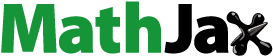
ABSTRACT
Chronic musculoskeletal pain is a health burden that may accelerate the aging process. Accelerated brain aging and epigenetic aging have separately been observed in those with chronic pain. However, it is unknown whether these biological markers of aging are associated with each other in those with chronic pain. We aimed to explore the association of epigenetic aging and brain aging in middle-to-older age individuals with varying degrees of knee pain. Participants (57.91 ± 8.04 y) with low impact knee pain (n = 95), high impact knee pain (n = 53), and pain-free controls (n = 26) completed self-reported pain, a blood draw, and an MRI scan. We used an epigenetic clock previously associated with knee pain (DNAmGrimAge), the subsequent difference of predicted epigenetic and brain age from chronological age (DNAmGrimAge-Difference and Brain-PAD, respectively). There was a significant main effect for pain impact group (F (2,167) = 3.847, P = 0.023, = 0.038, ANCOVA) on Brain-PAD and DNAmGrimAge-difference (F (2,167) = 6.800, P = 0.001,
= 0.075, ANCOVA) after controlling for covariates. DNAmGrimAge-Difference and Brain-PAD were modestly correlated (r =0.198; P =0.010). Exploratory analysis revealed that DNAmGrimAge-difference mediated GCPS pain impact, GCPS pain severity, and pain-related disability scores on Brain-PAD. Based upon the current study findings, we suggest that pain could be a driver for accelerated brain aging via epigenome interactions.
Introduction
Chronic pain is commonly reported with aging and often impacts physical mobility [Citation1,Citation2] and cognitive function [Citation3,Citation4]. The relationship between chronological age as a risk factor for many chronic pain states is well documented [Citation5–8]. However, chronic pain is heterogeneous with advancing age – welcoming the possibility that other biological and environmental ‘aging’ processes may be playing key roles in influencing this variation. There is evidence to support that individuals with chronic pain demonstrate premature aging of brain structures (grey matter atrophy [Citation9–11]) and systemic changes (increased markers of inflammation and decreased blood leukocyte telomere length [Citation12–14]). These central and peripheral mechanistic changes suggest that multiple physiological systems may contribute to accelerated biological aging process and increased mortality risk in those with chronic pain.
We have previously observed that chronic pain is associated with ‘age-like’ brain atrophy in otherwise healthy, community-dwelling older individuals [Citation11]. Brain volume has been shown to decrease as much as 11% in those who have chronic pain – equivalent to the amount of grey matter that is lost in 10–20 y of normal aging [Citation15]. Decreased volume in the prefrontal cortex and the thalamus of the brain was related to the duration of time spent in pain. Additionally, when calculating volumetric brain measures (brain grey and white matter, and CSF), a brain-aging biomarker was identified where an individual’s brain appears structurally younger or older than the average structure of those of the same chronological age by using a machine learning model [Citation16,Citation17]. Impressively, this model can detect deviations from healthy brain aging and has identified accelerated aging in Alzheimer’s disease [Citation18], mild cognitive impairment [Citation19], HIV [Citation20], and schizophrenia [Citation21]. Using this process to calculate brain age, brain-predicted age differences (Brain-PAD; the difference between chronological age and brain-predicted age) have been found in older adults with chronic musculoskeletal pain whereby the brain appears ‘older’ in those who have greater pain impact [Citation11,Citation22]. Multiple brain structures have been recognized as regions associated with the perception of pain such as the primary somatosensory cortex, secondary somatosensory cortex, anterior cingulate cortex (ACC), prefrontal cortex (PFC), insular cortex, amygdala, thalamus, cerebellum, and periaqueductal grey (PAG). Volumetric changes in any or all of these structures could affect the pain experience; however, chronic pain may play a role in brain remodelling.
In addition to brain changes, epigenetic modifications are a hallmark of aging as certain epigenetic factors mediate, in part, the relationship between the genome and environment which, over a lifetime, can lead to biological changes within the human body. Epigenetic clocks based on DNA methylation data are among the most promising biomarkers of aging to date. One epigenetic clock of interest, DNAmGrimAge, has been used as a biomarker of mortality [Citation23]. DNAmGrimAge is a linear combination of chronological age, sex, and DNAm-based surrogate biomarkers for several plasma proteins and smoking pack-years, and has stronger relationships with a variety of health-related metrics compared to other DNAm-based biomarkers [Citation23]. Many of these surrogates have been associated with pain development and maintenance [Citation24], and thus, DNAmGrimAge may be the most useful when establishing relationships between gene expression and chronic pain.
Historically, the pain–age relationship has been presented as unidirectional with increasing age associated with a higher risk of developing chronic pain, yet more recent evidence supports bidirectional associations, as chronic pain may also accelerate the biological aging process [Citation11,Citation16,Citation23,Citation25,Citation26]. However, the potential underlying neurobiological mechanisms whereby pain impacts brain aging processes are not currently known in those who experience chronic pain. It is plausible that environmental and lifestyle factors modify the epigenome (i.e., accelerated epigenetic aging), which in turn leads to accelerated brain aging and cognitive decline [Citation25]. The present study aimed to explore the association of epigenetic aging and brain aging in middle-to-older age individuals with varying degrees of knee pain, and to examine whether epigenetic aging mediates the pain-brain aging association. Based on the existing literature, we hypothesized that epigenetic aging significantly mediates the association between pain and brain aging in persons with knee pain.
Methods
Participants
Participants aged between 45 and 85 y with or without knee pain were recruited from University of Florida (UF; Gainesville, Florida, USA) and the University of Alabama at Birmingham (UAB; Birmingham, Alabama, USA). These individuals self-identified as non-Hispanic and ‘African American/Black’ or ‘White/Caucasian/European’ and were English speaking. If participants reported any of the following criteria, they were excluded from the study: (1) significant surgery to the index (i.e., most painful) knee (e.g., total knee replacement surgery); (2) cardiovascular disease or history of acute myocardial infarction; (3) uncontrolled hypertension (blood pressure >150/95 mmHg); (4) systemic rheumatic diseases (e.g., rheumatoid arthritis, systemic lupus erythematosus, and fibromyalgia); (5) neuropathy; (6) chronic opioid use; (7) serious psychiatric illness; (8) neurological disease (e.g., Parkinson’s, multiple sclerosis, stroke with loss of sensory or motor function, or uncontrolled seizures); (9) pregnant; (10) significantly greater pain in a body site other than the knee. All participants provided written informed consent prior to data collection procedures. The study was IRB approved and was completed in accordance with the Declaration of Helsinki. Our participants were recruited as part of a parent study aimed to examine race group differences in physical symptoms, psychosocial functioning, and pain-related central nervous system structure and functioning in those with or at risk of knee osteoarthritis (OA).
Procedures
We have previously reported on various aspects of our study [Citation27–29] where data were collected at the University of Florida and University of Alabama at Birmingham. Both study sites’ IRBs approved the study. Briefly, potential participants were first screened over the phone to determine study eligibility for sociodemographic (e.g., age, sex, race) and health information (e.g., knee pain symptoms). Those potentially eligible were scheduled for a Health Assessment Session (HAS) to obtain informed consent, a health and pain history (including smoking history and self-reported pain), and a physical exam to determine the most painful (i.e., index) knee. Self-reported pain measures were reported within 24 h of an unfasted blood draw, which was scheduled approximately 1 week following the initial visit (i.e., HAS).
Self-reported pain
The Graded Chronic Pain Scale (GCPS) was used to determined self-reported pain and provides characteristic pain intensity (items 1–3), and pain interference (items 4–6) subscales during the past 6 months [Citation30]. Scores range from 0 to 100 where higher scores indicate more severe pain intensity and interference. Using the self-reported GCPS measure [Citation30] and based on recent work [Citation31,Citation32], individuals were classified into three groups reflective of the impact chronic pain had on their life: high impact pain, low impact pain, and a no pain control group. This method is consistent with previous research [Citation32] and uses the recommendations from the Task Force for the Classification of Chronic Pain consensus for the 11th version of the International Classification of Diseases (ICD-11) of the World Health Organization (WHO) [Citation33].
Neuroimaging
MRI data were collected using 3-T Phillips scanners at the University of Florida (32-channel radio-frequency coil) and the University of Alabama at Birmingham (8-channel head coil) minimizing head movement via cushions positioned inside the head coil and instructions to participants. A high resolution, T1-weighted turbo field echo anatomical scan was collected using the following parameters: TR = 7.0 ms, TE = 3.2 ms, 176 slices acquired in a sagittal orientation, flip angle = 8 degrees, resolution = 1 mm3.
Brain-predicted age biomarker
The brain aging biomarker used here was derived as previously described [Citation16,Citation34]. This involved training a machine-learning model derived from neuroimaging data (training cohort = 3,377 healthy individuals, mean age = 40.6 ± 21.4 y, age range = 18–92 y). This used segmented and spatially normalized T1-weighted MRI scans as the predictor variables in a Gaussian Processes regression, with chronological age as the outcome variable.Footnote1 Model accuracy based on the held-out test data (using random assignment to training and test) was high, with a mean absolute error of 3.93 y and a correlation between chronological age and ‘brain-predicted’ age of r = 0.97, R2 = 0.95 Then, using the regression model trained on the full independent dataset (n = 3,377), brain-predicted age values were generated for the n = 202 participants in the current study. Consistent with our previous work, the individual participants’ chronological age was then subtracted from this brain-predicted age value to generate a brain-predicted age difference (Brain-PAD) score, which was used for further analysis [Citation11]. Neuroimaging data comprising the training dataset were obtained via publicly available repositories and were screened according to local study protocols to ensure that they were free of neurological and psychiatric disorders, had no history of head trauma and other major medical conditions [Citation17]. Ethical approval for each initial study and subsequent data sharing was verified for each data repository.
Blood collection and processing
A fasted blood sample was collected from each participant during the quantitative sensory testing session and included collection of a 10-ml K2 EDTA tube that was subsequently used for DNA extraction and methylation analysis. The EDTA tube was centrifuged at 3000 rpm for 10 min and the buffy coat was carefully extracted and transferred to a cryovial for −80-degree storage. To isolate genomic DNA, the frozen buffy coat samples were thawed at 37°C to dissolve homogeneously. Approximately 200 µl (or 150–200 µl) of sample was lysed in R.B.C lysis buffer and centrifuged at 6000 rpm for 5 min at room temperature. The supernatant was discarded and sodium EDTA solution was added to the pellet and vortex gently to remove RBC clumps. Homogenate was incubated at 50–55°C with Proteinase K and SDS solution. Following incubation, equal volume of phenol was added, mixed, and centrifuged at 10,000 rpm for 10 min. Supernatant was transferred in a fresh tube, and equal volume of phenol–chloroform–isoamyl alcohol was added, mixed, and centrifuged at the same rpm. Again, supernatant was transferred in a fresh tube and equal volume of chloroform-isoamyl alcohol was added followed by centrifugation at the same rpm conditions. Supernatant was transferred in a fresh tube and 1/10th volume of 3 M sodium acetate along with 2 volumes of absolute alcohol was added. The precipitated DNA was washed with 70% ethanol by centrifugation at 10,000 rpm for 5 min. The pellet was air dried and dissolved in Tris-EDTA buffer. The dissolved DNA was qubit quantified and visualized on agarose gel for quality assessment. Sodium Bisulfite conversion (Zymo EZ DNA Methylation Kit) and EPIC methylation array were performed by Moffitt Cancer Center, Molecular Genomics Core located at 3011 Holly Dr. Tampa, FL 33612.
DNA methylation age calculation for grimage
The raw data generated by Illumina EPIC array (.idat files) were processed using R package minfi [Citation35]. Methylation beta values (percentage of methylation for each CpG site) were obtained and uploaded to the DNA Methylation Age online calculator (https://dnamage.genetics.ucla.edu/home) [Citation36]. As recommended by the calculator tutorial, we checked normalize data and the advanced analysis options to obtain the epigenetic ages. We used a previously verified method of using buffy coat methylation profile-type proportions as opposed to blood cell proportions for the calculations [Citation37,Citation38]. The variable used in the analyses, DNAmGrimAge-difference, was calculated by subtracting each individual’s chronological age from their DNAmGrimAge.
Statistical analysis
All data processing and analyses were conducted in SPSS v27.0 (Armonk, NY: IBM Corp) and data were checked for distributional form and outliers. Characteristics of the sample were collected using one-way analysis of variance (ANOVA) to compare mean values in continuous/discrete ordinal variables between pain impact groups, and χ2 was used for comparison of nominal variables. Assumptions underlying each statistical test were examined. Brain-PAD and DNAmGrimAge-difference between pain impact groups were examined using one-way analyses of covariance (ANCOVAs), using sex, ethnicity/race, chronological age, and study site as covariates. Partial correlations were used to examine associations between Brain-PAD and DNAmGrimAge-difference while controlling for blood cell proportions (CD4T, CD8T, B cells, granulocytes, and monocytes), sex, ethnicity/race, chronological age, and study site. Using the Hayes PROCESS macro (i.e., Model 4), a mediation analyses was used to assess pain impact and its association with Brain-PAD with DNAmGrimAge-difference as the mediator using direct and total indirect effects. The mediation model controlled for sex, race, age, study site and blood cell proportions. To overcome potential unmet assumptions commonly found in mediation analysis, bootstrapping procedures were employed for all analyses with 5,000 samples and reported as estimates (b) and standard errors (SE) or as 95% bootstrapped confidence intervals.
Results
Of the 213 participants who took part in the epigenetic sub-study that examined pain impact and epigenetic aging, 174 participants had complete pain, brain, and covariate data. The analytic sample age was 57.91 ± 8.04 y, with 62.7% female and 55.4% Non-Hispanic white. Self-reported pain and disability measures differed significantly across pain impact groups as expected (Ps <0.001). Pain impact group differences are summarized in .
Table 1. Participant characteristics with and without knee pain (n = 174).
Differences in biological aging between pain impact groups
Data demonstrating Brain-PAD differences between pain impact groups while controlling for sex, race, chronological age, and study site has been previously reported [Citation39]. In the present study sub-sample, there was a significant main effect for pain group (F (2,167) = 3.847, P = 0.023, 0.038, ANCOVA) on Brain-PAD after controlling for sex, race, chronological age, and study site. Post hoc tests revealed a significantly older Brain-PAD in individuals with high impact pain (−0.89 ± 6.24) compared to those with low impact pain (−4.13 ± 7.63; P = 0.019). Similar to our previous findings [Citation40] a significant main effect for pain impact group was found in DNAmGrimAge-difference (F (2,167) = 6.800, P = 0.001,
= 0.075, ANCOVA) after accounting for the same covariates. Post-hoc tests revealed that those with high impact pain had a significantly older epigenetic age (5.14 ± 5.66) than those who had low impact pain (1.32 ± 5.41; P = 0.007) or no pain (0.09 ± 3.31; P = 0.004).
Associations between brain aging and epigenetic aging
Partial correlations revealed that DNAmGrimAge-difference was modestly correlated with Brain-PAD (r =0.198; P =0.010) when blood cell proportions, site, age, sex, and race were controlled for ().
Epigenetic aging as a mediator of pain and brain aging associations
To test if DNAmGrimAge-difference mediates the association between pain and Brain-PAD, bootstrapped mediation analyses (n =5,000) were performed while controlling for blood cell type sex, age, race, and study site. DNAmGrimAge-difference mediated the association of pain impact with Brain-PAD. Results are summarized in . DNAmGrimAge-difference also mediated the associations of GCPS pain severity and pain-related disability and WOMAC pain and physical function scores with Brain-PAD (). Furthermore, when we added smoking history as a covariate, the mediation pathway remained significant and did not change our findings.
Figure 2. Mediation analysis of pain group, DNAmGrimAge-difference and brain-PAD. Bias corrected bootstrapped estimates and confidence intervals.
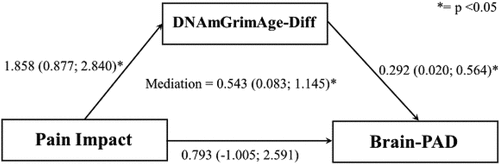
Table 2. Bias corrected bootstrapped estimates and confidence intervals for direct and mediated effects of pain severity and disability GCPS scores on brain-PAD adjusting for covariates.
Discussion
Chronic pain has been linked to multiple accelerated biological aging processes and advancements in aging research have led to the identification of potential biological age predictors. The present study demonstrated an association between two biological aging biomarkers: brain age and epigenetic age. The associations between aging and pain have been well-documented in the literature [Citation5–8]. Traditionally, this relationship has been assumed to be driven by chronological aging: that is, the longer one has spent causing physical ‘wear and tear’ on the body or exposure to environmental factors over time, the greater the degree of severity of pain [Citation41]. However, this relationship could be a part of a bidirectional feedback loop, whereby experiencing pain could drive physiological changes that contribute to and potentially accelerate the biological aging process, particularly in the brain. As the brain ages, there are significant changes in the grey and white matter throughout [Citation42], including but not limited to dysfunction of its cellular and molecular constituents [Citation22] (e.g., dendritic regression in neurons, synaptic atrophy, receptor changes, and reactive astrocytes and microglia). We have previously shown that older individuals (>65 y) with chronic musculoskeletal pain have less geometric complexity in neurite density and architecture across various white matter projection (i.e., corticospinal, anterior corona radiata, posterior thalamic radiation), association (i.e., uncinate fasciculus) and commissural fibres (i.e., corpus callosum) as well as fibres in the cerebellum (i.e., superior cerebellar peduncle) and the limbic system (i.e., cingulum, hippocampus, fornix); and that these differences were related to the pain experience [Citation43]. Pain has also been associated with volumetric grey matter changes in areas such as the prefrontal cortex, primary somatosensory cortex, insula, and inferior temporal gyrus, all of which are critically involved in the conception of the pain experience [Citation44]. Structural and functional changes across the aging brain, such as these, have been used to develop machine learning predictions (as used in this study), and can help us begin to understand how pain itself could be associated with age-related processes and accelerate biological age. How chronic pain is potentially driving these age-like brain changes, however, remains to be elucidated.
Chronological aging is associated with the decline of many functional and cognitive processes, however, not everyone appears to ‘biologically’ age at the same rate. Many environmental and biological factors, such as exposure to toxic chemicals, psychological stress, and poor diet quality have all been associated with age-related changes throughout the body through epigenetic modifications [Citation45]. Epigenetic research has provided insight as to how these factors go on to cause actual physical and functional changes within the system. During the aging process, epigenetic changes result in an altered accessibility to genetic and subsequent structural materials, abnormal gene expression, reactivation of transposable elements, and genomic instability. These epigenetic age-related changes in the brain [Citation46] may ultimately lead to volumetric loss in grey matter and white matter structures, with the largest changes seen in the frontal and temporal cortices, putamen, thalamus, and accumbens [Citation47], andmaladaptive metabolic states within the brain (compromised bioenergetics, impaired neuroplasticity, abnormal neuronal network activity and homoeostasis, oxidative stress, and inflammation) [Citation42]. Both of these phenomena can potentially create negative age-related outcomes such as cognitive and physical decline, and have been implicated in neurodegenerative diseases such as Alzheimer’s and Parkinson’s [Citation46]. In the aging prefrontal cortex, for example, loss of histone acetylation at proximal promotor regions of genes important for inhibitory neurotransmission (GABAergic), serotonergic signalling, and mitochondrial functions leads to abnormal functioning [Citation48]. Thus, it is plausible that epigenetic modifications that hasten cellular aging could also accelerate processes associated with traditional phenotypic aging.
In the current study, DNAmGrimAge was used to examine the association between epigenetic aging, pain, and brain aging. DNAmGrimAge is composed of DNA surrogates related to smoking-years, growth factors, and immune system function, all of which have various associations with chronological age [Citation23] as well as pain development and maintenance [Citation24]. The epigenetic modifications in the surrogates that make up DNAmGrimAge can lead to accelerated cellular aging that produces biochemical pathways, cells, and eventually systems to look and act as if they were much older than their average chronological counterparts. These age-related changes found in the brain in chronologically younger individuals could potentially lead to cognitive and physical decline at earlier stages of life.
We propose that pain itself could be a symptom of an aging body and may also accelerate aging in the brain via epigenetic modifications. Pain is a complex phenomenon that involves many physiological systems, including the nervous and immune systems. Neuronal dysfunction, sensitization, and chronic immune system activation resulting in inflammation are hallmarks of the pain experience. Immune system function can impact pain through the release of autoantibodies, cytokines, chemokines, and other inflammatory mediators (i.e., substance P, histamine, bradykinin, tumour necrosis factor, interleukins, and prostaglandins) [Citation24]. The human body is highly adaptable, so it is possible that immune-mediated changes in internal environment led to altered nociception, abnormal cellular signalling, and inflammation potentially causing epigenetic alterations in gene expression, since these processes involve many of the DNA surrogates used to calculate DNAmGrimAge (i.e., C-reactive protein). These changes may cause structural alterations, thereby contributing to an older appearing brain. Previous research has primarily examined spinal cord alterations relating to epigenetic changes in areas associated with pain processing and brain network changes [Citation49]. To our knowledge, however, this is the first work documenting high impact pain-associated epigenetic aging, related to morphological brain abnormalities compared to those with no pain or those with low impact pain. Therefore, additional experiments that examine epigenetic aging via epigenetic clocks at even older ages are needed, especially if the trajectory of biological age may not be linear in advanced age. The findings from this study may expedite the future use and refinement of larger scale epigenetic and brain imaging approaches for predicting clinically defined outcomes and subsequent individual risk predictions in those with chronic pain conditions.
Our study has several limitations worth considering. First, this is a cross-sectional study; thus, causality nor the temporal order of the associations cannot be directly established. Second, both the brain aging and the epigenetic aging biomarkers provide global information relative to chronological aging (i.e., older brain or epigenome compared to chronological age), thus specific information regarding where each individual may have an older brain or epigenome is not assessed. Future larger longitudinal studies can provide additional insights into these important associations. Our findings are mostly driven through statistical analysis rather than a mechanistic study designed to elucidate such causes, as such; experimental approaches examining the role of epigenetic aging in chronic pain conditions using experimental rodent models may be beneficial to understand the underlying mechanism driving this association. Our data focused on the potential role of chronic pain in biological aging in epigenetics and brain changes in middle-to-older age individuals and did not examine these changes in younger adults. Future research across the lifespan is needed to assess critical time points where accelerated aging may be most prevalent, and identifying these critical ages where possible interventions may be beneficial to prevent accelerated biological aging.
Despite these limitations, the current study findings implicate pain as a driver of accelerated brain aging via epigenome interactions. Given the high prevalence of pain in older adults, it is imperative that future research continues to investigate the bidirectional association of pain and aging. By doing so, we can begin to not only alleviate the physical and emotional suffering that many individuals with chronic pain face but also try to prevent accelerated brain aging that could be contributing to ailments that may be occurring at younger chronological ages compared to those that do not experience chronic pain.
Author contributions
J. Peterson and L. Strath contributed to the writing of the manuscript equally and share first authorship. J. Peterson and Y. Cruz-Almeida conceptualized the research question and J. Peterson drafted the introduction, computed the statistical analysis, and drafted the results. J. Peterson, L. Strath and Y. Cruz-Almeida interpreted the data and critically discussed findings. C. Laffitte-Nodarse performed and interpreted brain aging calculations from the MRI scans and reviewed the manuscript. A. Rani and S. Yoda were actively involved in obtaining and deriving the epigenetic data and reviewed the manuscript. L. Meng and Z. Huo performed epigenetic aging calculations, their interpretations and reviewed the manuscript. J. Cole, T.C. Foster, R.B. Fillingim edited and reviewed the manuscript for critical feedback. Y. Cruz-Almeida obtained funding, guided the papers’ progress, edited and reviewed the manuscript for critical feedback. All authors contributed to the paper and approved paper for submission for peer review.
Acknowledgements
The authors would like to thank the research team and the participants for their time to help complete this study.
Disclosure statement
No potential conflict of interest was reported by the author(s).
Data availability statement
The data that support the findings of this study are available from the corresponding author upon reasonable request.
Additional information
Funding
Notes
References
- Dudgeon BJ, Gerrard BC, Jensen MP, et al. Physical disability and the experience of chronic pain. Arch Phys Med Rehabil. 2002;83(2):229–235.
- Hairi NN, Cumming RG, Blyth FM, et al. Chronic pain, impact of pain and pain severity with physical disability in older people—Is there a gender difference? Maturitas. 2013;74(1):68–73.
- Moriarty O, McGuire BE, Finn DP. The effect of pain on cognitive function: a review of clinical and preclinical research. Prog Neurobiol. 2011;93(3):385–404.
- McGuire BE. Chronic pain and cognitive function. Pain. 2013;154(7):964–965.
- Patel K, Dansie E, Guralnik J, et al. Prevalence and impact of pain among older adults in the United States: findings from the national health and aging trends study. J Pain. 2013;14(4):S12.
- Karttunen NM, Turunen JH, Ahonen RS, et al. Persistence of noncancer-related musculoskeletal chronic pain among community-dwelling older people: a population-based longitudinal study in Finland. Clin J Pain. 2015;31(1):79–85.
- Elliott AM, Smith BH, Penny KI, et al. The epidemiology of chronic pain in the community. Lancet. 1999;354(9186):1248–1252.
- Smith BH, Elliott AM, Chambers WA, et al. The impact of chronic pain in the community. Fam Pract. 2001;18(3):292–299.
- Rodriguez-Raecke R, Niemeier A, Ihle K, et al. Brain gray matter decrease in chronic pain is the consequence and not the cause of pain. J Neurosci. 2009;29(44):13746–13750.
- Moayedi M, Weissman-Fogel I, Salomons TV, et al. Abnormal gray matter aging in chronic pain patients. Brain Res. 2012;1456:82–93.
- Cruz-Almeida Y, Fillingim RB, Riley JL 3rd, et al. Chronic pain is associated with a brain aging biomarker in community-dwelling older adults. Pain. 2019;160(5):1119–1130.
- Zhang J-M, An J. Cytokines, inflammation and pain. Int Anesthesiol Clin. 2007;45(2):27.
- Hassett AL, Epel E, Clauw DJ, et al. Pain is associated with short leukocyte telomere length in women with fibromyalgia. J Pain. 2012;13(10):959–969.
- Sibille KT, Chen H, Bartley EJ, et al. Accelerated aging in adults with knee osteoarthritis pain: consideration for frequency, intensity, time, and total pain sites. Pain Rep. 2017;2(3). DOI:10.1097/PR9.0000000000000591.
- Apkarian AV, Sosa Y, Sonty S. Chronic back pain is associated with decreased prefrontal and thalamic gray matter density. J Neurosci. 2004;24(46):10410–10415.
- Cole JH, Franke K. Predicting age using neuroimaging: innovative brain ageing biomarkers. Trends Neurosci. 2017;40(12):681–690.
- Cole JH, Ritchie SJ, Bastin ME, et al. Brain age predicts mortality. Mol Psychiatry. 2018;23(5):1385–1392.
- Habes M, Janowitz D, Erus G, et al. Advanced brain aging: relationship with epidemiologic and genetic risk factors, and overlap with Alzheimer disease atrophy patterns. Transl Psychiatry. 2016;6(4):e775.
- Franke K, Gaser C. Longitudinal changes in individual brainage in healthy aging, mild cognitive impairment, and Alzheimer’s disease. GeroPsych. 2012;25(4):235
- Cole JH, Underwood J, Caan MW, et al. Increased brain-predicted aging in treated HIV disease. Neurology. 2017;88(14):1349–1357.
- Nenadic I, Dietzek M, Langbein K, et al. BrainAGE score indicates accelerated brain aging in schizophrenia, but not bipolar disorder. Psychiatry Res Neuroimaging. 2017;266:86–89.
- Cruz-Almeida Y, Cole J. Pain, aging, and the brain: new pieces to a complex puzzle. Pain. 2020;161(3):461–463.
- Lu AT, Quach A, Wilson JG, et al. DNA methylation GrimAge strongly predicts lifespan and healthspan. Aging (Albany NY). 2019;11(2):303–327.
- Totsch SK, Sorge RE. Immune system involvement in specific pain conditions. Mol Pain. 2017;13:1744806917724559.
- Barter JD, Foster TC. Aging in the brain: new roles of epigenetics in cognitive decline. Neuroscientist. 2018;24(5):516–525.
- Peterson JA, Meng L, Rani A, et al. Epigenetic aging, knee pain and physical performance in community-dwelling middle-to-older age adults. Exp Gerontol. 2022;166:111861.
- Terry EL, Booker SQ, Cardoso JS, et al. Neuropathic-like pain symptoms in a community-dwelling sample with or at risk for knee osteoarthritis. Pain Med. 2020;21(1):125–137.
- Terry EL, Fullwood MD, Booker SQ, et al. Everyday discrimination in adults with knee pain: the role of perceived stress and pain catastrophizing. J Pain Res. 2020;13:883.
- Terry EL, Tanner JJ, Cardoso JS, et al. Associations of pain catastrophizing with pain-related brain structure in individuals with or at risk for knee osteoarthritis: sociodemographic considerations. Brain Imaging Behav. 2021;15(4):1769–1777.
- Von Korff M, Ormel J, Keefe FJ, et al. Grading the severity of chronic pain. Pain. 1992;50(2):133–149.
- Dahlhamer J, Lucas J, Zelaya C, et al. Prevalence of chronic pain and high-impact chronic pain among adults—United States, 2016. Morbidity Mortality Weekly Rep. 2018;67(36):1001.
- Pitcher MH, Von Korff M, Bushnell MC, et al. Prevalence and profile of high-impact chronic pain in the United States. J Pain. 2019;20(2):146–160.
- Nugraha B, Gutenbrunner C, Barke A, et al. The IASP classification of chronic pain for ICD-11: functioning properties of chronic pain. Pain. 2019;160(1):88–94.
- Clausen AN, Fercho KA, Monsour M, et al. Assessment of brain age in posttraumatic stress disorder: findings from the ENIGMA PTSD and brain age working groups. Brain Behav. 2021;12:e2413.
- Aryee MJ, Jaffe AE, Corrada-Bravo H, et al. Minfi: a flexible and comprehensive Bioconductor package for the analysis of Infinium DNA methylation microarrays. Bioinformatics. 2014;30(10):1363–1369.
- Horvath S. DNA methylation age of human tissues and cell types. Genome Biol. 2013;14(10):3156.
- Dou J, Schmidt RJ, Benke KS, et al. Cord blood buffy coat DNA methylation is comparable to whole cord blood methylation. Epigenetics. 2018;13(1):108–116.
- Ghamrawi R, Velickovic I, Milicevic O, et al. Buffy coat DNA methylation profile is representative of methylation patterns in white blood cell types in normal pregnancy. Front Bioeng Biotechnol. 2022;9:782843.
- Johnson A, Buchanan T, Laffitte C, et al. Added “Age-like” brain patterns with increasing pain impact in persons with knee osteoarthritis pain: considerations by race. J Pain. 2022;23(5):42.
- Cruz-Almeida Y, Sinha P, Rani A, et al. Epigenetic aging is associated with clinical and experimental pain in community-dwelling older adults. Mol Pain. 2019;15:1744806919871819.
- Wettstein M, Eich W, Bieber C, et al. Pain intensity, disability, and quality of life in patients with chronic low back pain: does age matter? Pain Med. 2019;20(3):464–475.
- Mattson MP, Arumugam TV. Hallmarks of brain aging: adaptive and pathological modification by metabolic states. Cell Metab. 2018;27(6):1176–1199.
- Cruz-Almeida Y, Coombes S, Febo M. Pain differences in neurite orientation dispersion and density imaging measures among community-dwelling older adults. Exp Gerontol. 2021;154:111520.
- Yang S, Chang MC. Chronic pain: structural and functional changes in brain structures and associated negative affective states. Int J Mol Sci. 2019;20(13):3130.
- Cavalli G, Heard E. Advances in epigenetics link genetics to the environment and disease. Nature. 2019;571(7766):489–499.
- Akbarian S, Beeri MS, Haroutunian V. Epigenetic determinants of healthy and diseased brain aging and cognition. JAMA Neurol. 2013;70(6):711–718.
- Fjell AM, Walhovd KB. Structural brain changes in aging: courses, causes and cognitive consequences. Rev Neurosci. 2010;21(3):187–221.
- Tang B, Dean B, Thomas EA. Disease- and age-related changes in histone acetylation at gene promoters in psychiatric disorders. Transl Psychiatry. 2011;1(12):e64–e.
- Descalzi G, Ikegami D, Ushijima T, et al. Epigenetic mechanisms of chronic pain. Trends Neurosci. 2015;38(4):237–246.