ABSTRACT
Social determinants of health (SDoH) are defined as the conditions in which people are born, grow, live, work, and age. The distribution of these conditions is influenced by underlying structural factors and may be linked to adverse pregnancy outcomes through epigenetic modifications of gestational tissues. A promising modification is epigenetic gestational age (eGA), which captures ‘biological’ age at birth. Measuring eGA in placenta, an organ critical for foetal development, may provide information about how SDoH ‘get under the skin’ during pregnancy to influence birth outcomes and ethnic/racial disparities. We examined relationships of placental eGA with sociodemographic factors, smoking, and two key clinical outcomes: Apgar scores and NICU length of stay. Using the Robust Placental Clock, we estimated eGA for placental samples from the Extremely Low Gestational Age Newborns cohort (N = 408). Regression modelling revealed smoking during pregnancy was associated with placental eGA acceleration (i.e., eGA higher than chronologic gestational age). This association differed by maternal race: among infants born to mothers racialized as Black, we observed greater eGA acceleration (+0.89 week, 95% CI: 0.38, 1.40) as compared to those racialized as white (+0.27 week, 95% CI: −0.06, 0.59). Placental eGA acceleration was also correlated with shorter NICU lengths of stay, but only among infants born to mothers racialized as Black (−0.08 d/week-eGA, 95% CI: −0.12, −0.05). Together, these observed associations suggest that interpretations of epigenetic gestational aging may be tissue-specific.
Background
Social determinants of health (SDoH) are the conditions in which people are born, grow, live, and work [Citation1]. These conditions (e.g., educational attainment, housing, and wealth) are shaped by upstream structural factors including cultural norms, policies, and the distribution of power and resources [Citation1]. Social context also shapes the distribution of environmental hazards, psychosocial stress, and access to and quality of clinical care, and several key SDoH factors are established protective or risk factors for preterm birth [Citation2], which has strong implications for racial/ethnic disparities in adverse pregnancy outcomes. In the United States, the prevalence of preterm birth is higher among mothers racialized as Black (16%), as compared to mothers racialized as white (11%) [Citation3]. This disparity is not fully explained by individual behaviours or genetic differences [Citation4], and one root cause is the unequal distribution of SDoH, environmental hazards, and stressors resulting from structural racism [Citation4]. Sociodemographic factors imperfectly conceptualize SDoH and partially predict preterm birth but are only proxies of true causes that may be linked to preterm birth by influences on biological signalling and epigenetic mechanisms [Citation3,Citation5]. A potential marker of the true biological mechanism is epigenetic age, which is estimated according to algorithms applied to DNA methylation levels at specific cytosine-guanine (CpG) loci.
These algorithms are called ‘epigenetic clocks’ and were developed using machine learning techniques [Citation6]. Epigenetic ages estimated by an epigenetic clock generally correlate with chronological age and are presumed to represent cognitive and physical capacity in an adult or aging population [Citation7]. Current understanding of the influence of in utero conditions on epigenetic aging and the influence of epigenetic aging on infant health is incomplete. Previous studies of the prenatal period highlight links between epigenetic gestational age (eGA) acceleration (i.e., the discrepancy between eGA and chronological gestational age) in cord blood and indicators of prenatal adversity [Citation8], prenatal selective serotonin reuptake inhibitors (SSRI) use [Citation9], birth weight and Medicaid use [Citation10], and anthropometric measures [Citation11]. There is also evidence to suggest that in utero exposures may influence gestational epigenetic aging estimates, as maternal characteristics (i.e., diet, cardiometabolic health, and age) have been shown to establish some portion of the health and aging trajectory of children [Citation12]. In regard to SDoH, maternal stress is a relatively well-accepted risk factor for early gestational age and pre-term birth [Citation13], and various types of stress have been linked to accelerated epigenetic aging in adults and children [Citation14,Citation15]. eGA acceleration in gestational tissue could reflect the biological process whereby SDoH get ‘under the skin’ during pregnancy to influence stress-related pathways, foetal development, and birth outcomes, but additional studies are needed to characterize this relationship [Citation12,Citation16].
To date, studies of eGA acceleration in gestational tissues have primarily examined DNA methylation patterns in umbilical cord blood [Citation10,Citation17–19]. However, the placenta is one of the first organs to develop during pregnancy and serves to exchange hormones, gases, and nutrients between mother and foetus. Since the placenta links maternal and foetal compartments, it is critical to establishing a healthy gestational environment for the foetus [Citation20]. During pregnancy, the placenta senses and responds to changes in the maternal environment [Citation20]. Adversity or stress during pregnancy may be associated with epigenetic changes in the placenta leading to alterations in blood flow, nutrient supply, and hormone secretion that may compromise foetal development [Citation18,Citation20]. Previously, smoking, neighbourhood disadvantage, and indicators of low socioeconomic position have been associated with epigenetic aging in child and adult studies [Citation21–23]. Evidence is less consistent during the prenatal window, where eGA acceleration in cord blood has been associated with maternal experiences of violence but not sociodemographic factors such as income and education [Citation12,Citation16]. While these and other SDoH have been investigated in relation to epigenetic aging in blood and saliva, a gap remains in our understanding of whether SDoH are related to epigenetic aging in placental tissue.
To date, four epigenetic clocks have been developed to estimate eGA using placental tissue [Citation17,Citation18]. The first comprises 62 CpG probes and was developed by Mayne et al. using placental DNA methylation data measured on the 450 K Illumina platform [Citation18]. The clock accurately predicts gestational age with a high correlation between chronological age and eGA (r = 0.99, p-value < 2.2E-16) [Citation18]. However, five of the 62 CpG probes are missing from the 850 K Illumina platform, and loss of probes has been shown to mis-estimate epigenetic age in other epigenetic clocks [Citation17,Citation24]. To ensure the estimation of gestational age would be applicable to data from both platforms, Lee et al. developed three additional placental eGA clocks using autosomal CpG probes shared between the 450 K and 850 K Illumina platforms [Citation17]. Leveraging a robust collection of placental DNA methylation datasets, each clock serves a different purpose: the Robust Placental Clock (RPC) is considered unaffected by pregnancy complications; the Control Placental Clock (CPC) is suitable for uncomplicated pregnancies; and, finally, the refined RPC (rRPC) is suitable for uncomplicated term pregnancies [Citation17].
In this study, we describe placental eGA acceleration in relation to six sociodemographic factors (maternal educational attainment, health insurance status, socioeconomic position, maternal self-reported race) and smoking during pregnancy. We hypothesize that associations may be modified by maternal race given the unequal distribution of SDoH, environmental hazards, and stressors influenced by structural racism [Citation1,Citation5]. Thus, we also contrasted estimates between mothers racialized as Black and white, separately. To estimate eGA, we utilized DNA methylation data from a large placental repository collected from infants born extremely preterm (<28 weeks gestation), a population in which social disadvantage tends to be over-represented [Citation25]. Given their extreme prematurity, we relied on the RPC as this clock was constructed using placentas collected from preterm births and is ‘robust’ to pregnancy complications such as preeclampsia and gestational diabetes that commonly shorten the duration of gestation. Finally, we explored the clinical implications of placental eGA acceleration by examining associations with Apgar scores at delivery and length of stay in the neonatal intensive care unit (NICU). We hypothesized that placental eGA acceleration would be associated with sociodemographic factors, smoking, and clinical outcomes and that estimated associations would vary between racialized groups, given the unequal distribution of SDoH, environmental hazards, and stressors resulting from structural racism. Because epigenetic aging is positively associated with harmful exposures in adults, we postulated that harmful exposures would also lead to accelerated epigenetic gestational aging of placental tissue.
Methods
Participant recruitment and study design
The Extremely Low Gestational Age Newborn (ELGAN) study is a multi-centre cohort designed to evaluate protective and risk factors for brain damage among newborns born prior to 28 weeks of gestation. Participating institutions recruited and enrolled women either shortly after being admitted but before delivering or soon after delivery according to clinical circumstances and/or institutional preference. A total of 1,506 newborns were enrolled in the overall ELGAN cohort between 2002 and 2004 from 14 participating institutions located across five states (Connecticut, Illinois, Massachusetts, Michigan, and North Carolina). Of these, quantification of placental DNA methylation was performed within a subset of 411 newborns for whom sufficient quantities of placental tissue samples were available and who later returned for follow-up assessments at 10 y of age. Institutional review boards at each participating institution approved the study procedures, and each mother provided written informed consent for herself and her newborn.
Gestational age ascertainment
Chronological gestational age was previously estimated using a tiered approach [Citation26]. When available, estimates based on the dates of embryo retrieval, intrauterine insemination, or ultrasound examination before the 14th week of gestation (62%) were deemed highest quality. When this information was not available, estimations were based sequentially on [Citation1] ultrasound examinations at 14 weeks’ or later (29%) [Citation2], menstrual dating without ultrasound confirmation (7%), or [Citation3] gestational age recorded in neonatal intensive care unit records (1%).
Placental sampling
Each infant had a placental sample collected for an initial pathological exam shortly after delivery. Placentas were placed in a sterilized basin and biopsied in a sampling room after delivery generally within one-hour post-partum. The amnion was pulled back to expose the chorion at the midpoint of the longest distance between the cord insertion and edge of the placental disk. For multifetal pregnancies, chorionicity was confirmed histologically. In the case of monochorionic pregnancies, care was taken to distinguish each sibling’s placental share by gross and microscopic evaluation of the dividing membranes. Samples (<1 gram) from the foetal side of the placenta that corresponded to each infant were removed by applying traction to the chorion and underlying trophoblast tissue. The collected specimen was immediately placed in a cryogenic vial and immersed in liquid nitrogen. Samples were frozen and stored at −80°C until shipped to the University of North Carolina at Chapel Hill for processing. There, a 0.2 g subsection of the placental tissue was cut from the frozen biopsy and washed with sterile 1x phosphate-buffered saline (PBS) to remove any remaining blood. Tissues were lysed by homogenizing the subsections with β-mercaptoethanol in Buffer RLT (Qiagen, Valencia CA).
DNA methylation assessment
Methylation profiling was conducted on a subset of placental tissue samples that were sufficient in volume and were randomly selected from liveborn infants who survived to 10 y of age.
This selection criterion was due to neurodevelopmental impairments in childhood being the primary outcome of the parent ELGAN ‘omics study; thus, high-throughput profiling was only conducted in children that survived to the age of 10 y. As such, the present work is a secondary analysis of available data.
DNA sequences >18 nucleotides long were isolated from the homogenized placental tissue samples using ALLPrep DNA/RNA/miRNA Universal Kit (Qiagen, Valencia, CA). To perform epigenome-wide DNA methylation measurements, the extracted DNA was bisulphite-converted using the EZ DNA Methylation Kit (Zymo Research, Irvine, CA) followed by quantification using the Illumina Infinium MethylationEPIC BeadChip (Illumina, San Diego, CA) to profile methylation status at more than 850,000 CpG sites across the genome. Functional normalization was performed using normal-exponential out-of-band (noob) correction method for background subtraction and dye normalization [Citation27], followed by the typical functional normalization [Citation28,Citation29]. To evaluate potential batch effects, we performed a principal component analysis (PCA).
Plate and chip position were identified as a significant source of variation; thus, we corrected the data using the ComBat function from the sva R package [Citation30]. Average methylation values (β- values) were computed to represent the ratio of methylated to unmethylated signal intensities.
Estimating placental cell composition
Proportions of the placental cell types were estimated via the R package planet, which uses a reference-based deconvolution method [Citation31]. The method was developed by characterizing methylation of placentas that were collected from terminated and healthy term pregnancies and therefore is specific to samples collected during either the first or third trimester. For our extremely preterm placentas (<28 weeks gestation), we specified our samples as both first and third trimester, then averaged across the resulting cell-type proportions per the planet authors’ recommendations (V. Yuan and W. Robinson, personal communication, 17 February 2021).
Estimating placental eGA using the robust placental clock (RPC)
Placental eGA was calculated using the RPC, which combines placental DNA methylation β- values from 558 CpG sites [Citation17]. This clock was selected for the estimation of epigenetic gestational age because it was constructed from placentas collected from pregnancies ranging from 5 to 42 weeks’ gestation and is robust to pregnancy complications such as preeclampsia. Despite the inclusion of preterm birth in its construction, predicted and chronological age were highly correlated (r = 0.99) in the original manuscript describing the RPC. Sites comprising the RPC were originally selected using a penalized elastic net regression model to predict chronological gestational age with high accuracy [Citation17]. To derive eGA acceleration, we fit a linear regression model of predicted eGA on chronological gestational age and extracted the model residuals. Compared to chronological gestational age, positive values represent accelerated eGA whereas negative values represent decelerated eGA. Three samples with RPC-estimated age acceleration residuals greater than three standard deviations from the mean were excluded as potential outliers (Additional File 1). This resulted in the final inclusion of 408 mother-infant pairs ().
Figure 1. Cohort construction of the 411 mother-infant pairs with placental epigenetic data, outlying observations (N = 3), where epigenetic Gestational Age (eGA) acceleration estimates were greater than three standard deviations from the mean, were excluded. The remaining 408 mother-infant pairs, included in the present analysis, had primarily white (N = 246) and Black (N = 115) mothers.
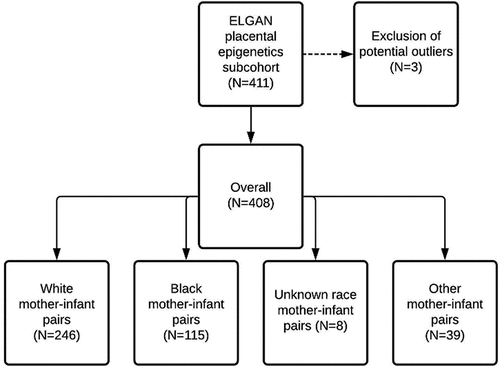
Construction of SDoH variables
Six SDoH or sociodemographic factors were evaluated in relation to eGA acceleration. These variables included educational attainment, health insurance status, sources of income, a composite indicator of socioeconomic position (SEP), self-reported race, and smoking during pregnancy.
Race
Self-reported races included white (N = 246), Black (N = 115), Asian (N = 5), Native American (N = 4), mixed (N = 14), and other (N = 16). Though considerable and important differences exist between each of these racial groups, to avoid data sparseness, women self-identifying as Asian, Native American, mixed, and other were grouped as ‘other.’ The final coding scheme included the following categories: (a) white, (b) Black, and (c) other.
Education
Educational attainment was self-reported as <12 y (less than high school diploma), 12–16 y (graduated high school to some college), and 16+ y (post-graduate education).
Health insurance
Participants self-reported health insurance status as public, private, self-pay, other, and/or no insurance. Several mothers reported multiple forms of health insurance (e.g., self-pay and private insurance coverage). In those cases, we re-coded the variable to reflect the category most strongly associated with economic hardship: self-pay and private insurance coverage (N = 1) was coded as self-pay; private and public insurance coverage (N = 10) was coded as publicly insured; private and other insurance coverage (N = 1) was coded as privately insured; and public and other insurance coverage (N = 2) was coded as publicly insured. We grouped private insurance (N = 243) and other (N = 4) together to avoid data sparseness. Similarly, we grouped participants who reported the use of self-pay (N = 6) or no insurance (N = 6). In a sensitivity analysis, we used educational attainment to determine how to categorize mothers reporting multiple forms of health insurance. Using this approach, only mothers with less than 12 y of education were assigned to the category associated with economic hardship, and self-paying and uninsured women were categorized separately. Given that this approach did not change our findings, the initial approach was used, and the final coding scheme included the following categories [Citation1]: private/other [Citation2], public, or [Citation3] uninsured.
Smoking during pregnancy
Smoking during pregnancy was self-reported as active (N = 41) and/or passive (N = 75); the remaining reported no smoking exposure (N = 306). The active smoking during pregnancy category included all women reporting active cigarette smoking during pregnancy, regardless of passive exposure. The passive smoking during pregnancy category was re-coded to include women who reported passive exposure to cigarette smoke only (N = 49).
Socioeconomic position
SEP was constructed by combining educational attainment, insurance status, income, and marital status. Specifically, educational attainment above 12 y (yes = 1, no = 0), private or other health insurance (yes = 1, no = 0), non-reliance on public assistance (yes = 1, no = 0), and marital status (cohabitating or married = 1, single = 0) were combined. A version of this variable has been used in a previous analysis of the ELGAN cohort and was found to be predictive of altered placental DNA methylation [Citation32]. This summative score was analysed as a categorical variable, ranging from 0 to 4, with higher scores indicative of a higher SEP. SEP was also evaluated as a continuous variable for comparison (Additional file 2).
Clinical variables
Given the various aetiologies of preterm delivery, the indication for preterm delivery was abstracted from medical records and classified as follows: preterm labour, preterm premature rupture of membranes, preeclampsia, abruption, cervical insufficiency, and foetal indication [Citation26]. Apgar scores, a standardized scoring system used to assess infants after delivery, were obtained from medical records by trained research nurses. The scores, which are measured at 1- and 5-minutes following delivery, are comprised of five components: 1) colour, 2) heart rate, 3) reflexes, 4) muscle tone, and 5) respiration, each of which is given a score of 0, 1, or 2 for a total possible score of 10 [Citation33]. As such, the Apgar score quantitates clinical signs of cyanosis or pallor, bradycardia, depressed reflex response to stimulation, hypotonia, and apnoea or gasping respirations, with higher scores, particularly those at 5 minutes, reflecting more optimal clinical condition. Lengths of stay in the NICU were calculated as the difference between the discharge and delivery dates recorded in the infant’s medical record.
Statistical analysis
Welch Two-Sample T-tests (for marital status) and ANOVA tests (for all other variables) were performed to compare mean RPC-estimated eGA acceleration (weeks) by levels of each sociodemographic factor, first overall and then among each racialized group separately. Given that there are multiple aetiologies of preterm delivery, mean RPC-estimated eGA acceleration was also compared between indicators of preterm birth (i.e., preterm labour, preterm premature rupture of membranes, preeclampsia, abruption, CI, and FI) using a chi-squared test. To evaluate associations with eGA acceleration, we fit separate linear mixed effects models for maternal race (white, Black, other), educational attainment (<12, 12–16, 16+ y), health insurance status (private/other, public, uninsured), smoking during pregnancy (active, passive only, none), source of income (self-employment, familial or other support, public assistance), and a composite indicator of SEP (0, 1, 2, or 3). Fitting mixed effects models with random intercepts allowed us to account for correlations within multiple sets (i.e., twins or triplets). The general model was as follows:
(RPC age accel)ij = α + Xij β + μj + ei
where (RPC age accel)ij is the measure of RPC-estimated placental eGA acceleration for each infant (i) relative to each sociodemographic factor. Xij is the level of the respective sociodemographic factor, μ j is a random intercept used to account for correlations among infants born to the same mother (j), α is the overall y-intercept, and ei is the error term. Each SDoH predictor variable was tested separately. Due to evidence of persisting racial disparities in birth outcomes between white and Black women while controlling for SEP [Citation4], models were fit [Citation1] among the overall cohort and [Citation2] among racialized groups separately. Models including [Citation1] smoking and [Citation2] smoking and cell-type proportions as additional covariates were also fit for sensitivity analyses.
Results
Cohort demographics
The present study evaluated 408 placentas () collected from infants born extremely preterm, defined as completing less than 28 weeks of gestation (; see Additional file 1). The average maternal age and pre-pregnancy body mass index (BMI) were 29 y and 25.5 kg/m2, respectively. Most mothers self-identified as white (60.3%), completed 12–16 y of education (49.0%), and reported having private or other health insurance (62.1%). Most pregnancies were delivered at 25 weeks on average. The mean infant birth weight was 829.9 grams, and most infants were singletons (58.6%). Most mothers reporting neither active nor passive smoking during pregnancy (75.0%). The source of income was primarily reported as ‘own employment’ (57.4%), though nearly 1 in 4 relied on some form of public assistance (e.g., Special Supplemental Nutrition Program for Woman, Infants, Children (WIC); survivor’s disability). Nearly half (45.3%) of the mothers had a composite indicator of socioeconomic position (SEP) score of 4, indicating education beyond high school, private or other insurance coverage, non-reliance on public assistance, and cohabitation or marriage with a partner. The distributions of some sociodemographic factors also varied between racialized groups (). Women with the highest level of educational attainment were mostly privately insured, in the highest SEP category, non-smokers, and married or co-habitating. Privately insured women were most likely in the highest SEP category, married, and non-smokers. Women in the highest SEP category were most likely to be married, non-smokers. And finally, most active smokers were single women. Mean RPC-estimated eGA acceleration is also described within strata of each sociodemographic factor overall and among each racialized group (). The distributions of some variables varied between racialized groups very modestly. The mean RPC-estimated eGA acceleration did not vary significantly between strata of any sociodemographic factors, smoking, or clinical variables (Additional file 3).
Table 1. Selected baseline characteristics of study population (N = 408), Extremely Low Gestational Aged Newborns (ELGAN). ELGAN study participants were recruited from one of 14 participating sites before 28 weeks gestation (2002–2004).
Table 2. Distribution of RPC-estimated eGA acceleration (weeks) in relation to sociodemographic factors. Distributions are summarized overall and across white and black race. P-values calculated from Welch Two-Sample T-tests (marital status) and ANOVA tests (other variables).
Description of epigenetic gestational age (eGA) overall
Overall, the correlation between RPC-estimated placental eGA and chronological gestational age estimated from clinical observations was 0.61 (p < 0.001; ). The median absolute error (MAE), defined as the absolute difference between predicted and observed gestational age, was 2.02 weeks (). All mothers delivered infants before 28 weeks gestation per study inclusion criteria, and mean gestational age did not vary significantly according to indication of preterm delivery (i.e., preterm labour, preterm premature rupture of membrances, preeclampsia, abruption, cervical insufficiency, or foetal indication); chi-squared p-value: 0.46).
Figure 2. Relationship between Robust Placental Clock (RPC)-estimated placental epigenetic Gestational Age (eGA) and chronological gestational age. Mean absolute error (MAE) represents the median absolute difference between predicted and observed gestational age, and r and p are the Spearman correlation coefficient and p-value, respectively, of the association between observed gestational age and predicted eGA.
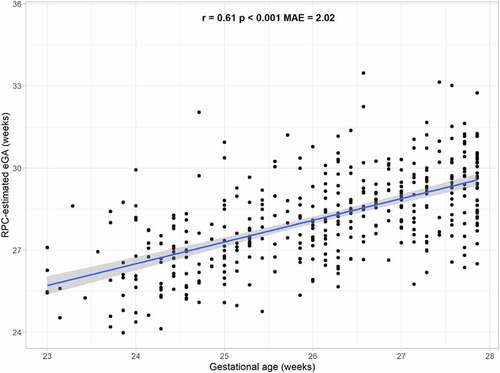
Placental eGA acceleration was associated with smoking during pregnancy
In unadjusted models evaluating crude associations with social factors, only smoking during pregnancy was associated with placental eGA acceleration (). Overall dose–response relationships were identified; some factors associated with a higher socioeconomic position were also associated with lower mean placental eGA acceleration, albeit insignificantly.
Table 3. Mean difference in RPC-estimated eGA acceleration (weeks) in relation to sociodemographic factors. Mean differences represent those observed overall and according to white and Black race.
Prenatal smoking alone or in combination with passive smoke exposure was associated with a +0.50-week (95% CI: 0.23, 0.77) accelerated placental eGA, as compared to no exposure to smoking during pregnancy (). This association was primarily observed among Mothers racialized as Black who experienced a + 0.89 week (95% CI: 0.38, 1.40) accelerated placental eGA but was not observed among mothers racialized as white (+0.27 weeks, 95% CI: −0.06, 0.59). Passive smoke exposure was not associated with differences in placental eGA acceleration. No other sociodemographic factors were associated with placental eGA acceleration ().
Figure 3. Distribution of epigenetic Gestational Age (eGA) acceleration according to smoking status (a) overall and across mothers racialized as (b) Black and (c) white. Active or active + passive smoking was associated with a mean +0.50-week (95% CI: 0.23 to 0.77) and +0.89-week (95% CI: 0.38 to 1.40) accelerated placental eGA overall and among Mothers racialized as Black, respectively.
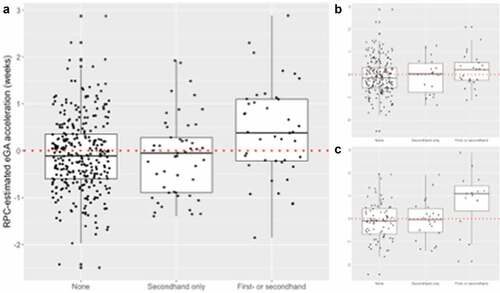
Findings were generally robust to the incorporation of smoking and cell-type proportions as covariates (Additional file 4). Controlling for smoking in models evaluating the association between SDoH or sociodemographic factors and eGA acceleration attenuated mean differences. Similarly, most mean differences were attenuated after additionally controlling for cell-type proportions. The association between smoking and eGA acceleration remained significant after correcting for cell-type proportions. Correction for cell-type proportions attenuated the association to a + 0.28-weeks (95% CI: 0.04, 0.51) mean difference.
Placental eGA acceleration was associated with shorter lengths of stay in the NICU
Finally, unadjusted models were fit to evaluate crude associations between both chronological GA and placental eGA acceleration with clinical outcomes (). Although chronological GA was strongly associated with higher Apgar scores, placental eGA acceleration was not associated with Apgar scores measured at 1- and 5-minutes following delivery, overall nor among each racialized group.
Table 4. Associations between chronological gestational age and RPC-estimated epigenetic age acceleration with clinical outcomes. Associations represent those observed overall and according to white and Black race.
In contrast, both chronological GA and placental eGA acceleration were associated with shorter lengths of stay in the NICU. Specifically, a 1-week increase in chronological GA was associated with 0.17 fewer days in the NICU (95% CI: −0.20, −0.14), which was consistent across racialized groups. Placental eGA acceleration was also associated with shorter NICU lengths of stay, but the association was only observed among infants born to Mothers racialized as Black, for whom a 1-week acceleration was associated with 0.08 (95% CI: −0.12, −0.05) fewer days in the NICU; smoking and NICU stay were not related (Additional file 5).
Discussion
The prenatal period is a sensitive window in which epigenetic mechanisms underlying foetal development can be perturbed by social and environmental factors [Citation34,Citation35]. Understanding how SDoH are biologically embedded to influence foetal development is a essential component in understanding the developmental origins of health and disease throughout the lifecourse. Thus, we aimed to describe the association between eGA and sociodemographic factors in placenta tissue. In line with current eGA literature, we observed counterintuitive findings, where both smoking, a harmful exposure, and shorter length of NICU stay, a positive outcome, were positively associated with epigenetic aging in our study population. Smoking-related eGA acceleration was higher in placentas collected from mothers racialized as Black; because race is socially constructed, this finding may reflect modification by the impact of factors associated with self-reported race as drivers of racial disparities in birth outcomes. While epigenetic age acceleration is associated with worse health outcomes and higher all-cause mortality among adults, the significance of placental eGA acceleration is less clear [Citation36]. In this population of extremely preterm infants, we found that placental eGA was associated with shorter lengths of stay in the NICU only among infants of mothers racialized as Black.
No sociodemographic factors were associated with placental eGA. In contrast, smoking was associated with ‘biological’ aging of placental tissues ranging from a few days to nearly one week. The exact mechanism through which smoking exerts effects on preterm birth is unclear, but foetal hypoxia, altered prostaglandin synthesis, and nicotine-induced reductions in uteroplacental blood flow are among those proposed [Citation37]. Extensive alterations in DNA methylation have also been consistently observed in relation to smoking, representing a plausible mechanism for the association between smoking and placental eGA acceleration [Citation38]. After stratification, the significant association between smoking and placental eGA acceleration was only conserved among placentas collected from mothers racialized as Black. The difference in the number of smokers among mothers racialized as Black (13%) and those racialized as white (10%) is negligible. Modification by self-reported race is more likely attributable to the unequal distribution of SDoH, environmental hazards, and stress related to longstanding structural racism in the United States. Women racialized as Black experience greater perceived stress than those racialized as white, which can potentiate toxic environmental exposures or independently lead to adverse birth outcomes [Citation4]. Stress and additional factors tracking with self-reported race (e.g., neighbourhood quality, environmental injustice, personal care practices) also influence epigenetic patterning and birth outcomes [Citation39] but are not measured in this study.
A one-week increase in eGA was associated with a 0.08-d shorter stay in the NICU for Black infants. While a 0.08-d (approximately 2 hours) decrease may not be clinically meaningful, it is consistent with the approximately 4 hour decrease observed in a previous study of the relationship between eGA acceleration and indicators of respiratory morbidity. In 143 extremely preterm infants, greater eGA acceleration, as measured in blood spots, was associated with better clinical health outcomes, including lower surfactant or postnatal corticosteroids use, fewer days of assisted ventilation, and less development of bronchopulmonary dysplasia [Citation40]. It is unclear why eGA acceleration was only protective against longer NICU stays among infants born to mothers racialized as Black, however, in the ELGAN study, placentas were profiled among children who survived to age 10 and those not lost to follow up. Differences in the association between eGA acceleration and length of NICU stay between groups could be an artefact of survival-related selection bias, where unmeasured factors (e.g., those related to socioeconomic position) influencing sample selection create a racial difference in our sample that may not reflect the target population [Citation41], or from the racialized social context that impacts the delivery and quality of health care [Citation1]. Interestingly, Apgar scores were not associated with eGA acceleration, even though a strong relationship between Apgar scores and chronological gestational age is well documented in the literature and replicated in our cohort [Citation42]. Given that eGA acceleration was associated both with smoking (a preterm birth risk factor) and slightly shorter NICU stays among Black infants in the ELGAN cohort, the utility of eGA as a clinical marker of either improved outcomes, of social disadvantages, or of developmental maturity is not clear-cut in this work.
A recent comparison of eGA as estimated in chorionic villi, cord blood and placenta (using tissue-specific epigenetic clocks) found dissonant estimates of eGA within the same neonate; this disparity in eGA acceleration suggests that eGA may reflect tissue-specific processes rather than the status of the neonate or their health sequelae [Citation43]. Given the relationship between other biological markers of aging and parturition, the rate of eGA acceleration (or deceleration) measured in placenta may better reflect the ‘countdown’ to parturition, as opposed to gestational aging in the foetus. Additional biomarkers accurately reflecting chronological age include telomere shortening and corticotropin-releasing hormone (CRH) concentrations. These biomarkers are associated with timing of parturition [Citation44–46].
Among adults, telomere shortening is an important determinant of aging, senescence, and death, but progressive telomere shortening in placenta is hypothesized to lead to apoptosis and cell-free foetal DNA (cffDNA) release [Citation47]. Together, these processes stimulate proinflammatory signalling and promote the progression of parturition under the cffDNA/telomere hypothesis [Citation47,Citation48].
Secretions of CRH are also hypothesized to regulate the duration of pregnancy [Citation49]. The relationship between CRH release and parturition is so strong that CRH concentrations as early as 16–20 weeks have been used to identify women destined to delivery early-, full, or post-term [Citation49,Citation50]. Notably, the hypothalamic-pituitary-adrenocortical (HPA axis) stress response – linked to social stressors [Citation36,Citation51,Citation52] – also induces CRH release. Similarly, in the present study being uninsured or completing less than 12 y of education were positively associated with placental eGA acceleration, albeit insignificantly. eGA acceleration in the placenta could reflect countdown to parturition, as opposed to senescence and aging, resulting from biological responses to stress.
This study is not without limitations. First, the Extremely Low Gestational Age Newborns (ELGAN) cohort is composed of infants born extremely preterm (<28 weeks) and findings are not generalizable to full-term populations. However, the estimation of gestational age based on the RPC represents a strength of this study, as it was developed to be robust to pregnancy complications. Additionally, estimated gestational age based on RPC was correlated with actual gestational age (r = 0.61, median absolute error = 2.02 weeks). Still, the analysis of a subset of placentas collected from children who survived to age 10 represents an additional source of bias as some participants were excluded due to losses to follow-up or early demise.
Second, Lee et al. were unable to consider the effect of ethnic differences on epigenetic gestational age acceleration estimates, as the test data did not include ethnic information [Citation17]. The lack of diversity in the racial/ethnic composition of the samples used to build the RPC limits generalizability to non-white populations. Third, given that many of the sociodemographic factors evaluated are also linked to preterm birth, the study population is enriched for participants who are socially disadvantaged by design. This will limit generalizability to other populations and may lead to overestimating the association between social factors and epigenetic aging in a liveborn population of all gestational ages. Fourth, the factors measured in this study imperfectly capture social determinants of health. While there are better indicators of SEP (e.g., wealth), it is notable that we have previously identified differential methylation of CpG sites in relation to maternal SEP using these variables [Citation32]. Fifth, the ELGAN study did not collect certain factors such as income or substance use during pregnancy that would have been informative to study as additional measures of SDoH or as intermediates linking SDoH and placental aging. Sixth, we decided against correcting for multiple tests to avoid over-penalizing potentially important findings, as described by Rothman et al. [Citation53]. However, in the present analysis, each model asks a distinct question about the association between a unique sociodemographic factor and epigenetic gestational age acceleration and correction for multiple tests is not warranted. Finally, it is unclear whether epigenetic markers of placental aging might represent mediators or byproducts of the relationship between risk factors and preterm delivery.
Conclusions
Every week of gestation is important to foetal development, and even a 1–2 week difference in gestational age at birth can influence health outcomes [Citation54]. In the present study, maternal smoking, an established risk factor for preterm birth, was associated with positive placental eGA acceleration. Smoking-related eGA acceleration was higher in placentas collected from mothers racialized as Black, but because race is socially constructed, this finding may reflect modification by the impact of factors associated with self-reported race as drivers of racial disparities in birth outcomes. Epigenetic age acceleration is associated with worse health outcomes and higher all-cause mortality among adults, but the significance of placental eGA acceleration is less clear [Citation55]. In this population of extremely preterm infants, we found that placental eGA was associated with shorter lengths of stay in the NICU, but only among infants born to mothers racialized as Black. A growing body of literature supports that progressive telomere shortening and CRH concentrations may regulate the progression of parturition [Citation44].
Positive eGA acceleration could reflect these or a similar process, which would explain the placental eGA acceleration observed in relation to smoking. Moving forward, it will be important to evaluate placental eGA acceleration in full-term pregnancies and in relation to validated measurements of SDoH and sociodemographic factors.
List of abbreviations
SDOH social determinants of health
eGA epigenetic gestational age
SEP socioeconomic position
PCA principal component analysis
CpG cytosine-guanine dinucleotide
RPC robust placental clock
CPC control placental clock
rRPC refined robust placental clock
BMI body mass index
WIC Special Supplemental Nutrition Program for Woman, Infants, Children
MAE median absolute error
95% CI 95% confidence interval
cffDNA cell-free fetal DNA
HPA axis hypothalamic-pituitary-adrenocortical axis
CRH corticotropin-releasing hormone
ELGAN Extremely Low Gestational Age Newborns cohort
NICU neonatal intensive care unit
Authors’ contributions
RD and CB conceived the analysis plan. JC analyzed and interpreted the data regarding the association between placental eGA acceleration and sociodemographic factors. JC wrote the manuscript under the guidance of CB, CM, and RD. CB generated placental epigenetic gestational age (eGA) estimates in the study population. HS and KR processed the high-throughput placental methylation data. LS oversaw laboratory processing of placental samples. RF and TMO oversee the ELGAN cohort and development of high throughput datasets. All authors read, revised, and approved the final manuscript.
Supplemental Material
Download Zip (536.6 KB)Disclosure statement
No potential conflict of interest was reported by the author(s).
Data availability statement
Clinical or survey data except for outcomes at 15 y are available by completing the NINDS Data Request Form, which is available from Archived Clinical Research Datasets (https://www.ninds.nih.gov/sites/default/files/sig_form_revised_508c.pdf) and sending the completed form to [email protected]. The 15 y data can be obtained when the NIH ECHO Program releases a public use dataset, which is anticipated in 2022. DNA methylation data (GSE167885) for the ELGAN cohort is hosted on the Gene Expression Omnibus at the National Center for Biotechnology Information (https://www.ncbi.nlm.nih.gov/).
Supplementary material
Supplemental data for this article can be accessed online at https://doi.org/10.1080/15592294.2022.2125717.
Additional information
Funding
References
- Crear-Perry J, Correa-de-Araujo R, Lewis Johnson T, et al. Social and structural determinants of health inequities in maternal health. J Women’s Health. 2021;30(2):230–235.
- Bangma JT, Kwiatkowski E, Psioda M, et al. Assessing positive child health among individuals born extremely preterm. J Pediatr. 2018;202:44–9 e4.
- Burris HH, Baccarelli AA, Wright RO, et al. Epigenetics: linking social and environmental exposures to preterm birth. Pediatr Res. 2016;79(1–2):136–140.
- Burris HH, Hacker MR. Birth outcome racial disparities: a result of intersecting social and environmental factors. Semin Perinatol. 2017;41(6):360–366.
- Burris HH, Wright CJ, Kirpalani H, et al. The promise and pitfalls of precision medicine to resolve black–white racial disparities in preterm birth. Pediatr Res. 2019;87(2):221–226.
- Horvath S. DNA methylation age of human tissues and cell types. Genome Biol. 2013;14(10):R115.
- Levine ME, Lu AT, Quach A, et al. An epigenetic biomarker of aging for lifespan and healthspan. Aging (Albany NY). 2018;10(4):573–591.
- Palma-Gudiel H, Eixarch E, Crispi F, et al. Prenatal adverse environment is associated with epigenetic age deceleration at birth and hypomethylation at the hypoxia-responsive EP300 gene. Clin Epigenetics. 2019;11(1):73.
- McKenna BG, Hendrix CL, Brennan PA, et al. Maternal prenatal depression and epigenetic age deceleration: testing potentially confounding effects of prenatal stress and SSRI use. Epigenetics. 2021;16(3):327–337.
- Knight AK, Craig JM, Theda C, et al. An epigenetic clock for gestational age at birth based on blood methylation data. Genome Biol. 2016;17(1):206.
- Simpkin AJ, Howe LD, Tilling K, et al. The epigenetic clock and physical development during childhood and adolescence: longitudinal analysis from a UK birth cohort. Int J Epidemiol. 2017;46(2):549–558.
- Dhingra R, Nwanaji-Enwerem JC, Aiello A, et al. Epigenetics, aging and early life. Environ Epigenet Toxicol Public Health. 2020;22:239–263.
- Bussières E-L, Tarabulsy GM, Pearson J, et al. Maternal prenatal stress and infant birth weight and gestational age: a meta-analysis of prospective studies. Dev Rev. 2015;36:179–199.
- Zannas AS, Arloth J, Carrillo-Roa T, et al. Lifetime stress accelerates epigenetic aging in an urban, African American cohort: relevance of glucocorticoid signaling. Genome Biol. 2015;16(1):266.
- Jovanovic T, Vance LA, Cross D, et al. Exposure to violence accelerates epigenetic aging in children. Sci Rep. 2017;7(1):8962.
- Martin CL, Ghastine L, Lodge EK, et al. Understanding health inequalities through the lens of social epigenetics. Annu Rev Public Health. 2022;43(1):235–254.
- Lee Y, Choufani S, Weksberg R, et al. Placental epigenetic clocks: estimating gestational age using placental DNA methylation levels. Aging (Albany NY). 2019;11(12):4238–4253.
- Mayne BT, Leemaqz SY, Smith AK, et al. Accelerated placental aging in early onset preeclampsia pregnancies identified by DNA methylation. Epigenomics. 2017;9(3):279–289.
- Bohlin J, Haberg SE, Magnus P, et al. Prediction of gestational age based on genome-wide differentially methylated regions. Genome Biol. 2016;17(1):207.
- Dimasuay KG, Boeuf P, Powell TL, et al. Placental responses to changes in the maternal environment determine fetal growth. Front Physiol. 2016;7:12.
- Lunagómez LS, Santiago-Roque I, Gheno-Heredia YA, et al. Teratogenic effects of Bocconia frutescens L. J Dev Orig Health Dis 2020 1–9. DOI:10.1017/S2040174419000461
- Ward-Caviness CK, Pu S, Martin CL, et al. Epigenetic predictors of all-cause mortality are associated with objective measures of neighborhood disadvantage in an urban population. Clin Epigenetics. 2020;12(1):44.
- Simpkin AJ, Hemani G, Suderman M, et al. Prenatal and early life influences on epigenetic age in children: a study of mother–offspring pairs from two cohort studies. Hum Mol Genet. 2016;25(1):191–201.
- Dhingra R, Kwee LC, Diaz-Sanchez D, et al. Evaluating DNA methylation age on the illumina methylationepic bead chip. PLoS One. 2019;14(4):e0207834.
- Blumenshine P, Egerter S, Barclay CJ, et al. Socioeconomic disparities in adverse birth outcomes: a systematic review. Am J Prev Med. 2010;39(3):263–272.
- McElrath TF, Hecht JL, Dammann O, et al. Pregnancy disorders that lead to delivery before the 28th week of gestation: an epidemiologic approach to classification. Am J Epidemiol. 2008;168(9):980–989.
- Triche TJ Jr., Weisenberger DJ, Van Den Berg D, et al. Low-level processing of illumina infinium DNA methylation beadarrays. Nucleic Acids Res. 2013;41(7):e90.
- Fortin J-P, Triche TJ Jr., Hansen KD. Preprocessing, normalization and integration of the Illumina HumanMethylationEPIC array with minfi. Bioinformatics. 2017;33(4):558–560.
- Fortin J-P, Labbe A, Lemire M, et al. Functional normalization of 450k methylation array data improves replication in large cancer studies. Genome Biol. 2014;15(11):503.
- Leek JT, Johnson WE, Parker HS, et al. The sva package for removing batch effects and other unwanted variation in high-throughput experiments. Bioinformatics. 2012;28(6):882–883.
- Yuan V, Hui D, Yin Y, et al. Cell-specific characterization of the placental methylome. BMC Genomics. 2021;22(1):6.
- Santos HP Jr., Bhattacharya A, Martin EM, et al. Epigenome-wide DNA methylation in placentas from preterm infants: association with maternal socioeconomic status. Epigenetics. 2019;14(8):751–765.
- Apgar V. A Proposal for a New Method of Evaluation of the Newborn Infant. Anesthesia Analg. 1953;32(1):260–267.
- Perera F, Herbstman J. Prenatal environmental exposures, epigenetics, and disease. Reprod Toxicol. 2011;31(3):363–373.
- Loomans EM, van Dijk AE, Vrijkotte TG, et al. Psychosocial stress during pregnancy is related to adverse birth outcomes: results from a large multi-ethnic community-based birth cohort. Eur J Public Health. 2013;23(3):485–491.
- Monk C, Feng T, Lee S, et al. Distress during pregnancy: epigenetic regulation of placenta glucocorticoid-related genes and fetal neurobehavior. Am J Psychiatry. 2016;173(7):705–713.
- Ion R, Bernal AL. Smoking and preterm birth. Reprod Sci. 2015;22(8):918–926.
- Rogers JM. Smoking and pregnancy: epigenetics and developmental origins of the metabolic syndrome. Birth Defects Res. 2019;111(17):1259–1269.
- Burris HH, Wright CJ, Kirpalani H, et al. The promise and pitfalls of precision medicine to resolve black-white racial disparities in preterm birth. Pediatr Res. 2020;87(2):221–226.
- Knight AK, Smith AK, Conneely KN, et al. Relationship between epigenetic maturity and respiratory morbidity in preterm infants. J Pediatr. 2018;198:168–73 e2.
- Howe CJ, Robinson WR. Survival-related selection bias in studies of racial health disparities. Epidemiology. 2018;29(4):521–524.
- Catlin EA, Carpenter MW, BSt B, et al. The Apgar score revisited: influence of gestational age. J Pediatr. 1986;109(5):865–868.
- Dieckmann L, Lahti-Pulkkinen M, Kvist T, et al. Characteristics of epigenetic aging across gestational and perinatal tissues. Clin Epigenetics. 2021;13(1):97.
- Smith R, Nicholson RC. Corticotrophin releasing hormone and the timing of birth. Front Biosci. 2007;12:912–918.
- Jylhava J, Pedersen NL, Hagg S. Biological age predictors. EBioMedicine. 2017;21:29–36.
- Cherkas LF, Aviv A, Valdes AM, et al. The effects of social status on biological aging as measured by white-blood-cell telomere length. Aging Cell. 2006;5(5):361–365.
- Phillippe M, Phillippe SM. Birth and death: evidence for the same biologic clock. Am J Reprod Immunol. 2017;77(5):e12638.
- Phillippe M, and Cell-ree fetal DNA, telomeres, and the spontaneous onset of parturition. Reprod Sci. 2015;22(10):1186–1201.
- McLean M, Bisits A, Davies J, et al. A placental clock controlling the length of human pregnancy. Nat Med. 1995;1(5):460–463.
- Sandman CA, Glynn L, Schetter CD, et al. Elevated maternal cortisol early in pregnancy predicts third trimester levels of placental corticotropin releasing hormone (CRH): priming the placental clock. Peptides. 2006;27(6):1457–1463.
- Herman JP, McKlveen JM, Ghosal S, et al. Regulation of the Hypothalamic-Pituitary-Adrenocortical Stress Response. Compr Physiol. 2016;6(2):603–621.
- Kertes DA, Kamin HS, Hughes DA, et al. Prenatal maternal stress predicts methylation of genes regulating the hypothalamic-pituitary- adrenocortical system in mothers and newborns in the democratic Republic of Congo. Child Dev. 2016;87(1):61–72.
- Rothman KJ. No adjustments are needed for multiple comparisons. Epidemiology. 1990;1(1):43–46.
- Joseph RM, O’Shea TM, Allred EN, et al. Neurocognitive and academic outcomes at age 10 years of extremely preterm newborns. Pediatrics. 2016;137(4):e20154343.
- Fransquet PD, Wrigglesworth J, Woods RL, et al. The epigenetic clock as a predictor of disease and mortality risk: a systematic review and meta-analysis. Clin Epigenetics. 2019;11(1):62.