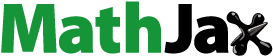
ABSTRACT
The global dynamics in a variety of biological processes can be revealed by mapping transcriptional m6A sites, in particular full-transcriptome m6A. And individual m6A sites have contributed to biological function, which can be evaluated by stoichiometric information obtained from the single nucleotide resolution. Currently, the identification of m6A sites is mainly carried out by experiment and prediction methods, based on high-throughput sequencing and machine learning model respectively. This review summarizes the recent topics and progress made in bioinformatics methods of deciphering the m6A methylation, including the experimental detection of m6A methylation sites, techniques of data analysis, the way of predicting m6A methylation sites, m6A methylation databases, and detection of m6A modification in circRNA. At the end, the essay makes a brief discussion for the development perspective in this area.
Introduction
The term ‘epigenetics’ was coined by Waddington in 1942. It was used to define the study to discover the mechanism of the correlation between phenotypes and genotypes and the relationship of this mechanism to the mechanics of development revealed by experimental embryology [Citation1]. However, Waddington was unable to explain the molecular processes associated with genes, as it took two years for Avery to identify DNA as genetic material [Citation2]. Over the last 80 years, with revolutionary findings of molecular mechanisms of epigenetic control [Citation3], nowadays epigenetic is recognized as the study of hereditary changes without altering the underlying DNA sequence, it focuses on how behaviours and the environment can bring about changes that affect the functioning of genes.
As an important field of research in epigenetics, relative to DNA and histone modification, understanding of RNA modification is still in its early stages. Although the pseudouridine (Ψ), the most abundant modification found in total RNA from human cells, was discovered only 2 years after the first modified DNA nucleoside. It was not until 2012 [Citation4] that the domain of deciphering RNA modifications got its own name, ‘Epitranscriptome.’ More than 170 different types of chemical RNA modifications have already been found on coding and non-coding RNAs (ncRNAs) to date, most of which are methylation modifications [Citation5]. The focus on the effect of RNA modifications in the aspect of gene regulation has already been as important as the modification of DNA and histone.
N6-methyladenosine (m6A), the methylation of the sixth carbon atom of adenosine, was firstly found in polyadenylated RNA from mammalian cells in the 1970s [Citation6]. Currently m6A has been considered the most abundant and best studied post-transcriptional modification in mammalian messenger and non-coding RNA. Because mRNA contains genetic information, previous studies regarding m6A modification mostly focused on mRNA. However, recently the crosstalk between circRNAs and m6A is beginning to gain attention. The RNA modification process for m6A methylation is catalysed by a multi-component methyltransferase complex named ‘m6A writers.’ There are four methyltransferases generating m6A in separate RNAs respectively in the mammalian transcriptome [Citation7]. The ribosomal m6A RNA modification is mediated by the N6-Methyladenosine methyltransferase ZCCHC4 [Citation8] and the METTL5-TRMT112 complex [Citation9]. And the m6A in the U6 small nuclear RNA (snRNA) are catalysed by METTL16 [Citation10]. It is remarkable that the m6A in the MAT2A mRNA sequences which encodes the enzyme responsible for S-adenosylmethionine (SAM) biosynthesis are also formed by METTL16. Finally, a large portion of m6A deposition on mRNA and other transcriptions derived from RNA polymerase II is formed by the heterodimer complex METTL3-METTL14 [Citation11,Citation12]. The primary function of METTL3 is the catalytic core, while METTL14 provides a platform for RNA-binding [Citation13]. RNA modifications were considered to be static and immutable following their covalent attachment in the past. In 2012, the confirmation of physiological substrate and function of fat mass and obesity-associated (FTO) protein [Citation14] provide a breakthrough insight into the state of RNA modification. Meanwhile, as the best physiological substrate for FTO, m6A in nuclear RNA was deemed to the first dynamic and reversible RNA modification [Citation15], making the argument more compelling. Demethylases included FTO and ALKBH5 are also called ‘eraser’ because of their catalysing function of removing m6A from the RNA sequence. m6A have a profound effect on the maturation process of mRNA closely related to gene expression, including pre-mRNA treatment, nuclear exportation of mRNA [Citation16], mRNA stability [Citation17], and translation efficiency [Citation18,Citation19]. The dominant mechanism by which m6A carries out its biological function m6A exerted is through the recruitment of specific proteins. These specific proteins are called ‘reader protein.’ The m6A reader proteins have two distinct mechanisms for recognizing m6A: direct reading and indirect reading. Cytoplasmic proteins the family of domain YTH, YTHDF1, YTHDF2, YTHDF3, bind directly m6A with their eponymous YTH domains [Citation17,Citation20]. Indirect reading is caused by the decreasing of the energy between m6A:U base pairs and A:U base pairs, this energy difference reduces the structural stability of RNA, which may change the binding between RNA and protein [Citation21]. Two types of heterogeneous nuclear ribonucleoprotein, HNRNPC [Citation22] and HNRNPG [Citation23], are indirect m6A reader proteins. In addition to recruiting reader proteins for transcription, m6A on chromosome-associated regulatory RNA (carRNAs) modulate gene expression by regulating the transcription of neighbouring mRNA [Citation24]. The extensive effects of m6A on gene expression imply essential roles on numerous physiological and pathophysiological. The fundamental function of m6A in brain development [Citation25], skeleton function [Citation26] and immune system development [Citation27] has been revealed by analysis of tissue-specific knockout mouse models of METTL3 and METTL14. Furthermore, the knockout of either METTL3 or METTL14 has a serous influence on differentiation in various stem cell or progenitor cell systems [Citation28,Citation29]. Currently, it has been confirmed that the pathogenesis of a variety of diseases is associated with m6A [Citation30,Citation31].
Dynamic m6A regulators have demonstrated the high research potential of m6A methylation. There are tens and thousands of m6A sites across the transcriptome. Methods that map m6A transcription sites, especially whole-transcriptome m6A sites, could uncover the potential global dynamic changes during different biological processes. The stoichiometric information obtained on single nucleotide resolution could be used to assess the contributions of individual m6A sites to biological functions. The METTL3/METTL14 methytransferase have the remarkable characteristic that the specificity of depositing m6A on the transcriptome. Previous studies have determined that the consensus motif is commonly occurring DRACH (D = G/A/U, R = G/A, H = A/U/C) consensus sequence [Citation32]. However, the percentage of methylated DRACH motifs is only 5%, which may have an impact on site recognition. In addition, the distribution of m6A in the transcriptome showed an obvious regional deviation. m6A can be found throughout the transcription length, but is strongly enriched near the stop codon and in abnormally long internal exons [Citation33,Citation34]. According the property and context sequence of m6A, many approaches have been developed to reveal the map of the m6A modification. Biophysical and biochemical methods have been primary means of identifying and quantifying the m6A modification nucleotide by nucleotide in the past. As a large number of high-throughput datasets have been accumulated, bioinformatics has been a cost-effective approach to deciphering epitranscriptome. The review systematically summarized the emerging topics and recent progress in bioinformatics methods to decipher the m6A methylation, including experimental m6A methylation sites detection, related data analysis techniques, m6A methylation sites prediction methods, identification of m6A sites from circular RNA and m6A methylation databases.
Experimental methods for N6-methyladenosine sites identification
The increasing size of the data and much larger RNAs have made it difficult and inefficient to detect the sequence context of modifications, advancement of the field has been stagnant for a long time. The application of high-throughput techniques that based on the detection and quantification of RNA modification has brought about a breakthrough (). Current experimental methods are based on next-generation sequencing, but reverse transcription may introduce errors. Nevertheless, this problem could be solved by single-molecule direct sequencing, with the result that this technology is beginning to attract attention in the detection of m6A sites ().
Table 1. Evaluation of experimental methods for m6A sites identification and quantification.
Antibody-based method to identify N6-methyladenosine site
The most common methods to detect N6-Methyladenosine site at the transcriptome-wide scale depend on a combination of immunoprecipitation and next-generation sequencing. The Methyl-RNA-immunoprecipitation-sequencing (MeRIP-seq) [Citation35] and m6A-seq [Citation33] are two of the most representative technologies. Although MeRIP-seq and m6A-seq were developed by two independent research groups, initial RNA preparation and the IP step are very similar. Poly(A)+-selected RNA was fragmented into 100 ~ 200-nucleotide-long oligonucleotides, parts of these RNA fragments were immunoprecipitated by anti-m6A affinity purified antibody, others were input control. Afterwards, immunoprecipitated as well as input control fragments were prepared as libraries, and subjected to massively parallel sequencing.
Nevertheless, only 100 ~ 200 nucleotides-length m6A modified regions could be detected by MeRIP-seq. Not being able to identify the precise location of m6A in mRNA may limit the understanding of the potential for m6A modification in the regulation of gene expression. Consequently, the specific mutational signatures induced by crosslinking antibodies to m6A modification before reverse transcription have been taken advantage of developing new methods. Most crosslinks of antibodies are induced by ultraviolet light (UV). m6A individual-nucleotide-resolution crosslinking and immunoprecipitation sequencing (miCLIP-seq) [Citation36] mappings m6A in human and mouse mRNA at single-nucleotide resolution by the UV-induced mutational signatures of m6A on the opposite sites in complementary DNA (cDNA) during reverse transcription. Analogously, photo-crosslink-assisted m6A-sequencing (PA-m6A-seq) [Citation37] was developed from photoactivatable ribonucleoside-enhanced crosslinking and immunoprecipitation (PAR-CLIP) [Citation38], which make use of the photoreactivation of 4-thiouridine (4SU) or 6-thioguansine (6SG).After incorporated into mRNA, 4SU could covalently crosslink with residues of nearby aromatic amino acid in RNA-binding proteins upon 365 nm UV irradiation, leading the transition of T-to-C in PCR step.
Quantitative information of m6A represents the proportion of m6A-modified transcripts of each gene in the total transcription, which is very important for the assessment of m6A regulatory impact. m6A-level and isoform-characterization sequencing(m6A-LAIC-seq) [Citation39] does not fragment the RNA prior to anti-m6A RNA immunoprecipitation (RIP) to keep full-length RNAs. Besides, m6A-LAIC-seq links the differences in alternative polyadenylation (APA) usage to m6A methylated transcriptions, revealing diverse proportions of m6A-modified transcriptions between the mRNA isoform.
To date, antibody-based methods have already made the identification and quantification of m6A across transcriptome range possible. In consideration of cost and efficiency, despite MeRIP-seq holds disadvantages of low reproducibility [Citation40], high false positives and limited quantitative information, it is still adopted by most research institutes compared to other methods.
Enzymatic methods to identify N6-methyladenosine sites
The cleavage specificity of RNases could be used to map m6A modifications in mRNA. The ability of an Escherichia coli toxin and RNA endoribonuclease, MazF, has been utilized by MAZFER-seq [Citation41] and m6A-sensitive RNA-endoribonuclease-facilitated sequencing (m6A-REF-seq) [Citation42] to specifically cleave the unmethylated ACA motif at 5’termina, leaving methylated (m6A)CA motifs intact [Citation43]. These methods have the characteristic of precision and could allow to transcriptome-wide identify m6A sites in single-base resolution. Moreover, the two methods have their own characteristics. The m6A stoichiometry information at ACA motifs on mRNAs was provided by MAZFER-seq. And the conservation of m6A sites in mammals was revealed by m6A-REF-seq applied to detect distribution patterns of m6A modifications in different tissues of human, mouse and rat. Enzymatic methods largely reduce the requirement of starting RNA amount. Therefore, they could be applied to identify m6A sites for rare samples from pathological tissues or early embryos. However, because MazF is specific to the ACA motif at 5’termina, only 16–25% of all methylated sites could be identified.
The mutational signatures of m6A during reverse transcription could be generated by the reaction between specific enzymes and m6A or its reader proteins. In deamination adjacent to RNA modification target sequencing (DART-seq) [Citation44], mutations at sites adjacent to m6A sites were induced by the conjugate of cytidine deaminase APOBEC1 and m6A reader protein YTHDF. Nevertheless, the narrow spatial distance between m6A and the adjacent sites possibly leads to bias m6A detections. Likewise, reverse transcriptase during reverse transcription could be induced by N1, N6-cyclized-m6A [Citation45]. m6A-selective allyl chemical labelling and sequencing(m6A-SAC-seq) [Citation46] converts m6A into N6-allyl, N6-methyladenosine(a6m6A) by Dim1/KsgA family of dimethyltransferases. After iodination-induced cyclization reaction, a6m6A is transferred to N1, N6-cyclized-m6A. However, m6A-SAC-seq shows a motif preference of GAC over AAC, which might result in the loss of m6A sites detection. Furthermore, the combination of enzymatic and antibody-based methods also enables to identify m6A modification at a single-base resolution.m6A-Crosslinking-Exonuclease-sequencing(m6ACE-seq) [Citation47] generate a comprehensive atlas of disparate methylomes uniquely mediated by every individual known methyltransferase or demethylase. And m6ACE-seq highlights the importance of m6Am in the detection of m6A, due to the catalytic preferences of FTO to demethylation of m6A and m6Am [Citation48].
Chemical methods to identify N6-methyladenosine sites
In the above methods, m6A sites are indirectly judged according to the adenine near the mutational point induced by RNA-antibody crosslinking or the enzyme reaction, giving rise to difficulty in the identification of real sites (especially cluster sites). Chemical methods could detect m6A sites in a direct way. The N6-methyl group in m6A has adenosine-like inert chemical property, so there are few chemical methods for the detection of m6A. Currently the positions of m6A are marked by the specific chemical reactions. S-adenosyl methionine (SAM) is the cofactor of methyltransferase, providing methyl donor groups for m6A biogenesis [Citation49]. By feeding human and mouse cells with Se-allyl-L-selenohomocysteine, which transfers SAM to allyl-SAM and allyl-SeAM, cellular RNAs could be modified by N6-allyladenosine(a6A) instead of N6-methyladenosine at special sites [Citation50,Citation51].After the iodination-induced cyclization reaction [Citation52], N6-allyladenosine could be identified as the opposite base misincorporation during reverse transcription in the high-throughput sequencing. The method called m6A-label-seq offers a strategy to label and identify cellular m6A nidification sites. However, the challenge of m6A-label-seq like the weak labelling and the lack of a long labelling period still leave room for it to improve. In addition, FTO-assisted m6A selective chemical labelling sequencing(m6A-SEAL-seq) [Citation53], which combines the oxidation of m6A methylation by demethylase FTO with dithiothreitol(DTT)-mediated thiol-addition chemical reaction to change N6-methyladenosine to N6-dithiolsitolmethyladenosine(dm6A) with the free sulphhydryl group. N6-dithiolsitolmethyladenosine(dm6A)-marked RNA species are more stable and the free sulphhydryl group could be exploited to instal various tags like biotin and fluorophores for follow-up of the sequencing operation through reaction with methanethiosulfonate (MTSEA). These tags could be identified as m6A modifications during the follow-up sequencing operation. Compared to antibody-dependent MeRIP-seq, m6A-SEAL-seq has a similar detection resolution but more a excellent target specificity because of unbiased in the enrichment process [Citation54]. Although chemical methods avoid antibody specificity and could identify m6A sites at higher resolution, the stoichiometric information is still unclear.
Methods based on direct RNA sequencing to identify N6-methyladenosine sites
Because methylation on the RNA might be influenced by reverse transcription and PCR amplification of methods based on next-generation sequencing, direct-RNA-sequencing based on Oxford Nanopore Technologies (ONT) provided a new insight into m6A modification detection. ONT’ nanopore-based platform [Citation55] uses electrophoresis to drive individual molecules through nanopores one by one for sequencing. m6A sites on the RNA will cause the perturbation of the current within the nanopore, making it possible to directly detect. The m6A sites on different isoforms of the same gene could be separated by ONT. Workman [Citation56] proved that the median current levels at GGACU motif of m6A modifications which belong to different isoforms of the same gene were significantly different. Raw electric current signals are translated to RNA sequences by sophisticated base-calling software used Hidden Markov Models (HMMs) or other models [Citation57]. Then the modified nucleoside in each position could be described. In addition, endogenous and exogenous RNA modification on long RNAs could be detected by ONT at the single-molecule level, making up the limitations on read length inherent to short-read-based methods [Citation58]. Meanwhile, zero-mode waveguides applicated in single-molecule, real-time (SMRT) could directly obtains the information of m6A sites from the RNA template by following the activity of reverse transcriptase enzymes synthesizing cDNA on thousands of single RNA templates simultaneously in real time with single nucleotide turnover resolution [Citation59]. However, the development of methods based on SMS is not yet mature and the problem of cost is not a small difficulty, but its perspective is still expected.
Bioinformatics approached for m6A methylation analysis
The overall performance of these m6A Methylation Analysis methods depends not only on chemical processing and library preparation steps, but also, to a large extent, on the performance of bioinformatics pipelines and the robustness of training data sets used for parameter optimization. The methods mentioned above are mainly based on the next generation sequencing and nanopore sequencing (). In this section, we discuss the process of bioinformatics analysis after library construction and sequencing.
Bioinformatics analysis tools and software for MeRIP-seq(m6A-seq) data
Peak calling
As the most widely adopted experimental approach for mapping m6A sites on the transcriptome wide, follow-up bioinformatics analysis and data mining for MeRIP-seq are also receiving considerable attention (). The sequencing data are quality control and then aligned to the reference genome. In comparison with input samples, more read aggregations have appeared on the transcriptomic regions with m6A of IP samples. When visualizing the read counts along the genome, these regions are identified as peak-like shapes. The process of inferring the position of m6A methylation is ‘peak calling.’
Table 2. Bioinformatics analysis tools and software based on MeRIP-seq(m6A-seq) data.
Chromatin Immunoprecipitation following by next generation sequencing (CHIP-seq) used to analyse protein interactions with DNA has similar data composition with MeRIP-seq. Therefore, MACS2 [Citation60] a CHIP-seq peak calling methods, has become a common peak calling method for MeRIP-seq data. Nevertheless, due to the great variation of input which measure the gene expression intensity, the methods developed for CHIP-seq data will call more peaks for highly expressed genes, generating peak calling errors. Moreover, replications in MeRIP-seq are few, which will lead to an unstable estimation of the variance. Special methods and software should be developed to analyse MeRIP-seq data. As a software tool specifically designed for MeRIP-seq data, exomePeak2 [Citation61] is calling exome-based peaks and sensitive to PCR amplification biases universally appearing in NGS data. Based on the exomePeak2, MeTPeak [Citation62], sensitive to lowly enriched peaks, is a graphical model-based peak calling method, improving the detection performances and robustness. Another software tool for connected exons of a specific gene is HEPeak [Citation63] which was based on the Hidden Markov Model (HMM), improving sensitivity and detection specificity. A graphical user interface was proposed by m6aViewer [Citation64] to reduce the difficulty of the entire process. Other approaches such as MeRIP-PF [Citation65], BaySeqPeak [Citation66], TRES [Citation67] and MoAIMS [Citation68] improved the output format and zero-inflated problems, making the presented results precise and intuitive.
Differential methylation analysis
Differential methylation analysis concentrates on the different modification status between two different biological conditions. There are three levels of differential analysis: differential methylation probe(DMP), differential methylation region(DMR) and differential methylation block(DMB). Because MeRIP-seq could not detect m6A modification at a single-nucleotide resolution, DMRs, the regions with different levels of methylation under two different conditions, are the best choice to research [Citation69]. The comparison of differences in RNA methylome in the case-control study is the point of differential methylation analysis. Compared to DNA differential methylation analysis, the total amount of methylated RNA and the relative amount of methylated RNA might have different trends because of the uncertainty of the level of expression. Consequently, the relative abundance or methylation ratio, especially the ratio of methylation to total RNA, is the primary focus of current tools and software.
As an available computational tool specialized for MeRIP-seq, exomePeak2 [Citation70] performed a simple Fishers precision test on normalized average read counts of two sets of IP/ control samples detected for the purest DMS region. Since MeRIP-seq read counts have significant biological and technical variations, detection based only on average read counts may introduce a large number of false positives. A β-binomial model was adopted by MeTDiff [Citation71] to model differences in transcriptions and multiple MeRIP-seq samples, achieving a significant improvement in detection sensitivity and specificity. exomePeak2 and HEPeak are both window-based methods of estimating the methylation signal, which imposes a strong dependence structure on the reads counts across the gene. Therefore, a distribution-free test statistic was adopted by MVT [Citation72] to control type I error, achieving high power to detect differential RNA methylation. Count-based small sample estimation of biological variability in MeRIP-seq is also a major challenge. The within-group variability was resolved with the models based on negative-binomial distribution in RNA-seq data. On the other hand, in MeRIP-seq data, within-group biological variability also has an impact on the differential RNA methylation analysis. Inspired by the similar research in RNA-seq data, the negative binomial model was used by DRME [Citation73] for the small sample size MeRIP-seq dataset. Furthermore, 2 independent negative binomial distributions are more included in QNB [Citation74], which could greatly improve the performance of tests for extremely low expression genes. However, the presence of covariates is not taken into consideration by both methods above. According to research needs, the covariates could be incorporated in the analysis by RADAR [Citation75], which identify altered methylation sites by modulated the expression level before immunoprecipitation and the change of count after immunoprecipitation by different strategies. The unification of various library sizes of the MeRIP-seq samples leads to a certain loss of data information. A binomial likelihood ratio test was used by bltest [Citation76] to identify differential methylation regions by the difference in the success rates of binomial distribution in the IP and input samples. And the prediction precision is improved, especially in the unbalanced sample library sizes. Moreover, the multiple methylated residues within a single methylation site cannot be identified effectively by existing peak-based methods. The detected m6A methylation site is divided by FET-HMM [Citation77] into several adjacent small bins and models the dependence between spatially adjacent bins by the hidden Markov model. Since antibody-based approaches lack the stoichiometric information of m6A sites, antibody-independent methods have attracted increasing attention in differential methylation analysis. However, most antibody-independent methods pay little attention to differential methylation analysis, it still takes time to develop novel bioinformatic analysis tools based on the stoichiometric information of m6A sites.
Clustering analysis of m6A sites
The m6A sites are enriched in the transcription initiation region with high CpG genes [Citation78], implying that m6A may directly regulate the epigenetic state and transcription of corresponding genes through co-transcription. Furthermore, the new phenomenon RNA epigenetic modification information flows from RNA to chromatin via co-transcription are revealed by the direct relationship between m6A methylation and dynamic modification of histone [Citation79].Consequently, the associated methylation mechanisms between the m6A function could be explained by the clustering analysis of m6A sites. The m6A methylation sites are classified by Schwartz [Citation80] as WTAP-dependent and independent sites that connected to different RNA functions by binary clustering, respectively. WTAP-dependent sites are located at internal positions in transcripts, and its topology is relatively static across mRNAs. The co-methylation pattern in MeRIP-seq data is discovered by MeTCluster [Citation81] with the cluster for the degree of m6A methylation peaks based on the expectation-maximization algorithm, providing a new direction for studying the mechanisms and functions of m6A modification. However, the low reads coverage of count-based RNA methylation sequencing data might influence the cluster result. Therefore, a beta-binomial mixture model [Citation82] was proposed to capture the clustering effect in methylation level and a nonparametric Dirichlet process was adopted to determine the optimal number of clusters. The method not only reveal novel m6A co-methylation patterns from sites of very low reads coverage, but also learns an optimal number of clusters adaptively from the data analysed. On the other hand, the linkage between the global RNA co-methylation patterns and the latent enzymatic regulators are unveiled by the clustering approaches [Citation83] including K-means, Hierarchical clustering (HC), Bayesian factor regression model (BFRM) and nonnegative matrix factorization (NMF), providing a promising prospect for researching of the epitranscriptome. However, the detailed regulatory mechanisms for m6A methylation sites under different conditions remain unclear. The phenomenon which the methylation levels of certain sites increase or decrease simultaneously under certain conditions and sites from the same co-methylation module exhibit simultaneous hypermethylation or hypomethylation under certain conditions could be explained by the local co-methylation patterns (LCPs). The phenomenon which the methylation levels of certain sites increase or decrease simultaneously under certain conditions and sites from the same co-methylation module exhibit simultaneous hypermethylation or hypomethylation under certain conditions could be explained by the local co-methylation patterns (LCPs). The situation could be regarded as a typical biclustering problem, which is widely used in the discovery of co-expression patterns. Biclustering algorithms, FBCwPlaid [Citation84] and BDBB [Citation85], have been proposed to mine LCPs of m6A epi-transcriptome data. Furthermore, an RNA Expression Weighted Iterative Signature Algorithm are adopted by RNREW-ISA V2 [Citation86], revealing the potential local functional patterns presented in m6A profiles, where sites are co-methylated under specific conditions. Compared with ordinary biclustering algorithms, overlapping local functional blocks (LFBs) in the input data could be obtained by REW-ISA V2. Moreover, the various high-throughput sequencing data artefacts induced by the intrinsic properties of RNA molecules, RNA modification and other influence elements could be tolerated based on a measurement weighting strategy [Citation87].
Bioinformatics workflow based on reverse transcription (RT) signatures
Reverse transcription (RT) signatures are caused by modifications on the Watson-Crick face [Citation88]. As mentioned in the above experimental methods, combined with the high-throughput sequencing, RT signatures occurred during the synthesis of complementary DNA (cDNA) is an effective mark to the analysis of m6A methylation. There are several library preparation protocols for capturing cDNA with RT signatures [Citation89–91]. HAMR [Citation92] was regarded as one of the first attempts to adopt the RT signatures to analysis the modifications in the RNA template. However, there is a lack of specialized visual function in early-stage viewers. The CoverageAnalyzer(CAn) did an automated screening of candidates for modification and a detailed visual inspection of RT signatures [Citation93].And the typical CAn session provides data sorting and filtering options, visualization tab, candidate casting tab, formula editor and control panel for the serial trace. In addition, a versatile graphical workflow system for modification calling based on machine learning models was presented by Galaxy [Citation94], which are equipped with the principal module (trimming, mapping and postprocessing) and other downstream modules. It should be noted that the detection of modifications by RT signatures can only be applied to a limited subset of given RNA modifications, as many modifications do not affect the Watson-Crick base pairing during cDNA synthesis, which called RT silent [Citation95]. Moreover, the biases and artefacts because of the conversion from RNA to cDNA will be introduced by the mapping approaches using RT signatures of mRNA and polymerase chain reaction (PCR) [Citation96]. These problems could be resolved by the direct long reads sequencing of Oxford Nanopore Technologies (ONT).
Bioinformatics analysis of nanopore sequencing
The perspective of mapping m6A methylation approaches by nanopore technology is luciferous. However, the raw signals counterpart to the sequence contexts of modified and unmodified base are still inexplainable until now. As a result, currently available algorithms that map RNA modification by nanopore sequencing are based on either modification-induced base calling errors or difference in raw signal levels ().
Table 3. Bioinformatics tool for nanopore sequencing.
The first category of algorithms concentrates on the error frequencies as compared to the background at specific sites in the transcriptome. The epitranscriptional landscape from glitches of ONT signals the percent Error of Specific Bases(%ESB) of native RNA has been found higher than unmodified RNA. ELIGOS [Citation97] infers the epitranscriptional landscape from glitches of ONT singnals, which is appropriate for various types of synthetic modified RNA. However, this method is influenced by the different nanopore motor, sequencing directions, and basecalling models between RNA and cDNA. Furthermore, based on differential base-calling errors, EpiNano [Citation98] and DiffErr [Citation99] were developed to identify m6A sites in paired conditions (wild type and METTL3 KD). Meanwhile, the ribonucleic acid modifications also could be detected is by DRUMMER [Citation100] used the frequency of matches/mismatches between a WT and a low modified sample. Nevertheless, due to the single error is not available to confirm the presence of modification, the methods based on modification-induced base calling errors provide the location of multiple modified sites, but could not achieve the detection at single-molecule resolution.
The second category of approaches is based on the raw current/signal intensity. The most common m6A sequence context, DRACH, was focused by MINES [Citation101]. The Tombo’s de novo detection algorithm [Citation102] used by MINES provides coverage of genomic reference yield only along the 3’ untranslated regions (UTRs). It could be supplied in combination with the cDNA reference. The modification stoichiometry of 30 nucleotide windows centred on the A of each DRACH motif with a minimum read coverage of 5 reads is filtered. And the Random Forest Model (RFM) is used to classify the central A of the DRACH motif as modified or unmodified by these stoichiometry values. Moreover, the comparation between the raw electrical signal for a sample of interest and the signal in a control sample containing fewer or no modifications are utilized by Nanocompore [Citation103] to detect potential RNA modifications. The significant difference in the distribution of reads into the two clusters between conditions is distinguished by a bivariate classification method based on two components Gaussian mixture model (GMM) clustering followed by a logistic regression test (logit). In addition, a multi-sample two Gaussian mixture model with the prior of the theoretical signal distribution of the unmodified k-mer is adopted by xPore [Citation104]. It is considered a significant innovation to attribute two distributions obtained from GMM to be assigned to a modified or unmodified state. The essence of xPore is to model the probability for each modified read, which could be used to calculate the fraction of reads that are assigned to the modified signal distribution. To improve accuracy and reduce false positives, similar positions where distributions for unmodified and modified signals and the one-directional signal shifts RNA modification induced the signal for each k-mer must be considered. Furthermore, the multivariate Gaussian mixture model is used by Yanocomp [Citation105] to model the average ionic current amplitude of the five k-mers sliding window. Outliers are represented by two Gaussian components and a third uniform component to minimize overfitting.
Similar to m6A modification, ONT is also applicable for the detection of other RNA modification like A-to-I [Citation106,Citation107], Ψ [Citation108] and m5C. However, the detection might be effect by the species of RNA modification and other factors.
Computational Methods for N6-Methyladenosine Sites Prediction
Given that experimental approaches are expensive and limited, in silico methods have attracted increasing attention as an alternative means of studying m6A modification. () The data produced by experimental methods provide benchmark datasets for model construction. Most m6A sites prediction methods and web servers extracted input features from the sequence-derived information and other genomic information and predicted m6A sites by various machine learning approaches. Finally, the performance of the measures is evaluated ().
Table 4. Summary of the reviewed predictors for m6A sites.
Dataset construction and feature extraction
The positive sample (m6A sites) and the negative sample are extracted according the published maps of m6A sites. And the potential influence of sequence redundancy could be removed by the CD-HIT-EST tool [Citation109]. The redundant sequence is defined as the training sample with high sequence identity compared to another testing sample. The threshold of sequence similarity ranged commonly from 60% to 80%. In addition, the bench-mark datasets are built by two modes. The genomic sequences are used by the full transcript mode as an input and the cDNA sequences are considered by the mature mRNA mode instead. However, most methods consider only one of these patterns.
In order to construct robust and precise machine learning predictors for predicting RNA modification sites, multiple features were designed and extracted to encode RNA sequences. In the computational approaches published at present, features are mainly divided into six categories [Citation110], including RNA primary sequence-derived features, nucleotide physicochemical properties, predicted RNA structural features, position-weighted matrix, RNA sequence similarity feature and genomic-derived features. Among them, the primary sequences with the most fundamental biomolecules information are most versatile features for developing approaches for RNA modification. Numerous tools have been developed for feature extraction and modelling of primary sequences, such as BioSeq-Analysis [Citation111,Citation112], PyFeat [Citation113] and BioSeq-BLM [Citation114]. However, high dimensional vectors may result in large computation, overfitting and low robustness of the proposed model. Therefore, the selection of feature is indispensable to exclude noise and improve the computational efficiency of the models. The mRMR algorithm [Citation115] is usually used to acquire the optimal feature subset because of its usability and efficiency. Furthermore, the perturb method and the SFS are also used for feature selection.
m6A methylation site prediction
Most of the RNA modification prediction methods are based on machine learning models. To date, almost 100 different approaches [Citation116–118] have been established, including notably, WHISTLE [Citation119], iRNA-m6A [Citation120], MethyRNA [Citation121], SMAMP [Citation122], HMpre [Citation123], M6AMRFS [Citation124], Gene2vec [Citation125], BERMP [Citation126],etc. Among them, WHISTLE predicts m6A sites based on the deep learning models. Because both the transcript structure and the relative position on the transcript are found to be related to the occurrence and function of RNA sub-molecular events, transcript annotation could be used as an information source for predicting m6A modification [Citation127,Citation128]. Therefore, geographic encoding of transcripts might be used for deep learning models applied to RNA transcripts. Compared to other deep learning models, the transcript region information incorporated into genomic features by WHISTLE greatly improves its performance. However, information regarding the position relative to the boundaries of the long-range is neglected. In addition, one-hot encoding is widely used to describe the transcript region [Citation129], but it may result in an incomplete landscape of the local transcript structure. To fill the gap, three novel encoding methods, landmarkTX, gridTX, and chunkTX, were developed by Geo2vec [Citation130]. Combined with one-hot encoding, more informative and interpretable sub-molecular geographic descriptors of transcripts are provided. Furthermore, experimental results indicate that the base, upstream, and downstream information of m6A sites are all critical to detection. Natural language processing is used to feature extraction and classification of m6A methylation sites with consideration of context information [Citation131–133] Most of above methods are based on strong supervision enabled by base-resolution data, but in some situation low-resolution m6A modification data can only be obtained. Weakly supervised learning models [Citation134,Citation135], which learns from datasets produced by low-resolution experimental methods like MeRIP-seq, were proposed to predict the situation of m6A modification. Moreover, the precise identification of all types of RNA modification is essential for understanding the functions and regulatory mechanisms of RNAs. The relationship among different types of RNA modifications was revealed by MultiRM [Citation136], allowing an integrated analysis of common RNA modifications. In addition to predicting m6A-containing sequences, the biological features surrounding m6A could be characterized to elucidate its regulatory code [Citation133,Citation137]. Besides, conservation analysis of individual m6A sites is achieved by a novel scoring framework, ConsRM [Citation137]. ConsRM has been confirmed to outperform phastCons [Citation138], a traditional and versatile conservation score, in distinguishing conserved m6A sites.
Performance evaluation measures and strategies
Sensitivity (Sn), Specificity (Sp), Accuracy (Ac) and Matthews Correlation Coefficient (MCC) are used to measure the predictor’s performance at certain thresholds. They are defined as:
Where TP, FP, TN and FN each represent the true positive, false positive, false negative and true negative predictions, respectively. Receiver-operating-characteristic (ROC) curves for the predictors and the area under ROC curve (AUC) are also calculated to evaluate the overall performance of the predictors. The higher the AUC and AUPRC value, the better the prediction performance.
Three validation methods, including the K-fold cross-validation test, jackknife validation test and independent dataset test, are often used to derive comparative metrics (values) among the reviewed predictors. Generally, the jackknife is commonly used in the prediction task with a smaller dataset size, while K-fold cross-validation and independent tests are most popular in the prediction task with a larger dataset size. Of the reviewed predictors, most carried out 1–2 cross-validation tests.
Identification of m6A modification in circRNAs
Circular RNA (circRNA) was first discovered in pathogens in 1976 [Citation139] and soon afterwards in eukaryotic cells [Citation140]. It is a class of single-stranded covalently closed RNA molecules generated by back-splicing [Citation141]. A growing body of research has confirmed that circRNAs play a critical role in the occurrence and development of various diseases [Citation142–144]. The m6A modification is the most common and well-studied post-transcriptional RNA modification pattern across almost every type of RNA molecules including circRNA [Citation145]. Currently, the research on m6A modification in circRNA is in an incipient state. Growing evidence has corroborated that m6A modification is critical to regulate the metabolism and functions of circRNAs [Citation146,Citation147]. The approaches mentioned in section 2 could also be used to detect m6A modification in circRNAs. For example, MeRIP is still the principal method for analysing the m6A level of circRNA [Citation148,Citation149], and circRNA-m6A-seq could identify the m6A-containing endogenous circRNAs after treating the RNA sample with exoribonuclease RNase R [Citation150]. Meanwhile, computational methods are developed based on the MeRIP-seq data to map m6A modifications in circRNA [Citation151,Citation152]. In addition, due to its unique property of low total RNA input, m6A-circRNA epitranscriptomic microarray [Citation153,Citation154] is coming into vogue. However, microarray based on the hybridization of probes must have the sequence of the samples in advance. Moreover, ‘silicon-on-insulator’ structures were used to fabricate biosensor-based chips [Citation155,Citation156] to detect the m6A modification in circRNA. Despite this method holds characteristics of sensitivity, stability and real-time, the reliability of identification is low. The relationship between circRNAs and m6A is not clear at this point, the detection of m6A modification in circRNAs remains difficult.
m6A methylation databases
Knowledgebase with the comprehensive collection and integration of various information related to m6A modification is of crucial importance for elucidating the functional and regulatory circuitry of it as well as for developing bioinformatics tools(). To date, more than a million m6A methylation sites have been found from studies between different species. These sites and other types of modification sites are all contained in RMBase [Citation157,Citation158]. The online visualization tool is also developed by RMBase to plot modification motifs and metagenes of RNA modification along a transcript model. As another database with the location of modified residues, MODOMICS [Citation159,Citation160] is currently the most comprehensive source of RNA modification pathway. In the latest version, the new external resources like RCBS Protein Data Bank database(links to structure), Human Metabolome Database [Citation161], PubChem [Citation162] and ChEMBL [Citation163]. Compared to RMBase, only 442,162 m6A sites are identified by m6A-Atlas [Citation164], but these sites with high-confidence are based on different base-resolution technologies and the quantitative epitranscriptome profiles estimated from high-throughput sequencing samples. Moreover, the biological functions of individual m6A and the potential pathogenesis of m6A could be predicted by m6A-Atlas from epitranscriptome data. On account of its function as a diseases (including various cancers) regulatory factor, the m6A modification is considered as a developing guideline for the treatment of targeted disease. 222 experimentally confirmed m6A-disease association are included by M6ADDsites and the potential pathogenesis of m6A could be predicted by m6A-Atlas from epitranscriptome data. On account of its function as a diseases (including various cancers) regulatory factor, the m6A modification is considered as a developing guideline for the treatment of targeted disease. 222 experimentally confirmed m6A-disease association are included by M6ADD [Citation165] to explore the associations between m6A modification and gene disorders and diseases. Furthermore, it remains a major challenge to distinguish disease-causing variants among a large number of single nucleotide varoants(SNP). The m6A-associated variants that potentially influence m6A modification are integrated by m6Avar [Citation166], deriving from three different m6A sources including miCLIP/PA-m6A-seq experiments (high confidence), MeRIP-seq experiments (medium confidence) and transcriptome-wide predictions (low confidence), to evaluate the effect of variants on m6A modification. The data on the effects of m6A-centred regulation on both disease development and drug response are covered by M6AREG [Citation167]. As is mentioned above, a variety of experimental methods have been developed for m6A site detection. Among them, MeRIP-seq is the earliest as well as the most popular method. REPIC [Citation168] and MeT-DB [Citation169] are specifically for recording peaks called from MeRIP-seq data. In addition, all of the above databases focus on the relationships between m6A modification and RNA-binding proteins (RBPs). A series of regulatory factors, m6A writers, erasers and readers (WERs), play a role in the dynamic and reversible process of m6A modification. Due to different downstream genes targeted by the WERs, the same WER might perform different functions under different conditions. The known m6A WER target genes are hosted by M6A2Target [Citation170] to elucidating the function of m6A dynamic modification.
Table 5. m6A methylation databases.
Validation method for N6-Methyladenosine sites
Different high throughput techniques are prone to different degrees of error. Current datasets including hundreds to thousands of sites, were primarily predicted using high-throughput sequencing data(). With the increasing of published datasets, a great number of detected m6A sites might be highly uncertainty. It’s necessary to regard detected m6A sites as candidate sites and validate the datasets based on high-throughput sequencing methods by at least one additional independent method. The greater the difference between the nature of the validation method and the original method, the higher the reliability of the validation [Citation88]. As an early way to identify m6A modifications, liquid chromatography-tandem mass spectrometry (LC-MS/MS) [Citation171] analysis the m6A methylation via neutral loss Scan (NLS) and dynamic multiple reaction monitoring(DMRM).The enriched RNA also could be directly quantified by quantitative PCR used by Methyl-RNA-immunoprecipitation-quantitative PCR(MeRIP-qPCR) after m6A antibody is enriched to RNA with methylation modification [Citation172]. But exact measurement of the m6A stoichiometry at the specific site is irrealizable in LC-MS/MS and MeRIP-qPCR. To solve the situation, site-specific cleavage and radioactive-labelling followed by ligation-assisted and thin-layer chromatography (SCARLET) [Citation173] could accurately determine m6A status at any site in mRNA/LncRNA. Moreover, the significant selectivity against m6A modification of T3 DNA ligase has been found [Citation174], which could be used to determine the m6A modification fraction at the precise location. In addition, SELECT [Citation175] exploit the decrease of the single-base elongation activity of DNA polymerases and the nick ligation efficiency of ligases hindered by m6A, quantifying by qPCR.
Table 6. Validation Method for N6-Methyladenosine Sites.
Conclusion and discussion
With increasing emphasis on Epitranscriptome, the bioinformatics capacity to analyse, digest, collect and share the rapidly growing epitranscriptome profiling data is sorely needed. The review summarizes the recent topics and progress made in bioinformatics methods of deciphering the m6A methylation, including the experimental detection of m6A methylation sites, techniques of data analysis, the way of predicting m6A methylation sites, m6A methylation databases, and detection of m6A modification in circRNA. Together, developments in bioinformatics have greatly facilitated research in the field and have improved understanding of the biological significance of RNA modifications.
Nevertheless, in spite of the rapid advances in epitranscriptome bioinformatics, there are still a number of limitations or open questions. The technological bias and limitations may not have received sufficient attention when developing bioinformatic tools. The issues of cost and efficiency remain the limitations of many methods, particularly the Oxford nanopore method. Furthermore, some bioinformatics pipelines have not been expended to accommodate the emergence of new modifications resulting from the development of new technologies. In addition, the crosstalk between circRNAs and m6A is in the incipient stage and remains challenging. And the user-friendly, freely accessible and comprehensive database integrating m6A-circRNA-related research is still not developed for the studies the relation of m6A and circRNA. Lastly, with the rapid development of single-cell DNA methylation, single-cell m6A sequencing is beginning to be a novel breakthrough in the study of m6A landscape and function.
Disclosure statement
No potential conflict of interest was reported by the author(s).
Additional information
Funding
References
- Waddington CH. The Epigenotype. Int J Epidemiol. 2012 Feb;41(1):10–24.
- Avery OT, MacLeod CM, McCarty M. Studies on the chemical nature of the substance inducing transformation of pneumococcal types induction of transformation by a Desoxyribonucleic Acid fraction isolated from pneumococcus Type III. J Exp Med. 1944 Feb;79(2):137–158.
- Allis CD, Jenuwein T. The molecular hallmarks of epigenetic control. Nat Rev Genet. 2016 Aug;17(8):487–500.
- Saletore Y, Meyer K, Korlach J, et al. The birth of the Epitranscriptome: deciphering the function of RNA modifications. Genome Biol. 2012;13(10):175. 2012,175.
- Boccaletto P, Stefaniak F, Ray A, et al. MODOMICS: a database of RNA modification pathways. 2021 update. Nucleic Acids Res. 2022 Jan 7;50(D1):D231–D235.
- Sommer S, Lavi U, Darnell JE. ABSOLUTE FREQUENCY OF LABELED N-6-METHYLADENOSINE IN HELA-CELL MESSENGER-RNA DECREASES WITH LABEL TIME. J Mol Biol. 1978;124(3):487–499.
- Zaccara S, Ries RJ, Jaffrey SR. Reading, writing and erasing mRNA methylation. Nat Rev Mol Cell Biol. 2019 Oct;20(10):608–624.
- Ma H, Wang X, Cai J, et al. N-6-Methyladenosine methyltransferase ZCCHC4 mediates ribosomal RNA methylation. Nat Chem Biol. 2019 Jan;15(1):88.
- Nhan Van T, Ernst FG, Hawley BR, et al. The human 18S rRNA m(6)A methyltransferase METTL5 is stabilized by TRMT112. Nucleic Acids Res. 2019 Sep 5;47(15):7719–7733.
- Pendleton KE, Chen B, Liu K, et al. The U6 snRNA m(6)A Methyltransferase METTL16 Regulates SAM Synthetase Intron Retention. Cell. 2017 May 18;169(5):824.
- Liu J, Yue Y, Han D, et al. A METTL3-METTL14 complex mediates mammalian nuclear RNA N-6-adenosine methylation. Nat Chem Biol. 2014 Feb;10(2):93–95.
- Geula S, Moshitch-Moshkovitz S, Dominissini D, et al. m 6 A mRNA methylation facilitates resolution of naïve pluripotency toward differentiation. Science. 2015 Feb 27;347(6225):1002–1006.
- Wang X, Feng J, Xue Y, et al. Structural basis of N-6-adenosine methylation by the METTL3-METTL14 complex. Nature. 2016 Jun 23;534(7608):575.
- Jia G, Fu Y, Zhao X, et al. N6-Methyladenosine in nuclear RNA is a major substrate of the obesity-associated FTO. Nat Chem Biol. 2011 Dec;7(12):885–887.
- Zheng G, Dahl J, Niu Y, et al. ALKBH5 Is a Mammalian RNA Demethylase that Impacts RNA Metabolism and Mouse Fertility. Mol Cell. 2013 Jan 10;49(1):18–29.
- Roundtree IA, Luo G-Z, Zhang Z, et al. YTHDC1 mediates nuclear export of N-6 - methyladenosine methylated mRNAs. Elife. 2017 Oct 6;6:e31311.
- Wang X, Lu Z, Gomez A, et al. N-6-methyladenosine-dependent regulation of messenger RNA stability. Nature. 2014 Jan 2;505(7481):117.
- Wang X, Zhao B, Roundtree I, et al. N-6-methyladenosine Modulates Messenger RNA Translation Efficiency. Cell. 2015 Jun 4;161(6):1388–1399.
- Slobodin B, Han R, Calderone V, et al. Transcription Impacts the Efficiency of mRNA Translation via Co-transcriptional N6-adenosine Methylation. Cell. 2017 Apr 6;169(2):326–337.
- Zhu T, Roundtree IA, Wang P, et al. Crystal structure of the YTH domain of YTHDF2 reveals mechanism for recognition of N6-methyladenosine. Cell Res. 2014 Dec;24(12):1493–1496.
- Roost C, Lynch SR, Batista PJ, et al. Structure and Thermodynamics of N 6 -Methyladenosine in RNA: a Spring-Loaded Base Modification. J Am Chem Soc. 2015 Feb 11;137(5):2107–2115
- Liu N, Dai Q, Zheng G, et al. N-6-methyladenosine-dependent RNA structural switches regulate RNA-protein interactions. Nature. 2015 Feb 26;518(7540):560–564.
- Zhou KI, Shi H, Lyu R, et al. Regulation of Co-transcriptional Pre-mRNA Splicing by m(6)A through the Low-Complexity Protein hnRNPG. Mol Cell. 2019 Oct 3;76(1):70.
- Liu J, Dou X, Chen C, et al. N 6 -methyladenosine of chromosome-associated regulatory RNA regulates chromatin state and transcription. Science. 2020 Jan 31;367(6477):580.
- Yoon K-J, Ringeling FR, Vissers C, et al. Temporal Control of Mammalian Cortical Neurogenesis by m(6)A Methylation. Cell. 2017 Nov 2;171(4):877.
- Wu Y, Xie L, Wang M, et al. Mettl3-mediated m(6)A RNA methylation regulates the fate of bone marrow mesenchymal stem cells and osteoporosis. Nat Commun. 2018 Nov 14;9(4772).
- Winkler R, Gillis E, Lasman L, et al. m(6)A modification controls the innate immune response to infection by targeting type I interferons. Nat Immunol. 2019 Feb;20(2):173.
- Lee H, Bao S, Qian Y, et al. Stage-specific requirement for Mettl3-dependent m(6)A mRNA methylation during haematopoietic stem cell differentiation. Nat Cell Biol. 2019 Jun;21(6):700.
- Vu LP, Pickering BF, Cheng Y, et al. The N-6-methyladenosine (m(6)A)-forming enzyme METTL3 controls myeloid differentiation of normal hematopoietic and leukemia cells. Nat Med. 2017 Nov;23(11):1369.
- Yi Y-C, Chen X-Y, Zhang J, et al. Novel insights into the interplay between m(6)A modification and noncoding RNAs in cancer. Mol Cancer. 2020 Aug 7;19(1).
- Barbieri I, Tzelepis K, Pandolfini L, et al. Promoter-bound METTL3 maintains myeloid leukaemia by m(6)A-dependent translation control. Nature. 2017 Dec 7;552(7683):126.
- Fu Y, Dominissini D, Rechavi G, et al. Gene expression regulation mediated through reversible m(6)A RNA methylation. Nat Rev Genet. 2014 May;15(5):293–306.
- Dominissini D, Moshitch-Moshkovitz S, Schwartz S, et al. Topology of the human and mouse m(6)A RNA methylomes revealed by m(6)A-seq. Nature. 2012 May 10;485(7397):201–U84.
- Xu W, He C, Kaye EG, et al. Dynamic control of chromatin-associated m(6)A methylation regulates nascent RNA synthesis. Mol Cell. 2022 Mar 17;82(6):1156.
- Meyer KD, Saletore Y, Zumbo P, et al. Comprehensive Analysis of mRNA Methylation Reveals Enrichment in 3 ‘ UTRs and near Stop Codons. Cell. 2012 Jun 22;149(7):1635–1646.
- Linder B, Grozhik AV, Olarerin-George AO, et al. Single-nucleotide-resolution mapping of m6A and m6Am throughout the transcriptome. Nat Methods. 2015 Aug;12(8):767–U114.
- Chen K, Luo G-Z, He C. High-Resolution Mapping of N-6-Methyladenosine in Transcriptome and Genome Using a Photo-Crosslinking-Assisted Strategy. Methods in Enzymology. 2015;560:161–185.
- Hafner M, Landthaler M, Burger L, et al. Transcriptome-wide Identification of RNA-Binding Protein and MicroRNA Target Sites by PAR-CLIP. Cell. 2010 Apr 1;141(1):129–141.
- Molinie B, Wang J, Lim KS, et al. m(6)A-LAIC-seq reveals the census and complexity of the m(6)A epitranscriptome. Nat Methods. 2016 Aug;13(8):692.
- McIntyre ABR, Gokhale NS, Cerchietti L, et al. Limits in the detection of m(6)A changes using MeRIP/m(6)A-seq. Sci Rep. 2020 Apr 20;10(1).
- Garcia-Campos MA, Edelheit S, Toth U, et al. Deciphering the “m(6)A Code” via Antibody-Independent Quantitative Profiling. Cell. 2019 Jul 25;178(3):731.
- Zhang Z, Chen L-Q, Zhao Y-L, et al. Single-base mapping of m 6 A by an antibody-independent method. Sci Adv. 2019 Jul;5(7):eaax0250.
- Imanishi M, Tsuji S, Suda A, et al. Detection of N-6-methyladenosine based on the methyl-sensitivity of MazF RNA endonuclease. Chem Comm. 2017 Dec 14;53(96):12930–12933.
- Meyer KD. DART-seq: an antibody-free method for global m(6)A detection. Nat Methods. 2019 Dec;16(12):1275.
- Shu X, Dai Q, Wu T, et al. N 6 -Allyladenosine: a New Small Molecule for RNA Labeling Identified by Mutation Assay. J Am Chem Soc. 2017 Dec 6;139(48):17213–17216.
- Hu L, Liu S, Peng Y, et al. m(6)A RNA modifications are measured at single-base resolution across the mammalian transcriptome. Nat Biotechnol. 2022 Aug;40(8):1210.
- Koh CWQ, Goh YT, Goh WSS. Atlas of quantitative single-base-resolution N-6-methyl-adenine methylomes. Nat Commun. 2019 Dec 10;10(5636).
- Wei J, Liu F, Lu Z, et al. Differential m(6)A, m(6)A(m), and m(1)A Demethylation Mediated by FTO in the Cell Nucleus and Cytoplasm. Mol Cell. 2018 Sep 20;71(6):973.
- Dalhoff C, Lukinavicius G, Klimasauskas S, et al. Direct transfer of extended groups from synthetic cofactors by DNA methyltransferases. Nat Chem Biol. 2006 Jan;2(1):31–32.
- Shu X, Cao J, Cheng M, et al. A metabolic labeling method detects m(6)A transcriptome-wide at single base resolution. Nat Chem Biol. 2020 Aug;16(8):887.
- Shu X, Cao J, Liu J. m6A-label-seq: a metabolic labeling protocol to detect transcriptome-wide mRNA N6-methyladenosine (m6A) at base resolution. STAR Protocols. 2022 march 18;3(1):101096.
- Cohn WE, Volkin E. NUCLEOSIDE-5’-PHOSPHATES FROM RIBONUCLEIC ACID. Nature. 1951;167(4247):483–484.
- Wang Y, Xiao Y, Dong S, et al. Antibody-free enzyme-assisted chemical approach for detection of N-6-methyladenosine. Nat Chem Biol. 2020 Aug 1;16(8):896.
- Cao J, Shu X, Feng X-H, et al. Mapping messenger RNA methylations at single base resolution. Curr Opin Chem Biol. 2021Aug;63:28–37.
- Garalde DR, Snell EA, Jachimowicz D, et al. Highly parallel direct RNA sequencing on an array of nanopores. Nat Methods. 2018 Mar;15(3):201.
- Workman RE, Tang AD, Tang PS, et al. Nanopore native RNA sequencing of a human poly(A) transcriptome. Nat Methods. 2019 Dec;16(12):1297.
- Rang FJ, Kloosterman WP, de Ridder J. From squiggle to basepair: computational approaches for improving nanopore sequencing read accuracy. Genome Biol. 2018 Jul 13;19(90).
- Stephenson W, Razaghi R, Busan S, et al. Direct detection of RNA modifications and structure using single-molecule nanopore sequencing. Cell genomics. 2022;2(2):2022-Feb–09.
- Vilfan ID, Tsai Y-C, Clark TA, et al. Analysis of RNA base modification and structural rearrangement by single-molecule real-time detection of reverse transcription. J Nanobiotechnology. 2013 Apr 3;11(8).
- Zhang Y, Liu T, Meyer CA, et al. Model-based Analysis of ChIP-Seq (MACS). Genome Biol. 2008;9(9). 137.
- Meng J, Cui X, Rao MK, et al. Exome-based analysis for RNA epigenome sequencing data. Bioinformatics. 2013 Jun 15;29(12):1565–1567.
- Cui X, Meng J, Zhang S, et al. A novel algorithm for calling mRNA m(6)A peaks by modeling biological variances in MeRIP-seq data. Bioinformatics. 2016 Jun 15;32(12):378–385.
- Cui X, Meng J, Rao MK, et al. HEPeak: an HMM-based exome peak-finding package for RNA epigenome sequencing data. Bmc Genomics. 2015 Apr 21;16(S2).
- Antanaviciute A, et al. m6aViewer: software for the detection, analysis, and visualization of N-6-methyladenosine peaks from m(6)A-seq/ME-RIP sequencing data. Rna. 2017 Oct;23(10):1493–1501.
- Li Y, Song S, Li C, et al. MeRIP-PF: an easy-to-use pipeline for high-resolution peak-finding in MeRIP-Seq data. Genomics Proteomics Bioinformatics. 2013 Feb;11(1):72–75.
- Zhang M, Li Q, Xie Y. A Bayesian hierarchical model for analyzing methylated RNA immunoprecipitation sequencing data. Quantitative Biology. 2018 Sep;6(3):275–286.
- Guo Z, Shafik AM, Jin P, et al. Detecting m(6)A methylation regions from Methylated RNA Immunoprecipitation Sequencing. Bioinformatics. 2021 Sep 15;37(18):2818–2824.
- Zhang Y, Hamada M. MoAIMS: efficient software for detection of enriched regions of MeRIP-Seq. Bmc Bioinformatics. 2020 Mar 14;21(1):103.
- Bock C. Analysing and interpreting DNA methylation data. Nat Rev Genet. 2012 Oct;13(10):705–719.
- Meng J, Lu Z, Liu H, et al. A protocol for RNA methylation differential analysis with MeRIP-Seq data and exomePeak R/Bioconductor package. Methods. 2014 Oct 1;69(3):274–281.
- Cui X, Zhang L, Meng J, et al. MeTDiff: a Novel Differential RNA Methylation Analysis for MeRIP-Seq Data. Ieee-Acm Transactions on Computational Biology and Bioinformatics. 2018 Mar-Apr;15(2):526–534.
- Ayyala DN, Lin J, Ouyang Z. Differential RNA methylation using multivariate statistical methods. Brief Bioinform. 2022 Jan 17;23(1):309.
- Liu L, Zhang S-W, Gao F, et al. DRME: count-based differential RNA methylation analysis at small sample size scenario. Anal Biochem. 2016 Apr 15;499:15–23.
- Liu L, Zhang S-W, Huang Y, et al. QNB: differential RNA methylation analysis for count-based small-sample sequencing data with a quad-negative binomial model. Bmc Bioinformatics. 2017 Aug 31;18(387).
- Zhang Z, Zhan Q, Eckert M, et al. RADAR: differential analysis of MeRIP-seq data with a random effect model. Genome Biol. 2019 Dec 23;20(1).
- Zhang L, Meng, J, Liu, H, et al., “Detecting differentially methylated mRNA from MeRIP-Seq with likelihood ratio test,” in IEEE Global Conference on Signal and Information Processing (GlobalSIP), Atlanta, GA, 2014. Dec 03–05 2014, pp. 1368–1371
- Zhang Y-C, Zhang S-W, Liu L, et al. Spatially Enhanced Differential RNA Methylation Analysis from Affinity-Based Sequencing Data with Hidden Markov Model. Biomed Res Int. 2015;2015:852070.
- Xiao S, Cao S, Huang Q, et al. The RNA N-6-methyladenosine modification landscape of human fetal tissues. Nat Cell Biol. 2019 May;21(5):651.
- Li Y, Xia L, Tan K, et al. N-6-Methyladenosine co-transcriptionally directs the demethylation of histone H3K9me2. Nat Genet. 2020 Sep;52(9):870.
- Schwartz S, Mumbach M, Jovanovic M, et al. Perturbation of m6A Writers Reveals Two Distinct Classes of mRNA Methylation at Internal and 5 ‘ Sites. Cell Rep. 2014 Jul 10;8(1):284–296.
- Cui X, Meng J, Zhang S, et al. A hierarchical model for clustering m(6)A methylation peaks in MeRIP-seq data. Bmc Genomics. 2016 Aug 22;17(520).
- Zhang L, He Y, Wang H, et al. Clustering Count-based RNA Methylation Data Using a Nonparametric Generative Model. Curr Bioinf. 2019;14(1):11–23.
- Liu L, Zhang S-W, Zhang Y-C, et al. Decomposition of RNA methylome reveals co-methylation patterns induced by latent enzymatic regulators of the epitranscriptome. Mol Biosyst. 2015 Jan;11(1):262–274.
- Chen S, Zhang L, Lu L, et al. FBCwPlaid: a Functional Biclustering Analysis of Epi-Transcriptome Profiling Data Via a Weighted Plaid Model. Ieee-Acm Transactions on Computational Biology and Bioinformatics. 2022 May-Jun;19(3):1640–1650.
- Liu Z, Xiao Y, Yin H, et al. BDBB: a Novel Beta-Distribution-Based Biclustering Algorithm for Revealing Local Co-Methylation Patterns in Epi-Transcriptome Profiling Data. IEEE J Biomed Health Inform. 2022 Jun;26(6):2405–2416.
- Zhang L, Chen S, Ma J, et al. REW-ISA V2: a Biclustering Method Fusing Homologous Information for Analyzing and Mining Epi-Transcriptome Data. Front Genet. 2021 May 28;12(654820).
- Chen K, Wei Z, Liu H, et al. Enhancing Epitranscriptome Module Detection from m(6)A-Seq Data Using Threshold-Based Measurement Weighting Strategy. Biomed Res Int. 2018;2018:2075173.
- Helm M, Motorin Y. Detecting RNA modifications in the epitranscriptome: predict and validate,” (in English. Nat. Rev. Genet., Review, 2017 May;18(5):275–291.
- Behm-Ansmant I, Helm M, Motorin Y. Use of specific chemical reagents for detection of modified nucleotides in RNA,” (in English. J Nucleic Acids. 2011 Apr;2011:408053.
- Schwartz S, Bernstein D, Mumbach M, et al. Transcriptome-wide Mapping Reveals Widespread Dynamic-Regulated Pseudouridylation of ncRNA and mRNA,” (in English. Cell. 2014 Sep;159(1):148–162.
- Head SR, Komori HK, LaMere SA, et al. Library construction for next-generation sequencing: overviews and challenges,” (in English. Biotechniques. 2014 Feb;56(2):61.
- Ryvkin P, Leung YY, Silverman IM, et al. HAMR: high-throughput annotation of modified ribonucleotides,” (in English. Rna. 2013 Dec;19(12):1684–1692.
- Hauenschild R, Werner S, Tserovski L, et al. CoverageAnalyzer (CAn): a Tool for Inspection of Modification Signatures in RNA Sequencing Profiles,” (in English. Biomolecules. 2016 Dec;6(4):7.
- Schmidt L, Werner S, Kemmer T, et al. Graphical Workflow System for Modification Calling by Machine Learning of Reverse Transcription Signatures,” (in English. Front Genet. 2019 Sep;10(876):11.
- Motorin Y, Helm M. Methods for RNA Modification Mapping Using Deep Sequencing: established and New Emerging Technologies,” (in English. Genes, Review. 2019 Jan;10(1):12.
- Hansen KD, Brenner SE, Dudoit S. Biases in Illumina transcriptome sequencing caused by random hexamer priming,” (in English. Nucleic Acids Res. 2010 Jul 7;38(12):e131.
- Jenjaroenpun P, Wongsurawat T, Wadley TD, et al. Decoding the epitranscriptional landscape from native RNA sequences. Nucleic Acids Res, vol. 49, no. 2, 2021 Jan 25:7.
- Liu H, Begik O, Novoa EM. EpiNano: detection of m6A RNA Modifications Using Oxford Nanopore Direct RNA Sequencing. Methods Mol Biol. 2021;2298:31–52.
- Parker MT, Knop K, Sherwood AV, et al. Nanopore direct RNA sequencing maps the complexity of Arabidopsis mRNA processing and m(6)A modification. Elife. 2020 Jan 14;9:e49658.
- Abebe JS, Price AM, Hayer KE, et al. DRUMMER-rapid detection of RNA modifications through comparative nanopore sequencing. Bioinformatics. 2022 May 26;38(11):3113–3115.
- Lorenz DA, Sathe S, Einstein JM, et al. Direct RNA sequencing enables m(6)A detection in endogenous transcript isoforms at base-specific resolution. Rna. 2020 Jan;26(1):19–28.
- Stoiber M, Quick J, Egan R, et al. De novo identification of DNA modifications enabled by genome-guided nanopore signal processing. BioRxiv. 2017.
- Leger A, Amaral PP, Pandolfini L, et al. RNA modifications detection by comparative Nanopore direct RNA sequencing. Nat Commun. 2021 Dec 10;12(1).
- Pratanwanich PN, Yao F, Chen Y, et al. Identification of differential RNA modifications from nanopore direct RNA sequencing with xPore. Nat Biotechnol. 2021 Nov;39(11):1394.
- Parker MT, Barton GJ, Simpson GG. Yanocomp: robust prediction of m6A modifications in individual nanopore direct RNA reads. bioRxiv. 2021.
- Nguyen TA, Heng JWJ, Kaewsapsak P, et al. Direct identification of A-to-I editing sites with nanopore native RNA sequencing. Nat Methods. 2022 Jul;19(7):833.
- Ramasamy S, Sahayasheela VJ, Sharma S, et al. Chemical Probe-Based Nanopore Sequencing to Selectively Assess the RNA Modifications. ACS Chem Biol. 2022 Oct 21;17(10):2704–2709.
- Begik O, Lucas MC, Pryszcz LP, et al. Quantitative profiling of pseudouridylation dynamics in native RNAs with nanopore sequencing. Nat Biotechnol. 2021 Oct;39(10):1278.
- Fu LM, Niu BF, Zhu ZW, et al. CD-HIT: accelerated for clustering the next-generation sequencing data,” (in English. Bioinformatics. 2012 Dec;28(23):3150–3152.
- Chen Z, Zhao P, Li F, et al. Comprehensive review and assessment of computational methods for predicting RNA post-transcriptional modification sites from RNA sequences. Brief Bioinform. 2020 Sep;21(5):1676–1696.
- Liu B. BioSeq-Analysis: a platform for DNA, RNA and protein sequence analysis based on machine learning approaches. Brief Bioinform. 2019 Jul;20(4):1280–1294.
- Liu B, Gao X, Zhang H. BioSeq-Analysis2.0: an updated platform for analyzing DNA, RNA and protein sequences at sequence level and residue level based on machine learning approaches. Nucleic Acids Res. 2019 Nov 18;47(20):e127–e127.
- Muhammod R, Ahmed S, Farid DM, et al. PyFeat: a Python-based effective feature generation tool for DNA, RNA and protein sequences. Bioinformatics. 2019 Oct 1;35(19):3831–3833.
- Li H-L, Pang Y-H, Liu B. BioSeq-BLM: a platform for analyzing DNA, RNA and protein sequences based on biological language models. Nucleic Acids Res. 2021 Dec 16;49(22):e129–e129.
- Peng HC, Long FH, Ding C. Feature selection based on mutual information: criteria of max-dependency, max-relevance, and min-redundancy. IEEE Trans Pattern Anal Mach Intell. 2005 Aug;27(8):1226–1238.
- Zhu X, He J, Zhao S, et al. A comprehensive comparison and analysis of computational predictors for RNA N6-methyladenosine sites of Saccharomyces cerevisiae. Brief Funct Genomics. 2019 Nov;18(6):367–376.
- El Allali A, Elhamraoui Z, Daoud R. Machine learning applications in RNA modification sites prediction. Comput Struct Biotechnol J. 2021;19:5510–5524.
- Chen X, Sun Y-Z, Liu H, et al. RNA methylation and diseases: experimental results, databases, Web servers and computational models. Brief Bioinform. 2019 May;20(3):896–917.
- Chen K, et al. WHISTLE: a high-accuracy map of the human N-6-methyladenosine (m(6)A) epitranscriptome predicted using a machine learning approach. Nucleic Acids Res. 2019 Apr 23;47(7):e41–e41.
- Dao F-Y, Lv H, Yang Y-H, et al. Computational identification of N6-methyladenosine sites in multiple tissues of mammals. Comput Struct Biotechnol J. 2020;18:1084–1091.
- Chen W, Tang H, Lin H. MethyRNA: a web server for identification of N-6-methyladenosine sites. J Biomol Struct Dyn. 2017;35(3):683–687.
- Zhou Y, Zeng P, Li Y-H, et al. SRAMP: prediction of mammalian N 6 -methyladenosine (m 6 A) sites based on sequence-derived features. Nucleic Acids Res. 2016 Jun 2;44(10):e91–e91.
- Zhao Z, Peng H, Lan C, et al. Imbalance learning for the prediction of N-6-Methylation sites in mRNAs. Bmc Genomics. 2018 Aug 1;19(574).
- Qiang X, Chen H, Ye X, et al. M6AMRFS: robust Prediction of N6-Methyladenosine Sites With Sequence-Based Features in Multiple Species. Front Genet. 2018 Oct 25;9(495).
- Zou Q, Xing P, Wei L, et al. Gene2vec: gene subsequence embedding for prediction of mammalian N-6-methyladenosine sites from mRNA. Rna. 2019 Feb;25(2):205–218.
- Huang Y, He N, Chen Y, et al. BERMP: a cross-species classifier for predicting m(6)A sites by integrating a deep learning algorithm and a random forest approach. Int J Biol Sci. 2018;14(12):1669–1677.
- Ke S, Alemu EA, Mertens C, et al. A majority of m 6 A residues are in the last exons, allowing the potential for 3’ UTR regulation. Genes Dev. 2015 Oct 1;29(19):2037–2053.
- Mendel M, Delaney K, Pandey RR, et al. Splice site m(6)A methylation prevents binding of U2AF35 to inhibit RNA splicing. Cell. 2021 Jun 10;184(12):3125.
- Koertel N, Rueckle C, Zhou Y, et al. Deep and accurate detection of m(6)A RNA modifications using miCLIP2 and m6Aboost machine learning. Nucleic Acids Res. 2021 Sep 20;49(16):92.
- Huang D, Chen K, Song B, et al. Geographic encoding of transcripts enabled high-accuracy and isoform-aware deep learning of RNA methylation. Nucleic Acids Res. 2022 Oct 14;50(18):10290–10310.
- Zhang L, Li G, Li X, et al. EDLm(6)APred: ensemble deep learning approach for mRNA m(6)A site prediction. Bmc Bioinformatics. 2021 May 29;22(1):288.
- Wang H, Liu H, Huang T, et al. EMDLP: ensemble multiscale deep learning model for RNA methylation site prediction. Bmc Bioinformatics. 2022 Jun 8;23(1):221
- Xiong Y, He X, Zhao D, et al. Modeling multi-species RNA modification through multi-task curriculum learning. Nucleic Acids Res. 2021 Apr 19;49(7):3719–3734.
- Huang D, Song B, Wei J, et al. Weakly supervised learning of RNA modifications from low-resolution epitranscriptome data. Bioinformatics. 2021 Jul;37(Supplement_1):I222–I230.
- Liang Z, Zhang L, Chen H, et al. m6A-Maize: weakly supervised prediction of m(6)A-carrying transcripts and m(6)A-affecting mutations in maize (Zea mays). Methods. 2022Jul;203:226–232.
- Song Z, Huang D, Song B, et al. Attention-based multi-label neural networks for integrated prediction and interpretation of twelve widely occurring RNA modifications. Nat Commun. 2021 Jun 29;12(1):4011.
- Zhang Y, Hamada M. DeepM6ASeq: prediction and characterization of m6A-containing sequences using deep learning. Bmc Bioinformatics. 2018 Dec 31;19(524).
- Siepel A, Bejerano G, Pedersen JS, et al. Evolutionarily conserved elements in vertebrate, insect, worm, and yeast genomes. Genome Res. 2005 Aug;15(8):1034–1050.
- Sanger HL, Klotz G, Riesner D, et al. VIROIDS ARE SINGLE-STRANDED COVALENTLY CLOSED CIRCULAR RNA MOLECULES EXISTING AS HIGHLY BASE-PAIRED ROD-LIKE STRUCTURES. Proc Natl Acad Sci U S A. 1976;73(11):3852–3856.
- Hsu MT, Cocaprados M. Electron-Microscopic Evidence For The Circular Form Of Rna In The Cytoplasm Of Eukaryotic Cells. Nature. 1979;280(5720):339–340.
- Chen -L-L, Yang L. Regulation of circRNA biogenesis. RNA Biol. 2015 Apr;12(4):381–388.
- Sirkisoon SR, Carpenter RL, Rimkus T, et al. TGLI1 transcription factor mediates breast cancer brain metastasis via activating metastasis-initiating cancer stem cells and astrocytes in the tumor microenvironment. Oncogene. 2020 Jan;39(1):64–78.
- Cardamone G, Paraboschi EM, Rimoldi V, et al. The Characterization of GSDMB Splicing and Backsplicing Profiles Identifies Novel Isoforms and a Circular RNA That Are Dysregulated in Multiple Sclerosis. Int J Mol Sci. 2017Mar;18(3):576.
- Dube U, Del-Aguila JL, Li Z, et al. An atlas of cortical circular RNA expression in Alzheimer disease brains demonstrates clinical and pathological associations. Nat Neurosci. 2019 Nov;22(11):1903.
- Meyer KD, Jaffrey SR. The dynamic epitranscriptome: n-6-methyladenosine and gene expression control. Nat Rev Mol Cell Biol. 2014 May;15(5):313–326.
- Li Z, Yang H-Y, Dai X-Y, et al. CircMETTL3, upregulated in a m6A-dependent manner, promotes breast cancer progression. Int J Biol Sci. 2021;17(5):1178–1190.
- Chen C, Yuan W, Zhou Q, et al. N6-methyladenosine-induced circ1662 promotes metastasis of colorectal cancer by accelerating YAP1 nuclear localization. Theranostics. 2021;11(9):4298–4315.
- Wu Q, Yin X, Zhao W, et al. Molecular mechanism of m(6)A methylation of circDLC1 mediated by RNA methyltransferase METTL3 in the malignant proliferation of glioma cells. Cell Death Discov. 2022 Apr 26;8(1): 229.
- Wu A, Hu Y, Xu Y, et al. Methyltransferase-Like 3-Mediated m6A Methylation of Hsa_circ_0058493 Accelerates Hepatocellular Carcinoma Progression by Binding to YTH Domain-Containing Protein 1. Front Cell Dev Biol. 2021 Nov 23;9(762588).
- Yang Y, Fan X, Mao M, et al. Extensive translation of circular RNAs driven by N-6-methyladenosine. Cell Res. 2017 May;27(5):626–641.
- Zhou C, Molinie B, Daneshvar K, et al. Genome-Wide Maps of m6A circRNAs Identify Widespread and Cell-Type-Specific Methylation Patterns that Are Distinct from mRNAs. Cell Rep. 2017 Aug 29;20(9):2262–2276.
- Ye Y, Feng W, Zhang J, et al. Genome-wide identification and characterization of circular RNA m(6)A modification in pancreatic cancer. Genome Med. 2021 Nov 19;13(1): 183.
- Liang W, et al. Microarray and bioinformatic analysis reveal the parental genes of m6A modified circRNAs as novel prognostic signatures in colorectal cancer. Front Oncol. 2022 Jul 29;12(939790).
- Fan H-N, Chen Z-Y, Chen X-Y, et al. METTL14-mediated m(6)A modification of circORC5 suppresses gastric cancer progression by regulating miR-30c-2-3p/AKT1S1 axis. Mol Cancer. 2022 Feb 14;21(1:51.
- Ivanov YD, et al. Nanoribbon-Based Electronic Detection of a Glioma-Associated Circular miRNA. Biosensors-Basel. 2021 Jul;11(7):237.
- Ivanov YD, Malsagova KA, Popov VP, et al. Micro-Raman Characterization of Structural Features of High-k Stack Layer of SOI Nanowire Chip, Designed to Detect Circular RNA Associated with the Development of Glioma. Molecules. 2021Jun;261:3715.
- Sun W-J, Li J-H, Liu S, et al. RMBase: a resource for decoding the landscape of RNA modifications from high-throughput sequencing data. Nucleic Acids Res. 2016 Jan 4;44(D1):D259–D265.
- Xuan -J-J, Sun W-J, Lin P-H, et al. RMBase v2.0: deciphering the map of RNA modifications from epitranscriptome sequencing data. Nucleic Acids Res. 2018 Jan 4;46(D1):D327–D334.
- Machnicka MA, Milanowska K, Osman Oglou O, et al. MODOMICS: a database of RNA modification pathways-2013 update. Nucleic Acids Res. 2013 Jan;41(D1):D262–D267.
- Boccaletto P, Machnicka MA, Purta E, et al. MODOMICS: a database of RNA modification pathways. 2017 update. Nucleic Acids Res. 2018 Jan 4;46(D1):D303–D307.
- Wishart DS, Feunang YD, Marcu A, et al. HMDB 4.0: the human metabolome database for 2018. Nucleic Acids Res. 2018 Jan 4;46(D1):D608–D617.
- Kim S, Chen J, Cheng T, et al. PubChem in 2021: new data content and improved web interfaces. Nucleic Acids Res. 2021 Jan 8;49(D1):D1388–D1395.
- Mendez D, Gaulton A, Bento AP, et al. ChEMBL: towards direct deposition of bioassay data. Nucleic Acids Res. 2019 Jan 8;47(D1):D930–D940.
- Tang Y, Chen K, Song B, et al. m(6)A-Atlasm6A-Atlas: a comprehensive knowledgebase for unraveling the N-6-methyladenosine N 6-methyladenosine (m6A) epitranscriptome. Nucleic Acids Res. 2021 Jan 8;49(D1):D134–D143.
- Zhou D, Wang H, Bi F, et al. M6ADD: a comprehensive database of m 6 A modifications in diseases. RNA Biol. 2021 Dec 2;18(12):2354–2362.
- Zheng Y, Nie P, Peng D, et al. m6AVar: a database of functional variants involved in m(6)A modification. Nucleic Acids Res. 2018 Jan 4;46(D1):D139–D145.
- Liu S, Chen L, Zhang Y, et al. M6AREG: m(6)A-centered regulation of disease development and drug response. Nucleic Acids Res. 2022.
- Liu S, Zhu A, He C, et al. REPIC: a database for exploring the N-6-methyladenosine methylome. Genome Biol. 2020 Apr 28;21(1:100.
- Liu H, Flores MA, Meng J, et al. MeT-DB: a database of transcriptome methylation in mammalian cells. Nucleic Acids Res. 2015 Jan 28;43(D1):D197–D203.
- Deng S, Zhang H, Zhu K, et al. M6A2Target: a comprehensive database for targets of m 6 A writers, erasers and readers. Brief Bioinform. 2021;22(3).
- Thuring K, Schmid K, Keller P, et al. LC-MS Analysis of Methylated RNA. Methods Mol Biol. 2017;1562:3–18.
- Wang Y, Li Y, Toth JI, et al. N-6-methyladenosine modification destabilizes developmental regulators in embryonic stem cells. Nat Cell Biol. 2014 Feb;16(2):191–198.
- Liu N, Parisien M, Dai Q, et al. Probing N-6-methyladenosine RNA modification status at single nucleotide resolution in mRNA and long noncoding RNA. Rna. 2013 Dec;19(12):1848–1856.
- Liu W, Yan J, Zhang Z, et al. Identification of a selective DNA ligase for accurate recognition and ultrasensitive quantification of N-6-methyladenosine in RNA at one-nucleotide resolution. Chem Sci. 2018 Apr 7;9(13):3354–3359.
- Xiao Y, Wang Y, Tang Q, et al. An elongation- and ligation-based qPCR amplification method for the radiolabeling-free detection of locus-specific N-6-methyladenosine modification. Angew Chem-Int Ed. 2018 Dec 3;57(49):15995–16000.
- Kumari A, Lobiyal DK. Efficient estimation of Hindi WSD with distributed word representation in vector space. Journal of King Saud University-Computer and Information Sciences. Sep 2022;34(8):6092–6103.
- Dai H, Umarov R, Kuwahara H, et al. Sequence2Vec: a novel embedding approach for modeling transcription factor binding affinity landscape. Bioinformatics. 2017 Nov 15;33(22):3575–3583.