ABSTRACT
Social epigenomics is an emerging field in which social scientist collaborate with computational biologists, especially epigeneticists, to address the underlying pathway for biological embedding of life experiences. This social epigenomics study included long-term childhood cancer survivors enrolled in the St. Jude Lifetime Cohort. DNA methylation (DNAm) data were generated using the Illumina EPIC BeadChip, and three social determinants of health (SDOH) factors were assessed: self-reported educational attainment, personal income, and an area deprivation index based on census track data. An epigenome-wide association study (EWAS) was performed to evaluate the relation between DNAm at each 5’-cytosine-phosphate-guanine-3’ (CpG) site and each SDOH factor based on multivariable linear regression models stratified by ancestry (European ancestry, n = 1,618; African ancestry, n = 258). EWAS among survivors of European ancestry identified 130 epigenome-wide significant SDOH–CpG associations (P < 9 × 10−8), 25 of which were validated in survivors of African ancestry (P < 0.05). Thirteen CpGs were associated with all three SDOH factors and resided at pleiotropic loci in cigarette smoking–related genes (e.g., CLDND1 and CPOX). After accounting for smoking and body mass index, these associations remained significant with attenuated effect sizes. Seven of 13 CpGs were associated with gene expression level based on 57 subsamples with blood RNA sequencing data available. In conclusion, DNAm signatures, many resembling the effect of tobacco use, were associated with SDOH factors among survivors of childhood cancer, thereby suggesting that biologically distal SDOH factors influence health behaviours or related factors, the epigenome, and subsequently survivors’ health.
Introduction
Social and behavioural epigenomics is an emerging transdisciplinary field that investigates how social determinants of health (SDOH) and health behaviours modulate the human epigenome and thus influence health and wellness [Citation1,Citation2]. Among various epigenetic modifications, DNA methylation (DNAm) has been the most widely studied; multiple groups have reported significant associations between DNAm and socio-economic status (SES) [Citation1,Citation3–7], educational attainment [Citation8,Citation9], and health behaviours [Citation10]. These findings support the hypothesis that DNAm is a potential underlying mechanism for biological embedding of life experiences, including physiologically distal SDOH factors and more directly relevant health behaviours. Previous studies have identified variations of DNAm across the methylome related to SDOH factors that resemble variations seen among persons who engage in smoking, risky or heavy alcohol consumption, or who are obese [Citation5,Citation8,Citation9,Citation11,Citation12]. Moreover, findings demonstrate that associations between some of these DNAm variations and educational attainment remain significant after accounting for known risk factors such as smoking, thereby suggesting traces of other exposures for less-educated people [Citation8,Citation9]
Epigenetic signatures provide insight into the mechanistic underpinnings of the link between SES and chronic disease risk [Citation6]. Heritability analyses have shown an approximately 18% variance of DNAm between monozygotic and dizygotic twins, but this difference decreased as the twins aged, implying that non-genetic factors, including SES, might influence DNAm [Citation13,Citation14]. A recent study showed persistent epigenetic ageing and differentially methylated loci among adolescent/young adult Hodgkin lymphoma survivors compared to their unaffected twins [Citation15].
A recent epigenetic analysis of peripheral blood samples from long-term survivors of childhood cancer participating in the St. Jude Lifetime Cohort Study (SJLIFE) found that specific cancer treatments are associated with variations in DNAm decades after exposure [Citation16,Citation17]. Moreover, a subset of treatment-associated DNAm 5’-cytosine-phosphate-guanine-3’ (CpG) sites was also significantly associated with cardiometabolic risk, and hence potentially mediated treatment-related toxicities leading to chronic health conditions [Citation16]. Although intensive treatment exposures would be expected to leave persistent epigenetic signatures within a survivor’s methylome, socio-economic and behavioural factors during the person’s life-course may also have an enduring impact on his/her DNAm.
To our knowledge, no social epigenomic studies investigated the links between SDOH factors and potential epigenetic modifications in survivors of childhood cancer. To fill this gap, we employed a comprehensive epigenome-wide approach to identify DNAm CpGs associated with three SDOH factors (i.e., educational attainment, personal income, and area SES deprivation). These factors may influence the health disparity of childhood cancer survivors who have different racial backgrounds [Citation18] and were taken into consideration based on the data availability in the SJLIFE survey and missingness of available variables. In addition, we annotated these CpGs with associated traits (exposures or outcomes) catalogued in the EWAS Atlas [Citation19].
Methods
Study population
SJLIFE is a retrospective hospital-based cohort study of 5-year survivors of childhood cancer with prospective clinical follow-up [Citation20]. During periodic follow-up visits, survivors reported social/demographic information based on standard questionnaires and underwent comprehensive, systematic clinical assessments. Among the SJLIFE participants, epigenome-wide DNAm profiling data were available for 2,052 survivors of European ancestry and 370 survivors of African ancestry. For the current report, we excluded survivors younger than 25 years of age (n = 533) who may have not yet completed their education (e.g., college or post-graduate degree). In addition, we excluded outliers from principal components analysis (PCA) of genotypes (n = 13) to ensure a relatively homogenous population substructure in the analytic data set. The remaining 1,876 survivors (1,618 of European ancestry and 258 of African ancestry) were included in the final analysis. All survivors were deemed free of childhood cancer (survival ≥10 years from cancer diagnosis) and fewer than 0.5% of SJLIFE survivors experienced subsequent haematologic malignancies, as of the most last follow-up [Citation21].
Social determinants of health
The SDOH factors of cancer survivors at personal and neighbourhood levels were collected before or at the same time as the blood draw for DNAm analysis. The personal-level SDOH factors included educational attainment and personal annual income as reported in SJLIFE surveys. Personal educational attainment was categorized into five levels (below high school, high school or training after high school, some college, college graduate, and post-graduate), and personal annual incomes was categorized into five levels (none, <$20,000, $20,000 to $40,000, $40,000 to $60,000, and ≥$60,000 [U.S. dollars]). Neighbourhood social determinants included area SES deprivation for each survivor by using the Area Deprivation Index (ADI), which consists of 17 neighbourhood-based SES measures, including income, employment, education, and housing status, mainly collected from the American Community Survey [Citation22,Citation23]. Participants’ residential addresses were geocoded using geographic identifiers from the U.S. Census Bureau, which were then linked to the national ADI file. These measures present disadvantaged SES and physical environment by census blocks in the U.S.A., and each census block receives a percentile ranking, with minimum disadvantage in the first percentile and maximum disadvantage in the 100th percentile. For ADI, we considered >75th percentile, 40th to 75th percentile, and <40th percentile as high, moderate, and low SES deprivation area, respectively.
BMI and smoking
At each follow-up examination, the height and weight of the SJLIFE participant was measured. BMI of survivors was calculated as weight (kg) divided by height (m) squared (kg/m2). According to the standardized classification of the World Health Organization, BMI was categorized as <18.5, 18.5 to <25.0, 25.0 to <30.0 and ≥30.0 kg/m2. BMI was considered a health behaviour–related factor [Citation24,Citation25]. Smoking status was assessed based on self-reported questionnaires at each follow-up visit and categorized as non-smoker versus former or current smoker. BMI and smoking status at the same or closest date of blood draw for DNAm analysis were used in the current analysis.
DNA methylation profiling and analysis
DNAm data were generated using MethylationEPIC BeadChip and analysed as previously described [Citation16,Citation26]. In brief, methylation raw intensity data were analysed in R (version 3.6.1) using the minfi package [Citation27]. M-values (logit transformation of β-value) were calculated and subsequently used as the dependent variable of regression analyses [Citation28]. Houseman’s method was used to estimate leukocyte cell subtype proportions (monocyte, granulocyte, CD4 + T cells, CD8 + T cells, natural killer cells, and B cells) [Citation29,Citation30]. A PCA of DNAm data was performed to quantify latent structures or batch effects (Supplementary Figure 1). We used the array annotations (GRCh38 version) provided by Illumina (San Diego, CA) to map probes to their corresponding genes.
RNA-sequence profiling and data processing
All RNA-sequencing libraries underwent 151-cycle paired-end sequencing on the Illumina NovaSeq 6000 System. Sequencing reads were mapped to the GRCh38 build using STAR [Citation31]. Read counts by gene were generated using HTSeq [Citation32], and genes with read counts fewer than 10 were excluded. Transcript per million (TPM) reads were further calculated, and log2(TPM+0.01) values were quantile-normalized using Limma [Citation33]. The processed gene-by-sample data matrices were used to quantify the gene expression level in further statistical analyses.
Statistical analyses
To illustrate the rationale underlying our modelling approaches, we have provided a conceptual framework for the social epigenomics study in survivors of childhood cancer (Supplementary Figure 2). We hypothesized poor SDOH as ‘upstream exposures’ and risky health behaviours or related factors as ‘downstream exposures’ that influence epigenetic mechanisms (e.g., DNAm) and regulate gene expression, which ultimately affects health outcomes. Survivors of European ancestry (n = 1,618) and those of African ancestry (n = 258) were analysed separately, with the former designated as the primary dataset and the latter as the exploratory dataset due to its limited sample size. The EWAS analysis for the association between methylation level at each CpG site (dependent variable) and each SDOH factor (independent variable) was conducted using multiple linear regressions with covariate adjustments including sex, attained age, specific cancer treatment exposures (chemotherapy agents and radiation therapy sites), leukocyte-subtype proportions, significant genetic principal components based on genotypes derived from existing whole-genome sequencing [Citation34] and methylation principal components (model 1). Health behaviours and related factors (smoking and BMI) were also adjusted to evaluate the contribution of each SDOH factor on the DNAm level independent of health behaviours and related factors (model 2). However, because health behaviours and related factors are potentially intermediate variables and on the causal path from SDOH factors to DNAm, to avoid over adjustment [Citation35], we started with epigenome-wide significant results from model 1 as the primary results but retained only those CpGs satisfying P < 0.05 after Bonferroni correction in model 2. We further conducted a meta-analysis of SDOH EWAS among survivors of African ancestry and those of European ancestry to provide overall evidence of epigenetic association with SDOH factors and evaluations for potential heterogeneity in epigenetic association with SDOH factors between the two ancestral groups by using I2 and P-value (Phet) calculated from the Cochran’s Q statistic [Citation36]. All analyses were repeated considering an alternative modelling scheme for SDOH factors in which each factor was classified as one of two groups (i.e., a binary variable): high school and above vs. others for educational attainment; none vs. others for personal income; and >75% vs. others for ADI. We used R package CpGassoc [Citation37] for the linear-regression analysis and P < 9 × 10−8 as the epigenome-wide significance threshold [Citation38]. EWAS results were visualized using circos plots (Circos v.0.69) [Citation39]. Using the EWAS Atlas, we annotated the significant CpGs in our current EWAS [Citation19]. A linear-regression model was fit for the association between the expression level of each gene and the DNAm level of a CpG site while adjusting for age and sex. The Spearman correlations across each paired SDOH factors were illustrated by a heatmap plot. Differentially methylated region (DMR) analysis was performed using the ipDMR method [Citation40]. Potential gene-environment interactions between single-nucleotide polymorphisms (SNPs) (within 1-Mb window) and SDOH factors for DNAm of CpGs were examined using multiple linear regression (DNAm = β0 + β1 × SNP + β2 × SDOH + β3 × SNP × SDOH + other covariates) with multiple testing correction by the Benjamini-Hochberg method. All statistical analyses were performed using R.3.6.3 [Citation41] or SAS 9.4 (SAS Institute Inc., Cary, NC) and all statistical tests were two-sided.
Results
Characteristics of the study population
General characteristics, SDOH factors, and clinical features, including primary cancer diagnoses and treatment information, for the study population are provided in . Study participants included childhood cancer survivors of African ancestry (n = 258; median time between cancer diagnosis to blood draw for DNAm = 25.2 years, interquartile range [IQR] = 19.9–32.1 years) and European ancestry (n = 1,618; median time between cancer diagnosis to blood draw for DNAm = 27.3 years, IQR = 21.1–33.7 years). For participants of African ancestry, the median age at primary cancer diagnosis was 9.6 (IQR = 4.2–14.4) years and that at blood draw for DNAm was 33.9 (IQR = 29.4–39.6) years; for participants of European ancestry, the ages were 9.0 (IQR = 3.8–14.4) years and 35.3 (IQR = 30.3–42.1) years. The proportion of participants who were female was 53.1% or 40.4% in those of African or European ancestry, respectively. More than 60% of participants were overweight (BMI 25.0 to <30 kg/m2, 29.5% in African ancestry and 29.2% in European ancestry) or obese (BMI ≥30 kg/m2, 32.2% in African ancestry and 32.6% in European ancestry). A higher proportion of participants of European ancestry was smokers compared to that of participants of African ancestry (41.8% versus 29.8%, P < 0.0001). The three SDOH factors were significantly different between survivors of African ancestry and those of European ancestry, with those of African ancestry having lower educational attainment (P < 0.0001), lower personal annual income (P < 0.0001), and residing in neighbourhoods with more disadvantaged socio-economic and physical environment, as measured by ADI (P < 0.0001). Furthermore, SDOH factors showed weak correlations with each other (|Spearman’s correlation| <0.4, Supplementary Figure 3).
Table 1. Characteristics of study participants.
Association of smoking and BMI with SDOH factors among survivors of European ancestry
Smoking behaviour was associated with educational attainment with a significant trend across five categories (P < 2.2 × 10−16). Most (67.9%) survivors with less than a high school education were current of former smokers, as compared to 28.9% of college graduates and 14.5% of post-graduates (). Smoking behaviour was also associated with personal income with ever smokers ranging between 39.3% and 51.7% and a significant trend across five categories (P = 8.5 × 10−5). Smoking was associated with ADI, and the ever smokers were 29.0%, 39.1%, and 50.3% for survivors with low, intermediate, or high SES deprivation, respectively. Similarly, BMI was associated with educational attainment and ADI but not with personal income ().
Table 2. Association of smoking and BMI with SDOH factors among survivors of European ancestry.
Association of DNAm sites with SDOH factors among survivors of European ancestry
The association p-values are shown in the circos plot for three series of EWAS analyses among survivors of European ancestry (). Quantile-Quantile plots for the distribution of observed and expected p-values for EWAS between DNAm CpG sites and SDOH factors showed moderately low genomic inflation factors between 1.20 and 1.35 with adjustments for genetic and methylation principal components, as compared to much higher genomic inflation factors (1.71–3.87) without such adjustments (Supplementary Figure 1). We identified 130 epigenome-wide significant SDOH–CpG associations (Supplementary Table 1), including educational attainment (n = 88), personal income (n = 23), and ADI (n = 19). Thirteen significant DNAm CpGs (cg01731783, cg08840017, cg00385142, cg18754985, cg08064403, cg05659611, cg19859270, cg04180924, cg00010201, cg02978227, cg02657160, cg05575921, cg26768182) were associated with all three SDOH factors and mapped to five genomic regions on chromosomes 2, 3, 5, 9, and 14 harbouring genes, including GPR55, CLDND1, CPOX, GPR15, AHRR, PRRC2B, and ELMSAN1, which previously have been reported as associated with smoking exposures (). After we adjusted for BMI and smoking, all 13 CpGs remained statistically significant (P < 0.05) but with attenuated effect sizes for educational attainment (mean effect sizes remaining = 36.8% of the effect sizes unadjusted for BMI and smoking, range = 30.8%-48.8%), personal income (mean = 48.3%, range = 38.5%-58.8%), and ADI (mean, 43.3%, range = 35.1%-61.5%). In the model for each SDOH factor adjusted for BMI and smoking, there were epigenome-wide significant associations between educational attainment and cg04180924 (chr3, CPOX, P = 2.0 × 10−8), cg04885881 (chr1, intergenic, P = 1.3 × 10−9), cg06359375 (chr22, HPS4, P = 2.0 × 10−8) and a significant association between personal income and cg04180924 (chr3, CPOX, P = 5.4 × 10−9). No single CpG reached an epigenome-wide significant level for ADI.
Table 3. DNA methylation CpG sites that are significantly associated with all three socio-economic factors in survivors of European ancestry.
Figure 1. Circos plot for epigenome-wide association studies of SDOH factors among survivors of European ancestry.
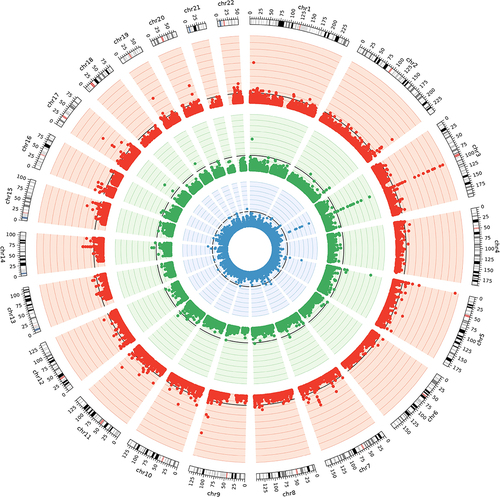
Functional implication of SODH-associated CpG sites
Based on the RNA sequencing data available for 57 samples, we calculated the expression quantitative methylation (eQTM) for each pair of the 13 CpGs and mapped genes ( and Supplementary Table 2). Three pairs, including cg00385142 (CLDND1), cg18754985 (CLDND1), and cg05575921 (EXOC3), were significant in survivors and were previously known cis-eQTMs (https://genenetwork.nl/biosqtlbrowser/). However, the following four known cis-eQTMs were not statistically significant in our study: cg19859270 (CPOX), cg19859270 (CLDND1), cg02657160 (CLDND1), and cg02657160 (CPOX). Another four pairs [cg08064403 (CLDND1), cg05659611 (CLDND1), cg19859270 (GPR15), and cg00010201 (CPOX)] were significant in survivors but have not been catalogued in the aforementioned biosqtl database.
Regional association with the peak and neighbouring CpGs was illustrated with coMET plot for all five genomic regions ( and Supplementary Figure 4). Nine CpGs mapped to the chr3 region () and were moderately correlated with pairwise Pearson correlation coefficients ranging between 0.48 and 0.73. highlights four CpGs (cg00385142, cg18754985, cg05659611, and cg08064403) that were eQTMs for CLDND1, one CpG (cg19859270) was an eQTM for GPR15, and another (cg02657160) was an eQTM for CPOX. We also performed PANTHER pathway analysis but did not find any significant biological pathway with the overrepresentation test based on the group of genes to which all significant CpGs were mapped.
Comparison to previously established findings in the EWAS catalogue
To determine whether the SDOH-associated CpGs identified in the current study have been previously associated with diseases or traits (Supplementary Table 3), we cross-referenced the EWAS Atlas. In the EWAS Atlas, a total of 101 associations between blood-based DNAm at 47 CpGs and 24 traits were reported at epigenome-wide significance level (P < 9 × 10−8). Across traits, smoking had the highest number of associated CpGs (n = 35), followed by ageing (n = 8) and educational attainment (n = 7). Among the CpGs associated with SDOH factors in our analysis, cg05575921 was associated with the highest number of different traits (n = 12), followed by cg21566642 (n = 10) and cg01940273 (n = 9). Among the 88 CpGs associated with educational attainment in the current study, 15 overlapped with findings from two previous EWAS studies of educational attainment: detailed association results for 37 CpGs identified in one study and 58 CpGs identified in the other study were provided in the current study (Supplementary Table 4).
Association of DNAm sites with SDOH factors among survivors of African ancestry
EWAS analyses were performed in survivors of African ancestry, and no DNAm CpGs reached epigenome-wide significance level (P < 9 × 10−8) due to the limited sample size. However, we assessed the 130 SDOH–CpG associations of genome-wide significance found in survivors of European ancestry in survivors of African ancestry for comparison (Supplementary Table 5). Among the 130 SDOH–CpG associations, 26 were validated in survivors of African ancestry (P < 0.05), which included 15 for educational attainments, eight for personal income, and three for ADI.
Secondary analyses including Meta-EWAS, dichotomous SDOH classifications, differentially methylated regions, and gene-SDOH interactions
Meta-analysis of SDOH EWAS among survivors of African ancestry and those of European ancestry showed a total of 448 epigenome-wide significant SDOH–CpG associations (Supplementary Table 6); 118 reached the epigenome-wide significance level among survivors of European ancestry alone and were included as one of the 130 SDOH–CpG associations listed in Supplementary Table 1. Notably, 88 (19.6%) associations demonstrated at least moderate heterogeneity with I2 > 50% between two-ancestral groups. We also considered an alternative modelling scheme for SDOH factors by classifying each factor into two groups (i.e., a binary variable). Meta-EWAS results based on the dichotomous SDOH classifications showed fewer epigenome-wide significant SDOH–CpG associations (n = 65) (Supplementary Table 7). DMR analysis identified 330, 27, and 32 novel associations with educational attainment, personal income, and ADI, respectively (Supplementary Table 8). We examined potential gene-SDOH interactions for DNAm of CpGs within 130 epigenome-wide significant SDOH–CpG associations and identified three statistically significant (FDR <0.05) interactions between 3 SNPs (rs77289732, rs2470852, and rs2470835) and personal income for DNAm level of cg08064403 and between five SNPs (rs142403317, rs149398072, rs112330421, rs12242855, and rs12255625) and educational attainment for cg22543377, after correction for multiple testing by the Benjamini-Hochberg method (Supplementary Table 9).
Discussion
This first social epigenomic study conducted among survivors of childhood cancer examined the associations between SDOH factors and DNAm. We demonstrated 130 SDOH–CpG associations for educational attainment, personal income, or ADI at epigenome-wide significance. However, we found far fewer CpGs than those previously associated with cancer treatment exposures (n = 935) [Citation16,Citation17], and no sites overlapped. The absolute values of effect size for associations between CpGs and SDOH factors (mean = 0.027, SD = 0.023) was significantly smaller than those for associations between CpGs and intensive cancer treatment modalities (mean = 0.11, SD = 0.059). Of the three SDOH factors we examined, educational attainment was the most informative with 88 significant CpG associations.
Most of the SDOH-associated CpGs mapped to genes known to have DNAm associated with smoking exposure, suggesting that social and behavioural exposures to lower educational attainment, lower income, and a higher SES-disadvantaged neighbourhood resemble the effect of tobacco use. Specifically, smoking influences the expression or DNAm of GPR15 [Citation42], CPOX [Citation43], and CLDND1 [Citation44], suggesting that these gene-environment interactions are implicated along the mechanistic pathway of SDOH factors and health outcomes. This evidence supports our hypothesized framework in which social adversity is more of a distal exposure factor, whereas smoking or any other health behaviour is more of a proximal factor related to health outcomes. Notably, these CpGs remained significant after adjusting for smoking and BMI, though the average effect sizes were smaller than the unadjusted effect sizes for educational attainment, personal income, and ADI, respectively. Moreover, most effect sizes were very small (<5%) with a few up to as high as 13%.
The study’s overall findings are consistent with our hypothesis, and the consensus in the field that health behaviours or health behaviour–related factors are the key mediating mechanism between distal social milieu of health and health outcomes [Citation45–47]. Furthermore, some of our robust findings were not related to smoking exposures. For example, cg06359375 was uniquely associated with educational attainment (but no personal income or ADI) at the epigenome-wide significant level, with no attenuation for the effect size after adjusting for BMI and smoking. This CpG is mapped to the HPS4 gene, which was previously associated with cognitive function [Citation48].
DNAm associations with educational attainment do not necessarily imply anything about cognitive function but can be proxies for SES, as well as all the associated stressors and comorbidities that accompany lower SES. The effects on cognitive function cannot be disentangled from other social status confounders, such as stress and health behaviours, that are also linked with lower educational attainment.
In non-cancer populations, two large EWAS for SDOH factors have been conducted that specifically addressed educational attainment [Citation8,Citation9]. One large meta-analysis including 27 cohort studies and 10,767 individuals of European ancestry identified 37 CpGs associated with educational attainment in the basic model with nine CpGs remaining in the model adjusted for BMI and smoking status (P < 9 × 10−8). The other meta-analysis with four cohorts and 4,152 individuals from the Netherlands identified 58 CpGs that were associated with educational attainment, and nine CpGs remained significant (P < 9 × 10−8) after adjusting for smoking.
The current study identified 130 CpGs associated with SDOH factors including 88 educational attainment related CpGs, of which only 15 were previously identified as CpGs for educational attainment (Supplementary Table 10), suggesting that substantial differences exist in the methylome and its relations with educational attainment between a cancer survivor population and the general population. This was further supported by previously established eQTMs in the general population that were not significant in survivors. To the contrary, we observed strong eQTMs that were not in the biosqtl database.
The observed differences in DNAm associations with educational attainment between cancer survivors and individuals in the general population may have arisen from survivors’ experience of cancer and its treatment in childhood. For instance, we speculate that cancer survivors are more resilient to disadvantages associated with lower educational attainment due to having overcome extreme difficulties associated with childhood cancer. Financial hardship associated with childhood cancer may impede survivors from attaining higher education, and those who achieve higher education under financial hardship may be exceptionally resourceful/successful.
We did not find any significant biological pathways in PANTHER pathways, with the overrepresentation test based on the group of genes to which all significant CpGs were mapped. Biological pathways underpinning the SES influences that increase survivors’ vulnerability to adverse health outcomes have not been determined and epidemiological research has thus far yielded only weak and inconsistent evidence on the epigenetics of early-life stress [Citation49]. However, according to a biological embedding of childhood adversity model, childhood stress can be programmed into macrophages through epigenetic markings, post-translational modifications, and tissue remodelling [Citation50]. This could stimulate immune cells to mount an excessive inflammatory response to microbial challenges associated with insensitivity to inhibitory hormonal signals, resulting in a chronic inflammatory state in the body [Citation50]. Moreover, several studies have supported that SES is biologically embedded by showing that low SES across the life course is associated with a blunted pattern of diurnal cortisol production [Citation51], higher level of allostatic load [Citation51], increased inflammatory activity [Citation52–54], and higher pathogen burden [Citation55,Citation56].
Our study has four central limitations. First, the sample size of the survivors of African ancestry was too small to identify ancestry-specific epigenetic associations with SDOH factors, but 20% (26/130) of the epigenome-wide significant CpGs among survivors of European ancestry were validated in the survivors of African ancestry. It is important to note that the observed difference of SDOH-associated CpGs across ancestral groups may be explained by different sociocultural/environmental experiences in addition to genetics. We performed the ancestry-stratified analysis, instead of a pooled analysis, because we found that the DNAm levels for many CpG sites significantly differed between survivors of African ancestry and those of European ancestry. Specifically, 54,125 CpGs were significantly associated with ancestry at the epigenome-wide significance level (P < 9 × 10−8) with a substantial genomic inflation factor (λ = 5.32). To supplement our ancestry-stratified analysis, we further conducted a meta-analysis to combine the summary statistics from two stratified results, by following the same analytic strategy used in a recently published multi ethnic EWAS [Citation57].
Second, our analysis was based on a cross-sectional study design, which does not allow assessment of a temporal sequence to establish the causality. Furthermore, we did not have data on the survivors’ childhood SDOH factors, such as their parents’ educational attainment and income and childhood home addresses to calculate the ADI, all of which potentially affect DNAm throughout their childhood and influence their current (adult) SES. Ideally, DNAm measured at two time points several years apart for the same set of survivors would enable a more rigorous assessment of the effect of baseline SDOH factors (or change of SDOH) on the changes of DNAm between the two time points.
Third, we found fewer CpGs that were significantly associated with income or ADI than were associated with educational attainment. This probably reflects the fact that educational attainment is a relatively stable SDOH factor, compared to income and ADI, which most likely change over the course of survivorship. Therefore, it may be difficult to capture their associations with DNAm in this cross-sectional analysis. In addition, we had significant missingness for the variables of household income and household size in our data set, so we chose personal income as the variable for SDOH modelling. It is important to note that personal income does not always reflect the poverty of the survivor (e.g., a survivor having high household income may have zero or low personal income because he/she does not work for financial, health, or other reasons), and hence the total number of significant epigenetic associations with personal income in our study appeared to be low and should be interpreted with caution.
Fourth, our evaluation of the functional implication of SDOH-associated CpGs was based on a small set of samples that included RNA-sequencing data. We tried to circumvent this limitation by identifying and comparing the eQTMs in the public database. Future expanded analysis is needed to confirm or refute the eQTM findings.
In summary, DNAm signatures, many resembling the effect of tobacco use, were associated with SDOH factors among survivors of childhood cancer, suggesting that biologically distal SDOH factors influence heath behaviours and modulate the human epigenome. Although disentangling mechanistic pathways remains challenging, future longitudinal studies with SDOH factors and DNAm measured at multiple time points may facilitate the causal inference.
Authorship contributions
Z.W. and I-C.H. designed and performed the research. N.S., J-A.S., Q.D., Z.L., Y.Z., L.H., C-W.H., H.P., C.L.W., K.K.N., K.R.K., D.K.S., Y.Y., M.M.H., L.L.R., I-C.H., and Z.W. collected, analyzed, and interpreted data. N.S., J-A.S., Q.D., Z.L. Y.Z., L.H., C-W.H., H.P., C.L.W., K.K.N., K.R.K., Y.Y., M.M.H., L.L.R., I-C.H., and Z.W. performed the statistical analyses. N.S., J-A.S., I-C.H., and Z.W. wrote the manuscript. M.M.H., L.L.R., I-C.H., and Z.W. contributed administrative, technical, or material support. Z.W. and I-C.H supervised all aspects of the study.
Statement of prior presentation
Part of this work was preprinted in medRxiv (10.1101/2020.10.30.20223313) and presented in abstract from at the AACR Annual Meeting 2021, which was virtual.
Supplemental Material
Download MS Word (1.4 MB)Acknowledgments
The authors acknowledge Dr. Angela McArthur, Department of Scientific Editing, St. Jude Children’s Research Hospital for her scientific editing.
Disclosure statement
The authors declare no potential conflicts of interest.
Supplementary Material
Supplemental data for this article can be accessed here.
Additional information
Funding
References
- Cunliffe VT. The epigenetic impacts of social stress: how does social adversity become biologically embedded? Epigenomics. 2016;8:1653–1669.
- Smyth GK. limma: linear models for microarray data. In: Gentleman R, Carey VJ, Huber W, et al., editors. Bioinformatics and computational biology solutions using R and bioconductor. New York: Springer New York; 2005. p. 397–420.
- Borghol N, Suderman M, McArdle W, et al. Associations with early-life socio-economic position in adult DNA methylation. Int J Epidemiol. 2012;41:62–74.
- Lam LL, Emberly E, Fraser HB, et al. Factors underlying variable DNA methylation in a human community cohort. Proc Natl Acad Sci U S A. 2012;109(Suppl 2):17253–17260.
- McGuinness D, McGlynn LM, Johnson PC, et al. Socio-economic status is associated with epigenetic differences in the pSoBid cohort. Int J Epidemiol. 2012;41:151–160.
- Stringhini S, Polidoro S, Sacerdote C, et al. Life-course socioeconomic status and DNA methylation of genes regulating inflammation. Int J Epidemiol. 2015;44:1320–1330.
- Needham BL, Smith JA, Zhao W, et al. Life course socioeconomic status and DNA methylation in genes related to stress reactivity and inflammation: the multi-ethnic study of atherosclerosis. Epigenetics. 2015;10:958–969.
- Karlsson Linnér R, Marioni RE, Rietveld CA, et al. An epigenome-wide association study meta-analysis of educational attainment. Mol Psychiatry. 2017;22:1680–1690.
- van Dongen J, Bonder MJ, Dekkers KF, et al. DNA methylation signatures of educational attainment. NPJ Sci Learn. 2018;3: 7.
- Joehanes R, Just AC, Marioni RE, et al. Epigenetic signatures of cigarette smoking. Circ Cardiovasc Genet. 2016;9:436–447.
- Wang X, Zhu H, Snieder H, et al. Obesity related methylation changes in DNA of peripheral blood leukocytes. BMC Med. 2010;8:87.
- Dugué PA, Wilson R, Lehne B, et al. Alcohol consumption is associated with widespread changes in blood DNA methylation: analysis of cross-sectional and longitudinal data. Addict Biol. 2021;26:e12855.
- Bell JT, Spector TD. DNA methylation studies using twins: what are they telling us? Genome Biol. 2012;13:172.
- Grundberg E, Meduri E, Sandling JK, et al. Global analysis of DNA methylation variation in adipose tissue from twins reveals links to disease-associated variants in distal regulatory elements. Am J Hum Genet. 2013;93:876–890.
- Wang J, Van Den Berg D, Hwang AE, et al. DNA methylation patterns of adult survivors of adolescent/young adult Hodgkin lymphoma compared to their unaffected monozygotic twin. Leuk Lymphoma. 2019;60:1429–1437.
- Song N, Hsu CW, Pan H, et al. Persistent variations of blood DNA methylation associated with treatment exposures and risk for cardiometabolic outcomes in long-term survivors of childhood cancer in the St. Jude Lifetime Cohort. Genome Med. 2021;13:53.
- Song N, Hsu C-W, Pan H, et al. Persistent variations of blood DNA methylation associated with treatment exposures and risk for cardiometabolic outcomes among long-term survivors of childhood cancer: a report from the St. Jude Lifetime Cohort. medRxiv 2020:2020.09.10.20192393.
- Reeves TJ, Mathis TJ, Bauer HE, et al. Racial and ethnic disparities in health outcomes among long-term survivors of childhood cancer: a scoping review. Front Public Health. 2021;9:741334.
- Li M, Zou D, Li Z, et al. EWAS Atlas: a curated knowledgebase of epigenome-wide association studies. Nucleic Acids Res. 2019;47:D983–d8.
- Howell CR, Bjornard KL, and Ness KK, et al. Cohort profile: The St. Jude Lifetime Cohort Study (SJLIFE) for paediatric cancer survivors. Int J Epidemiol. 2020;50(1):39–49.
- Howell CR, Bjornard KL, Ness KK, et al. Cohort profile: The St. Jude Lifetime Cohort Study (SJLIFE) for paediatric cancer survivors. Int J Epidemiol. 2021;50:39–49.
- Kind AJ, Jencks S, Brock J, et al. Neighborhood socioeconomic disadvantage and 30-day rehospitalization: a retrospective cohort study. Ann Intern Med. 2014;161:765–774.
- Kind AJH, Buckingham WR. Making neighborhood-disadvantage metrics accessible - the neighborhood Atlas. N Engl J Med. 2018;378:2456–2458.
- Stolley MR, Restrepo J, Sharp LK. Diet and physical activity in childhood cancer survivors: a review of the literature. Ann Behav Med. 2010;39:232–249.
- Cugnetto ML, Saab PG, Llabre MM, et al. Lifestyle factors, body mass index, and lipid profile in adolescents. J Pediatr Psychol. 2008;33:761–771.
- Qin N, Li Z, and Song N, et al. Epigenetic age acceleration and chronic health conditions among adult survivors of childhood cancer. J Natl Cancer Inst. 2020;113(5):597–605.
- Aryee MJ, Jaffe AE, Corrada-Bravo H, et al. Minfi: a flexible and comprehensive bioconductor package for the analysis of Infinium DNA methylation microarrays. Bioinformatics. 2014;30:1363–1369.
- Du P, Zhang X, Huang CC, et al. Comparison of Beta-value and M-value methods for quantifying methylation levels by microarray analysis. BMC Bioinformatics. 2010;11:587.
- Houseman EA, Accomando WP, Koestler DC, et al. DNA methylation arrays as surrogate measures of cell mixture distribution. BMC Bioinformatics. 2012;13:86.
- Jaffe AE, Irizarry RA. Accounting for cellular heterogeneity is critical in epigenome-wide association studies. Genome Biol. 2014;15:R31.
- Dobin A, Davis CA, Schlesinger F, et al. STAR: ultrafast universal RNA-seq aligner. Bioinformatics. 2013;29:15–21.
- Anders S, Pyl PT, Huber W. HTSeq–a Python framework to work with high-throughput sequencing data. Bioinformatics. 2015;31:166–169.
- Smyth GK. limma: linear models for microarray data. In: Gentleman R, Carey VJ, and Huber W, et al., editors. Bioinformatics and computational biology solutions using R and bioconductor. Statistics for biology and health. New York (NY): Springer; 2005:229–248. DOI:10.1007/0-387-29362-0_23
- Wang Z, Wilson CL, Easton J, et al. Genetic risk for subsequent neoplasms among long-term survivors of childhood cancer. J Clin Oncol. 2018;36:2078–2087.
- Schisterman EF, Cole SR, Platt RW. Overadjustment bias and unnecessary adjustment in epidemiologic studies. Epidemiology. 2009;20:488–495.
- Higgins J, Thomas J, and Chandler J, et al. Cochrane handbook for systematic reviews of interventions version 6.2 (updated February 2021). United Kingdom: Cochrane; 2021.
- Barfield RT, Kilaru V, Smith AK, et al. CpGassoc: an R function for analysis of DNA methylation microarray data. Bioinformatics. 2012;28:1280–1281.
- Mansell G, Gorrie-Stone TJ, Bao Y, et al. Guidance for DNA methylation studies: statistical insights from the Illumina EPIC array. BMC Genomics. 2019;20:366.
- Krzywinski M, Schein J, Birol I, et al. Circos: an information aesthetic for comparative genomics. Genome Res. 2009;19:1639–1645.
- Xu Z, Xie C, Taylor JA, et al. ipDMR: identification of differentially methylated regions with interval P-values. Bioinformatics. 2021;37:711–713.
- Team. RDC. R: a language and environment for statistical computing. Vienna: R Foundation for Statistical Computing; 2010.
- Kõks S, Kõks G. Activation of GPR15 and its involvement in the biological effects of smoking. Exp Biol Med (Maywood). 2017;242:1207–1212.
- Harlid S, Xu Z, Panduri V, et al. CpG sites associated with cigarette smoking: analysis of epigenome-wide data from the Sister Study. Environ Health Perspect. 2014;122:673–678.
- Maas SCE, Mens MMJ, Kühnel B, et al. Smoking-related changes in DNA methylation and gene expression are associated with cardio-metabolic traits. Clin Epigenetics. 2020;12:157.
- Krieger N. Embodiment: a conceptual glossary for epidemiology. J Epidemiol Community Health. 2005;59:350–355.
- Short SE, Mollborn S. Social determinants and health behaviors: conceptual frames and empirical advances. Curr Opin Psychol. 2015;5:78–84.
- Hertzman C. Commentary on the symposium: biological embedding, life course development, and the emergence of a new science. Annu Rev Public Health. 2013;34:1–5.
- Kuratomi G, Saito A, Ozeki Y, et al. Association of the Hermansky-Pudlak syndrome type 4 (HPS4) gene variants with cognitive function in patients with schizophrenia and healthy subjects. BMC Psychiatry. 2013;13:276.
- Marzi SJ, Sugden K, Arseneault L, et al. Analysis of DNA methylation in young people: limited evidence for an association between victimization stress and epigenetic variation in blood. Am J Psychiatry. 2018;175:517–529.
- Miller GE, Chen E, Parker KJ. Psychological stress in childhood and susceptibility to the chronic diseases of aging: moving toward a model of behavioral and biological mechanisms. Psychol Bull. 2011;137:959–997.
- Dowd JB, Simanek AM, Aiello AE. Socio-economic status, cortisol and allostatic load: a review of the literature. Int J Epidemiol. 2009;38:1297–1309.
- Ranjit N, Diez-Roux AV, Shea S, et al. Socioeconomic position, race/ethnicity, and inflammation in the multi-ethnic study of atherosclerosis. Circulation. 2007;116:2383–2390.
- Loucks EB, Pilote L, Lynch JW, et al. Life course socioeconomic position is associated with inflammatory markers: the Framingham offspring study. Soc Sci Med. 2010;71:187–195.
- Carroll JE, Cohen S, Marsland AL. Early childhood socioeconomic status is associated with circulating interleukin-6 among mid-life adults. Brain Behav Immun. 2011;25:1468–1474.
- Steptoe A, Shamaei-Tousi A, Gylfe A, et al. Socioeconomic status, pathogen burden and cardiovascular disease risk. Heart. 2007;93:1567–1570.
- Dowd JB, Zajacova A, Aiello A. Early origins of health disparities: burden of infection, health, and socioeconomic status in U.S. children. Soc Sci Med. 2009;68:699–707.
- Jhun MA, Mendelson M, Wilson R, et al. A multi-ethnic epigenome-wide association study of leukocyte DNA methylation and blood lipids. Nat Commun. 2021;12:3987.