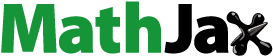
ABSTRACT
Alternative splicing is a process causing mRNA translation to produce different proteins, and it is crucial for the development of tumours. In this study, we constructed a prognostic model related to alternative splicing events in hepatocarcinoma using bioinformatics analysis, including the alternative splicing of CSAD, AFMID, ZDHHC16, and IRF3. The model is an independent prognostic factor and can accurately predict a patient’s prognosis. IRF3 is a transcription factor related to the immune response. Its alternative splicing can affect the expression of various genes related to prognosis and plays an essential role in the tumour microenvironment. We also verified the expression of IRF3 exon skipping isoform in hepatocarcinoma at the mRNA level. In conclusion, we discovered that the alternative splicing of IRF3 is essential for the development of hepatocarcinoma. This study provides new insight into the development of treatments for hepatocarcinoma.
Introduction
Hepatocellular carcinoma (HCC) is a prevalent malignant and invasive tumour. Early-stage tumours can be effectively treated with surgery, interventional chemotherapy, radiofrequency ablation or even liver transplantation. However, following the tumour has spread, these therapies are no longer effective [Citation1]. Therefore, early detection and precision therapy are particularly important for HCC. Several valuable markers for early HCC identification have been discovered [Citation2,Citation3], while the effect remains to be considered. As a result, new strategies for early diagnosis and effective treatment should be further explored.
Alternative splicing (AS) is considered as a key factor in expanding the complexity of cell functions. AS of mRNA precursor molecules can produce a variety of mRNA and protein isoforms that differ in structure, location, and function [Citation4]. The existence of AS provides a molecular basis for the diversity of biological phenotypes, and it also participates in and affects the progression of diseases, as evidenced by tumour research. Th1 cells, for example, can generate the replacement subtype of IRF1, decrease IFN γ secretion, and influence the anti-tumour effect [Citation5]. AS may also alter the protein function and influence the incidence of HCC [Citation6,Citation7]. For instance, muscle blind splicing regulator 3 (MBNL3) can induce the transcript of lncRNA-PXN-AS1 containing exon 4, which can prevent the degradation of paxillin (PXN) and promote the occurrence of HCC [Citation8]. AS has also become a novel target for tumour therapy [Citation9–11], due to its critical function in tumour proliferation and invasion [Citation12].
Approximately 90% of HCC cases are accompanied by chronic liver disease, which is caused by excessive drinking, viral hepatitis, and inflammation caused by non-alcoholic fatty liver disease [Citation13]. The liver, being the most significant metabolic organ, is impacted by a variety of external factors. Chronic hepatitis is frequently caused by immune cell activation, the production of pro-inflammatory factors, the accumulation of exogenous toxins, and an excess of metabolic products. Chronic inflammation may alter immunological modulation and impact the development of liver cancer [Citation14]. The immune microenvironment can induce alterations in alternative splicing types to ensure the survival advantage of cancer cells [Citation15]. Therefore, the immune microenvironment plays a crucial role in the progression of HCC.
In this research, we structured a prognostic model for HCC based on AS events. The exon skip event of IRF3, an immune-related gene, was incorporated in the model Therefore, we thoroughly analysed the IRF3 AS events and explored their relationship with tumour microenvironment. This study provides new insight into the early diagnosis, prognosis assessment and targeted treatment of HCC.
Material and methods
Data acquisition and sorting
The transcriptome and clinical data were downloaded from LIHC patients in The Cancer Genome Atlas (TCGA) database. The TCGA SpliceSeq database was used to download the alternative splice events data. The events, which have a percent-splice-in (PSI) value in 75% of the samples, meet screening criteria. The exon structure of IRF3, the function of the corresponding region, and the isoforms of IRF3 were derived from the Ensembl database and the UniProt protein database. The IRF3 target gene set was from the GSE31477 data set which was selected in the Htftarget database [Citation16].
Screening of survival-related exon skip events
ES (exon skip), AD (alternate donor site), AP (alternate promoter), AA (alternate acceptor site), AT (alternate terminator), ME (mutually exclusive exons) and RI (retained intron) are the seven types of AS events in TCGA SpliceSeq database. The AS events are named by combining the corresponding parent gene, ID number and splicing type. As an illustration, consider the meaning of ‘IRF3 | 50994 | ES,’ where IRF3 represents the corresponding gene 50,994 for the ID number, and ES for the splicing type. The KNN algorithm was used to fill the data gaps, and the events with a standard deviation of PSI < 0.05 were eliminated. ES events were combined with the survival time of clinical data, and a univariate Cox analysis was conducted to screen survival-related ES events. The UpSet plot diagram is depicted through the ‘upsetR’ package.
Construction of the prognostic model and verification of its independence
LASSO regression analysis was used to screen candidate ES events to prevent over fitting of the model. The risk score of each prognostic predictor was calculated by multivariate Cox regression analysis, which was also utilized to screen prognostic predictors. The formula was as follows: . The ESi represents the ith prognostic ES events, PSI (ESi) is the PSI value of the ESi, and coef (ESi) is obtained by the regression coefficient of the multivariate Cox analysis, which represents the contribution of ESi. This method served as the foundation for our prognostic. Kaplan-Meier survival curves were analysed with the ‘survival’ R package. The ROC curve was created with the ‘timeROC’ R package. Cox regression analyses and stratified survival analyses were performed to verify the independence of this model.
Differentially expressed genes were screened for functional enrichment and prognostic analysis
Two groups of patients were established based on IRF3 expression. 25 patients were chosen as the high ES group and 26 patients as the low ES group based on the proportion of IRF3 ES events. Differentially expressed genes were screened with a cut-off of |logFC|>1 and FDR < 0.05 through the ‘limma’ R package. If logFC > 1, the gene was upregulated in the high ES group, and if logFC < −1, the gene was downregulated. The functional enrichment analysis was performed on the differentially expressed genes through the Metascape website (http://metascape.org). With the use of the GEPIA database and the Kaplan-Meier plotter database, the expression and prognosis of the intersection genes between differentially expressed genes and IRF3 target genes were examined.
Correlation among IRF3 expression, ES events and immune checkpoint genes
The Timer2.0 database was used to evaluate the correlation between IRF3 and tumour immune microenvironment. The correlation coefficient among IRF3 expression, IRF3 ES event proportion and immune checkpoints genes expression were calculated by ‘ggcorrplot’ R package.
cDNA microarray and real-time PCR analysis
The liver cancer cDNA microarray, containing 66 samples, was purchased from Shanghai Outdo Biotech co., Ltd (Cat. No.: MecDNA-HLivH087Su02; Shanghai, China). The clinical information of these patients was displayed in supplementary table S1. We designed characteristic primers according to the exon ENSE0002224211 missing or not. The specific primers were as follows: IRF3-IN: forward 5’- CGACA ATCCC ACTCC CTTCC-3“ and reverse 5”-CGACC CCACC AGCCG CAG-3;“ IRF3-EX: forward 5”-AAGCG GGGAA GATCT GATTAC-3“ and reverse 5”-GAGAG TGGGT GGCTG TGGGA-3.’ According to the instructions, the ChamQ Universal SYBR qPCR Master Mix (Vazyme, Q711–02) and Roche LightCycler 480-II instrument (Roche) were used for quantitative polymerase chain reaction (qPCR). In all of the samples, we measured the mRNA expression levels of IRF3-IN and EX isoforms relative to ACTIN. The relative mRNA expression was determined using the cycle threshold (CT) formula: , where
. The formula for calculating the proportion of IRF3-EX isoform in IRF3 expression was calculated by
.
Statistics analysis
The differences between the two groups were evaluated using the Wilcoxon test. The period from the date of diagnosis to the date of death is known as overall survival. The survival curve was drawn with the Kaplan-Meier log-rank test. The relations were evaluated with the Pearson correlation test. Univariate and multivariate analyses were performed by the Cox regression model. The prognostic value of the prognostic model was evaluated by ROC curve analysis. The p < 0.05 indicates that the difference is statistically significant. All statistical calculations were performed using R software (version 4.0.2).
Results
Exon skip events in HCC are the main component of alternative splicing
We downloaded TCGA-LIHC AS events from the TCGA SpliceSeq and visualized them with an UpSet plot (Fig. S1A). The AS data of 352 patients were retained after being combined with the clinical data and eliminating patient information with seriously incomplete PSI values. Using a univariate Cox analysis, we selected AS events related to prognosis and drew an Upset plot (). The findings indicate that ES events accounted for the central part.
Figure 1. Construction of ES event prediction model.
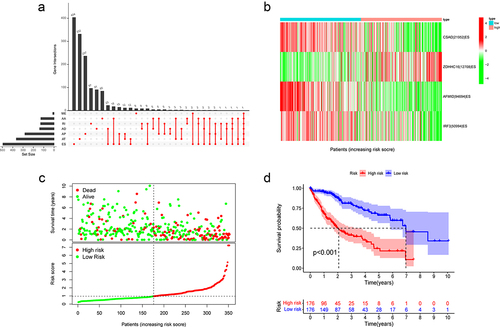
Constructing a prognostic model with ES events
Four ES events were included in the model to assess the prognosis using LASSO regression analysis and multivariate Cox analysis (). PSI value represents the proportion of the exon retained. Therefore, the exon retention of ZDHHC16 is a risk factor, whereas the exon retention of CSAD, AFMID and IRF3 are protective factors for the prognosis of HCC. We calculated the risk score of each patient and divided them into two groups according to the median value. The PSI value of ZDHHC16 in the high-risk group was higher, while the PSI values of the other three genes were the opposite (). The survival time was decreased with increasing scores (). There was a substantial difference in survival between the two groups, with a poor prognosis in the high-risk group ().
Table 1. The prognostic model related to exon skip events.
Independent validation of the model
We subsequently examined the ROC curve for the purpose to assess the effectiveness of this prognostic model. The AUCs of 1-, 2- and 3-year survival were 0.779, 0.762, and 0.753, respectively, indicating that the model was effective at predicting prognosis (). In addition, univariate and multivariate Cox regression analyses explained that this model might act as an independent prognostic indicator in HCC (). The prognosis is often impacted by stage and age. Therefore, we stratified the patients according to age and stage and verified the model’s predictive accuracy. The prognosis in the high-risk group was worse than in the low-risk group (). Then, we investigated into the association between the model and clinical features. The risk score was gradually increased with increasing tumour grade and pathological stage, (). This model has the greatest accuracy for prediction compared with the ROC curve of other clinical features ().
Figure 2. The prediction ability and independent verification of the model.
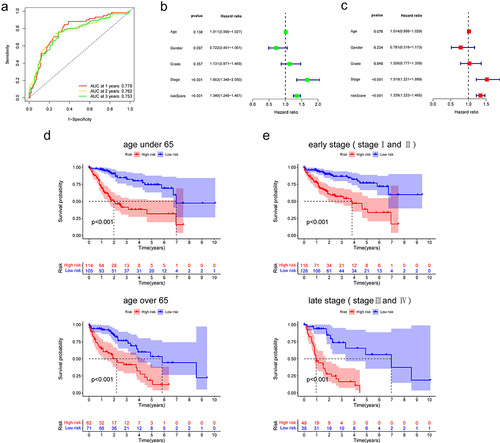
Figure 3. Correlation of model with clinical features.
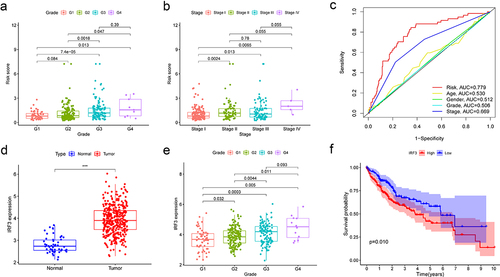
The function and expression of genes in the prognostic model
Three of the four genes studied in this model are CSAD, which is involved in the metabolism of synthesizing L-cysteine into hypotaurine, ZDHHC16, which is engaged in protein palmitoylation, and AFMID, which is involved in the subpathway of converting L-tryptophan into L-kynurenine. These three genes are all related to the protein metabolism pathway, whereas IRF3 participates in innate immune response and induces IFN expression. In addition, IRF3 is a transcription factor that can activate or repress protein expression. When we compared the expression of these genes between tumour and normal tissues, we found that only IRF3 was upregulated in tumours while the expression of other genes remained the same according to TCGA data (). The prognosis for patients with high IRF3 expression was poor (). Moreover, the expression of IRF3 was related to tumour grade (), but not stage (Fig. S1B). We speculate that the increased proportion of IRF3 ES events affects the function of IRF3.
The type of ES events in IRF3
Seven ES events related to IRF3 were discovered when we reviewed the ES events associated with prognosis from the previous analysis (). We revealed that the proportion of ES events arose as IRF3 expression increased, and some ES events were strongly correlated with one another by analysing the correlation between IRF3 expression and the proportion of ES events (). We validated the nucleotide and amino acid sequences of these events in the TCGA SpliceSeq database. The amino acid sequence mainly involved three exons: ENSE00003686782, ENSE00003656814, and ENSE00002224211 by searching the Ensembl database (). Two IRF3 isoforms, searching the UniProt protein database, were identified as meeting the ES conditions (). Both isoforms lack exon ENSE00002224211, which binds to HERC5. It is hypothesized that the deletion of this exon may have an impact on ISG15 binding and protein function since it contains ISG15 binding sites at both ends. The exons of ENSE00003686782 and ENSE00003656814 form the DNA binding region, and deletion of the region may disrupt IRF3 regulatory function.
Figure 4. The structure and isoforms of IRF3.
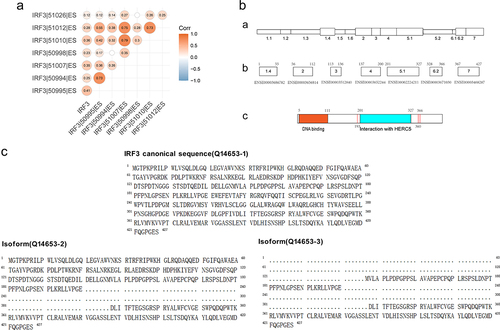
Table 2. The IRF3 ES events associated with prognosis.
The proportion of ES events related to IRF3 affects gene expression
We further explored whether the proportion of ES events related to IRF3 would affect gene expression. Based on the correlation between IRF3 and its ES events, the patients with PSI values of IRF3|50995|ES, IRF3|50994|ES, IRF3|51007|ES, IRF3|51010|ES, and IRF3|51012|ES are 100% were considered as the low ES group (26 patients). Due to both two IRF3 isoforms missing the exon ENSE0002224211, the patients with PSI values of IRF3|50995|ES and IRF3|50994|ES less than 75% were regarded as the high ES group (25 patients). The analysis of differentially expressed genes showed that 255 genes were upregulated and 175 genes were downregulated in the high ES group ( and Fig. S1C). And the overall survival indicated that the high ES group had a worse prognosis (). These genes were connected to the cell cycle, carbon metabolism, apoptosis, and DNA replication according to GO analysis and KEGG analysis (). Then we searched the target genes of IRF3 in HepG2 cells through the Htftarget database. Seventeen genes that intersect in two sets were examined (). Additionally, the expression and prognosis of these 17 genes were queried through the GEPIA and Kaplan-Meier plotter databases (). Surprisingly, 15 genes upregulated in the high ES group were highly expressed in tumour tissues, and the majority of them were linked to poor prognosis. The two genes upregulated in the low ES group were highly expressed in normal tissues and correlated with a better prognosis. This suggests that the proportion of IRF3 ES events may significantly impact the gene expression of HCC.
Figure 5. Screening and grouping of patients based on the proportion of IRF3 ES events.
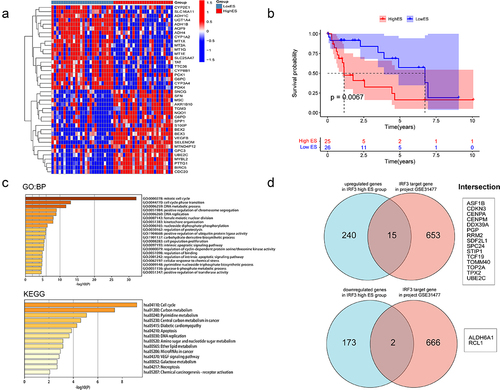
Figure 6. Expression and prognosis of differentially expressed target genes in HCC.
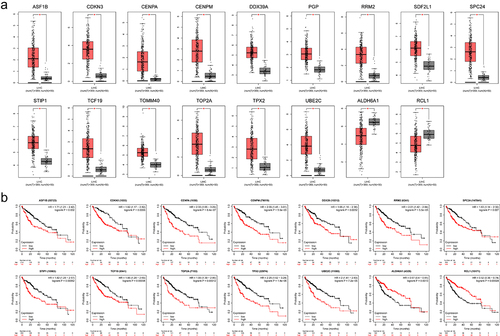
Correlation of IRF3 ES events with tumor immune characterization
During tumorigenesis, DNA damage can promote the activation of an inflammatory response by activating the cGAS-STING-IRF3 pathway [Citation17]. Through the Timer2 database, we discovered that IRF3 was positively correlated with various immune cells, including inhibitory immune cells such as M2 and Treg cells (). In addition, we observed a connection between IRF3 expression and the expression of multiple immune checkpoint genes ( and Fig. S2). We screened the immune checkpoint genes with a correlation coefficient above 0.2 and found that these gene expressions were also associated with the proportion of ES events. Moreover, DNA binding region deletion-related ES events were relatively more strongly correlated ().
Figure 7. Correlation of IRF3 and its ES events with tumour immune characterization.
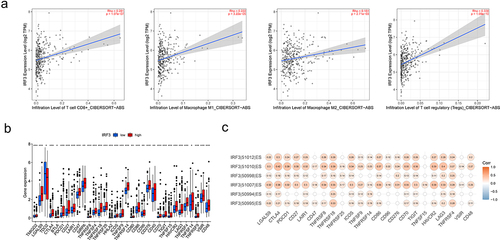
Detection of IRF3 exon skipping by cDNA microarray
We named exon ENSE0002224211 skipping isoform as IRF3-EX (represents two splicing variants of IRF-3 in , Q14653–2 and Q14653–3), and exon ENSE0002224211 included isoform as IRF3-IN (represents the normal form of IRF3, Q14653–1). A cDNA microarray containing the clinical data of 66 liver cancer patients was used to identify the relative expression levels of the two IRF3 isoforms (, Fig. S3A). The IRF3-EX was prevalent in 66 patients, and its relative expression level was significantly positively correlated with the total relative expression level of IRF3 (Fig. S3B). depicted the percentage of IRF3-EX, and showed a positive relationship between it and the overall relative expression level of IRF3. A univariate Cox analysis displayed that the proportion of IRF3-EX was a risk factor for prognosis (). Although there was no statistically significant difference in the proportion of IRF3-EX below 10% (20 patients) and beyond 15% (14 patients), the overall survival of patients with high IRF3-EX proportion was inferior (Fig. S3C).
Figure 8. Detection of IRF3 exon skipping by cDNA microarray.
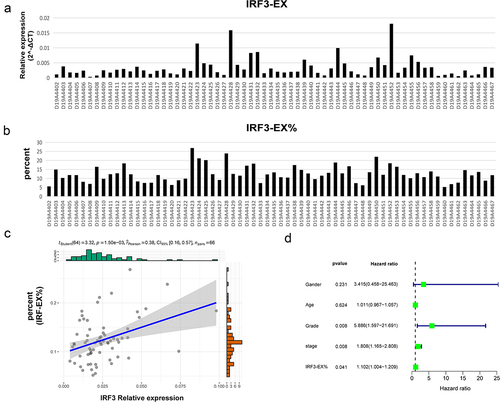
Discussion
HCC is one of the most widespread clinical malignancies, and it is impacted by the genes, transcription, and epigenetic modification of tumour cells. Its high heterogeneity leads to a poor prognosis. Early detection, diagnosis, and treatment have been identified as therapeutic principles for treating liver cancer. From the standpoint of epigenetics, there are currently numerous prognostic models, such as the lncRNA [Citation18] and N6-methyladenosine (m6A) modification [Citation19] models. These models are crucial for the early diagnosis and prognosis evaluation of HCC.
In contrast to epigenetics, AS is a process following gene transcription. It is regulated by splice factors, which produce a diversity of proteins. Mutations in some splice factors lead to changes in splicing patterns and further induce the occurrence and development of HCC [Citation20]. Poly(rC) binding protein 1 can regulate the AS of various cancer-related genes in HCC [Citation21], and ATP-dependent RNA helicase MTR4 can drive liver cancer metabolism by controlling the AS of essential glycolytic genes [Citation22]. Therefore, we analysed the AS events in HCC and constructed a prognostic model based on ES events. The model shows excellent prediction capacity.
Changes in the AS pattern can affect the function and even guide treatment. The lncRNA CRNDE can reduce the chemoresistance of gastric cancer by inducing SRSF6 to regulate the skipping of exon 14 of PICALM [Citation23]. Targeted skipping of exon 17 of NF1 can treat type I neurofibromatosis [Citation24]. Therefore, we investigated how these four genes functioned in the model. CSAD is considered the rate-limiting enzyme for taurine synthesis. In HepG2 cells, taurine can inhibit the expression of key glycolytic enzymes to suppress proliferation [Citation25], and enhance the effect of tumour chemotherapy drugs [Citation26]. ZDHHC16 regulates the palmitoylation of proteins, which is essential to signal transduction of cancer cells [Citation27]. AFMID is involved in the metabolism of kynurenine, which can promote the invasion, metastasis, and drug resistance of tumour cells [Citation28]. These three genes participate in protein metabolism. IRF3 is a transcription factor and plays a central role in innate immunity [Citation29,Citation30], and has been linked to the anti-cancer immune response [Citation31]. Therefore, we focused on the role of IRF3 ES events in HCC.
The sequence of amino acids constitutes the primary structure of the protein, and the subsequent interactions between these amino acids form the protein domains that can determine the function of the protein. IRF3 has a DNA binding region, a disordered region, a mediating interaction with ZDHHC11 region, an interaction with HERC5 region and a nuclear export signal region according to the UniProt. The skipping of exons will affect the composition of domains, and may potentially result in proteins losing their corresponding functions. There are seven types of IRF3 ES events related to the prognosis of HCC, involving the deletion of three exons. The exons ENSE00003686782 and ENSE00003656814 form the DNA binding region, which can bind to the interferon-stimulated response element (ISRE) in the target promoter region and regulate the expression of related genes [Citation32]; Exon ENSE00002224211 can bind to the E3 ligase HERC5, in which ISG15 binding regions exist at both ends. ISG15 can result in ISGlyation of the target protein, which can influence the activity and localization of the target protein, whereas HERC5 can catalyse the coupling of ISG15 and IRF3 and induce the continuous activation of IRF3 [Citation33]. The deletion of these exons may consequently result in a reduction in the transcriptional activity of IRF3 and affect the expression of downstream target proteins. Currently, it has confirmed that the IRF-3 alternatively spliced isoform can regulate the function of IRF3 [Citation34]. Additionally, the splicing isoforms of IRF3 have an obvious cancer-specific pattern in HCC, and can compete with the standard IRF3 protein to bind to the target promoter, inhibiting the transcriptional activation ability [Citation35].
The pathway enrichment of differentially expressed genes revealed that the group with a high proportion of IRF3 ES events had higher proliferative and metabolic capacities. The effects of these genes on HCC have been confirmed by research. RCL1 and ALDH6A1 were downregulated in the high ES group. RCL1 can regulate the cell cycle and restrain the growth and metastasis of HCC cells [Citation36]. ALDH6A1 is a mitochondrial protein that participates in mitochondrial respiration. Hepatic neoplastic transformation suppresses the expression of ALDH6A1, which influences mitochondrial function [Citation37]. DDX39 is one of the upregulated genes that promote the development of HCC by activating the Wnt/β-Catenin pathway [Citation38]. ASF1B, CDKN3, and CENPA can enhance cell proliferation and affect cancer development [Citation39–41]. RRM2 has the ability to control nucleotide metabolism and promote tumour proliferation [Citation42]. SPC24 and TPX2 can affect the PI3K/AKT pathway to support in tumour progression [Citation43,Citation44]. STIP1 can promote HCC development through the PI3K-Akt-dependent anti-apoptotic pathway [Citation45]. TCF19 can control the progression of HCC by binding to histone H3K4me3 [Citation46], interacting with p53 to mediate metabolic regulation [Citation47]. TOP2A may trigger EMT to accelerate the metastasis of HCC by activating the p-ERK1/2/p-Smad2/Snail pathway [Citation48]. These results suggest that the ES events of IRF3 May affect the development of HCC.
The immunological effects of IRF3 have been the primary subject of existing research. IRF3 regulates the transcription of type I IFN genes and IFN-stimulated genes (ISG) by combining the ISRE in their promoters [Citation49,Citation50]. We discovered that the expression of IRF3 was positively interrelated with the infiltration of cells that inhibited tumour immunity, such as Treg cells and M2 macrophages. Moreover, the IRF3 ES events were also interrelated with the expression of immune checkpoint genes. Therefore, in addition to interferon related pathways, IRF3 May affect the tumour immune response in other ways.
In addition, we found that IRF3-EX subtypes influenced the prognosis of patients, and the patients with a proportion over 25% had a worse prognosis than the patients with a proportion is 0 in TCGA-LIHC patients. Furthermore, the IRF3-EX was prevalent in the cDNA microarray (MecDNA-HLivH087Su02), and the patients with a proportion over 15% indicated a trend of poorer prognosis than the patients with a proportion below 10%, although there is no statistical significance. Therefore, a larger sample size is required for the analysis of the relationship between IRF3-EX proportion and prognosis in a larger sample size.
In this study, we constructed a model with ES events in HCC, which can effectively evaluate the prognosis. We conducted an in-depth analysis of the IRF3 ES events, providing broader insights into the progress and treatment of HCC. Nevertheless, there are some deficiencies in this application as well. For instance, the samples we obtained were the cDNA microarray from liver cancer patients, but not tissue. Therefore, we are unable to verify the protein expression level of IRF3 splicing isoforms. Besides, we explore the gene expression difference based on the transcriptome data of TCGA, and speculate the effect of IRF3 isoforms on hepatocarcinoma, without further exploration through experiments. Therefore, it is necessary to verify in clinical samples or cell lines. Additionally, calculating the PSI value in clinical practice may be challenging and the reasons for the generation of IRF3 splicing isoforms are also worth further research.
Ethical approval
The cDNA microarray experiments were conducted according to the guidelines of the Declaration of Helsinki, and approved by the Medical Ethics Committee of the Shanghai Outdo Biotech Company (ID: YBM-05-02).
Author contributions
Z.L. wrote the manuscript. Z.L. performed the bioinformatics analysis. W.G. analysed the results of cDNA microarray, Z.L. and Z.D. conducted the statistical analysis and data interpretation. W.G., T.L. and Y.Z. performed the data review. D.X. and C.H. participated in the study conception and supervision, data analysis, and manuscript editing. All authors read and approved the final manuscript.
Supplemental Material
Download ()Disclosure statement
No potential conflict of interest was reported by the author(s).
Data availability statement
The original contributions presented in the study are included in the article/Supplementary Material. Further inquiries can be directed to the corresponding authors.
Supplementary material
Supplemental data for this article can be accessed online at https://doi.org/10.1080/15592294.2023.2276371
Additional information
Funding
Notes
1. Coefficient.
2. Hazard Ratio.
3. Confidence interval.
4. The exon number is obtained based on the TCGA SpliceSeq database.
References
- Chidambaranathan-Reghupaty S, Fisher PB, Sarkar D. Hepatocellular carcinoma (HCC): Epidemiology, etiology and molecular classification. Adv Cancer Res. 2021;149:1–15. doi: 10.1016/bs.acr.2020.10.001
- Chalasani NP, Ramasubramanian TS, Bhattacharya A, et al. A novel blood-based panel of methylated DNA and protein markers for detection of early-stage hepatocellular carcinoma. Clin Gastroenterol Hepatol. 2021;19(12):2597–605 e4. doi: 10.1016/j.cgh.2020.08.065
- Luo P, Wu S, Yu Y, et al. Current status and perspective biomarkers in AFP negative HCC: towards Screening for and diagnosing hepatocellular carcinoma at an earlier stage. Pathol Oncol Res. 2020;26(2):599–603. doi: 10.1007/s12253-019-00585-5
- Wang ET, Sandberg R, Luo S, et al. Alternative isoform regulation in human tissue transcriptomes. Nature. 2008;456(7221):470–476. doi: 10.1038/nature07509
- Bernard A, Hibos C, Richard C, et al. The tumor microenvironment impairs Th1 IFNgamma secretion through alternative splicing modifications of Irf1 pre-mRNA. Cancer Immunol Res. 2021;9(3):324–336. doi: 10.1158/2326-6066.CIR-19-0679
- Kashyap A, Tripathi G, Tripathi A, et al. RNA splicing: a dual-edged sword for hepatocellular carcinoma. Med Oncol. 2022;39(11):173. doi: 10.1007/s12032-022-01726-8
- Tremblay MP, Armero VE, Allaire A, et al. Global profiling of alternative RNA splicing events provides insights into molecular differences between various types of hepatocellular carcinoma. BMC Genomics. 2016;17(1):683. doi: 10.1186/s12864-016-3029-z
- Yuan JH, Liu XN, Wang TT, et al. The MBNL3 splicing factor promotes hepatocellular carcinoma by increasing PXN expression through the alternative splicing of lncRNA-PXN-AS1. Nat Cell Biol. 2017;19(7):820–832. doi: 10.1038/ncb3538
- Martinez-Montiel N, Rosas-Murrieta NH, Anaya Ruiz M, et al. Alternative splicing as a target for cancer treatment. Int J Mol Sci. 2018;19(2). doi: 10.3390/ijms19020545
- Yu S, Cai L, Liu C, et al. Identification of prognostic alternative splicing events related to the immune microenvironment of hepatocellular carcinoma. Mol Med. 2021;27(1):36. doi: 10.1186/s10020-021-00294-3
- Wang S, Wang S, Zhang X, et al. Comprehensive analysis of prognosis-related alternative splicing events in ovarian cancer. RNA Biol. 2022;19(1):1007–1018. doi: 10.1080/15476286.2022.2113148
- Oltean S, Bates DO. Hallmarks of alternative splicing in cancer. Oncogene. 2014;33(46):5311–5318. doi: 10.1038/onc.2013.533
- Llovet JM, Kelley RK, Villanueva A, et al. Hepatocellular carcinoma. Nat Rev Dis Primers. 2021;7(1):6. doi: 10.1038/s41572-020-00240-3
- D’Souza S, Lau KC, Coffin CS, et al. Molecular mechanisms of viral hepatitis induced hepatocellular carcinoma. World J Gastroenterol. 2020;26(38):5759–5783. doi: 10.3748/wjg.v26.i38.5759
- Silva AL, Faria M, Matos P. Inflammatory microenvironment modulation of alternative splicing in cancer: a way to adapt. Adv Exp Med Biol. 2020;1219:243–258. doi: 10.1007/978-3-030-34025-4_13
- Zhang Q, Liu W, Zhang HM, et al. hTftarget: a Comprehensive database for regulations of human transcription factors and their targets. Int J Genomics Proteomics. 2020;18(2):120–128. doi: 10.1016/j.gpb.2019.09.006
- Dhanwani R, Takahashi M, Sharma S. Cytosolic sensing of immuno-stimulatory DNA, the enemy within. Curr Opin Immunol. 2018;50:82–87. doi: 10.1016/j.coi.2017.11.004
- Shen Y, Peng X, Shen C. Identification and validation of immune-related lncRNA prognostic signature for breast cancer. Genomics. 2020;112(3):2640–2646. doi: 10.1016/j.ygeno.2020.02.015
- Li Y, Qi D, Zhu B, et al. Analysis of m6A RNA methylation-related genes in liver hepatocellular carcinoma and their correlation with survival. Int J Mol Sci. 2021;22(3). doi: 10.3390/ijms22031474
- Lee SE, Alcedo KP, Kim HJ, et al. Alternative Splicing in Hepatocellular Carcinoma. Cell Mol Gastroenterol Hepatol. 2020;10(4):699–712. doi: 10.1016/j.jcmgh.2020.04.018
- Huang S, Luo K, Jiang L, et al. PCBP1 regulates the transcription and alternative splicing of metastasis‑related genes and pathways in hepatocellular carcinoma. Sci Rep. 2021;11(1):23356. doi: 10.1038/s41598-021-02642-z
- Yu L, Kim J, Jiang L, et al. MTR4 drives liver tumorigenesis by promoting cancer metabolic switch through alternative splicing. Nat Commun. 2020;11(1):708. doi: 10.1038/s41467-020-14437-3
- Zhang F, Wang H, Yu J, et al. LncRNA CRNDE attenuates chemoresistance in gastric cancer via SRSF6-regulated alternative splicing of PICALM. Mol Cancer. 2021;20(1):6. doi: 10.1186/s12943-020-01299-y
- Leier A, Moore M, Liu H, et al. Targeted exon skipping of NF1 exon 17 as a therapeutic for neurofibromatosis type I. Mol Ther Nucleic Acids. 2022;28:261–278. doi: 10.1016/j.omtn.2022.03.011
- Nabi AA, Atta SA, El-Ahwany E, et al. Taurine upregulates miRNA-122-5p expression and suppresses the metabolizing enzymes of glycolytic pathway in hepatocellular carcinoma. Mol Biol Rep. 2021;48(7):5549–5559. doi: 10.1007/s11033-021-06571-y
- Afifi AM, El-Husseiny AM, Tabashy RH, et al. Sorafenib- Taurine Combination Model for Hepatocellular Carcinoma Cells: Immunological Aspects. Asian Pac J Cancer Prev. 2019;20(10):3007–3013. doi: 10.31557/APJCP.2019.20.10.3007
- Resh MD. Palmitoylation of proteins in cancer. Biochem Soc Trans. 2017;45(2):409–416. doi: 10.1042/BST20160233
- Ala M. The footprint of kynurenine pathway in every cancer: a new target for chemotherapy. Eur J Pharmacol. 2021;896:173921. doi: 10.1016/j.ejphar.2021.173921
- Liu S, Cai X, Wu J, et al. Phosphorylation of innate immune adaptor proteins MAVS, STING, and TRIF induces IRF3 activation. Science. 2015;347(6227):aaa2630. doi: 10.1126/science.aaa2630
- Zhao B, Shu C, Gao X, et al. Structural basis for concerted recruitment and activation of IRF-3 by innate immune adaptor proteins. Proc Natl Acad Sci U S A. 2016;113(24):E3403–12. doi: 10.1073/pnas.1603269113
- Petro TM. IFN regulatory factor 3 in health and disease. J Immunol. 2020;205(8):1981–1989. doi: 10.4049/jimmunol.2000462
- Andrilenas KK, Ramlall V, Kurland J, et al. DNA-binding landscape of IRF3, IRF5 and IRF7 dimers: implications for dimer-specific gene regulation. Nucleic Acids Res. 2018;46(5):2509–2520. doi: 10.1093/nar/gky002
- Shi HX, Yang K, Liu X, et al. Positive regulation of interferon regulatory factor 3 activation by Herc5 via ISG15 modification. Mol Cell Biol. 2010;30(10):2424–2436. doi: 10.1128/MCB.01466-09
- Karpova AY, Ronco LV, Howley PM. Functional characterization of interferon regulatory factor 3a (IRF-3a), an alternative splice isoform of IRF-3. Mol Cell Biol. 2001;21(13):4169–4176. doi: 10.1128/MCB.21.13.4169-4176.2001
- Li Y, Hu X, Song Y, et al. Identification of novel alternative splicing variants of interferon regulatory factor 3. Biochim Biophys Acta. 2011;1809(3):166–175. doi: 10.1016/j.bbagrm.2011.01.006
- Jiaze Y, Sinan H, Minjie Y, et al. Rcl1 suppresses tumor progression of hepatocellular carcinoma: a comprehensive analysis of bioinformatics and in vitro experiments. Cancer Cell Int. 2022;22(1):114. doi: 10.1186/s12935-022-02533-x
- Shin H, Cha HJ, Lee MJ, et al. Identification of ALDH6A1 as a Potential Molecular Signature in Hepatocellular Carcinoma via Quantitative Profiling of the Mitochondrial Proteome. J Proteome Res. 2020;19(4):1684–1695. doi: 10.1021/acs.jproteome.9b00846
- Zhang T, Ma Z, Liu L, et al. DDX39 promotes hepatocellular carcinoma growth and metastasis through activating Wnt/β-catenin pathway. Cell Death Dis. 2018;9(6):675. doi: 10.1038/s41419-018-0591-0
- Ouyang X, Lv L, Zhao Y, et al. ASF1B Serves as a Potential Therapeutic Target by Influencing Cell Cycle and Proliferation in Hepatocellular Carcinoma. Front Oncol. 2021;11:801506. doi: 10.3389/fonc.2021.801506
- Xing C, Xie H, Zhou L, et al. Cyclin-dependent kinase inhibitor 3 is overexpressed in hepatocellular carcinoma and promotes tumor cell proliferation. Biochem Biophys Res Commun. 2012;420(1):29–35. doi: 10.1016/j.bbrc.2012.02.107
- Li Y, Zhu Z, Zhang S, et al. ShRNA-targeted centromere protein a inhibits hepatocellular carcinoma growth. PLoS One. 2011;6(3):e17794. doi: 10.1371/journal.pone.0017794
- Gandhi M, Gross M, Holler JM, et al. The lncRNA lincNMR regulates nucleotide metabolism via a YBX1 - RRM2 axis in cancer. Nat Commun. 2020;11(1):3214. doi: 10.1038/s41467-020-17007-9
- Yue H, Wu K, Liu K, et al. LINC02154 promotes the proliferation and metastasis of hepatocellular carcinoma by enhancing SPC24 promoter activity and activating the PI3K-AKT signaling pathway. Cell Oncol. 2022;45(3):447–462. doi: 10.1007/s13402-022-00676-7
- Huang DH, Jian J, Li S, et al. TPX2 silencing exerts anti‑tumor effects on hepatocellular carcinoma by regulating the PI3K/AKT signaling pathway. Int J Mol Med. 2019;44(6):2113–2122. doi: 10.3892/ijmm.2019.4371
- Chen Z, Xu L, Su T, et al. Autocrine STIP1 signaling promotes tumor growth and is associated with disease outcome in hepatocellular carcinoma. Biochem Biophys Res Commun. 2017;493(1):365–372. doi: 10.1016/j.bbrc.2017.09.016
- Mondal P, Sen S, Klein BJ, et al. TCF19 promotes cell proliferation through binding to the histone H3K4me3 mark. Biochemistry. 2020;59(4):389–399. doi: 10.1021/acs.biochem.9b00771
- Mondal P, Gadad SS, Adhikari S, et al. TCF19 and p53 regulate transcription of TIGAR and SCO2 in HCC for mitochondrial energy metabolism and stress adaptation. FASEB J. 2021;35(9):e21814. doi: 10.1096/fj.202002486RR
- Dong Y, Sun X, Zhang K, et al. Type IIA topoisomerase (TOP2A) triggers epithelial-mesenchymal transition and facilitates HCC progression by regulating snail expression. Bioengineered. 2021;12(2):12967–12979. doi: 10.1080/21655979.2021.2012069
- Honda K, Takaoka A, Taniguchi T. Type I interferon [corrected] gene induction by the interferon regulatory factor family of transcription factors. Immunity. 2006;25(3):349–360. doi: 10.1016/j.immuni.2006.08.009
- Ashley CL, Abendroth A, McSharry BP, et al. Interferon-independent innate responses to cytomegalovirus. Front Immunol. 2019;10:2751. doi: 10.3389/fimmu.2019.02751