.
- Bonné S, Gilbert B, Hatzfeld M, et al. Defining desmosomal plakophilin-3 interactions. J Cell Biol. 2003;161(2):403–416.
- Dusek RL, Attardi LD. Desmosomes: new perpetrators in tumour suppression. Nat Rev Cancer. 2011;11(5):317–323.
- Hatzfeld M, Wolf A, Keil R. Plakophilins in desmosomal adhesion and signaling. Cell Commun Adhes. 2014;21(1):25–42.
- Hofmann I, Casella M, Schnölzer M, et al. Identification of the junctional plaque protein plakophilin 3 in cytoplasmic particles containing RNA-binding proteins and the recruitment of plakophilins 1 and 3 to stress granules. Mol Biol Cell. 2006;17(3):1388–1398.
- Schmidt A, Langbein L, Prätzel S, et al. Plakophilin 3 – a novel cell-type-specific desmosomal plaque protein. Differentiation. 1999;64(5):291–306.
- Adam D, Roux-Delrieu J, Luczka E, et al. Cystic fibrosis airway epithelium remodelling: involvement of inflammation. J Pathol. 2015;235(3):408–419.
- Besnard V, Dagher R, Madjer T, et al. Identification of periplakin as a major regulator of lung injury and repair in mice. JCI Insight. 2018;3(5). DOI:10.1172/jci.insight.90163
- Müller L, Rietscher K, Keil R, et al. Plakophilin 3 phosphorylation by ribosomal S6 kinases supports desmosome assembly. J Cell Sci. 2020;133:8.
- Breuninger S, Reidenbach S, Sauer CG, et al. Desmosomal plakophilins in the prostate and prostatic adenocarcinomas: implications for diagnosis and tumor progression. Am J Pathol. 2010;176(5):2509–2519.
- Demirag GG, Sullu Y, Yucel I. Expression of Plakophilins (PKP1, PKP2, and PKP3) in breast cancers. Med Oncol. 2012;29(3):1518–1522.
- Takahashi H, Nakatsuji H, Takahashi M, et al. Up-regulation of plakophilin-2 and Down-regulation of plakophilin-3 are correlated with invasiveness in bladder cancer. Urology. 2012;79(1):240.e241–248.
- Valladares-Ayerbes M, Diaz-Prado S, Reboredo M, et al. Evaluation of plakophilin-3 mRNA as a biomarker for detection of circulating tumor cells in gastrointestinal cancer patients. Cancer Epidemiol Biomarkers Prev. 2010;19(6):1432–1440.
- Furukawa C, Daigo Y, Ishikawa N, et al. Plakophilin 3 oncogene as prognostic marker and therapeutic target for lung cancer. Cancer Res. 2005;65(16):7102–7110.
- Sklyarova T, Bonne S, D’Hooge P, et al. Plakophilin-3-deficient mice develop hair coat abnormalities and are prone to cutaneous inflammation. J Invest Dermatol. 2008;128(6):1375–1385.
- Sklyarova T, van Hengel J, Van Wonterghem E, et al. Hematopoietic plakophilin-3 regulates acute tissue-specific and systemic inflammation in mice. Eur J Immunol. 2015;45(10):2898–2910.
- Chae YK, Choi WM, Bae WH, et al. Overexpression of adhesion molecules and barrier molecules is associated with differential infiltration of immune cells in non-small cell lung cancer. Sci Rep. 2018;8(1):1023.
- Bruscia EM, Bonfield TL. Innate and Adaptive Immunity in Cystic Fibrosis. Clin Chest Med. 2016;37(1):17–29.
- Borghese B, Barbaux S, Mondon F, et al. Research resource: genome-wide profiling of methylated promoters in endometriosis reveals a subtelomeric location of hypermethylation. Mol Endocrinol. 2010;24(9):1872–1885.
- Dyson MT, Roqueiro D, Monsivais D, et al. Genome-wide DNA methylation analysis predicts an epigenetic switch for GATA factor expression in endometriosis. PLoS Genet. 2014;10(3):e1004158.
- Toiyama Y, Okugawa Y, Kondo S, et al. Comprehensive analysis identifying aberrant DNA methylation in rectal mucosa from ulcerative colitis patients with neoplasia. Oncotarget. 2018;9(69):33149–33159.
- Li L, Wei J, Li S, et al. The deubiquitinase USP13 stabilizes the anti-inflammatory receptor IL-1R8/Sigirr to suppress lung inflammation. EBioMedicine. 2019;45:553–562.
- Horne DJ, Randhawa AK, Chau TT, et al. Common polymorphisms in the PKP3-SIGIRR-TMEM16J gene region are associated with susceptibility to tuberculosis. J Infect Dis. 2012;205(4):586–594.
- Blanchard AC, Waters VJ. Microbiology of Cystic Fibrosis Airway Disease. Semin Respir Crit Care Med. 2019;40(6):727–736.
- Françoise A, Héry-Arnaud G. The Microbiome in Cystic Fibrosis Pulmonary Disease. Genes (Basel). 2020;11(5):536.