ABSTRACT
Bacopa monnieri known as ‘Brahmi’ is a well-known medicinal plant belonging to Scrophulariaceae family for its nootropic properties. To the best of our knowledge, no characterization data is available on the potential role of micro RNAs (miRNAs) from this plant till date. We present here the first report of computational characterizations of miRNAs from B. monnieri. Owing to the high conservation of miRNAs in nature, new and potential miRNAs can be identified in plants using in silico techniques. Using the plant miRNA sequences present in the miRBase repository, a total of 12 miRNAs were identified from B. monnieri which pertained to 11 miRNA families from the shoot and root transcriptome data. Furthermore, gene ontology analysis of the identified 68 human target genes exhibited significance in various biological processes. These human target genes were associated with signaling pathways like NF-kB and MAPK with TRAF2, CBX1, IL1B, ITGA4 and ITGB1BP1 as the top five hub nodes. This cross-kingdom study provides initial insights about the potential of miRNA-mediated cross-kingdom regulation and unravels the essential target genes of human with implications in numerous human diseases including cancer.
1. Introduction
Plant miRNAs are significant elements that regulate genes post-transcriptionally by targeting mRNAs translation suppression or cleavage. MiRNAs are single-stranded, small non-coding RNAs belonging to endogenous class mostly located within non-coding genomic regions and generally transmitted from the promoters of RNA polymerase II.Citation1,Citation2 Mature miRNA formation is a complex process of enzymecatalysation from the actual primary (pri-miRNA) transcript to the precursor (pre-miRNA) with a distinctive hairpin structure, leading to a miRNA duplex (miRNA: miRNA*).Citation3 A miRNA duplex is constructed at the RNA-induced silencing complex (RISC) in the previous stage to guide its action on a target mRNA.Citation4 This complex’s activity relies upon the base-pairing stage between miRNA and reactive elements leading to the target mRNA’s translational suppression.Citation5 The ideal pairing leads to cleavage, while incorrect pairing results in translational plant suppression. miRNA constitutes about 1–2% of the genome and plays a significant role as therapeutic agents and regulates multiple metabolic procedures at distinct rates. The key plant miRNAs were recognized in mid-2002 from the ideal plant Arabidopsis thaliana. Subsequently, many plant miRNAs have been submitted in the miRBase sequence database.Citation6 Countless, in-silico approaches have been applied to discover miRNA provided that the organism’s entire genome is available.Citation7 The lack of complete genome sequences formed a major roadblock for miRNA identification.Citation8 EST (Expressed Sequence Tag) analysis is an effective tool for identifying conserved miRNAs among distinct plant species whose entire genome sequences are unavailable. The approach is also helpful in studying the conservation and development of miRNAs between various organisms.Citation9 EST analytic techniques have been consistently proven fruitful in discovering new miRNAs from different species of plants.Citation10,Citation11 Therefore, the insilco methods are effective in predicting novel miRNAs.
Among the plants considered for medicinal significance, the plant Bacopa monnieri (Brahmi) belonging to the Scrophulariaceae family is very important for its therapeutic potentials and pharmacologically relevant phytochemicals.Citation12 B.monnieri has traditionally been utilized as a neurological substance and cognitive enhancer. A lot of efforts are now devoted to study its neuroprotective properties traced in traditional medicines.Citation13 This plant is well known for its improvement of brain function along with memory enhancement, antioxidant, antitoxic, hepatoprotactive, anti-hypertensive, anti-diarrheal, analgesic, antiulcer, antibacterial, antifungal, anticancer, antioxidant, anti-inflammatory, antidepressant and antiepileptic activities.Citation14,Citation15
Since first discovered Caenorhabditis elegans miRNA, a significant number of microRNAs have been discovered but no miRNAs have been reported in the miRBase repository for the B. monnieri species. Therefore, in the present study, a B. monnieri transcriptome from the Sequence Read Archive database, assembled by paired-end short reads of the plant shoot and root was used for computational prediction of miRNAs and their human targets.Citation16 In addition, the identified human targets have been characterized and functional annotation was performed to explore the pathways, which are most influenced by the predicted miRNAs. Eventually, the targets which participated in encoding transcription factors were analyzed using the co-expression network approach.Citation17
2. Methodology
2.1 Retrieval of data
The available transcriptome data of B. monnieri shoot and root (generated by an Illumina NextSeq 500) was retrieved from NCBI SRA (Sequence Read Archive) repositoryCitation18 having the accession number SRR2826837. The whole transcriptome data was downloaded in SRA (raw) format for further analysis.
2.2 Reference set of miRNAs
A total of 35,828 mature and 28,645 precursor FASTA sequences of microRNAs were retrieved from the miRBase v 21 repository.Citation19 An aggregate of 8,496 known plant mature miRNAs were collected from the miRNA Registry database.Citation20 One hundred percent identical recurrence of these miRNA sequences was excluded using in-house Perl script. As a result, 4,617 non-redundant unique Viridiplantae miRNAs were obtained.
2.3 Denovo assembly from available RNAseq data using in-silico approach
The raw format of whole transcriptome data was transformed into Fastq format using SRA Toolkit v 2.4. The trimming tool, Trimmomatic v 0.39 was used to remove low quality reads (QV<20) and adapter contamination from the raw data.Citation21 The filtered data was then de novo assembled using the Trinity v 2.1.1 program. CD-HIT-EST v 4.5.4 was utilized to cluster the assembled transcripts on default parameters to remove transcripts redundancy which resulted in unigenes.Citation22
2.4 Identification of potential miRNAs of B. monnieri
The sequences of plant miRNAs were searched against the Unigene sequences to identify the homologous region. The blastn program of NCBI BLAST+ package was used to perform the alignment process.Citation23 The E-value and mismatch parameters were set at 1000 and 2, respectively. The Unigenes having appropriate alignment with plant miRNAs were filtered out and their 100 base flanking regions along with aligned portion were selected as probable precursor miRNA candidate.Citation24
2.5 Precursor microRNA secondary structure prediction
The precursor miRNA candidate sequences were given as an input to the mfold web server for secondary structure prediction using RNA binding method with default parameters.Citation25 The output consisted of ID, complete sequence length, nucleotide composition, matching region coordinates, (GC) and (A + U/T) content in percentage and minimum free folding energy (MFE, ΔG in kcal/mol). The MFEI (Minimal Folding free Energy Index) and AMFE (Adjusted Minimal Folding Free Energy) were calculated as per the equations AMFE = (MFE/(length of a potential pre-miRNA)) *100 and MFEI = ((100*MFE)/Length of RNA/(G + C)) %.Citation26
To consider the sequence as miRNA, below mentioned criteria were used:
The sequence of precursor miRNA must fold into an appropriate stem-loop hairpin topology
The mature miRNA sequence must reside within either arm of the structure
0.53 (kcal/mol) > MFEI > 1.01 (kcal/mol) and MFE < −18 (kcal/mol)
The percentage of (A + U) content in the range of 30–70%
No break or loop in miRNA sequence
Bulge size ranges between 3 and 7 nucleotides in miRNA
Maximum 6 mismatches are allowed between miRNA and miRNA*
2.6 Prediction and functional annotation of potential miRNA target
The assembled Unigenes of B. monnieri were used as the reference for identifying targets of the candidate miRNAs by opting the criterion “Function user submitted small RNAs user submitted transcripts” in the webserver psRNATargetCitation10,Citation27 The putative targets of miRNAs were predicted against Homo sapiens transcripts with default parameter including, maximum expected threshold: 3, Length of complementarity scoring (hsp Size): 20, maximum energy to unpair target site (UPE): 25, and translation inhibition was set between 9 and 11 nucleotides.Citation16 The blastx analysis was performed against the non-redundant (NR) database (January 2015) followed by gene ontology analysis using PANTHER to distinguish biological and molecular functions.Citation28
2.7 Identification of hub proteins
The target genes were analyzed using the bottleneck, stress and betweenness algorithm using the cytohubba plugin of Cytoscape v 3.7.Citation29
2.8 Phylogenetic analysis
Known mature plant miRNA sequences from miRBase and predicted B. monnieri miRNA sequences were combined and processed for multiple sequence alignment using the ClustalOmega tool. The phylogenetic analysis was then performed using the MEGA7 phylogenetic package. Further, neighbor-joining tree was constructed to study the evolutionary relationships of miRNAs within the same family.Citation30
3. Results
3.1 MiRNA identification and characterization
The prediction of new potential miRNAs of B. monnieri was performed using a combination of various computational methods. The workflow is shown in . The 70,825,073 raw reads of B. monnieri transcriptome was acquired from SRA belonging to plant shoot and root samples. The program Trinity was used to assemble the data by de novo method and 273,687 transcripts were acquired with N50 of 1309 bp. By using the standalone BLAST program, a total of 4,803 distinct Viridiplantae miRNA sequences were aligned with assembled Unigene sequencesCitation31 which were further processed using CD-HIT-EST to remove redundancy and resulted in 1,878 unigene sequences. The validation process of predicted miRNA sequences was performed using the web server mfold to verify the requirements listed in the previous section (Materials & Methods). A total of 12 miRNA candidates from 11 miRNA families were screened out. The length of the predicted miRNA precursor sequence ranges from 75 to 156 nucleotides. The predicted mature miRNAs varied from 18 to 23 nucleotides (). It was observed that the structures with low MFE values are stable thermodynamically.Citation32 The MFE values for the mfold predicted structures were in the range of −19.7 to −56.30 -kcal/mol. For each sequence, the MFE index (MFEI) was calculated to avoid the prioritization of other RNAs as miRNA candidates. The MFE index (MFEI) was found to be in the range of −1.90 to −5.63 kcal/mol for each sequence. Candidate miRNAs tend to be present in the stem region of the hairpin structure consisting of A + U (53.90%) and G + C (46.09%) contents during the screening of potential miRNAs ().Citation7
Table 1. Details of the predicted miRNA from ESTs of B.monnieri.
3.2 Nomenclature of predicted miRNAs
The nomenclature of predicted miRNAs was adopted in accordance with miRBase conventions. The mature sequences are designated as ‘MIR’ and the precursor hairpins are labeled as ‘miR’ with the prefix ‘Bmn’ for B. monnieri as Bmo is already assigned to Bombyx mori in miRBase.Citation33
3.3 Target prediction
To identify the human targets that are probably regulated by plant-derived miRNAs, the identified miRNA sequences were analyzed against the human transcripts using psRNAtarget web-server.Citation24 In this cross-kingdom study, we identified 68 human target genes for 11 miRNA families. Out of these 68 targeted genes, the predicted miRNAs exhibited cleavage and translation inhibition for 62 and 6 genes (), respectively. Considering the significance of the above results, to analyze these genes in detail became imperative. Circos plot was created to visualize the interaction between the miRNAs and their target genes. ()
Figure 3. Circos plot depicting regulation of different 68 human genes with a segment and each connection with a ribbon targeted by twelve B.monnieri miRNAs. The width of categories depends on the number of genes targeted by miRNAs. A connection between a miRNA and a gene implies that this miRNA and its predicted target are regulated by B.monnieri.
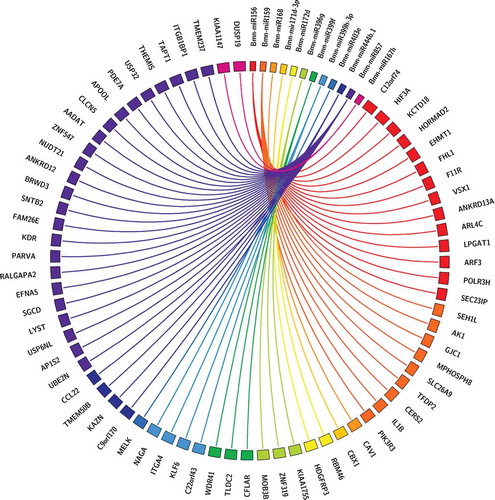
3.4 Functional annotation and pathway analysis
Functional annotation of the identified targeted genes is an important step to understand the respective functions. Gene ontology (GO) analysis was conducted to determine which functions were largely represented for the genes regulated by predicted miRNAs. Top GO terms are mainly categorized into Biological Process (BP), Molecular Function (MF) and Cellular Component (CC) (). In the molecular function category, the miRNA-regulated genes were highly involved in binding (GO:0005488), molecular function regulator (GO:0098772) and catalytic activity (GO:0003824). In the biological process category, cellular process (GO:0009987), localization (GO:0051179) and metabolic process (GO:0008152) were highly represented groups.Citation17 In the cellular component cluster, the genes were highly enriched in the cell junction (GO:0030054), cell (GO:0005623), and membrane (GO:0016020) cohorts. () Moreover, the KEGG (Kyoto Encyclopedia of Genes and Genomes) database was used for pathway analysis. Most of the genes were involved in Integrin and VEGF (vascular endothelial growth factor) signaling pathways, angiogenesis, PI3K Phosphatidylinositol 3-kinase pathway, p53 pathway and MAPK (mitogen-activated protein kinase) signaling pathway.
Table 2. Prediction of miRNA target genes of B.monnieri
Table 3. Gene ontology analysis of B.monnieri.
3.5 Protein–protein interaction and hub node identification
It became essential to evaluate the extent of node interconnection and identify hubs (nodes with the highest numbers of interactions) in order to recognize the key regulators of the cell signaling pathway. By degree method, the top 10 hub nodes were identified using centrality parameters such as Bottleneck, Stress, and betweenness in order to understand the nature, significance and their underlying biological processes.Citation11 Having score 16 and highest number of interactions, the topmost Hub node was TRAF2 (TNF Receptor Associated Factor 2). The other significant proteins such as CBX1 (Chromobox 1), IL1B (Interleukin 1 beta), ITGA4 (Integrin, Alpha 4), ITGB1BP1 (Integrin beta-1-binding protein 1), NAGA (Alpha-N-Acetylgalactosaminidase), ASGR2 (Asialoglycoprotein Receptor 2), UBE2N (Ubiquitin Conjugating Enzyme E2 N), MAP3K5 (Mitogen-Activated Protein Kinase Kinase Kinase 5) and KLF6 (Kruppel-like factor 6) were identified as the other top nodes with 15, 14, 8, 7, 7, 6, 6 and 6 scores, respectively (). The top nodes showed complex network interconnection which may pinpoint toward the major role of TRAF2 in the downstream signaling pathway by regulating the other interacting proteins in this network.
Figure 5. Top hub nodes identification detected by the bottleneck method using the cytohubba plugin of cystoscape suite. Hub nodes in the combined network and GO enrichment analysis of their targets. (a) Hub nodes in the combined network. Hub nodes are indicated with a color scheme from highly essential (red) to essential (yellow).
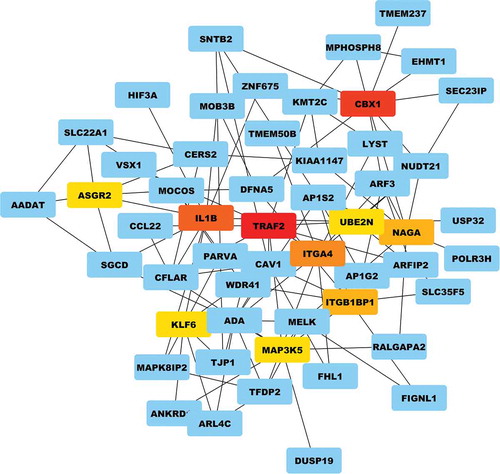
3.6 Phylogenetic analysis
Phylogenetic analysis was carried out to understand the relationship between the identified miRNA in B. monnieri with the other plant species’miRNAs available in miRBase database for family identification.Citation34 No miRNAs were listed for B. monnieri in the miRBase database. Phylogenetic tree method was used for the identification of different families and a total of 11 miRNA families were identified ().Citation35
4. Discussion
B. monnieri is one of the significant medicinal plants used widely for multiple phycological disorders and industrial uses.Citation36 Several phytochemical tests have been performed to detect essential bioactive compounds with medicinal properties to heal illness and to investigate the pharmacological activities of this medicinal plant.Citation37,Citation38 However, the basic mechanism by which therapeutic plants affects the human genome remains to be undisclosed.Citation39 MicroRNAs have appeared as a significant regulatory component expressed by most eukaryotic genomes including plants. Evolutionary conservation of these miRNAs has helped the prediction of new miRNA homologs by using insilico methods.Citation24 We used insilico approach in this study for the prediction of miRNAs from B. monnieri root and shoot transcriptome data and to identify their targets in Homo sapiens. By using the information of known plant miRNAs present in miRBase, we found 12 novel miRNAs which falls under 11 miRNA families.
The precursor miRNA structures were checked for their ability to adopt hairpin loop and other energetic parameters such as MFE, MFEI, AMFE, and stability. It is well established that lesser the value of MFE, the more stable is the miRNA secondary structure.Citation40 It was also found that the AU content (53.90%) was greater than GC content (46.10%) of predicted miRNAs. Precursor miRNA had high negative scores of MFE and MFEI values. The average value for MFE and MFEI were 33.79 kcal/mol and 3.57 kcal/mol, respectively. ()Citation24 Identifying miRNA targets to evaluate miRNA’s biological function in plants is also an important area of intensive research. Cross-kingdom complementarity and target location accessibility were the two primarily focused variables in this study which were applied in the psRNATarget tool to identify the potential human targets.Citation41 In this study, potential miRNA targets of B. monnieri were predicted. Certain miRNAs were also associated to constitute multiple targets. Most of the predicted miRNA targets were coding genes for transcription factors.
Furthermore, miRNAs bind at complementary sites of their target genes either partially or completely. Our results indicate that 12 miRNA sequences predominantly identified in B. monnieri transcriptome including bmn-miR857, bmn-miR156, bmn-miR159, bmn-miR444b.1, bmn-miR399h-3p, bmn-miR396 g, bmn-miR172d, bmn-miR399f, bmn-miR167h, bmn-miR171d-3p, bmn-miR168 and bmn-miR403e. Noteworthy, these miRNAs possess the ability to regulate 68 vital human target genes of enormous biological and functional importance. Out of 68 target genes, 46 targets have an immense potential role in human diseases as well as in human metabolic processes. It has been discovered that Bmn-miR857 and Bmn-miR156 have the largest 24 and 14 potential human transcriptome objectives, respectively. The human target genes regulated by B. monnieri miRNAs displayed their crucial role in catalytic activity (molecular function) binding activity and metabolic process (biological process). Potential target genes regulated by cross-kingdom analysis such as TRAF2, CAV1, KLF6, CFLAR, PIK3R3, IL1B, HIF3A, ITGA4 have been found to play a major role in various diseases such as prostate cancer, breast cancer, lung disease, prostate cancer, colorectal cancer, Alzheimer’s disease, epilepsy, multiple sclerosis and mental retardation (). These hub nodes are cell signaling pathway inhibitors and therefore the TRAF2- the top most hub node might be a possible master regulator accountable for modulating distinct downstream signaling pathways.
Table 4. List of miRNA, type of inhibition and its human disease associations B.monnieri.
These miRNAs identified from B. monnieri may have a significant role to play in targeting the prognostic marker such as TRAF2 which has been identified as the top node regulated by the cross-kingdom analysis.Citation42 As TRAF2 is the top most hub node, it clearly demonstrates that the target genes of B. monnieri corresponding to selected miRNAs regulate NF-kB and JNK (c-Jun N-terminal kinase) activity and play a key role in controlling cell growth and apoptosis in osteo-tropical breast and prostate cancer.Citation43 In addition, IL1B is also a crucial gene and plays an important role in regulation of the NF-kB signaling and MAPK signaling.Citation38 B. monnieri miRNAs could have a potential role in regulating the negative feedback loop of these two pathways by modulating IL1B activity.Citation44 In addition, most of the target genes affected by these miRNAs are largely reported in different kinds of cancer and multiple neuronal diseases, indicating that these miRNAs would have possible anti-neoplastic characteristics with clinical significance.
Finally, the results showed the potential role for B. monnieri species. miRNA derived from B. monnieri and its targeted genes are known to be involved in multiple biological processes, having important disease connections and modulate essential downstream signaling pathways in different organs and functional processes.Citation45 Thus, cross-kingdom regulation provides initial and strong clues to the ongoing research of how medicinal plants affect human transcriptome. The identification of these novel processes could significantly improve our knowledge of molecular communication between species.Citation41
5. Conclusion
B. monnieri0 shoot and root transcriptome data was used for miRNA identification and their targets were identified using cross-kingdom approach. Twelve potential miRNAs were predicted from the transcriptome data. Total 68 targets were predicted, and 11 miRNAs were conserved in miRNA families. Bmn-miR857 and Bmn-miR156 were found to have the highest 24 and 14 potential targets on human transcriptome, respectively. Network analysis showed that the regulation of 68 human genes was based on the interaction with 10 hub nodes. These target genes were engaged in multiple downstream signaling pathways in numerous functional processes and present in different species. Conclusively, these findings may further enhance our understanding of the plant miRNAs regulating the human transcriptome. It also prioritizes new miRNA candidates which may have therapeutic potentials for prevention as well as treatment of various human diseases.
Author contributions
Pandya H, Rawal R and Patel SK conceived and designed the experiments; Gadhavi H, Patel M and Mangukia N performed the experiments; Gadhavi H and Patel M analyzed and interpreted the data; Gadhavi H, Patel M, Shah K, Bhadresa K, and Pandya H drafted the article, revised it critically for important intellectual content and final approval of the paper.
Disclosure of potential conflicts of interest
No potential conflicts of interest were disclosed.
Additional information
Funding
References
- Andreassi MG. Non-coding RNA in cardiovascular disease: a general overview on microRNAs, long non-coding RNAs and circular RNAs. Non-coding RNA Invest. 2018;2:1. doi:10.21037/ncri.
- Jiang M, Sang X, Hong Z. Beyond nutrients: food‐derived microRNAs provide cross‐kingdom regulation. Bioessays. 2012;34(4):280–16. doi:10.1002/bies.v34.4.
- LAI EC, WIEL C, RUBIN GM. Complementary miRNA pairs suggest a regulatory role for miRNA: miRNA duplexes. Rna. 2004;10(2):171–175. doi:10.1261/rna.5191904.
- MacFarlane LA, R Murphy P. MicroRNA: biogenesis, function and role in cancer. Curr Genomics. 2010;11(7):537–561. doi:10.2174/138920210793175895.
- Frazier TP, Sun G, Burklew CE, Zhang B. Salt and drought stresses induce the aberrant expression of microRNA genes in tobacco. Mol Biotechnol. 2011;49(2):159–165. doi:10.1007/s12033-011-9387-5.
- Bennett C. Combination biomarkers for precision oncology: drug development goes beyond the one biomarker, one drug model. Genet Eng Biotechnol News. 2019;39(5):44–45. doi:10.1089/gen.39.05.13.
- Yin Z, Li C, Han X, Shen F. Identification of conserved microRNAs and their target genes in tomato (Lycopersicon esculentum). Gene. 2008;414(1–2):60–66. doi:10.1016/j.gene.2008.02.007.
- Vaucheret H, Chupeau Y. Ingested plant miRNAs regulate gene expression in animals. Cell Res. 2012;22(1):3–5. doi:10.1038/cr.2011.164.
- Sahu S, Khushwaha A, Dixit R. Computational identification of miRNAs in medicinal plant senecio vulgaris (Groundsel). Bioinformation. 2011;7(8):375–378. doi:10.6026/bioinformation.
- Gupta H, Tiwari T, Patel M, Mehta A, Ghosh A. An approach to identify the novel miRNA encoded from H. Annuus EST sequences. Genomics Data. 2015;6:139–144. doi:10.1016/j.gdata.2015.09.005.
- Kumar D, Kumar S, Ayachit G, Bhairappanavar S, Ansari A, Sharma P, Soni S, Das J. Cross-kingdom regulation of putative miRNAs derived from happy tree in cancer pathway: a systems biology approach. Int J Mol Sci. 2017;18(6):1191. doi:10.3390/ijms18061191.
- Kean JD, Downey LA, Stough C. A systematic review of the ayurvedic medicinal herb Bacopa monnieri in child and adolescent populations. Complement Ther Med. 2016;29(29):56–62. doi:10.1016/j.ctim.2016.09.002.
- Simpson T, Pase M, Stough C. Bacopa monnieri as an antioxidant therapy to reduce oxidative stress in the aging brain. Evid Based Complement Alternat Med. 2015;615384.
- Basak A, Hossain M, Parvin M. Evaluation of phytochemical and pharmacological activities of Bacopa monnieri (L.). Int J Sci Rep. 2016;2(10):242. doi:10.18203/issn.2454-2156.IntJSciRep20163394.
- Chaudhari KS, Tiwari NR, Tiwari RR, Sharma RS. Neurocognitive effect of nootropic drug Brahmi (Bacopa monnieri) in Alzheimer’s disease. Ann Neurosci. 2017;24(2):111–122. doi:10.1159/000475900.
- Patel M, Mangukia N, Jha N, Gadhavi H, Shah K, Patel S, Mankad A, Pandya H, Rawal R. Computational identification of miRNA and their cross kingdom targets from expressed sequence tags of Ocimum basilicum. Mol Biol Rep. 2019;46(3):2979–2995. doi:10.1007/s11033-019-04759-x.
- Sobhani Najafabadi A, Naghavi M. Mining ferula gummosa transcriptome to identify miRNAs involved in the regulation and biosynthesis of terpenes. Gene. 2018;645:41–47. doi:10.1016/j.gene.2017.12.035.
- Leinonen R, Sugawara H, Shumway M, International Nucleotide Sequence Database Collaboration. The sequence read archive. Nucleic Acids Res. 39;2010:D19–D21.
- Zhang J, Xue B, Gai M, Song S, Jia N, Sun H. 2017. Small RNA and transcriptome sequencing reveal a potential miRNA-mediated interaction network that functions during somatic embryogenesis in lilium pumilum DC. Fisch. Front Plant Sci. 8:566.
- Kozomara A, Griffiths-Jones S. miRBase: integrating microRNA annotation and deep-sequencing data. Nucleic Acids Res. 2011;39:152–157. doi:10.1093/nar/gkq1027.
- Mehta A, Gupta H, Rawal R, Mankad A, Tiwari T, Patel M, Ghosh A. In silico microRNA identification from stevia rebaudiana transcriptome assembly. Eur J Med Plants. 2016;15(2):1–14. doi:10.9734/EJMP.
- Dash P, Rai R, Mahato A, Gaikwad K, Singh N. transcriptome landscape at different developmental stages of a drought tolerant cultivar of flax (Linum usitatissimum). Front Chem. 2017;5(5). doi:10.3389/fchem.2017.00082.
- Altschul SF, Gish W, Miller W, Myers EW, Lipman DJ. Basic local alignment search tool. J Mol Biol. 1990;215(3):403–410. doi:10.1016/S0022-2836(05)80360-2.
- Zhang BH, Pan XP, Cox SB, Cobb GP, Anderson TA. Evidence that miRNAs are different from other RNAs. Cell Mol Life Sci. 2006;63(2):246–254. doi:10.1007/s00018-005-5467-7.
- Zuker M. Mfold web server for nucleic acid folding and hybridization prediction. Nucleic Acids Res. 2003;31(13):3406–3415. doi:10.1093/nar/gkg595.
- Trotta E. On the normalization of the minimum free energy of RNAs by sequence length. PLoS One. 2014;9(11):e113380. doi:10.1371/journal.pone.0113380.
- Dai X, Zhao PX. psRNATarget: a plant small RNA target analysis server. Nucleic Acids Res. 2011;39(Web Server issue):W155–9. doi:10.1093/nar/gkr319.
- Thomas PD, Kejariwal A, Campbell MJ, Mi H, Diemer K, Guo N, Ladunga I, Ulitsky-Lazareva B, Muruganujan A, Rabkin S, et al. PANTHER: a browsable database of gene products organized by biological function, using curated protein family and subfamily classification. Nucleic Acids Res. 2003;31(1):334–341. doi:10.1093/nar/gkg115.
- Chin CH, Chen SH, Wu HH, Ho CW, Ko MT, Lin CY. cytoHubba: identifying hubobjects and sub-networks from complex interactome. BMC Syst Biol. 2014;8:S11. doi:10.1186/1752-0509-8-S4-S11.
- Tamura K, Stecher G, Peterson D, Filipski A, Kumar S. MEGA7: molecular evolutionary genetics analysis version 6.0. Mol Biol Evol. 2013;30(12):2725–2729. doi:10.1093/molbev/mst197.
- Patnaik B, Park S, Kang S, Hwang H, Wang T, Park E, Chung JM, Song DK, Kim C, Kim S, et al. Transcriptome profile of the Asian giant hornet (Vespa mandarinia) using illumina HiSeq 4000 sequencing: deNovoAssembly, functional annotation, and discovery of SSR markers. Int J Genomics. 2016;2016(1):1–15. doi:10.1155/2016/4169587.
- Hamasaki-Katagiri N, Lin B, Simon J, Hunt R, Schiller T, Russek-Cohen E, Komar AA, Bar H, Kimchi‐Sarfaty C The importance of mRNA structure in determining the pathogenicity of synonymous and non-synonymous mutations in haemophilia. Haemophilia. 2016;23(1):e8–e17. doi:10.1111/hae.13107.
- Griffiths-Jones S, Grocock RJ, Van Dongen S, Bateman A, Enright AJ. miRBase: microRNA sequences, targets and gene nomenclature. Nucleic Acids Res. 2006;34:140–144. doi:10.1093/nar/gkj112.
- Mirzaei K, Bahramnejad B, Shamsifard M, Zamani W. In silico identification, phylogenetic and bioinformatic analysis of argonaute genes in plants. Int J Genomics. 2014;2014(1–17):1–17. doi:10.1155/2014/967461.
- Jeyaraj A, Zhang X, Hou Y, Shangguan M, Gajjeraman P, Li Y, Wei C. Genome-wide identification of conserved and novel microRNAs in one bud and two tender leaves of tea plant (Camellia sinensis) by small RNA sequencing, microarray-based hybridization and genome survey scaffold sequences. BMC Plant Biol. 2017;17(1). doi:10.1186/s12870-017-1169-1.
- Saini N, Singh D, Sandhir R. Bacopa monnieri prevents colchicine-induced dementia by anti-inflammatory action. Metab Brain Dis. 2019;34(1–14):505–518. doi:10.1007/s11011-018-0332-1.
- Zhang B, Pan X, Cobb GP, Anderson TA. Plant microRNA: a small regulatory molecule with big impact. Dev Biol. 2006;289(289):3–16. doi:10.1016/j.ydbio.2005.10.036.
- Wang KS, Li J, Wang Z, Mi C, Ma J, Piao LX, Xu GH, Li X, Jin X. Artemisinin inhibits inflammatory response via regulating NF-κB and MAPK signaling pathways. Immunopharmacol Immunotoxicol. 2017;39(1):28–36. doi:10.1080/08923973.2016.1267744.
- Singh N, Sharma A. Turmeric (Curcuma longa): miRNAs and their regulating targets are involved in development and secondary metabolite pathways. CR Biol. 2017;340(11–12):481–491. doi:10.1016/j.crvi.2017.09.009.
- Zhu Q, Luo Y. Identification of miRNAs and their targets in tea (Camellia sinensis). J Zhejiang Univ Sci B. 2013;14:916–923. doi:10.1631/jzus.B1300006.
- Patel M, Patel S, Mangukia N, Patel S, Mankad A, Pandya H, Rawal R. Ocimum basilicum miRNOME revisited: a cross kingdom approach. Genomics. 2019;111:772–785.
- Wei B, Liang J, Hu J, Mi Y, Ruan J, Zhang J, Wang Z, Hu Q, Jiang H, Ding Q. TRAF2 is a valuable prognostic biomarker in patients with prostate cancer. Med Sci Monit. 2017;31(23):4192–4204. doi:10.12659/MSM.903500.
- Wei B, Ruan J, Mi Y, Hu J, Zhang J, Wang Z, Hu Q, Jiang H, Ding Q. Knockdown of TNF receptor-associated factor 2 (TRAF2) modulates in vitro growth of TRAIL-treated prostate cancer cells. Biomed Pharmacother. 2017;93:462–469. doi:10.1016/j.biopha.2017.05.145.
- Tulotta C, Lefley DV, Freeman K, Gregory WM, Hanby AM, Heath PR, … Bradbury SM. Endogenous production of IL1B by breast cancer cells drives metastasis and colonization of the bone microenvironment. Clin Cancer Res. 2019;25(9):2769–2782. doi:10.1158/1078-0432.CCR-18-2202.
- Rastogi S, Meena S, Bhattacharya A, Ghosh S, Shukla RK, Sangwan NS, Lal RK, Gupta MM, Lavania UC, Gupta V, et al. De novo sequencing and comparative analysis of holy and sweet basil transcriptomes. BMC Genomics. 2014;15:588–605. doi:10.1186/1471-2164-15-588.