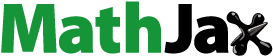
ABSTRACT
Sufficient evidence supports a relationship between certain myeloid neoplasms and exposure to benzene or formaldehyde. DNA methylation could underlie benzene- and formaldehyde-induced health outcomes, but data in exposed human populations are limited. We conducted two cross-sectional epigenome-wide association studies (EWAS), one in workers exposed to benzene and another in workers exposed to formaldehyde. Using HumanMethylation450 BeadChips, we investigated differences in blood cell DNA methylation among 50 benzene-exposed subjects and 48 controls, and among 31 formaldehyde-exposed subjects and 40 controls. We performed CpG-level and regional-level analyses. In the benzene EWAS, we found genome-wide significant alterations, i.e., FWER-controlled P-values <0.05, in the mean and variance of methylation at 22 and 318 CpG sites, respectively, and in mean methylation of a large genomic region. Pathway analysis of genes corresponding to benzene-associated differential methylation sites revealed an impact on the AMPK signalling pathway. In formaldehyde-exposed subjects compared to controls, 9 CpGs in the DUSP22 gene promoter had genome-wide significant decreased methylation variability and a large region of the HOXA5 promoter with 44 CpGs was hypomethylated. Our findings suggest that DNA methylation may contribute to the pathogenesis of diseases related to benzene and formaldehyde exposure. Aberrant expression and methylation of HOXA5 previously has been shown to be clinically significant in myeloid leukaemias. The tumour suppressor gene DUSP22 is a potential biomarker of exposure to formaldehyde, and irregularities have been associated with multiple exposures and diseases.
Introduction
Benzene and formaldehyde are major industrial chemicals and ubiquitous environmental pollutants. Both chemicals are present in cigarette smoke and automobile emissions, and formaldehyde is also found in household and consumer products[Citation1], [Citation2]. The current U.S. permissible occupational exposure limit (time-weighted average in an 8-hour period) is 0.75 ppm for formaldehyde [Citation3] and 1 ppm for benzene [Citation4]. In the U.S., occupational benzene exposure levels are typically below 1 ppm [Citation5] and formaldehyde exposure in certain occupational settings can be relatively high [Citation6,Citation7]. For example, short-term exposures to levels of 3 ppm and higher were reported for embalmers, pathologists, and paper workers [Citation8]. Mean air concentrations ranging from less than 1 ppm to greater than 3 ppm were reported in factories that produced formaldehyde-resins in the 1980s [Citation9].
Benzene and formaldehyde have been classified as carcinogenic to humans (Group 1) by the International Agency for Research on Cancer (IARC) [Citation10]. Benzene is an established risk factor for various myeloid neoplasms, including myelodysplastic syndromes and acute myeloid leukaemia (AML) [Citation11]. IARC concluded that there is sufficient evidence to associate formaldehyde with leukaemia, particularly the myeloid subtype [Citation12].
The potential molecular mechanisms by which benzene and formaldehyde are currently understood to cause leukaemia need further elucidation [Citation13–15]. Benzene and formaldehyde exhibit multiple key characteristics (KCs) of carcinogens [Citation16]. Although both chemicals could induce epigenetic alterations, the fourth KC of carcinogens, the strength of the evidence is limited. A systematic review of epigenetic changes associated with 28 Group 1 carcinogens, including benzene and formaldehyde, showed that DNA methylation was the most studied epigenetic endpoint [Citation17].
Altered global and candidate gene DNA methylation in peripheral blood cells from workers occupationally exposed to low levels of airborne benzene compared with unexposed controls has been reported in earlier studies. Small reductions in global LINE-1 and Alu DNA methylation, as well as hypermethylation in p15 and hypomethylation in MAGE1, were reported in 77 healthy Italian traffic officers and 78 gas station attendants exposed to ambient benzene (~20 ppb) compared to 58 controls [Citation18,Citation19]. Hypomethylation in LINE-1 and p15 was associated with urinary S-phenylmercapturic acid (SPMA) levels, a biomarker of benzene exposure, in 158 Bulgarian petrochemical workers exposed to benzene (median 0.46 ppm) compared with 50 unexposed office workers [Citation20]. LINE-1 methylation was significantly lower, and O6-methyl- guanine-DNA methyltransferase (MGMT) and human MutL Homolog 1 (hMLH1) promoter methylation were significantly higher in 83 benzene-exposed (median 2 ppm) Chinese shoe factory workers, compared with 48 unexposed control workers. Hypermethylation of hMLH1 was also found in AML-1 cells after treatment with p-benzoquinone (BQ). In 96 non-smoking Chinese male petrochemical industry workers exposed to benzene (median 0.34 ppm) compared with 100 matched unexposed control workers, promoter methylation in MGMT was negatively associated with urinary SPMA [Citation21]. Altered levels of promoter methylation in IL6, CYP2E1, and iNOS were found in 14 gas station attendants exposed to benzene (mean 0.06 ppm) compared with 22 administrative workers with no solvent exposure [Citation22]. Global DNA hypomethylation was associated with cumulative benzene exposure in 410 Chinese shoe factory workers compared with 102 controls [Citation23]. Some of these populations may have been exposed to factors other than benzene and no findings have been published on genome-wide DNA methylation.
As a reactive methyl donor known to enter the one-carbon metabolism (methyl) pool and interact with enzymes in the associated pathway [Citation24,Citation25], formaldehyde could alter DNA methylation. Few studies have reported on DNA methylation in relation to formaldehyde exposure. A time-related decrease in global DNA methylation was found in human bronchial (16HBE) cells treated for 24 weeks with 10 µM formaldehyde for 24 hours once per week [Citation26]. A 2019 study of 49 salon workers reported increased global DNA methylation in 15 lower-exposed workers (0.03–0.06 ppm) and 26 higher-exposed workers (0.08–0.24 ppm) compared with 8 workers unexposed to formaldehyde (<0.01 ppm) [Citation27].
Given the limited information on genome-wide DNA methylation effects in benzene- and formaldehyde-exposed populations, we sought to perform epigenome-wide association studies (EWAS) to assess DNA methylation in two occupational studies of factory workers in China exposed to benzene and formaldehyde, with extensive personal monitoring, in which hematotoxicity [Citation28–31] and perturbed expression of many genes and pathways were previously reported [Citation32–35]. As DNA methylation biomarkers are stable and represent a potential link between environmental exposure and disease [Citation36], identification of DNA methylation biomarkers of lower-dose exposure to benzene and formaldehyde would be a useful step towards improving risk assessment and minimizing adverse health effects. We examined differences in the mean and variance of DNA methylation. Increased DNA methylation variability has been associated with cancer progression [Citation37], Type 1 diabetes in three immune effector cell types [Citation38], and chronic obstructive pulmonary disease [Citation39].
Materials and methods
Study design, sample collection, and exposure measurement and definition
Detailed information on study design, exposure assessment, and haematological data for the benzene and formaldehyde studies was previously reported [Citation28–31]. In brief, the benzene study was conducted in Tianjin, China, on 250 exposed workers from two shoe manufacturing facilities and 140 unexposed workers from other factories. Personal benzene exposure was repeatedly monitored using 3 M organic vapour passive monitoring badges that were worn by workers for a full work shift for a period up to 16 months. The formaldehyde study was conducted in Guangdong, China, on 43 exposed workers in two factories that used or manufactured melamine and 51 unexposed workers from separate factories in the same geographic region that were matched to the exposed workers by sex and age (± 5 y). Personal formaldehyde exposure was monitored with SKC UMEx 100 passive samplers, that were worn by workers in the exposed factories for a full work shift for around 3 workdays across a 3-week period.
Questionnaires were administered to subjects in both studies to gather information on occupational and medical history, environmental exposures, and current tobacco and alcohol use. Blood and urine samples, and other biologic specimens were collected from every worker. Informed consent was obtained from all subjects, and the studies were approved by Institutional Review Boards at the U.S. National Cancer Institute, the Guangdong National Poison Control Centre and the Chinese Center for Disease Control and Prevention.
The larger number of study subjects and the wider range of exposure in the benzene study provided adequate exposure contrasts for the continuous exposure evaluation. The smaller formaldehyde study consisted of relatively highly exposed workers and as such did not provide sufficient variability for continuous exposure evaluation. Instead, the formaldehyde study design was analysed based on dichotomized exposure analysis, i.e., either exposed to formaldehyde or unexposed to formaldehyde.
DNA methylation assay
Peripheral blood samples were collected and delivered to the processing laboratory within 6 hours. The complete blood count and differential were analysed using a Beckman-Coulter® T540 blood counter (benzene) or a Sysmex XT-1800i automated haematology analyser (formaldehyde). Lymphocyte subsets were measured using a FACS Calibur flow cytometer (Software: SimulSET v. 3.1).
Genome-wide DNA methylation was analysed in 107 benzene samples (53 controls and 54 exposed workers) and 74 formaldehyde samples (43 controls and 31 exposed workers) assay. The sample numbers include technical replicate pairs (9 for benzene and 1 for formaldehyde) selected from study samples with the highest available DNA mass. DNA was extracted from blood cells using the phenol-chloroform extraction method. DNA (1000 ng), quantitated by Quant-iT PicoGreen dsDNA kits (Life Technologies, Grand Island, NY), was treated with sodium bisulphite using the EZ-96 DNA Methylation MagPrep Kit (Zymo Research, Irvine, CA) according to manufacturer-provided protocol. Bisulphite-converted DNA samples were hybridized to the 12 sample Illumina HumanMethylation 450 BeadChips using the Infinium HD Methylation protocol (Document 15,019,519 v01). The methylation β-value, the proportion of DNA methylation at each CpG site, is obtained as a ratio of the intensities of fluorescent signals. A β-value of 0 indicates a completely unmethylated CpG site and a β-value of 1 indicates a fully methylated CpG site. The M-value, the logit2 transform of the β-value, was utilized in downstream statistical analyses.
DNA methylation data preprocessing
Data preprocessing for methylation arrays commonly considers quality control, probe and sample filtering, normalization, and batch effect correction [Citation40]. The quality control report provided by the minfi software [Citation41] on Bioconductor [Citation42] was used to evaluate the quality of the raw data from the benzene and formaldehyde experiments.
For the benzene data, experimental abnormalities, such as bisulphite conversion outliers, were not detected from the strip plots of internal control probes. According to the density plots, the samples did not exhibit an irregular distribution of methylation values. All samples had less than 1% of failed probes and no sample had a low raw signal intensity (>13 average log median signal in the methylated and unmethylated channels). Based on these various sample-specific quality control metrics, no samples were filtered out of the benzene data, aside from filtering out one of the technical replicates from each pair (details below). The relative proportion of leukocytes within each sample was estimated from the DNA methylation data with the minfi function, estimateCellCounts: an algorithm that implements a regression calibration approach for deconvolution of heterogeneous tissues by integrating reference data that is sorted into pure cellular populations [Citation43]. This function returns estimates of the relative proportions of lymphocytes, monocytes, B-cells, and neutrophils, using reference data provided by the FlowSorted.Blood.450k software on Bioconductor. To reduce any batch effects present in the provided and sorted reference data cell-type discriminating probes, stratified quantile normalization of the provided data with the reference data is performed. This stratified quantile normalization [Citation44] also corrects for probe-type bias [Citation40]. Probe filtering consisted of omitting probes that failed to hybridize to the array (detection P-value >0.01), non-CpG probes, SNP-related probes according to Zhou [Citation45], multi-hit (i.e., cross-reactive) probes, and probes located on sex chromosomes (Table S1) [Citation40].
The criteria for selecting the technical replicate to retain from the pair was based on the quality of the methylation data, in particular the sum of detection P-values across all retained probes. The technical replicate with the lowest total detection P-value, and thus highest quality of methylation across all probes, was retained. After filtering 407,241 probes and 98 samples remained (48 unexposed to benzene and 50 exposed to benzene). Lastly, batch effects remained in the data due to the sample plate, as is common, so methylation M-values were adjusted to account for sample plate batch effects using the sva ComBat algorithm [Citation46] on Bioconductor.
Probe filtering, normalization, batch effect correction, and cell count estimation procedures for the formaldehyde EWAS mirrored the approach detailed above for the benzene EWAS. For the formaldehyde data, two samples with failed signal intensities (<13 average log median signal in the methylated and unmethylated channels) were removed (Supplemental Figure S1). One technical replicate was also selected at random and removed. Afterwards, 402,327 probes and 71 formaldehyde samples remained (40 controls and 31 exposed subjects).
Assessment of associations of DNA CpG methylation with exposure
Genome-wide differential methylation analysis of the benzene and formaldehyde data was performed with limma [Citation47] and missMethyl [Citation48] software, both available on Bioconductor. The identification of differentially methylated CpG positions (DMPs), i.e., regressing CpG mean DNA methylation on exposure and confounders and then examining the coefficient in front of the exposure variable, is a standard analytical approach to identify CpG sites associated with an exposure of interest when confounders are held constant. Differentially variable CpG positions (DVPs) can identify larger differences in CpG methylation than DMPs [Citation38].
To assess DMPs in the two studies (separately), linear models were fit to the methylation probe M-values. For the benzene EWAS, the linear models included as main terms the continuous exposure measurement as well as the following confounders: estimated blood cell counts (monocytes, granulocytes, B cells, NK cells, CD4 cells, CD8 cells), body mass index (BMI), age, smoking, and sex. For the formaldehyde data, the linear models included as main terms the binary formaldehyde exposure, the estimated blood cell counts (granulocytes, B cells, NK cells, CD4 cells, CD8 cells) and individual characteristics (BMI, age, sex). Since smoking was confounded with sex in the formaldehyde study (no females smoked), it was not included as a main term in the regression. To assess DVPs, missMethyl was used to first calculate the variance of DNA methylation probe M-values and then linear models were fit to each CpG probe’s variance [Citation49]. The same set of variables that were included as main terms in the DMP analyses were included as main terms in both DVP analyses.
After fitting linear models for DVP and DMP analyses in both the formaldehyde and benzene studies, we performed a stabilization of the t-statistics. This procedure results in increased statistical power and better performance compared to ordinary t-statistics, which are highly prone to false discoveries in high-dimensional data [Citation50]. A family-wise error rate (FWER) threshold of 0.05 for Bonferroni corrected P-values was used to define genome-wide significance. CpGs that met a false discovery rate (FDR) threshold of 0.05, i.e., Benjamini-Hochberg corrected P-value <0.05, were filtered for single nucleotide polymorphisms (SNPs) specific to the East Asian super-population (EAS) and then matched to genomic features and considered in pathway analysis. The population-specific SNP annotation is provided by the omicsPrint software [Citation51] on Bioconductor. We also calculated the genomic inflation factor () [Citation52] from the results returned by the DMP and DVP analyses, which compares the genome-wide distribution of the P-values with the expected null distribution.
Analysis of differentially methylated regions associated with exposure
We used the bumphunter algorithm [Citation53] methods within the minfi software to examine differences in mean DNA methylation across smaller regions of CpG probes (DMRs) and blocks (DMBs), large-scale regions comprised of open-sea CpG probe clusters. A maximum separation of 1,000 base pairs defined potential clusters of probes in the DMR analysis. A total of 1,000 bootstrap samples were used to simulate a null distribution for both DMR and DMB analysis.
In both the benzene and formaldehyde DMR analyses, the 99th quantile of the bootstrap sampled null distribution defined the cutoff to select candidate regions; loess smoothed regression coefficients above (in absolute value) this quantile were selected as candidates. For the DMB analyses, absolute loess smoothed regression coefficients above 0.1 defined the cutoff to select candidate blocks. For both the regional and block analyses, regressions adjusted for the same confounders included in the DVP/DMP analyses. A bootstrap-based FWER <0.05 was used as the cutoff to denote genome-wide significance of regions and blocks, and an unadjusted P-value <0.05 was the cutoff for individual significance.
Mapping significant probes and regions to genes
Probe-wise results (DMPs and DVPs) that achieved a BH-corrected P-value <0.05 and did not match to EAS SNPs were annotated to gene information, and regional results (DMRs and DMBs) that achieved an unadjusted P-value <0.05 were annotated to gene information. The 450 K array annotation file provided by Illumina (HumanMethylation450 v1.2 Annotation File) was used to match these significant regions and CpGs to genes.
Biological pathway analysis with genes mapped to significant results
For the genes identified from significant results, as defined above, we performed gene set enrichment analysis (GSEA), specifically biological pathway analysis with the online Enrichr interactive software [Citation54,Citation55]. We examined enrichment of Kyoto Encyclopedia of Genes and Genomes (KEGG) pathways. There is a probe bias that is introduced by having a different number of CpG probes for each gene, and Enrichr does not account for this bias because it takes genes as input. With Enrichr, we were able to consider all candidate genes (i.e., those matching to both probe-wise and region/block significant results). Therefore, the Enrichr biological pathway analysis is more comprehensive than the probe-wise approach, but it may be biased. However, since the gene list in this GSEA was established from results deemed significant after multiple testing corrections, the impact of probe bias here is likely less severe than previously reported analyses that aimed to investigate this bias [Citation56].
Results
In the benzene study, we analysed genome-wide DNA methylation in the blood of 48 controls, non-occupationally exposed subjects (<0.035 ppm benzene exposure), and 50 occupationally exposed subjects (benzene exposure mean of 6.02 ppm and standard deviation of 12.9 ppm) [Citation30]. Demographic characteristics were comparable among these two groups (). As ambient benzene levels were below the level of detection in the control subjects, we estimated benzene exposure using unmetabolized urinary benzene levels as described previously [Citation57]. We previously reported that urinary benzene and mean individual air levels of benzene were strongly correlated (Spearman correlation coefficient = 0.88, P-value <0.0001) in the epidemiologic study population [Citation30]. This continuous benzene exposure measurement was used for the benzene EWAS, as this was the intention of the benzene study’s design. Also, continuously defined exposures are the most faithful representation of exposure, and continuous exposure–response relationships offer the potential to be more informative than a binary (control vs. exposed) classification because they provide insight regarding how the response changes under small, 1-unit increases in exposure.
Table 1. Demographic characteristics and benzene occupational exposure level.
For the formaldehyde study, we examined genome-wide DNA methylation in the blood of 40 controls, non-occupationally exposed subjects (<0.020 ppm formaldehyde exposure), and 31 occupationally exposed subjects (formaldehyde exposure mean of 1.26 ppm and standard deviation of 0.62 ppm). Demographic characteristics were comparable among the binary comparison groups (). In the formaldehyde EWAS, we defined formaldehyde exposure as a binary variable because this study consisted of relatively highly exposed workers, and a smaller sample size; it did not provide adequate exposure contrasts for continuous exposure evaluation.
Table 2. Demographic characteristics and formaldehyde occupational exposure level.
Association between exposure and DNA CpG methylation
In the benzene study, we identified differentially methylated CpGs positions associated with continuous benzene exposure measurements, where differential CpG methylation was examined in terms of both the mean (DMPs) and variance (DVPs). For the DMP and DVP analyses, we adjusted for estimated blood cell counts (monocytes, granulocytes, B cells, NK cells, CD4 cells, CD8 cells), BMI, age, smoking, and sex. After BH P-value adjustment to control the FDR, 61 DMPs remained significant and 26 DMPs were significant after the more conservative Bonferroni correction, which controls the FWER. Larger numbers of significant DVPs remained after BH and Bonferroni P-value corrections, 1,688 DVPs and 324 DVPs, respectively. All significant DVPs exhibited increased variance of DNA methylation with increased exposure to benzene. The genomic inflation factor ()
for the DMP results was 1.04, suggesting no inflation of the DMP P-values. The
value for the DVP results was 0.70, suggesting deflated DVP P-values. Manhattan plots of the DMP and DVP results are shown in Supplemental Figure S2. Filtering out EAS-specific SNPs from the BH-significant CpG probes led to the removal of 6 of the 61 significant DMPs and 32 of the 1,688 significant DVPs, leaving 55 significant DMPs (Table S2), and 1,656 significant DVPs (Table S3). After filtering out EAS SNPs and applying Bonferroni P-value correction, there were 22 significant DMPs () and 318 significant DVPs, the top 25 of which are listed in (in order of decreasing significance). Thirty-two of the 55 remaining BH-based significant DMPs (58%) were also BH-based significant DVPs (Table S2).
Table 3. Genome-wide significant (Bonferroni corrected P-value <0.05) differentially mean methylated probes (DMPs) from a cross-sectional study of occupational exposure to benzene, where differential methylation was assessed with respect to continuous benzene exposure.
Table 4. Top 25 out of 318 genome-wide significant (Bonferroni corrected P-value <0.05) differentially methylated probes, where differential methylation was defined in terms of the variance of CpG probe methylation (DVPs), from a cross-sectional study of occupational exposure to benzene, and differential methylation was assessed with respect to continuous benzene exposure.
In the formaldehyde study, we looked for DMPs and DVPs that were differentially methylated between the exposed and unexposed groups. For both the DMP and DVP analyses, linear models were adjusted for sex, BMI, age, and estimated granulocytes, monocytes, CD4 cells, CD8 cells, NK cells, and B cells. We identified 25,035 DMPs with an unadjusted P-value <0.05, none of which remained significant after BH-based P-value correction (Table S4). Of 19,970 DVPs identified with an unadjusted P-value <0.05, 9 – located in the dual specificity protein phosphatase 22 gene (DUSP22) – remained significant after Bonferroni P-value correction and exhibited decreased variance of DNA methylation in the formaldehyde-exposed workers relative to controls ( and ). An additional DVP in DUSP22 was also significant after BH-based P-value correction (Table S5). A Manhattan plot of the DVP results is shown in . The values for the DMP results were 1.125, and for the DVP results 1.018, suggesting no inflation of the P-values for probe-wise results in the formaldehyde EWAS. For both the formaldehyde and benzene EWAS, the full list of probe-wise results is available on GitHub (https://github.com/rachaelvp/EWAS-BZ-FA) and Open Science Framework (https://osf.io/exqzy/).
Table 5. Genome-wide significant (Bonferroni corrected P-value <0.05) differentially variable probes (DVPs) from a cross-sectional study of occupational exposure to formaldehyde, where differential methylation was assessed with respect to binary, exposed versus controls, comparison groups.
Figure 1. Formaldehyde-associated differential DNA methylation in the DUSP22 gene, highlighting nine differentially variable probes (DVPs) that exhibited significant (Bonferroni-adjusted P-values <0.05) decreased variance of DNA methylation in formaldehyde-exposed workers compared to controls (a). All CpG probes on the 450 K array that are annotated to the DUSP22 gene (excluding any that might have been filtered out) are shown, with the nine significant DVPs being those in the red box (A). The pronounced significance of these 9 DVPs can be seen in the Manhattan plot, where the red and blue lines represent the family-wise error rate (FWER) and false discovery rate (FDR) thresholds, respectively, for statistical significance (b).
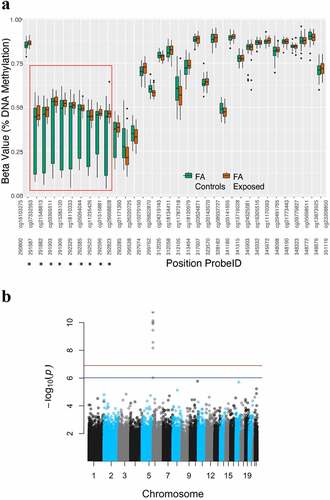
Association between exposure and regions of DNA methylation
We identified differential mean methylation across small-scale regions of CpG probes (DMRs) and large open-sea regions, or ‘blocks,’ of CpG probes (DMBs), where differences were defined with respect to associations with continuous benzene exposure measurements and the binary formaldehyde exposure classification. DMR and DMB analyses were adjusted for the same measured variables as in the DMP and DVP analyses. For both the formaldehyde and benzene EWAS, the full list of regions and blocks identified is available on GitHub (https://github.com/rachaelvp/EWAS-BZ-FA) and Open Science Framework (https://osf.io/exqzy/).
In the benzene DMR analysis, 1,202 candidate regions were identified, 144 achieved significance based on an unadjusted P-value <0.05 (Table S6), and no DMRs achieved genome-wide significance based on an FWER threshold of 0.05. For the benzene DMB analysis, 440 candidate blocks were identified and 60 achieved significance based on an unadjusted P-value <0.05 (Table S7). One DMB containing four CpG probes, and exhibiting slight hypomethylation with increased benzene exposure, achieved genome-wide significance with an FWER-controlled P-value of 0.018 and was annotated to the following three genes: C19orf53, CCDC130, and MRI1 (Table S7).
In the formaldehyde DMR analysis, we identified 1,349 candidate DMRs, 147 of which were significant based on an unadjusted P-value <0.05 (Table S8). We identified one genome-wide significant DMR (FWER-controlled P-value of 0.01), consisting of 2,174 base-pairs and located in the HOXA5 promoter (). This DMR exhibited hypomethylation in the exposed group compared to controls. The second most significant DMR, consisting of 1,598 base-pairs and located in the DUSP22 gene (), exhibited hypermethylation and nearly reached genome-wide significance (FWER-controlled P-value of 0.063). The DMB analysis led to the identification of 367 candidate DMBs, 52 of which were significant based on an unadjusted P-value <0.05 (Table S9). No DMB achieved significance after adjustment for multiple comparisons.
Figure 2. Genomic annotation of two regions exhibiting differential mean methylation (DMRs) in formaldehyde-exposed subjects versus controls. A 2,174 base-pair region on chromosome 7, comprised of 44 CpG probes, and located in the promoter of HOXA5 exhibited significant hypomethylation in formaldehyde exposed workers compared to controls, with a family-wise error rate (FWER)-controlled P-value = 0.01 (a). A 1,598 base-pair region on chromosome 6, comprised of 10 CpG probes, and located in the DUSP22 gene exhibited nearly genome-wide significant hypermethylation in formaldehyde exposed subjects compared to controls, with an FWER-controlled P-value = 0.063 (b).
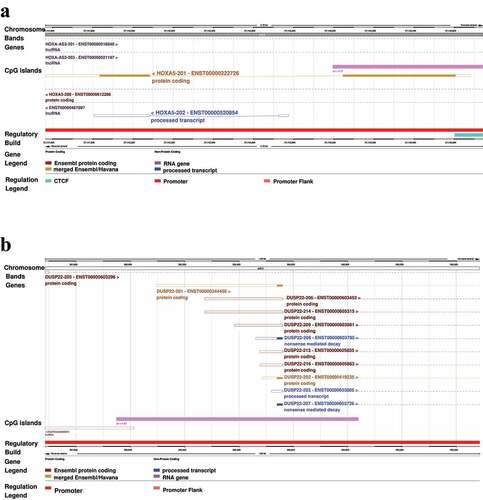
Mapping significant probes and regions to genes
Significant differentially methylated probes, regions, and blocks identified in the benzene EWAS were mapped to genes. The criteria for probe-wise significance were probes that did not match to EAS SNPs and achieved BH-based significant P-values <0.05, and the criteria for region- and block-wise significance were regions/blocks that achieved unadjusted P-values <0.05. Out of the 55 significant DMPs and 1,656 significant DVPs; 44 and 1,346 were located within 52 and 1,299 genes, respectively (). A total of 89 genes and 63 genes were mapped to the significant DMRs and DMBs, respectively (Tables S6 and S7). Some genes were identified in common across these various analyses with consistent directionality of differential methylation, which was significantly associated with increasing benzene exposure. One gene, PTPRN2, was found among three of the four analyses of differential methylation. With increased benzene exposure, a DMR (unadjusted P-value <0.05) and a DMP (Bonferroni-adjusted P-value <0.05) in PTPRN2 exhibited hypomethylation, and seven significant DVPs (two with Bonferroni-adjusted P-value <0.05 and five with BH-adjusted P-value <0.05) in PTPRN2 exhibited increased variability. Genes ARPP21 and TRPC3 overlapped across the significant DMR and DMB-identified genes (unadjusted P-values <0.05), both exhibiting a relationship of hypomethylation with benzene exposure (Table S5). There was overlap of 37 genes across those annotated to significant DVPs and DMPs (Table S2). In addition to PTPRN2, 11 other genes were annotated to both significant DVPs (Bonferroni or BH-adjusted P-values <0.05) and significant DMRs (unadjusted P-values <0.05): UNC45A, MUC4, CIDEB, LTB4R2, BRF1, BTBD6, F11R, EHHADH, TXNRD1, GTDC1, ACTA1 (Table S3). Lastly, the following 11 genes were mapped to both significant DVPs (Bonferroni or BH-adjusted P-values <0.05) and significant DMBs (unadjusted P-values <0.05): CCDC130, FUT10, STK35, TMEM155, CCNA2, SLC23A2, INSR, YTHDF1, SNORD50A, SNHG5, SNORD50B (Table S3).
Mapping the significant DMRs and DMBs (unadjusted P-values <0.05) in the formaldehyde EWAS to genes resulted in 114 DMR genes and 75 DMB genes (Tables S8 and S9, respectively). None of these genes were found in both types of endpoints. The DUSP22 gene, which was shown to have nine genome-wide significant DVPs in the promoter region was identified as a DMR, nearly reaching genome-wide significance with an FWER-controlled of P-value 0.063.
Association of benzene exposure with biological pathways
We performed pathway analysis using the genes that matched to significant differentially methylation regions and probes. This resulted in the identification of 16 significantly (P-value <0.05) enriched KEGG pathways (Table S10). The KEGG pathway ‘AMPK signaling pathway’ (hsa04152) was the most significant KEGG pathway GSEA (P-value = 0.0004, BH-adjusted P-value = 0.1086). A total of 20 genes identified from significant regions/probes of differential methylation were found in the AMP-activated protein kinase (AMPK) signalling pathway ().
Figure 3. Benzene occupational exposure-associated enrichment of the AMP-activated protein kinase (AMPK) signalling pathway. This was the most significant finding from the gene set enrichment analysis (GSEA) of Kyoto Encyclopedia of Genes and Genomes (KEGG) pathways. The 20 genes that corresponded to sites exhibiting significant, benzene exposure-associated differential DNA methylation are highlighted in pink.
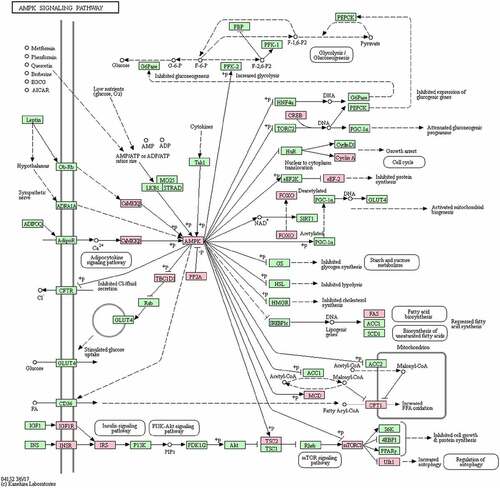
Discussion
In these first reported, to the best of our knowledge, observational EWAS in human study populations occupationally to exposed benzene or formaldehyde, we assessed epigenome-wide DNA methylation, incorporated individual-level exposure assessment data, and adjusted for demographic variables as well as detailed blood cell and subset counts in our analyses. We found that benzene and formaldehyde exposure were each distinctly associated with DNA methylation.
The findings of differential variability among many genes in the benzene EWAS support the links between benzene exposure and cancer [Citation11,Citation58]. Increased epigenetic variability is considered to result from epigenetic instability or the loss of epigenetic control of genomics [Citation59], and it has been described in cancer [Citation60], rheumatoid arthritis (RA) [Citation61] and Type 1 diabetes [Citation38]. It has also been associated with exposure to trichloroethylene [Citation62]. Whereas all the genome-wide significant DVPs showed increased variance (Table S3) with increased continuous benzene exposure, the deflated (0.70)
is suggestive of underpowered genome-wide testing or other population stratification and thus results must be interpreted with caution. Many benzene DVPs (32) were also DMPs, and this pattern has previously been reported [Citation62], but it remains unclear if there is a relationship between differential variability and differential mean methylation. Additional research is required to determine if benzene-induced health outcomes are related to benzene-induced epigenetic drift. The majority of genome-wide significant DMPs showed decreased methylation with increased benzene exposure.
Several genes identified by the DVP/DMP analyses have previously been associated with benzene, AML, or lymphoma. For example, in the same benzene study population, we previously reported that benzene exposure increased the mRNA expression of PHLPP2, ARRDC2, and EIF4ENIF1 (all significant DVPs in the current benzene study) and decreased mRNA expression of TRIM11 (significant DVP and DMP in the current benzene study) [Citation63]. TRIM11 gene expression was shown to be elevated in lymphoma cell lines and may act as an oncogene in lymphoma by activating the β-catenin signalling[Citation64]. Expression of the tumour suppressor PHLPP2 [Citation65] is reduced in certain AML subtypes and is regulated by the oncomiR-17–92 [Citation66]. Expression of RUNX2 (significant DVP in the current benzene study), an AML oncogene [Citation67], was increased in human bone marrow mesenchymal stem cells after treatment with benzene and its metabolites P-benzoquinone (BQ) and hydroquinone (HQ) [Citation68]. A mutation in ZBTB7A (significant DVP in the current benzene study) in AML with t(8;21) translocation [Citation69], may cooperate with t(8;21) to drive leukaemogenesis[Citation70].
The AMPK signalling pathway was significantly impacted by benzene exposure in the more comprehensive gene-wise pathway analysis of genes corresponding to significant probe-wise (DMPs and DVPs) and regional (DMRs and DMBs) results. The effect may be driven by increased variance, as several AMPK pathway genes were significant in the DVP analysis (INSR, PPP2R3A, CAMKK2, CCNA2, and FASN) whereas none were significant in the DMP analysis. AMP-activated protein kinase (AMPK) is a master regulator of cellular energy homoeostasis that is activated in response to cellular ATP depletion by stressors such as low glucose. Activated AMPK regulates metabolic pathways and affects the activity of various proteins involved in ageing, cell growth and apoptosis, and it promotes autophagy [Citation71,Citation72] and longevity [Citation73,Citation74]. AMPK plays a key role in the differentiation of haematopoietic stem cells (HSCs), progenitors, and myeloid cells, through the induction of autophagy, and the AMPK pathway is a potential clinical target to subvert dysregulated differentiation during myeloid malignancy [Citation75]. AMPK can act as both a tumour suppressor and tumour supporter. Drugs that inhibit mTOR and activate AMPK have beneficial effects in promoting differentiation and blocking proliferation of AML [Citation76]. Liver B Kinase (LKB1) is a tumour suppressor that activates AMPK in response to energy stress. The Liver kinase B1 (LKB1)-AMPK axis was shown to potentially mediate the biological effects (proliferation and differentiation inhibition, G1 cell cycle arrest, and apoptosis induction) of HQ on murine foetal liver and bone marrow HSCs [Citation77].
The key relationships of formaldehyde and differential DNA methylation were in the promoter region of tumour suppressor gene, DUSP22, in which we found decreased variability of DNA methylation across 9 CpGs (many of which were encompassed in a DMR exhibiting increased mean methylation, FWER-controlled P-value of 0.063). Dual specificity phosphatase (DUSP) proteins are major modulators of critical signalling pathways that are dysregulated in various diseases [Citation78]. DUSP22 inactivates various protein kinases and transcription factors through dephosphorylation and influences the duration and intensity of multiple signalling pathways [Citation79–85] including mitogen-activated protein kinase (MAPK), T-cell activation [Citation86], oestrogen receptor [Citation82] and epidermal growth factor (EGF) and androgen receptor (AR) signalling[Citation87]. Loss of DUSP function may lead to aberrant proliferation, inflammation, or malignancy [Citation87]. DUSP22 genetic rearrangements or loss of function have been associated with anaplastic lymphoma kinase–negative anaplastic large cell lymphoma [Citation88,Citation89], prostate cancer progression [Citation87] and systemic lupus erythematosus [Citation86]. Altered methylation of DUSP22 in association with environmental stressors and disease has been reported. Increased methylation in the same region of the DUSP22 gene (Chr6: 291687–293285) as detected in our formaldehyde study was previously shown to be involved in the response to early-life exposure to famine and was associated with schizophrenia regardless of famine exposure [Citation90]. Hypomethylation was found in erosive RA in immune cell subpopulations [Citation91], and was associated with post-traumatic stress disorder (PTSD) symptoms in deployed military servicemen over time [Citation92] and with duration of service in firefighters [Citation93]. Hypomethylation was also reported in newborns prenatally exposed to perfluoroalkyl substances (PFAS) [Citation94], and maternal diabetes or obesity [Citation95]. The overlapping combination of decreased methylation variability and increased mean methylation in DUPS22 is unique. This pattern of decreased variance accompanying mean methylation alterations may suggest controlled epigenetic reprogramming. Further studies are needed to understand the potential contribution of these effects to formaldehyde toxicity and disease. DUSP22 was identified as a DMR (but not a DVP, DMP, or DMB) in the benzene study, exhibiting decreased mean methylation, but did not reach significance based on FWER control.
Formaldehyde was also associated with a genome-wide significant DMR in HOXA5, with decreased mean methylation in the exposed group compared to controls. HOXA5 encodes a transcription factor that regulates differentiation of the myeloid and erythroid lineages [Citation96,Citation97]. Aberrations in HOXA gene family members as well as their cofactor, homeobox protein Meis 1 (MEIS1), occur in AML [Citation98]. Irregular expression and methylation of HOXA5 has been shown to be clinically significant in AML. HOXA5 is frequently hypermethylated in adult AML and its consequent inactivation has prognostic value [Citation99,Citation100]. HOXA5 expression distinguished between AML with mutations in nucleophosmin 1 (NPM1) compared to wild-type AML and increased HOXA5 expression was correlated with poor survival [Citation101]. Patients with favourable chromosomal aberrations had decreased expression levels of HOXA5 and MEIS1 compared with normal-karyotype AML and the adverse cytogenetic risk patients [Citation102]. The role of DNA methylation and dysregulation of HOXA5 in formaldehyde-induced hematotoxicity, and the potential for AML induction requires further study.
The results should be considered in the context of the study’s strengths and limitations. Strengths include the use of study populations with well-characterized exposures, and a rigorous study design that incorporated phenotypic information for each subject [Citation28–31]. A conservative analytical pipeline, which considered genome-wide significance on the basis of Bonferroni corrected P-values <0.05 (FWER threshold of 0.05), and stabilized t-statistics, which have been shown to confer increased statistical power and enhanced performance compared to ordinary t-statistics [Citation50], minimized the likelihood of false discoveries. Evaluation of differential DNA methylation was comprehensive, assessed in terms of mean and variance, and at the level of individual CpGs and genomic regions. These strengths support the reliability of the findings for benzene and formaldehyde.
In the formaldehyde study, differential DNA methylation considered the binary comparison groups (exposed vs. controls), so it cannot be interpreted how DNA methylation changes with increased formaldehyde exposure. Further work in larger sample sizes with a greater range of exposure will be needed to address this open question. However, the exposed workers experienced relatively high levels of formaldehyde, providing a striking contrast to unexposed controls, and as such the study is useful for its intended purpose for initial exploratory comparisons. The benzene study, a larger study with a wide range of exposure that was designed to assess the exposure–response relationship with biomarker endpoints, was able to assess differential DNA methylation with respect to continuous benzene exposure, taking advantage of the additional power this provides. In the formaldehyde study, none of the mean comparisons for individual CpG sites (DMPs) were significant after accounting for multiple testing. However, we did observe significant variability comparisons for individual CpG sites (DVPs) after multiple hypothesis correction in both the benzene and formaldehyde data. Whereas no DMPs were reliably significant in the formaldehyde study, 22 DMPs achieved genome-wide significance in the benzene study. Also, 9 DVPs achieved genome-wide significance in the formaldehyde study and 318 DVPs achieved genome-wide significance in the benzene study. The reduced number of genome-wide significant results in the formaldehyde EWAS compared to the benzene EWAS could reflect differences in sample size (71 for FA vs. 98 for benzene) and lower statistical power to detect differential methylation. Alternatively, relatively high levels of workplace benzene exposure might have a greater impact on peripheral blood cell DNA methylation compared to relatively high levels of workplace formaldehyde exposure. Also, due to the number of sites assayed by the 450 K array, we likely missed some associations for both exposures. For example, the fact that only nine DMPs in the formaldehyde study covered a 1,136-base-pair-long region () is a technicality, and limitation, of the 450 K microarray.
Smoking is a potential source of exposure to both benzene and formaldehyde. However, occupational exposure to benzene and formaldehyde in the study factories was substantially greater than exposure that could result from tobacco smoking [Citation103,Citation104]. Further, the proportion of current smokers in the exposed and control factories in both the benzene study and the formaldehyde study were comparable, and in addition, statistical analyses were adjusted for smoking status. As such, it is highly unlikely that benzene or formaldehyde from smoking could have influenced the study results or conclusions.
As differential DNA methylation in blood cells may be confounded by blood cell composition variations [Citation105], and since occupational exposure to benzene and formaldehyde in these populations has been previously associated with altered blood cell subset counts [Citation30,Citation31], it was critical to account for differences in cell counts in these studies. When linear models were fit to methylation probes, we adjusted for estimated blood cell counts (granulocytes, monocytes, B cells, NK cells, CD4 cells, CD8 cells), in addition to sex, smoking, BMI, and age. Estimated counts have been experimentally validated to reflect actual cell counts [Citation106,Citation107]. For all fitted models, the coefficient in front of the exposure variable of interest (benzene or formaldehyde) corresponds to the relationship between this exposure and DNA methylation when blood cell counts, BMI, age, smoking, and sex are fixed. Therefore, by including these factors in the models, estimates of the association of the exposure (to benzene or formaldehyde) on DNA methylation are not confounded by them in the fitted model. Nonetheless, it is important to replicate these findings in larger studies and in other exposed populations, given the limited diversity in these studies’ populations. Also, we acknowledge that confounding might not be adequately captured, and associations between DNA methylation and these exposures might be missed or biased, by assuming a linear model. A final limitation inherent in the cross-sectional design is the inability to evaluate temporal changes in endpoints, here DNA methylation, including possible reversibility of those associations over time. Future longitudinal studies with repeated sampling during an extended time of workplace exposure, as well as after exposure ceases, will be especially valuable.
In conclusion, these EWAS provide new insights into potential genes and pathways that may be involved in the human response to benzene and formaldehyde exposure. The findings provide additional evidence that DNA methylation may play a role in the pathogenesis of benzene and formaldehyde-related diseases. Further validation of these findings in larger and independent study populations is warranted, as well as examination of the downstream effects of the benzene- and formaldehyde-induced DNA methylation patterns on gene and protein expression. Our findings suggest that DNA methylation may play a role in the pathogenesis of benzene and formaldehyde exposure-related diseases, via distinct mechanisms.
Supplemental Material
Download Zip (852.1 KB)Acknowledgments
This project was supported by the Superfund Research Center at UC Berkeley NIEHS Grant P42ES004705 and by the Intramural Research Program, NCI, NIH.
Disclosure statement
MTS is retained as a consultant and expert witness in U.S. litigation involving benzene and cancer. All other authors declare no actual or competing financial interest.
Data availability statement
Summary results and analysis code (R) for both EWAS are available on GitHub (https://github.com/rachaelvp/EWAS-BZ-FA) and Open Science Framework (https://osf.io/exqzy/).
Supplementary material
Supplemental data for this article can be accessed online at https://doi.org/10.1080/15592294.2022.2115604
Additional information
Funding
References
- Kim KH, Jahan SA, Lee JT. Exposure to formaldehyde and its potential human health hazards. J Environ Sci Health C Environ Carcinog Ecotoxicol Rev. 2011;29:277–299.
- Wallace LA. Major sources of benzene exposure. Environ Health Perspect. 1989;82:165–169.
- Occupational Safety and Health Administration. Occupational exposure to formaldehyde. Federal Regulation. 1992.
- Occupational Safety and Health Administration. Occupational exposure to benzene. Federal Regulation. 1987.
- Weisel CP. Benzene exposure: an overview of monitoring methods and their findings. Chem Biol Interact. 2010;184:58–66.
- Tang X, Bai Y, Duong A, et al. Formaldehyde in China: production, consumption, exposure levels, and health effects. Environ Int. 2009;35:1210–1224.
- Zhang L, Steinmaus C, Eastmond DA, et al. Formaldehyde exposure and leukemia: a new meta-analysis and potential mechanisms. Mutat Res. 2009;681:150–168.
- IARC. Formaldehyde, 2-butoxyethanol and 1-tert-butoxypropan-2-ol. IARC Monogr Eval Carcinog Risks Hum. 2006;88:1–478.
- Stewart PA, Cubit DA, Blair A. Formaldehyde levels in seven industries. Appl Ind Hyg. 1987;2:231–236.
- Benzene IARC. IARC monographs on the evaluation of carcinogenic risks to humans. Vol. 120. Lyons: France: International Agency for Research on Cancer; 2018.
- Hayes RB, Songnian Y, Dosemeci M, et al. Benzene and lymphohematopoietic malignancies in humans. Am J Ind Med. 2001;40:117–126.
- IARC. A review of human carcinogens – part F: chemical agents and related occupations, IARC monographs on the evaluation of carcinogenic risks to humans. Lyons: France: International Agency for Research on Cancer; 2012.
- McHale CM, Zhang L, Smith MT. Current understanding of the mechanism of benzene-induced leukemia in humans: implications for risk assessment. Carcinogenesis. 2012;33:240–252.
- National Toxicology Program. Report on carcinogens, fourteenth edition;https://ntp.niehs.nih.gov/go/roc14. U.S. Department of Health and Human Services; Research Triangle Park, NC; U.S., 2016. Accessed November 9, 2021.
- Zhang L. Formaldehyde: exposure, toxicity and health effects. London, United Kingdom: Royal Society of Chemistry; 2018.
- Smith MT, Guyton KZ, Gibbons CF, et al. Key characteristics of carcinogens as a basis for organizing data on mechanisms of carcinogenesis. Environ Health Perspect. 2016;124:713–721.
- Chappell G, Pogribny IP, Guyton KZ, et al. Epigenetic alterations induced by genotoxic occupational and environmental human chemical carcinogens: a systematic literature review. Mutat Res Rev Mutat Res. 2016;768:27–45.
- Bollati V, Baccarelli A, Hou L, et al. Changes in DNA methylation patterns in subjects exposed to low-dose benzene. Cancer Res. 2007;67:876–880.
- Fustinoni S, Rossella F, Polledri E, et al. Global DNA methylation and low-level exposure to benzene. Med Lav. 2012;103:84–95.
- Seow WJ, Pesatori AC, Dimont E, et al. Urinary benzene biomarkers and DNA methylation in Bulgarian petrochemical workers: study findings and comparison of linear and beta regression models. PLoS One. 2012;7:e50471.
- Li J, Zhang X, He Z, et al. MGMT hypomethylation is associated with DNA damage in workers exposed to low-dose benzene. Biomarkers. 2017;22:470–475.
- Jimenez-Garza O, Guo L, Byun HM, et al. Promoter methylation status in genes related with inflammation, nitrosative stress and xenobiotic metabolism in low-level benzene exposure: searching for biomarkers of oncogenesis. Food Chem Toxicol. 2017;109:669–676.
- Zhang GH, Lu Y, Ji BQ, et al. Do mutations in DNMT3A/3B affect global DNA hypomethylation among benzene-exposed workers in Southeast China?: effects of mutations in DNMT3A/3B on global DNA hypomethylation. Environ Mol Mutagen. 2017;58:678–687.
- Agency for Toxic Substances and Disease Registry (ATSDR). Toxicological profile for benzene. Atlanta: GA: U.S. Department of Health and Human Services, Public Health Service; 2007.
- Kalasz H. Biological role of formaldehyde, and cycles related to methylation, demethylation, and formaldehyde production. Mini Rev Med Chem. 2003;3:175–192.
- Liu Q, Yang L, Gong C, et al. Effects of long-term low-dose formaldehyde exposure on global genomic hypomethylation in 16HBE cells. Toxicol Lett. 2011;205:235–240.
- Barbosa E, Dos Santos ALA, Peteffi GP, et al. Increase of global DNA methylation patterns in beauty salon workers exposed to low levels of formaldehyde. Environ Sci Pollut Res Int. 2019;26:1304–1314.
- Bassig BA, Zhang L, Vermeulen R, et al. Comparison of hematological alterations and markers of B-cell activation in workers exposed to benzene, formaldehyde and trichloroethylene. Carcinogenesis. 2016;37:692–700.
- Hosgood HDs3rd, Zhang L, Tang X, et al. Occupational exposure to formaldehyde and alterations in lymphocyte subsets. Am J Ind Med. 2013;56:252–257.
- Lan Q, Zhang L, Li G, et al. Hematotoxicity in workers exposed to low levels of benzene. Science. 2004;306:1774–1776.
- Zhang L, Tang X, Rothman N, et al. Occupational exposure to formaldehyde, hematotoxicity, and leukemia-specific chromosome changes in cultured myeloid progenitor cells. Cancer Epidemiol Biomarkers Prev. 2010;19:80–88.
- McHale CM, Zhang L, Lan Q, et al. Global gene expression profiling of a population exposed to a range of benzene levels. Environ Health Perspect. 2011;119:628–634.
- Schiffman C, McHale CM, Hubbard AE, et al. Identification of gene expression predictors of occupational benzene exposure. PLoS One. 2018;13:e0205427.
- Thomas R, Hubbard AE, McHale CM, et al. Characterization of changes in gene expression and biochemical pathways at low levels of benzene exposure. PLoS One. 2014;9:e91828.
- Thomas R, McHale CM, Lan Q, et al. Global gene expression response of a population exposed to benzene: a pilot study exploring the use of RNA-sequencing technology. Environ Mol Mutagen. 2013;54:566–573.
- Chung FF, Herceg Z. The promises and challenges of toxico-epigenomics: environmental chemicals and their impacts on the epigenome. Environ Health Perspect. 2020;128:15001.
- Teschendorff AE, Gao Y, Jones A, et al. DNA methylation outliers in normal breast tissue identify field defects that are enriched in cancer. Nat Commun. 2016;7:10478.
- Paul DS, Teschendorff AE, Dang MA, et al. Increased DNA methylation variability in type 1 diabetes across three immune effector cell types. Nat Commun. 2016;7:13555.
- Clifford RL, Fishbane N, Patel J, et al. Altered DNA methylation is associated with aberrant gene expression in parenchymal but not airway fibroblasts isolated from individuals with COPD. Clin Epigenetics. 2018;10:32.
- Morris TJ, Beck S. Analysis pipelines and packages for infinium humanMethylation450 beadchip (450k) data. Methods. 2015;72:3–8.
- Aryee MJ, Jaffe AE, Corrada-Bravo H, et al. Minfi: a flexible and comprehensive bioconductor package for the analysis of Infinium DNA methylation microarrays. Vol. 30. Oxford: England: Bioinformatics; 2014. p. 1363–1369.
- Gentleman RC, Carey VJ, Bates DM, et al. Bioconductor: open software development for computational biology and bioinformatics. Genome Biol. 2004;5:R80.
- Houseman EA, Accomando WP, Koestler DC, et al. DNA methylation arrays as surrogate measures of cell mixture distribution. BMC Bioinformatics. 2012;13:86.
- Touleimat N, Tost J. Complete pipeline for Infinium((R)) human methylation 450K beadchip data processing using subset quantile normalization for accurate DNA methylation estimation. Epigenomics. 2012;4:325–341.
- Zhou W, Laird PW, Shen H. Comprehensive characterization, annotation and innovative use of Infinium DNA methylation BeadChip probes. Nucleic Acids Res. 2017;45:e22.
- Leek JT, Johnson WE, Parker HS, et al. The sva package for removing batch effects and other unwanted variation in high-throughput experiments. Vol. 28. Oxford: England: Bioinformatics; 2012. p. 882–883.
- Ritchie ME, Phipson B, Wu D, et al. limma powers differential expression analyses for RNA-sequencing and microarray studies. Nucleic Acids Res. 2015;43:e47.
- Phipson B, Maksimovic J, Oshlack A. missMethyl: an R package for analyzing data from Illumina’s HUMANMETHYLATION450 platform. Vol. 32. Oxford: England: Bioinformatics; 2016. p. 286–288.
- Phipson B, Oshlack A. DiffVar: a new method for detecting differential variability with application to methylation in cancer and aging. Genome Biol. 2014;15:465.
- Phipson B, Lee S, Majewski IJ, et al. Robust hyperparameter estimation protects against hypervariable genes and improves power to detect differential expression. Ann Appl Stat. 2016;10:946–963.
- van Iterson M, Cats D, Hop P, et al. omicsPrint: detection of data linkage errors in multiple omics studies. Bioinformatics. 2018;34:2142–2143.
- Devlin B, Roeder K. Genomic control for association studies. Biometrics. 1999;55:997–1004.
- Jaffe AE, Murakami P, Lee H, et al. Bump hunting to identify differentially methylated regions in epigenetic epidemiology studies. Int J Epidemiol. 2012;41:200–209.
- Kuleshov MV, Jones MR, Rouillard AD, et al. Enrichr: a comprehensive gene set enrichment analysis web server 2016 update. Nucleic Acids Res. 2016;44:W90–7.
- Wu MF, Chen ST, Yang AH, et al. CLEC5A is critical for dengue virus-induced inflammasome activation in human macrophages. Blood. 2013;121:95–106.
- Geeleher P, Hartnett L, Egan LJ, et al. Gene-set analysis is severely biased when applied to genome-wide methylation data. Bioinformatics. 2013;29:1851–1857.
- Kim S, Vermeulen R, Waidyanatha S, et al. Using urinary biomarkers to elucidate dose-related patterns of human benzene metabolism. Carcinogenesis. 2006;27:772–781.
- Steinmaus C, Smith AH, Jones RM, et al. Meta-analysis of benzene exposure and non-Hodgkin lymphoma: biases could mask an important association. Occup Environ Med. 2008;65:371–378.
- Hansen KD, Timp W, Bravo HC, et al. Increased methylation variation in epigenetic domains across cancer types. Nat Genet. 2011;43:768–775.
- Teschendorff AE, Widschwendter M. Differential variability improves the identification of cancer risk markers in DNA methylation studies profiling precursor cancer lesions. Bioinformatics. 2012;28:1487–1494.
- Webster AP, Plant D, Ecker S, et al. Increased DNA methylation variability in rheumatoid arthritis-discordant monozygotic twins. Genome Med. 2018;10:64.
- Phillips RV, Rieswijk L, Hubbard AE, et al. Human exposure to trichloroethylene is associated with increased variability of blood DNA methylation that is enriched in genes and pathways related to autoimmune disease and cancer. Epigenetics. 2019;14:1112–1124.
- McHale CM, Zhang L, Lan Q, et al. Changes in the peripheral blood transcriptome associated with occupational benzene exposure identified by cross-comparison on two microarray platforms. Genomics. 2009;93:343–349.
- Hou Y, Ding M, Wang C, et al. TRIM11 promotes lymphomas by activating the beta-catenin signaling and Axin1 ubiquitination degradation. Exp Cell Res. 2020;387:111750.
- Agarwal NK, Zhu X, Gagea M, et al. PHLPP2 suppresses the NF-kappaB pathway by inactivating IKKbeta kinase. Oncotarget. 2014;5:815–823.
- Yan Y, Hanse EA, Stedman K, et al. Transcription factor C/EBP-beta induces tumor-suppressor phosphatase PHLPP2 through repression of the miR-17-92 cluster in differentiating AML cells. Cell Death Differ. 2016;23:1232–1242.
- Kuo YH, Zaidi SK, Gornostaeva S, et al. Runx2 induces acute myeloid leukemia in cooperation with Cbfbeta-SMMHC in mice. Blood. 2009;113:3323–3332.
- Zolghadr F, Sadeghizadeh M, Amirizadeh N, et al. How benzene and its metabolites affect human marrow derived mesenchymal stem cells. Toxicol Lett. 2012;214:145–153.
- Hartmann L, Dutta S, Opatz S, et al. ZBTB7A mutations in acute myeloid leukaemia with t(8;21) translocation. Nat Commun. 2016;7:11733.
- Redondo Monte E, Wilding A, Leubolt G, et al. ZBTB7A prevents RUNX1-RUNX1T1-dependent clonal expansion of human hematopoietic stem and progenitor cells. Oncogene. 2020;39:3195–3205.
- Herzig S, Shaw RJ. AMPK: guardian of metabolism and mitochondrial homeostasis. Nat Rev Mol Cell Biol. 2018;19:121–135.
- Mihaylova MM, Shaw RJ. The AMPK signalling pathway coordinates cell growth, autophagy and metabolism. Nat Cell Biol. 2011;13:1016–1023.
- Greer EL, Dowlatshahi D, Banko MR, et al. An AMPK-FOXO pathway mediates longevity induced by a novel method of dietary restriction in C. elegans. Curr Biol. 2007;17:1646–1656.
- Templeman NM, Murphy CT. Regulation of reproduction and longevity by nutrient-sensing pathways. J Cell Biol. 2018;217:93–106.
- Jacquel A, Luciano F, Robert G, et al. Implication and regulation of AMPK during physiological and pathological myeloid differentiation. Int J Mol Sci. 2018;19:2991.
- Visnjic D, Dembitz V, Lalic H. The role of AMPK/mTOR modulators in the therapy of acute myeloid leukemia. Curr Med Chem. 2019;26:2208–2229.
- Li Z, Wang C, Zhu J, et al. The possible role of liver kinase B1 in hydroquinone-induced toxicity of murine fetal liver and bone marrow hematopoietic stem cells. Environ Toxicol. 2016;31:830–841.
- Patterson KI, Brummer T, O’Brien PM, et al. Dual-specificity phosphatases: critical regulators with diverse cellular targets. Biochem J. 2009;418:475–489.
- Li JP, Yang CY, Chuang HC, et al. The phosphatase JKAP/DUSP22 inhibits T-cell receptor signalling and autoimmunity by inactivating Lck. Nat Commun. 2014;5:3618.
- Huang CY, Tan TH. DUSPs, to MAP kinases and beyond. Cell Biosci. 2012;2:24.
- Li JP, Fu YN, Chen YR, et al. JNK pathway-associated phosphatase dephosphorylates focal adhesion kinase and suppresses cell migration. J Biol Chem. 2010;285:5472–5478.
- Sekine Y, Ikeda O, Hayakawa Y, et al. DUSP22/LMW-DSP2 regulates estrogen receptor-alpha-mediated signaling through dephosphorylation of Ser-118. Oncogene. 2007;26:6038–6049.
- Sekine Y, Tsuji S, Ikeda O, et al. Regulation of STAT3-mediated signaling by LMW-DSP2. Oncogene. 2006;25:5801–5806.
- Chen AJ, Zhou G, Juan T, et al. The dual specificity JKAP specifically activates the c-Jun N-terminal kinase pathway. J Biol Chem. 2002;277:36592–36601.
- Alonso A, Merlo JJ, Na S, et al. Inhibition of T cell antigen receptor signaling by VHR-related MKPX (VHX), a new dual specificity phosphatase related to VH1 related (VHR). J Biol Chem. 2002;277:5524–5528.
- Chuang HC, Tan TH. MAP4K family kinases and DUSP family phosphatases in t-cell signaling and systemic lupus erythematosus. Cells. 2019;8(11):1433. https://doi.org/10.3390/cells8111433.
- Lin HP, Ho HM, Chang CW, et al. DUSP22 suppresses prostate cancer proliferation by targeting the EGFR-AR axis. FASEB J. 2019;33:14653–14667.
- Lim MS, Bailey NG, King RL, et al. Molecular genetics in the diagnosis and biology of lymphoid neoplasms. Am J Clin Pathol. 2019;152:277–301.
- Parrilla Castellar ER, Jaffe ES, Said JW, et al. ALK-negative anaplastic large cell lymphoma is a genetically heterogeneous disease with widely disparate clinical outcomes. Blood. 2014;124:1473–1480.
- Boks MP, Houtepen LC, Xu Z, et al. Genetic vulnerability to DUSP22 promoter hypermethylation is involved in the relation between in utero famine exposure and schizophrenia. NPJ Schizophr. 2018;4:16.
- Mok A, Rhead B, Holingue C, et al. Hypomethylation of CYP2E1 and DUSP22 promoters associated with disease activity and erosive disease among rheumatoid arthritis patients. Arthritis Rheumatol. 2018;70:528–536.
- Rutten BPF, Vermetten E, Vinkers CH, et al. Longitudinal analyses of the DNA methylome in deployed military servicemen identify susceptibility loci for post-traumatic stress disorder. Mol Psychiatry. 2018;23:1145–1156.
- Ouyang B, Baxter CS, Lam HM, et al. Hypomethylation of dual specificity phosphatase 22 promoter correlates with duration of service in firefighters and is inducible by low-dose benzo[a]pyrene. J Occup Environ Med. 2012;54:774–780.
- Miura R, Araki A, Miyashita C, et al. An epigenome-wide study of cord blood DNA methylations in relation to prenatal perfluoroalkyl substance exposure: the Hokkaido study. Environ Int. 2018;115:21–28.
- Rizzo HE, Escaname EN, Alana NB, et al. Maternal diabetes and obesity influence the fetal epigenome in a largely Hispanic population. Clin Epigenetics. 2020;12:34.
- Crooks GM, Fuller J, Petersen D, et al. Constitutive HOXA5 expression inhibits erythropoiesis and increases myelopoiesis from human hematopoietic progenitors. Blood. 1999;94:519–528.
- Fuller JF, McAdara J, Yaron Y, et al. Characterization of HOX gene expression during myelopoiesis: role of HOX A5 in lineage commitment and maturation. Blood. 1999;93:3391–3400.
- Shah N, Sukumar S. The Hox genes and their roles in oncogenesis. Nat Rev Cancer. 2010;10(5):361–371.
- Kim SY, Hwang SH, Song EJ, et al. Level of HOXA5 hypermethylation in acute myeloid leukemia is associated with short-term outcome. Korean J Lab Med. 2010;30:469–473.
- Strathdee G, Holyoake TL, Sim A, et al. Inactivation of HOXA genes by hypermethylation in myeloid and lymphoid malignancy is frequent and associated with poor prognosis. Clin Cancer Res. 2007;13:5048–5055.
- Nagy A, Osz A, Budczies J, et al. Elevated HOX gene expression in acute myeloid leukemia is associated with NPM1 mutations and poor survival. J Adv Res. 2019;20:105–116.
- Musialik E, Bujko M, Kober P, et al. Promoter DNA methylation and expression levels of HOXA4, HOXA5 and MEIS1 in acute myeloid leukemia. Mol Med Rep. 2015;11:3948–3954.
- Godish T. Formaldehyde exposures from tobacco smoke: a review. Am J Public Health. 1989;79:1044–1045.
- Wallace L, Pellizzari E, Hartwell TD, et al. Exposures to benzene and other volatile compounds from active and passive smoking. Arch Environ Health. 1987;42:272–279.
- Jacoby M, Gohrbandt S, Clausse V, et al. Interindividual variability and co-regulation of DNA methylation differ among blood cell populations. Epigenetics. 2012;7:1421–1434.
- Heiss JA, Breitling LP, Lehne B, et al. Training a model for estimating leukocyte composition using whole-blood DNA methylation and cell counts as reference. Epigenomics. 2017;9:13–20.
- Cardenas A, Allard C, Doyon M, et al. Validation of a DNA methylation reference panel for the estimation of nucleated cells types in cord blood. Epigenetics. 2016;11:773–779.