Abstract
Although it is widely accepted that the detention performance of green roofs is of interest to stormwater engineers and planners, no single metric allows detention to be unambiguously defined. Detention effects are highly sensitive to rainfall characteristics and antecedent conditions, and individual roofs typically exhibit wide variations in detention performance between storm events. This paper uses a straightforward hydrological model to explore two alternative approaches to describing detention performance: a probabilistic approach based on long time-series simulations; and a design storm approach. It is argued that the non-linear reservoir routing parameters (scale, k and exponent, n) provide fundamental descriptors of the detention process, with modelling enabling performance to be determined for specific rainfall inputs. The study utilises 30-year rainfall time-series predictions for four contrasting UK locations to demonstrate the utility of the two proposed design approaches and to comment on locational variations in detention performance.
1. Introduction
Many researchers have analysed green roof rainfall and runoff data to derive quantitative descriptions of the systems’ hydrological performance. The derived measures typically include retention parameters (cumulative volumetric retention or mean/median/minimum/maximum per-event retention) and detention parameters (percentage Peak Attenuation and indicators of lag time such as Time to Start of Runoff, Peak Delay, Centroid Delay and t50Delay).
Although it is widely accepted that the detention performance of green roofs is of interest to stormwater engineers and planners, there is no single agreed metric in common usage that allows detention performance to be unambiguously defined and understood. This may be contrasted with the characterisation of retention performance, which is simply determined as the proportion of rainfall that is retained, either on an annual or per event basis. Figure highlights seven alternative detention metrics, all of which have been applied within green roof research.
Definitions of terms can sometimes be open to interpretation and/or affected by external factors. For example, for the same storm event one would expect Peak Attenuation to be greater if the peak 1-minute intensities are used rather than the peak 1-hour intensities. Therefore the term Peak Attenuation should strictly always be qualified by time-step, although it rarely is.
Indicators of average performance (such as cumulative volumetric retention, mean per-event retention, mean t50Delay) may be useful in some contexts, and can certainly facilitate comparisons between different systems and/or the same system exposed to different climatic inputs. However, they have quite limited value for stormwater management purposes where system design and management is reliant on a more direct understanding of what performance may be expected in response to specific rainfall inputs. The events used for drainage design purposes depend upon local attitudes to risk and the consequences of failure, but are often in the order of 1 in 10, 1 in 30 or 1 in 100 year return period events. In the same way that a sewerage designer would not design a storm sewer to cope with only routine (or sub-annual return period) rainfall events, the indicators used to describe green roof performance should reflect the need to understand performance in non-routine, or design storm, events. Some authors have therefore chosen to focus on a sub-set of the monitored data, arguing that drainage engineers are likely to only be interested in ‘significant’ events. For example, Stovin et al. (Citation2012) considered all events with a return period of greater than one year to be significant, whilst Voyde et al. (Citation2010) focused on events with >2 mm rainfall. Getter et al. (Citation2007) and Carpenter and Kaluvakolanu (Citation2011) both classified their monitored events into small, medium and large rainfall events although different depth thresholds were used in the two studies. Fassman-Beck et al. (Citation2013) used exceedance probability plots to highlight the frequency statistics of both runoff depth and peak runoff rate, and also classified runoff depths according to rainfall depth categories to highlight the strong dependency of runoff depths on rainfall characteristics. Locatelli et al. (Citation2014) classified their results according to both return period and rainfall intensity.
If the rainfall event generates no runoff, then the temporal detention parameters become incalculable/infinite, whilst Peak Attenuation = 1.0 and Peak Runoff = 0.0. The t50Delay parameter can only be determined for the sub-set of events in which the runoff exceeds 50% of the rainfall depth. Any mean detention values that are expected to be indicative of overall performance will clearly be very sensitive to the sub-set of rainfall events that is included in the calculations.
Detention metrics derived from monitoring studies also include the effects of retention (or initial losses). In Figure , approximately 15% of the rainfall event is retained. If a greater/smaller proportion of the event were retained, then all of the metrics presented above would assume different values. Similarly, if the event depth and losses were the same, but the temporal profile of the rainfall were different (e.g. more or less intense), different metrics would again be expected. These interacting effects mean that the detention characteristics reported from monitoring studies typically exhibit extremely large standard deviations, with the reported mean values not being representative of the majority of actual events. For example, Stovin et al. (Citation2012) reported – for a sub-set of ‘significant’ rainfall events – Centroid Delay values ranging from 4.50 to 261.32 minutes and Peak Attenuation values ranging from 19.81 to 99.93%. The reported mean values are likely to be strongly biased towards the relatively small sub-set of events considered, and cannot be considered useful or valid for estimating the likely performance in an unseen, arbitrary, rainfall event or rainfall time-series. Using the median value for design (or modelling) purposes would lead to a system that failed to meet its design objectives (or a model that underestimated runoff, by potentially significant amounts) 50% of the time. These uncertainties and biases inevitably lead to low levels of confidence in published data relating to green roof detention performance.
The lack of consistency in published performance data, particularly concerning detention performance, almost certainly reflects the general lack of specific performance objectives for green roofs. Traditionally in an urban drainage design context, design storms are used to assess performance of options against stipulated performance requirements. The Forschungsgesellschaft Landschaftsentwicklung Landschaftsbau (FLL) guidance (FLL, Citation2008) outlines a standard test to determine the coefficient of discharge, C, based on the ratio of cumulative runoff to cumulative rainfall at the end of a 15-minute constant intensity rainfall of 27 mm. The test is undertaken in a 5 m laboratory rainfall simulator, with the substrate pre-wetted to ensure that it is at field capacity. The resultant value of C can be used to determine worst-case drainage requirements for the roof, and to compare the relative performance of different green roof systems. The FLL coefficient of discharge could potentially be used to define a minimum performance standard for green roof detention. However, the design storm depth and duration were selected specifically to reflect German stormwater management requirements, and are therefore not universally applicable.
A more generic approach requires the processes affecting detention to be modelled, such that the response to an arbitrary rainfall input (e.g. a design storm) can be predicted, Several different approaches to modelling green roof detention processes have been presented in the literature, including finite element (Hilten et al., Citation2008; Palla et al., Citation2012) and unit hydrograph-based (Villarreal & Bengtsson, Citation2005) approaches. However, many authors have shown that simple reservoir routing approaches are suitable for modelling green roof detention processes (Jarrett & Berghage, Citation2008; Kasmin et al., Citation2010). Villareal and Bengtsson (Citation2005) and Jarrett and Berghage (Citation2008) both demonstrated model applications to locally-relevant design storms.
Design storms are appropriate to ensure that drainage systems meet minimum performance thresholds in extreme conditions, particularly concerning flood protection. However, they provide no insight into the contribution that alternative drainage options may make to the day-to-day stormwater management within a catchment; this is particularly pertinent when assessing water quality impacts. Information on hydrological performance during routine events is critical to determine whether a system achieves its water quality objectives, and continuous simulation methods are increasingly utilised within urban stormwater management planning. Jarrett and Berghage (Citation2008) and Stovin et al. (Citation2013) have demonstrated that proper representation of evapotranspiration processes is critical to the development of robust continuous simulation models for green roof retention. Locatelli et al. (Citation2014) presented a similar retention model, but also implemented a detention model based on non-linear reservoir routing, with a 10-minute time-step. She and Pang (Citation2010) presented a physically-based green roof model that comprised three sub-modules to describe evapotranspiration, infiltration and flow routing (detention). The model was shown to be capable of reproducing continuous monitored runoff data from a full scale green roof in Portland, Oregon.
The integration of green roof modelling capabilities into mainstream urban drainage simulation tools is critical for understanding their potential contribution within the total catchment. Li and Babcock (Citation2014) provide a useful review of recent attempts to adapt existing continuous simulation modelling tools such as SWMM to incorporate green roofs. Alternatively, Zhang and Guo (Citation2013) developed an analytical model to evaluate green roof long-term hydrological performance characteristics. Local precipitation characteristics were described probabilistically, and the hydrological processes were described by mass balance equations.
Whereas Stovin et al. (Citation2013) employed a 1 year return period threshold to separate out significant events from the long term record, Locatelli et al. (Citation2014) used probability distributions to explore how roof configuration affected runoff volume, peak time delay and runoff rate as a function of the rainfall event return period.
In many environmental contexts, where complex and interacting factors lead to high degrees of variability in performance, it is common to express performance in terms of acceptable probabilities of failure. For example, the UK's UPM (Urban Pollution Management) Manual (FWR, Citation2012) describes how percentile based river quality standards for BOD, dissolved oxygen, total ammonia and un-ionised ammonia may be utilised to regulate impacts associated with intermittent discharges from combined sewer overflows. Long term monitoring programmes and continuous simulation studies enable green roof performance to be described in probabilistic terms, and one of the objectives of this paper is to further demonstrate the value of probabilistic performance metrics in providing a complete description of the hydrological benefits (and limitations) of green roof installations.
Although the detention performance metrics cited above are all strongly influenced by both the rainfall characteristics and antecedent conditions (retention processes), the fundamental hydrological detention processes occurring within the green roof are essentially independent of these factors and dependent only on the roof's physical configuration (its slope, substrate type and depth, drainage layer composition etc.). It may be argued, therefore, that the critical parameters for describing green roof detention are those that describe detention processes rather than observable detention effects. If the underlying detention processes can be described by an hydrological model, then the model parameters provide a robust and complete description of the system's detention performance. It also follows that the model may be employed to objectively predict the detention effects associated with a specific design storm or rainfall time-series. If the same form of conceptual detention model can be fitted to several different green roof runoff time-series, then the differences in identified parameter values will provide an objective indication of differences in detention performance, even if the original monitoring programmes were not comparable.
The overall aim of this paper is to demonstrate how a validated green roof runoff detention model may be employed to generate objective and complete data to characterise the roof's hydrological performance, using both long time-series continuous rainfall inputs and a design storm approach. Simulated runoff responses corresponding to four UK locations with contrasting climatic regimes will be analysed in detail, leading to discussion and recommendations on those performance indicators that might be considered most useful for stormwater management purposes.
2. Methodology
2.1 Input data
Stovin et al. (Citation2013) presented a detailed comparison of long-term green roof retention performance, using four contrasting UK climatic regimes to demonstrate the importance of local climate in determining runoff retention performance for a typical extensive green roof system. It was demonstrated that, for example, the total volumetric retention over a 30-year period varied from 0.19 (cool, wet climate) to 0.59 (warm, dry climate). The study employed a conceptual hydrological flux model operating at an hourly time-step. Although an hourly time-step is appropriate for retention studies, it does not permit the (meaningful) modelling and interpretation of the roof's detention (i.e. lag and attenuation) performance. The present study utilises the same rainfall input data, temporally disaggregated to 5-minute time-steps, to comment on the influence of local climatic controls on green roof runoff detention performance.
The four locations (Figure ) were chosen to represent four contrasting UK climatic conditions. As detailed in Stovin et al. (Citation2013), climatic inputs for the model were taken from the UK Climate Projections (UKCP09, http://ukclimateprojections.defra.gov.uk/).
The rainfall was disaggregated from hourly to 5-minute time-steps using STORMPAC (WRc, Citation2009).
2.2 Retention model
The retention model estimates the soil moisture content based on a balance between the moisture gains due to rainfall and losses due to evapotranspiration (ET). ET is estimated using the Thornthwaite formula to estimate Potential ET and applying a linear SMEF (Soil Moisture Extraction Function) to account for the influence of soil moisture content on actual ET rates. The roof has an assumed maximum retention capacity of 20 mm. The model is fully explained and validated in Stovin et al. (Citation2013).
2.3 Detention model
Kasmin et al. (Citation2010) suggested that the detention performance of a green roof test bed could be modelled using reservoir routing concepts:
These initial estimates of k and n represent the combined detention effects due to the roof's vegetation, substrate and drainage layer. Subsequent investigations have provided a more detailed breakdown of the specific detention effects due to the substrate and drainage layer (see Yio et al. (Citation2013) and Vesuviano and Stovin (Citation2013)), and a two-stage reservoir routing model has been proposed and validated (Vesuviano et al., Citation2014). Key findings from these studies are that: the detention in green roof substrates increases as a function of depth and organic matter content (which reduces permeability) (Yio et al., Citation2013); detention due to the drainage layer is typically negligible, unless the system includes a fibrous mat (Vesuviano & Stovin, Citation2013); and the reservoir routing parameters are independent of rainfall intensity (both studies). The Yio et al. (Citation2013) study suggested that the value of k would reduce (i.e. the detention effect increases) by an order of magnitude if the depth of the substrate layer were significantly increased (e.g. doubled) and/or the organic content was increased (reduced permeability). k also reduces in the presence of a fibrous mat below the drainage layer. These studies have shown that the predicted runoff profiles are relatively insensitive to the value of the exponent n, and – indeed – that co-dependency between k and n may compromise attempts at independent parameter identification. Yio et al. (Citation2013) demonstrated that a model based on a fixed value of n was capable of predicting observed runoff profiles with almost no loss of accuracy when compared with a model for which both parameters had been optimised.
In the present study we apply a single non-linear reservoir model to contrast the detention effects associated with a typical extensive green roof system (k = 0.03, n = 2.0) with the performance of a system with increased levels of detention (assumed k = 0.003, n = 2.0). The smaller value of k is indicative of the increased levels of detention that might be expected if the substrate depth were increased from 80 mm to 150 mm (Yio et al., Citation2013). A substrate depth of 150 mm would typically be described as a semi-intensive green roof. Note that a deeper, semi-intensive, system would also be expected to demonstrate some retention benefits, due to the higher moisture retention capacity and higher levels of actual ET (reduced influence of the SMEF), even if the vegetation was essentially the same as on the extensive roof. However, these benefits would be relatively minor as the main control on retention is Potential ET, which is independent of the substrate depth or composition. It should also be noted that a smaller value of k could be achieved without any alteration to the substrate depth, for example through the inclusion of increased levels of organic matter or other soil amendments, or through the inclusion of a moisture retention mat beneath the drainage layer. Therefore, as not all incidences of increased detention would necessarily be associated with increased substrate depth and increased retention, the detention comparison (k = 0.03 versus k = 0.003) is undertaken using identical (extensive system) retention assumptions. The typical extensive green roof detention coefficients are employed at all four of the locations, whereas the detention performance of a high-detention system is only evaluated for the Sheffield location, such that five configurations are assessed for detention. For brevity, the higher detention configuration is referred to as ‘Shef-HighDet’ throughout the paper. The ‘combined’ system value of n is fixed at 2.0, as originally proposed and validated by Kasmin et al. (Citation2010). The models have been implemented in MATLAB (Citation2007).
2.4 Data analysis
Individual storm events were isolated from the continuous simulation record based on an assumed six-hour inter-event period, as reported by Stovin et al. (Citation2013). Retention was determined for each event, as well as overall volumetric retention. As highlighted above, given that per event retention may vary from 0 to 100%, it may be argued that a mean value is of limited use. Therefore, Probability Density Functions (PDFs) of both absolute and proportional retention are presented. The retention data are also classified by rainfall depth for one example location, Sheffield.
In Figure , seven different detention performance indicators were highlighted. Three of these will be considered in detail here: Peak Runoff; Peak Attenuation and Centroid Delay. Centroid Delay is perceived to be a more robust indicator of the delay in bulk runoff than either Peak Delay or t50Delay. Peak Delay was not selected as the irregular nature of natural rainfall events means that the peak rainfall is often not a good indicator of the storm centre; indeed it may occur after the peak in runoff (Stovin et al., Citation2012). t50Delay can only be determined for a subset of the runoff-generating events. The Time to Start of Runoff is influenced more by retention than detention, and the Runoff Duration is influenced more by rainfall duration than by detention.
It should be noted that the assumption of a 6-hour inter-event period resulted in some very minor discrepancies in runoff mass-balance for the Shef-HighDet system, due to low levels of runoff occurring outside of this period. The Peak Runoff and Peak Attenuation data are unaffected by this, and no Centroid Delay times are affected by more than 1%.
The data was analysed to identify any significant trends in the detention parameters as a function of rainfall depth, and then in terms of PDFs to assess the effects of location and of increased detention at the Sheffield location only. This analysis included a probabilistic evaluation of Peak Runoff compared with potential greenfield runoff rates. Finally, mean values of the three parameters were considered with respect to rainfall depth.
2.5 Detention design charts
The long time-series continuous simulation provides a robust statistical characterisation of system performance unique to a specific location, encapsulating both extreme and day-to-day rainfall-runoff responses. However, it is likely that many regulatory bodies will continue to set performance targets in terms of design storms, so it may also be useful to consider how different roof configurations might be expected to respond to the same design storm.
Detention performance design charts have been produced by running the detention simulation for a range of different storm depths (1 to 50 mm) with a range of different initial losses (or retention capacities) (0–20 mm). The design storm profile was a symmetrical summer 50% profile peakedness 1-hour event (Flood Studies Report (NERC, Citation1975)). This was chosen to represent a relatively short-duration high intensity event of the type likely to be associated with urban flash flooding, although it should be noted that alternative design events may be more appropriate in other jurisdictions, and may easily be modelled in exactly the same way. Detention has been modelled for the two roof configurations assumed previously (i.e. ‘extensive’, k = 0.03; and ‘high detention’, k = 0.003). Two of the performance metrics were selected: Peak Attenuation and Centroid Delay.
3. Results
3.1 Long-term performance
3.1.1 Retention performance
Figure presents the PDFs for the retention performance metrics for each of the four locations. Note that the same retention data applies to both of the two alternative detention configurations for Sheffield. Across the four locations, the absolute retention depth distributions are similar, with the two drier, eastern, locations – Sheffield and East Midlands – experiencing marginally lower retention depths overall when compared with the two western locations. It is particularly interesting to note that, although the roof has an assumed maximum retention capacity of 20 mm, this depth is extremely rarely retained in practice. This is because, for the vast majority of rainfall events, the event depth is less than 20 mm or the available retention capacity prior to the onset of rainfall is less than 20 mm. Only 10% of events experience retention in excess of 5 mm. In terms of percentage retention, there are more obvious locational differences, with the drier East Midlands and the wetter NW Scotland locations experiencing respectively far greater and far lower per event retention compared with Cornwall and Sheffield. Only 20% of events in NW Scotland are fully retained, compared with 50% full retention in the East Midlands.
The basic retention metrics are also presented in Table . It may be seen that the number of events, mean retention and per-event data are almost identical to the values presented by Stovin et al. (Citation2013) for the same rainfall time-series. Any differences are due to the fact that the rainfall data has been disaggregated down from one-hour to 5-minute time-steps in the present analysis. It may be seen that volumetric retention varies from 0.19 (NW Scotland) to 0.59 (East Midlands).
Table 1 Retention performance characteristics.
In all cases the broad spread of retention proportions (between 0.0 and 1.0) suggests that the use of a mean retention value is not appropriate. However, systematic variations in the retention proportion are to be expected in response to variations in rainfall depth. For rainfall events larger than 20 mm (maximum retention capacity), complete retention is physically impossible and proportionately smaller maximum and mean retention values should be expected. Conversely, as the rainfall depths drop below 20 mm, the likelihood of the rainfall depth being lower than the available retention capacity increases, and higher mean retention values should be expected. The box-whisker plots presented in Figure (showing sample data for Sheffield) confirm that this is the case.
3.1.2 Detention performance
Figure shows how the key detention metrics – Peak Runoff, Peak Attenuation and Centroid Delay – vary with respect to rainfall depth for the Sheffield extensive roof configuration data set. Comparable variations with respect to rainfall depth were observed at all four locations and for the Shef-HighDet configuration. The data presented encompasses all events for which the computed runoff was non-zero (strictly > 0.2 mm). The actual numbers of events considered are detailed in Table . Only 31% of the events generated runoff in the East Midlands, compared with 63% of events in NW Scotland. Deeper rainfall events tend to lead to higher runoff depths and runoff rates, so the observed positive and negative relationships between rainfall depth and Peak Runoff and Peak Attenuation respectively are expected. The Centroid Delay behaviour is less intuitive, with the time delay increasing with rainfall depth up to 20–40 mm, but flattening out for higher rainfall depths. The initial positive correlation probably reflects the tendency for larger storms to have longer durations. Long duration storms (particularly ones in which the initial portion of rainfall is retained) will lead to long Centroid Delay times. However, some of the very largest storms may have been more intense convectional rainfall occurring over shorter durations and with limited retention. Under these circumstances long Centroid Delay times are less likely than with the partially-retained lower-intensity events.
Additional analysis (not shown) revealed similar dependencies on rainfall intensity for Peak Runoff and Peak Attenuation, together with a weak negative relationship between Centroid Delay and rainfall intensity across all five of the modelled configurations.
Figure presents the PDFs for Peak Runoff, Peak Attenuation and Centroid Delay for the four locations and also for the Shef-HighDet configuration. For all detention performance metrics there are very wide ranges of performance, but also observable differences between location/configuration. The PDFs permit a probabilistic approach to the assessment of performance against design standards.
Two versions of the Peak Runoff PDFs are included. The plot on the left uses a log x-axis to show the complete range of Peak Runoff values, whilst the plot on the right zooms in, using a truncated y-axis and a linear x-axis, to allow comparison of the statistical performance data against a potential regulatory target value, the equivalent greenfield runoff rate. Sample greenfield runoff rates of 5 and 2 l/s/ha have been included, with 2 l/s/ha appearing in the UK SuDS Standards (DEFRA, Citation2011). It is evident that the highest Peak Runoff rates are associated with the locations that experience the greatest total rainfall (NW Scotland and Cornwall). In NW Scotland more than 50% of runoff-generating events have a peak 5 minute runoff rate of nearly 0.5 mm per 5 minutes. This is equivalent to 16 l/s/ha (1 mm/5 mins = 33 l/s/ha). In contrast, the same roof located in the East Midlands would achieve the 5 l/s/ha target for almost 50% of events. In NW Scotland and Cornwall almost 90% of runoff-generating events would exceed the 2 l/s/ha threshold, whereas in Sheffield and the East Midlands this falls to less than 80%. An alternative view of the same data is that we can be 90% confident of achieving peak runoff rates of less than 20 l/s/ha for the East Midlands, but only less than 50 l/s/ha in NW Scotland.
The Shef-HighDet system demonstrates a very significant improvement in the Peak Runoff performance, with Peak Runoff rates generally being halved compared with the baseline extensive system's performance at the same location. The 90 percentile confidence limit would be improved from 30 l/s/ha down to 13 l/s/ha through the implementation of system that offered this level of increased detention.
The performance data in Figure relates only to runoff-generating events. However, it should be appreciated that the events that do not generate runoff (i.e. are fully retained) also form part of the overall system performance characteristics. For example, whilst Figure suggests that the East Midlands roof limits Peak Runoff to below 5 l/s/ha for approximately 50% of the runoff-generating events, this equates to 83% of the total number of rainfall events. It is difficult to definitively state which of these versions of the PDF data is likely to be most useful for stormwater management purposes.
Acknowledging the wide range of detention performance metrics, Figures and suggest that these variations are strongly influenced by rainfall depth. Figure therefore presents a comparison of the mean values of the three detention metrics across all five configurations, classified according to rainfall depth. The basic trends that were highlighted in the Sheffield data are apparent at all four locations. Variations between locations for both Peak Runoff and Peak Attenuation are negligible; far greater differences are evident as a function of the rainfall event depth. The differences in the PDFs arise as a result of each location being exposed to different numbers of events in each rainfall category.
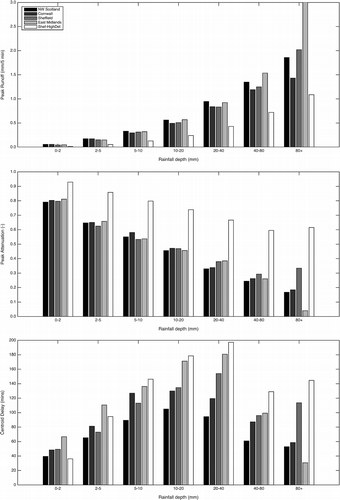
The influence of roof configuration (Sheffield versus Shef-HighDet) is striking, with the Peak Runoff halving when the higher detention system is employed and with commensurate increases in the resultant Peak Attenuation. This is to be expected, given that detention processes are configuration-specific.
The Centroid Delay parameter shows greater variation between the locations, with the rainfall patterns in the East Midlands resulting in detention effects that are quite close to those observed when the higher detention system is exposed to the Sheffield rainfall profile.
3.1.3 Review of findings
The analysis presented above has highlighted a number of issues associated with any attempt to quantify green roof detention performance from long-term records. In this case the 30-year continuous simulations ensure a statistical validity that is often difficult to achieve with real field monitoring programmes. Nonetheless, several fundamental questions remain, including which metrics to use, whether or not it is useful to consider performance probabilistically and whether or not subsets of events should be considered.
Probabilistic representations of the data have the advantage of clearly showing the range of performance that is to be expected; this can be missed when only global mean or median values are presented. It is suggested that the use of probabilistically-defined thresholds may provide a useful basis for setting performance targets. For example, it would be feasible to specify a maximum proportion (or a minimum return period) of events that may be allowed to exceed a threshold such as the greenfield runoff rate.
To overcome the bias introduced by large numbers of small events that may not be relevant for flow control planning purposes, the data may be classified in terms of rainfall event depth or based on return period thresholds. An extension of this idea is to consider a design storm.
3.2 Detention design charts
Figure shows the detention design charts for the two sample roof configurations. In each sub-plot, the lower right triangle corresponds to combinations of rainfall depth and available retention that do not result in any runoff. For these combinations the attenuation and delay are effectively infinite/unquantifiable.
As the storm depth increases, both Peak Attenuation and Centroid Delay decrease. Any retention capacity effectively reduces the storm depth and therefore increases the delay. For the largest rainfalls, with no retention available, attenuation drops to 10% for the extensive system, but always exceeds 45% for the high-detention system. Similarly, the worst-case Centroid Delay is 27 mins for the high-detention system, but 8 mins for the extensive system. For a 30 mm storm, with 10 mm available retention capacity, expected values for Peak Attenuation and Centroid Delay would be 0.34 and 16.95 mins respectively for the extensive system compared with 0.77 and 48.8 mins respectively for the high-detention system (Figure ). This equates to enhancements of 125% to Peak Attenuation and 188% to the Centroid Delay.
4. Discussion
The analysis of long-term rainfall-runoff predictions presented in this paper has highlighted the difficulty of identifying detention metrics that will unambiguously describe the performance of green roofs (and other SuDS devices). Various parameters to characterise delay have been outlined, including the t50Delay and the Centroid Delay. However, the natural variability of rainfall events and antecedent conditions leads to a wide spread in the derived values for any specific roof system.
For comparison with design requirements, it is more useful to consider the absolute values of peak runoff than the mean proportion retained. It is suggested that future design standards could be expressed in terms of 90% compliance against the greenfield runoff rate for the peak runoff rate. Here the peak runoff rate has been determined based on a 5-minute time interval; lower peak values (i.e. higher rates of compliance) would have been obtained if the data were amalgamated to peak 1-hour rates. It is therefore critical to ensure that the time-step is stipulated as part of any performance requirements. Locatelli et al. (Citation2014), for example, identified a 10-minute rainfall intensity as being most relevant.
The categorisation of performance metrics based on rainfall depth has highlighted the criticality of this parameter in determining system performance. Even within each rainfall depth category, wide variations in performance are observed. This reflects the random nature of natural storm events, and the influence of antecedent conditions on retention, which impacts markedly upon the observed detention performance. The same roof system can exhibit very different detention characteristics between different storm events, even though the system's physical travel time (as determined by substrate type and depth, drainage board and roof slope and length) does not change from one event to the next. Alternatively, the use of design charts can provide an objective assessment of a given system's detention performance across a range of rainfall depth and retention capacity scenarios.
Stovin et al. (Citation2013) showed that considerable differences in retention performance should be expected as a result of the UK's climatic gradients. These retention differences have a minor impact on the spread of detention metrics, with locations that experience higher levels of retention also demonstrating improved detention.
The introduction of a high detention roof configuration resulted in significant improvements in overall detention performance. Various green roof manufacturers are currently exploring opportunities to provide enhanced detention. Deeper (more intensive) substrates will provide greater detention, but potentially at the cost of unacceptably increased roof weight. Fibrous mats and alternative drainage layer components may deliver this function at reduced weight, whilst restricted orifices at the roof outlet can provide a low-cost option.
Finally, it has been argued that the reservoir routing model parameters k and n provide an objective and unambiguous indication of the roof's inherent detention properties. When combined with a suitable retention model, a detention modelling approach enables both a long-term statistical evaluation and a design storm-based evaluation of detention performance.
5. Conclusions
The following conclusions confirm key findings already reported in the literature:
Both retention and detention are strongly correlated with rainfall depth.
The characterisation of a green roof system with average values of retention or detention metrics fails to recognise the significant spread in event-by-event performance observed in field monitoring programmes.
This research has also demonstrated that:
A specific green roof system will exhibit different apparent detention characteristics when exposed to different climatic inputs because detention metrics also incorporate the effects of retention.
The non-linear reservoir routing model parameters k (scale) and n (exponent) provide an objective and unambiguous indication of the roof's inherent detention properties. When combined with a suitable retention model, a detention modelling approach enables both a long-term statistical evaluation and a design storm-based evaluation of detention performance.
The use of PDFs derived from long-term continuous simulation can facilitate the use of probabilistic performance metrics, such as an assessment of the 90 percentile Peak Runoff in comparison with a regulatory greenfield runoff rate.
Acknowledgements
The authors would like to express their very sincere thanks to Jamie Margetts and Michael Rowlatt of Clear Environmental Consultants Ltd for their assistance with the rainfall disaggregation. Some of the ideas developed within the paper have stemmed from research undertaken as part of the EU-funded ‘Green Roof Systems’ collaboration (Ref. 230636) between ZinCo and the University of Sheffield. We would particularly like to thank Ralf Walker of ZinCo for valuable technical input. Figure is reprinted from Stovin et al. (Citation2013) with permission from Elsevier.
© Crown Copyright 2009. The UK Climate Projections (UKCP09) have been made available by the Department for Environment, Food and Rural Affairs (Defra) and the Department of Energy and Climate Change (DECC) under licence from the Met Office, UKCIP, British Atmospheric Data Centre, Newcastle University, University of East Anglia, Environment Agency, Tyndall Centre and Proudman Oceanographic Laboratory. These organisations give no warranties, express or implied, as to the accuracy of the UKCP09 and do not accept any liability for loss or damage, which may arise from reliance upon the UKCP09 and any use of the UKCP09 is undertaken entirely at the users risk.
Disclosure statement
No potential conflict of interest was reported by the author(s).
Additional information
Funding
Notes
1. Present address: Centre for Ecology and Hydrology, Maclean Building, Benson Lane, Crowmarsh Gifford, Wallingford, OX10 8BB, UK.
References
- Carpenter, D. and Kaluvakolanu, P., 2011. Effect of roof surface type on storm-water runoff from full-scale roofs in a temperate climate. Journal of Irrigation and Drainage Engineering, 137, 161–169.
- DEFRA, 2011. National standards for sustainable drainage systems. London: DEFRA.
- Fassman-Beck, E., Voyde, E., Simcock, R., and Hong, Y.S., 2013. 4 living roofs in 3 locations: does configuration affect runoff mitigation? Journal of Hydrology, 490, 11–20.
- FLL (Forschungsgesellschaft Landschaftsentwicklung Landschaftsbau), 2008. Guidelines for the Planning, Construction and Maintenance of Green Roofing. Bonn, Germany: Forschungsgesellschaft Landschaftsentwicklung Landschaftsbau e.V.
- FWR (Foundation for Water Research), 2012. Urban Pollution Management Manual. 3rd ed. Marlow, UK: FWR, Available online: http://www.fwr.org/UPM3/.
- Getter, K.L., Rowe, D.B., and Andresen, J.A., 2007. Quantifying the effect of slope on extensive green roof stormwater retention. Ecological Engineering, 31, 225–231.
- Hilten, R.N., Lawrence, T.M., and Tollner, E.W., 2008. Modeling stormwater runoff from green roofs with HYDRUS-1D. Journal of Hydrology, 358 (3–4), 288–293.
- Jarrett, A.R. and Berghage, R.D., 2008. Annual and individual green roofs stormwater response models, Paper presented at the 6th Greening Rooftops for Sustainable Communities Conference, Baltimore, MD.
- Kasmin, H., Stovin, V.R., and Hathway, E.A., 2010. Towards a generic rainfall-runoff model for green roofs. Water Science & Technology, 62 (4), 898–905.
- Li, Y. and Babcock, R.W., 2014. Green roof hydrologic performance and modelling: a review. Water Science & Technology, 69 (4), 727–738.
- Locatelli, L., Mark, O., Mikkelsen, P.S., Arnberg-Nielsen, K., Jensen, M.B., and Binning, P.J., 2014. Modelling of green roof hydrological performance for urban drainage applications. Journal of Hydrology, 519, 3237–3248. doi:10.1016/j.jhydrol.2014.10.030.
- MATLAB, 2007. MATLAB version R2007b. Natick, MA: The MathWorks Inc.
- NERC (Natural Environment Research Council), 1975. Flood Studies Report. London: NERC.
- Palla, A., Gnecco, I., and Lanza, L.G., 2012. Compared performance of a conceptual and a mechanistic hydrologic models of a green roof. Hydrological Processes, 26 (1), 73–84, 10.1002/hyp.8112.
- She, N. and Pang, J., 2010. Physically based green roof model. Journal of Hydrological Engineering, 15, 458–464.
- Stovin, V., Vesuviano, G., and Kasmin, H., 2012. The hydrological performance of a green roof test bed under UK climatic conditions. Journal of Hydrology, 414–415, 148–161.
- Stovin, V., Poë, S., and Berretta, C., 2013. A modelling study of long term green roof retention performance. Journal of Environmental Management, 131, 206–215.
- Vesuviano, G., Sonnenwald, F., and Stovin, V., 2014. A two-stage routing model for green roof runoff detention. Water Science and Technology, 69 (6), 1191–1197.
- Vesuviano, G. and Stovin, V., 2013. A generic hydrological model for a green roof drainage layer. Water Science and Technology, 68 (4), 769–775.
- Villarreal, E.L. and Bengtsson, L., 2005. Response of a Sedum green-roof to individual rain events. Ecological Engineering, 25 (1), 1–7.
- Voyde, E., Fassman, E., and Simcock, R., 2010. Hydrology of an extensive living roof under sub-tropical climate conditions in Auckland. New Zealand. Journal of Hydrology, 394 (3–4), 384–395.
- WRc, 2009. STORMPAC User Guide, Version 4.1. Swindon: WRc plc.
- Yio, M.H.N., Stovin, V., Werdin, J., and Vesuviano, G., 2013. Experimental Analysis of Green Roof Detention Characteristics. Water Science and Technology, 68 (7), 1477–1486.
- Zhang, S.H. and Guo, Y.P., 2013. Analytical probabilistic model for evaluating the hydrologic performance of green roofs. Journal of Hydrologic Engineering, 18 (1), 19–28.