Abstract
Gully pots and lateral connections represent the anterior part of the sewer infrastructure responsible for the majority of flooding events in public areas. This study provides a statistical procedure to quantify the effectiveness of proactive management strategies to improve their performance. The first part consists of non-parametric methods to determine the evolution of gully pot blockages. The second part presents a Bayesian approach to quantify the development of reported flooding events when lateral connections are subject to proactive strategies. To this end, call data were collected from areas that were maintained proactively instead of reactively. Application of the procedure revealed a significant call decrease in one area. In addition, an increasing blockage likelihood over time indicated cyclic cleaning to be effective to improve sewer serviceability. By linking management strategies to performance, this flexible procedure can support sewer managers to balance the merits of proactive and reactive management strategies.
List of notations | ||
T | = | random variable denoting the time to a reported blockage event |
τ | = | elapsed time since cleaning |
n | = | population size of gully pots |
Y | = | number of registered calls |
= | Kaplan–Meier estimate of the cumulative call distribution function | |
= | hazard function | |
= | cumulative hazard function | |
K | = | Kernel function |
b | = | Kernel bandwidth |
= | constant call rate Exponential distribution | |
α | = | scale parameter Weibull distribution |
β | = | shape parameter Weibull distribution |
E | = | expected number of calls |
SCR | = | standardised call ratio |
θ | = | scale parameter Gamma distribution |
υ | = | shape parameter Gamma distribution |
1. Introduction
Gully pots (catch basins) and corresponding lateral connections constitute the anterior part of the sewer infrastructure dedicated to prevent the flooding of public areas by collecting and transporting run-off from urban surfaces. In addition, gully pots are designed to capture particles suspended in run-off by gravity separation, as these particles will otherwise deposit in the downstream urban water system (Deletic, Ashley, & Rest, Citation2000; Karlsson & Viklander, Citation2008). Pollutants arising from corrosion, atmospheric deposition, vehicular wear and leaks can be attached to these deposits (Li, Lau, Kayhanian, & Stenstrom, Citation2005). A schematisation of a typical gully pot and lateral connection is depicted in Figure .
There is a growing consensus that these assets are the predominate cause of flooding events (see, e.g. Caradot, Granger, Chapgier, Cherqui, & Chocat, Citation2011; Cherqui, Belmeziti, Granger, Sourdril, & Le Gauffre, Citation2015; ten Veldhuis, Clemens, & van Gelder, Citation2011). Next to considerable tangible and intangible damage (Freni, La Loggia, & Notaro, Citation2010), floods are associated with potential health risks (de Man et al., Citation2014). The hydraulic performance of a gully pot can be impaired as the continuous trapping of particles induces progressive silting over time. In addition, main sewer infrastructures are subject to continuous deterioration processes (Hahn, Palmer, Merrill, & Lukas, Citation2002; Wirahadikusumah, Abraham, & Iseley, Citation2001), which are also typical for the anterior part of the system. Silting of gully pots is managed by vacuum excavation cleaning, while blockages and collapses of lateral connections call for hydraulic cleaning or rehabilitation.
Appropriate asset management of sewer infrastructure is necessary to maintain the level of service provision, given the available resources. For main sewers, there is a concerted move towards adopting proactive condition-based management strategies (Salman & Salem, Citation2012; Wirahadikusumah et al., Citation2001). This approach is considered to be more cost-effective compared to reactive strategies (Fenner, Citation2000; Fontecha et al., Citation2016), as actions are planned before performance losses result in unacceptable risks. Decision-making for maintenance and rehabilitation relies on condition data, which are generally based on Closed Circuit Television Inspections (CCTV) (Dirksen et al., Citation2013), occasionally supported by available deterioration models. Yet, inspection reports on the condition of lateral connections and gully pots are generally absent. Furthermore, the performance of decision support models relies on the formation of groups with similar characteristics (Ana et al., Citation2009; Duchesne, Beardsell, Villeneuve, Toumbou, & Bouchard, Citation2013) such as age and materials, which are mostly unknown for the anterior part of the sewer system. Therefore, this part of the system is primarily maintained by reactive strategies based on registered citizen calls concerning flooding events. Cyclic cleaning of gully pot sand traps is an exception, as this involves proactive preventive cleaning activities after a fixed period of time (generally every 6–24 months).
Municipalities in the Netherlands spent around 26 million euros on cyclic gully pot cleaning annually, with cleaning costs varying between €3.5 and €7 per pot (Ashley, Bertrand-Krajewski, Hvitved-Jacobsen, & Verbanck, Citation2004). The effectiveness of these proactive activities depends on the flood risk within the cleaning interval (Swanson, Citation2001). Instead of quantitative data, this interval is based on the available budget (Fenner, Citation2000), vulnerability of the draining area or expert judgement. Still, Caradot et al. (Citation2011) reported gully pot blockages due to sediment accumulation to remain the dominant cause of urban flooding. For main sewers, Plihal, Kretschmer, Schwarz, and Ertl (Citation2014) found that the efficiency of cleaning strategies for sediment deposits improves when quantitative data are used to determine the cleaning interval. Several studies proposed physically based models to predict the accumulation of sediment in gully pots (e.g. Butler & Karunaratne, Citation1995; Ciccarello, Bolognesi, Maglionico, & Artina, Citation2012; Deletic et al., Citation2000). Since sewer sediment processes are currently not well described and understood, the predictive power of these models is limited (Ashley, Fraser, Burrows, & Blanksby, Citation2000; Freni, Mannina, & Viviani, Citation2008). Therefore, these models rely on the calibration of site-specific parameters (Memon & Butler, Citation2002) that limit the feasibility on a catchment scale.
Despite the lack of data, the direct impact of the anterior part of the sewer infrastructure on the overall level of sewer service provision calls for the optimisation of management strategies. Evaluating the effectiveness of proactive actions is pivotal, as it provides explicit motivation for management efforts. Due to the limited understanding of the complex particle transport processes that may induce blockages, statistical models are considered to be more successful (Rodríguez, McIntyre, Díaz-Granados, & Maksimović, Citation2012). This study describes a statistical approach to quantify the effectiveness of proactive actions to reduce citizen calls. Calls on flooding events are a measure of serviceability, a performance indicator that has received wide acceptance in sewerage provision (Arthur, Crow, Pedezert, & Karikas, Citation2009; Ashley & Hopkinson, Citation2002). By evaluating the relationship between proactive management strategies and their contribution to system performance, the techniques proposed in this study support sound decision-making where the merits of proactive and reactive management strategies are balanced to optimise costs and sewer serviceability.
This paper first describes a statistical procedure to analyse gully pot blockages and lateral connection blockages. The analysis of gully pot blockages is based on a database comprising call data collected in two municipalities covering over 150,000 gully pots and 3478 reported flooding events. For the analysis of lateral connection blockages, call data were collected for two areas in two periods: period A, the reference period, prior to the proactive maintenance of all lateral connections and period B, the period after the proactive maintenance. Results from the application of the proposed procedure to these data and the implications are discussed in Section 4.
2. Methods
This Section presents statistical methods to analyse call data with the aim to quantify the effect of proactive management strategies. The first part is dedicated to analysing the effect of cyclic gully pot cleaning. The second part describes a Bayesian method to quantify the ability of lateral connection rehabilitation activities to decrease call rates.
2.1. Analysing the effect of cyclic gully pot cleaning
The effectiveness of gully pot cleaning is determined by the evolution of the number of registered calls on flood events over time. When the probability of a call is unaffected by preventive cleaning activities, the strategy is considered to be ineffective in maintaining the hydraulic performance of the system. This study analyses trends in the operational performance of gully pots by describing the distribution of times to a registered call on a blockage event. The time to a blockage event is defined as the random variable T, which represents the time between cleaning and a registered call.
Analysis of the data is complicated by the fact that not all gully pots in a given population experience a blockage before scheduled cleaning activities are initiated. All that is known about these gully pots is that their time to blockage is beyond the cleaning interval. This is commonly referred to as right censored data. Further complexity is added by the initiation of a cleaning cycle, during which the size of the remaining population eligible for blockage changes continually. Both issues are schematised in Figure , for six arbitrary gully pots.
Figure 2. Example of the timelines of six gully pots. A timeline ends when either a gully pot is preventively cleaned (censored) or is reported blocked by citizens. Consequently, the remaining population at risk of a blockage varies over time.
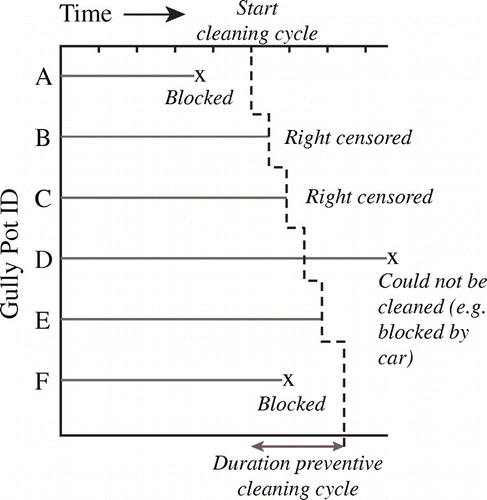
The Kaplan–Meier estimate (Kaplan & Meier, Citation1958) is a non-parametric method that does not suffer from the aforementioned issues, as it considers the varying number of gully pots at risk of a blockage at a given time. The estimate of the cumulative distribution function (CDF), giving the probability of a call before time τ, is given by:(1)
where ni are all the gully pots at risk at time step i, Yi all the calls on blocked gully pots and τ the elapsed time since cleaning. For comparison, two other common parametric failure models are evaluated: the Exponential distribution and the Weibull distribution. The versatile Weibull distribution (Weibull, Citation1951) is able to approximate several continuous failure distributions and is generally capable of modelling both increasing and decreasing trends in call rates (Tobias & Trindade, Citation2011). The CDF of this distribution is given by:(2)
with scale parameter α and shape parameter β. Estimates of the parameter β that exceed 1 indicate increasing call rates, corresponding to deteriorating gully pot performance over time. Confidence intervals for the parameters of the Weibull distribution are obtained by bootstrapping (Efron, Citation1979). This involves randomly resampling, with replacement, observed call data and deriving the Weibull parameters for each sample.
The Exponential distribution is characterised by a constant call rate . This implies that blockages occur randomly over time and are unaffected by preventive cleaning. This distribution is a special case of the Weibull distribution where β = 1 and
. A more formal way to illustrate trends in registered calls rates is the hazard function. It represents the conditional probability of a call in the interval
, given that no call was registered prior to τ. In mathematical form this is denoted as:
(3)
The hazard function is undefined at time steps with no calls. Therefore, Tanner and Wong (Citation1983) proposed the application of kernel smoothers (i.e. local weighted averaging) to construct a continuous function from a set of point estimates at each time step. Considering that the cumulative hazard function is related to Equation (Equation1(1) ) by
, the kernel estimate of the hazard function is given by:
(4)
where K is the kernel function and b is the bandwidth. It is generally accepted that the choice of the kernel function is not crucial (Silverman, Citation1986). This study employed the Epanechnikov kernel. The bandwidth was optimised by minimising the integrated mean squared error, since the effect of the value on the hazard estimate is considered pivotal. When the derived hazard function is horizontal, it corresponds to the constant call rate
assumed by the Exponential distribution.
2.2. Modelling the spatial structure of lateral connection calls
Quantifying the effect of lateral connection rehabilitation activities requires the collection of data both before and after the rehabilitation intervention. Standard hypothesis testing relies on a direct comparison of these data to detect an intervention effect. For lateral connections, this effect can be obscured by the inherent variation in hydraulic and solids loadings over time (Ellis & Harrop, Citation1984; Pratt, Elliott, & Fulcher, Citation1987). For example, an observed effect in the number of calls can be the result of a severe storm event or seasonal leaf-fall instead of an improved operational condition.
In the field of spatial epidemiology, it is common to analyse the relative risk (see e.g. Bernadinelli, Pascutto, Best, & Gilks, Citation1997; Clayton & Kaldor, Citation1987). Relative risk represents the degree to which the probability of an event in an area is higher or lower than the standardised city mean. This can be expressed as the ratio between the observed number of reported calls in a rehabilitated area and the expected number of events, which is derived from the underlying city mean call rate. As the city is assumed to be subject to similar hydraulic loads, this approach is considered to be more appropriate than standard hypothesis testing. Serviceability improvements can be quantified by comparing differences in the standardised call ratio (SCR) before and after rehabilitation activities. In addition, analysis of the spatial distribution of standardised call ratios allows for the identification of areas with a significant increased call incidence, where proactive strategies are most promising.
Assuming mutual independence between observed calls concerning lateral connection blockages, the Poisson distribution is a typical candidate to model observed calls Yj in neighbourhood j of the p neighbourhoods in a city and is given by:(5)
where the SCR (Standardised Call Ratio) represents the true, but unknown, neighbourhood-specific relative call ratio. In the case where SCRj equals unity, the neighbourhood in question shows no deviation from the overall call probability in the city. The expected number of calls Ej is estimated by:(6)
which is simply the mean number of calls per gully pot in the city multiplied by the number of gully pots in area j.
A limitation of the Poisson distribution is its inability to capture the extent of variation generally present in count data compared to the expected variance (i.e. over-dispersion) (Lawson, Citation2013). This is due to the fixed relation between the mean and the variance assumed by this distribution. Consequently, the model may improperly indicate the presence of a significant effect as credible intervals are too narrow. Alternatively, the excess variation in the data can be accommodated by a second model layer, describing the prior distribution of the SCRj as a Gamma distribution with shape parameter υj and scale parameter θj. The linkage between the different elements of the model is depicted in Figure . The left part of this figure contains all registered calls before inspection and rehabilitation activities, while the right part contains all registered calls following these activities. ∆SCR is the difference distribution that yields information on whether the intervention resulted in a statistically significant difference in the call ratio.
Figure 3. Directed Acyclic Graph (DAG) illustrating the linkage between the different elements in the Poisson–Gamma model used to quantify developments of the SCR (Standardised Call Ratio).
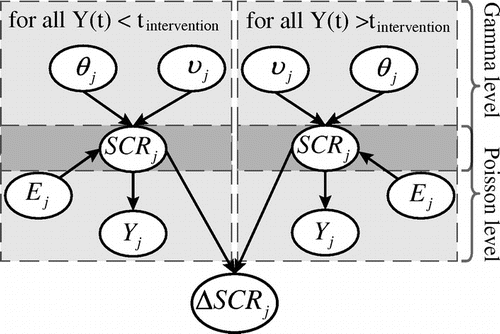
The SCR was estimated by applying a Bayesian approach, which considers unknown parameters as random variables. Bayesian inference takes into account parameter uncertainty and is therefore particularly suited for interpreting the significance of any change in the SCR owing to rehabilitation activities. Given Bayes theorem, the joint posterior density can be expressed as:(7)
where P(SCR) represents the second level of the model, described by a gamma distribution. P(SCR|Y) is referred to as the likelihood function, which for the first level is given by:(8)
and for the second level:(9)
The posterior distribution was evaluated by means of the Markov Chain Monte Carlo (MCMC) method. This study applied the Gibbs sampler (Geman & Geman, Citation1984) as MCMC algorithm, which was implemented in the open source software JAGS (Plummer, Citation2003) and was called from the R software environment (R Core Team, Citation2014). Non-informative flat gamma (0.01, 0.01) priors for and
, represented the lack of knowledge about the gamma parameters.
3. Call data
The procedure described in Section 2 was applied to a data-set of linked proactive (Sections 3.1–3.2) and reactive maintenance data. Reactive maintenance data from the citizen call registers of two municipalities in The Netherlands were interrogated. The different failure mechanisms responsible for flooding events are depicted in Figure . In total, 92% of all registered calls were attributed to the performance of gully pots and lateral connections. Since both sediment deposits and non-particulate matter (cans, packaging material, etc.) are both removed by cyclic cleaning, potentially 72% of all gully pot blockages can be prevented. Rehabilitation of lateral connections can reduce the number of structural defects and the susceptibility to tree root intrusion, which form 55% of all calls pertaining to lateral connections. Consequently, intensifying proactive strategies for these components can potentially reduce the total number of calls by up to 63%.
Figure 4. Tree diagram of the contribution of different failure mechanisms to flooding events registered in the call database.
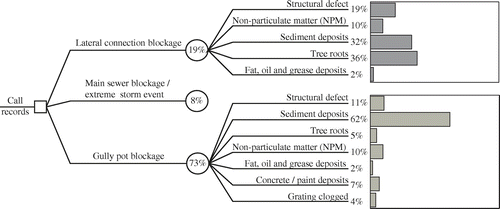
A subset of call data that were attributed to the operational condition of gully pots and lateral connections by municipal workers were selected for this study. With a combined population of 524,000 inhabitants (CBS, Citation2014), these subsets contained 3096 calls (period 03-2012–08-2015) for the city of Utrecht and 382 calls (period 10-2014–10-2015) for the city of Almere. The latter is a relatively new city with most of the sewer infrastructure developed after 1970, while most of the sewer infrastructure in Utrecht has been developed after 1950. Characteristics of these two cities are presented in Table .
Table 1. Characteristics of the cities of Utrecht and Almere.
3.1. Preventive cyclic cleaning of gully pots
Data on the location of gully pots eligible for blocking were collected during cyclic preventive cleaning of the gully pot sand traps. This included the time of cleaning. Although the normal cleaning frequency was once a year, cleaning frequencies for individual gully pots varied due to local circumstances (e.g. inability to clean due to parked vehicles). In some cases, this resulted in the exclusion from a cleaning cycle. As a consequence, data were available for well beyond the standard cleaning interval of one year.
3.2. Proactive inspection and rehabilitation of lateral connections
Two neighbourhoods in the city of Utrecht with close to 1250 gully pots were selected to investigate the effect of extensive proactive maintenance. Call data from the period prior to the proactive activities served as a reference to quantify the evolution of calls with respect to the period after these activities.
Next to the normal cyclic cleaning activities of the gully pot, lateral connections were hydraulically jetted and inspected by means of CCTV. The resulting data-set contained detailed information on the condition of these assets. If the condition of an asset was rated unacceptable, an intervention was planned and the asset was proactively rehabilitated. Characteristics of the two neighbourhoods are presented in Table . The majority of pipes that were rated unacceptable experienced tree root intrusion or a (partial) collapse.
Table 2. Characteristics of the two neighbourhoods where lateral connections were subject to proactive maintenance.
4. Results and discussion
The results presented in this section consist of two parts. First, the impact of preventive gully pot cleaning on the reactive maintenance workload is determined. To this end, the Kaplan–Meier estimate and the hazard function was computed to determine how the number of calls concerning gully pot blockages developed as a function of time. Second, the influence of lateral connection inspection and rehabilitation was investigated by comparing standardised call ratios (SCR’s) before and after an intervention.
4.1. Evolution of sediment induced blockages in gully pots
The probability of a call due to excessive sediment deposits in a gully pot in Utrecht are depicted in Figure . Increasing confidence interval widths are the result of right censoring, as the remaining population of gully pots eligible for blocking decreased over time. In addition to the Kaplan–Meier estimate, the parametric exponential distribution with a constant instantaneous call rate is depicted in this figure. The empirical blockage data exhibit an increasing departure from this distribution, as the elapsed time since cleaning increases. This implies an increasing call rate and the invalidity of the constant call rate assumption. The shape parameter β of the Weibull distribution in Table provides further evidence for an increasing call rate over time, as both the estimate and the 95% confidence interval exceeds 1. The scale parameter α has wide confidence interval corresponding to substantial parameter uncertainty. Since this parameter is an indicator of the characteristic time until a blockage, more precisely the 63.2th percentile, this uncertainty can be attributed to the maximum bound of the data (550 days).
Figure 5. Kaplan–Meier estimate of the probability that a gully pot is reported blocked for Utrecht as a function of the elapsed time since cleaning (dotted line), including 95% confidence intervals. An Exponential model assuming a constant call rate is added (solid line).
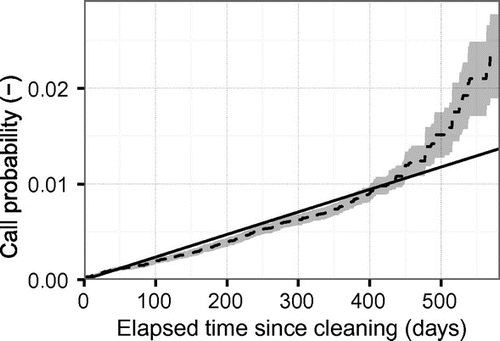
Table 3. Parameter estimates for the Weibull distribution parameters α and β, for the city of Utrecht and Almere. Bootstrap resampling was used to obtain 95% confidence intervals.
The hazard function presented in Figure illustrates the increasing trend in the call rate more clearly. These results suggest that progressive silting of the sand trap eventually takes its toll on the hydraulic performance of gully pots. The sudden increase in the call rate suggests that for this case, the interval between successive cleaning cycles should not exceed 400 days. Figure displays a similar trend for call data in Almere. Blockages due to excessive sediment deposits in gully pots appear to be non-random in time and subject to increasing call rates as the time since cleaning progresses. Table shows that confidence intervals for the scale parameter α and the shape parameter β of the Weibull distribution are of the same order of magnitude as for Utrecht.
Figure 6. Kernel estimate of the hazard function for Utrecht as a function of the elapsed time since cleaning.
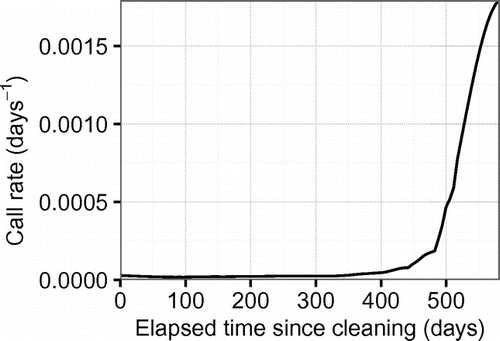
Figure 7. Kaplan–Meier estimate of the probability that a gully pot is reported blocked for Almere as a function of the elapsed time since cleaning (dotted line), including 95% confidence intervals. An Exponential model assuming a constant call rate is added (solid line).
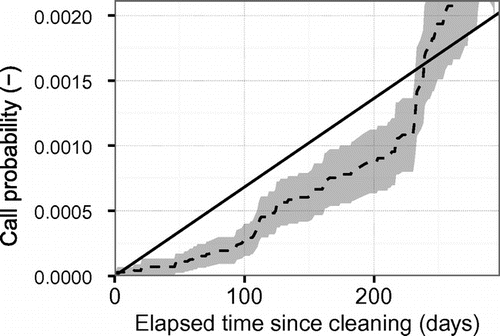
Figure 8. Kernel estimate of the hazard function for Almere as a function of the elapsed time since cleaning.
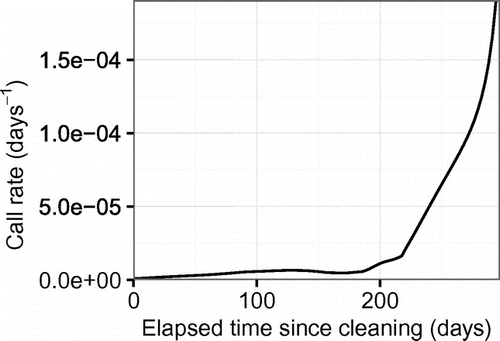
On the whole, the hazard function shows an increasing call rate for both cities, indicating that progressive silting may eventually impair the gully pots ability to transport run-off to the sewer system. These findings provide evidence that cyclic cleaning is effective to reduce citizen calls caused by this failure mechanism. Generalisation of the characteristic time scales of derived blockage rates may be limited, as different parameters influence the trapping efficiency of gully pots (Memon & Butler, Citation2002). However, these methods can be applied to any catchment where sufficient call data are available.
Results for the presented cases are in line with the experimental findings of Butler and Karunaratne (Citation1995), who reported an overall positive particle retention efficiency. A monitoring study by Conradin (Citation1989) resulted in somewhat different conclusions, as sediment beds were observed to reach an equilibrium state below the level of the outflow pipe after approximately 6 months. Post et al. (Citation2016) observed two distinct sedimentation evolutions: stabilising sediment beds and progressive accumulation. The latter group being largely responsible for gully pot blockages. Both properties of the area draining to a gully pot and the design of a gully pot were reported to influence the blockage propensity.
Kaplan–Meier estimates can aid to balance preventive and reactive actions to determine an optimum level of service, given the available budget. It should be noted that although calls are an indicator of events deemed unacceptable by citizens, it is likely that the number of recorded blockages is an underestimation of the true number of gully pot blockages. For example, some events may not be observed (events at remote locations or during the night), alternative flow paths might be available or single flooding events may involve multiple blocked gully pots. For example, Post et al. (Citation2016) measured the build-up of sediments in gully pots and found 5% to be blocked after 14 months. Yet, no citizen calls in the area were received by the municipal call centre in that period. Although the derived call probabilities are low, gully pots are still responsible for a majority of all calls (see Figure ) due to their sheer numbers. With cyclic gully pot cleaning costs varying between €3 and €6, proactive costs are low compared to €100–€200 for reactive actions (Ten Veldhuis, Citation2010). Figure shows the financial trade-off between proactive and reactive maintenance, given this cost range and the Kaplan–Meier estimates. This figure shows that cyclic gully pot cleaning is cost-effective. The total costs are minimised when a cleaning frequency between 12 and 16 months is maintained. However, these costs do not include damage costs and societal consequences and should therefore only serve as an absolute upper limit for the cleaning interval.
Figure 9. Estimated proactive and reactive maintenance costs for Utrecht as a function of the cleaning interval. Reactive maintenance needs are based on the Kaplan–Meier estimates. Uncertainty bands represent the maintenance cost range given by Ten Veldhuis (Citation2010).
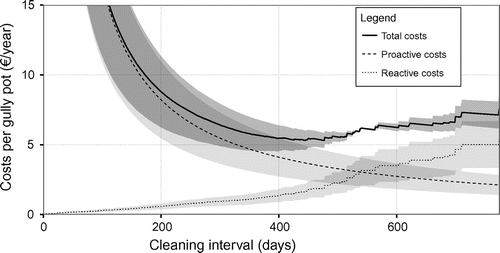
4.2. The effectiveness of proactive strategies for lateral connections
The Standardised Call Ratio (SCR) discussed in Section 2.2, reflects the degree to which the call rate in a neighbourhood is different from the overall city mean rate. Analysis of the Poisson model used to estimate the SCR revealed substantial overdispersion (15.56 ≫ 1), as the data exhibited extra variation which could not be captured by the model. Therefore, results were analysed using the two-level Poisson–Gamma model. The Gelman–Rubin diagnostic (Gelman & Rubin, Citation1992) revealed no convergence problems of the MCMC chains. Figure shows the estimated SCR’s for neighbourhoods in Utrecht. In total 14 neighbourhoods were found to have significantly higher call rates, indicating areas where proactive inspection and rehabilitation is most promising.
Figure 10. Ninety-five per cent credible intervals of the Standardised Call Ratio (SCR) for neighbourhoods in Utrecht, The Netherlands before the intervention. The dotted line indicates the standardised mean city call rate and significant increased rates are indicated by black credible intervals.
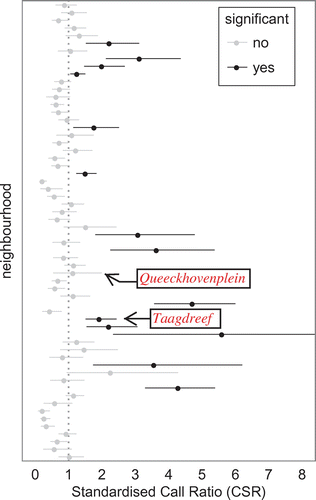
The neighbourhoods Taagdreef and Queeckhovenplein introduced in Section 3.2 were inspected and lateral connections were rehabilitated when deemed necessary. From these two neighbourhoods, only Taagdreef has a significantly increased call ratio according to Figure . Table shows both the observed and expected number of calls before and after rehabilitation activities. The expected number of calls was based on the overall mean city call rate, according to Equation (Equation6(6) ). For Queeckhovenplein, the number of observed calls was lower than expected, while for Taagdreef the number of observed calls exceeded the expectation prior to intervention.
Table 4. Observed and expected calls for two neighbourhoods in Utrecht before and after maintenance activities.
The effectiveness of these proactive activities to reduce the number of calls was quantified by computing the change in SCR after the intervention. Figure shows differences in posterior samples for the SCR before and SCR after rehabilitation activities. For the Taagdreef case, there is evidence of a significant improvement in the number of calls as the 95% credible interval does not contain zero. There is insufficient evidence to make similar statements for Queeckhovenplein. This could be the result of the size of the area, which limits the amount of registered calls (see Table ). Alternatively, the relatively low SCR before the intervention (see Figure ) implies that the overall potential to improve the operational condition in this neighbourhood was limited. It should be noted that lateral connection rehabilitation does not only safeguard these components from collapse, but may also reduce susceptibility to more recurring failure mechanisms such as the intrusion of tree roots (Ridgers, Rolf, & Stål, Citation2006).
5. Conclusions
The objective of this study was to describe an approach to quantify the effectiveness of proactive actions to maintain a level of service provision. Based on this objective a procedure has been presented which can support sewer managers to optimise management strategies by evaluating the effect on the level of service provision. From the application of this procedure to examples concerning registered calls on flood incidents due to failures of the anterior part of the sewer system, the following conclusions are drawn.
Increasing call rates as a function of the time since cleaning provide evidence that gully pot blockages are non-random and that preventive cyclic cleaning is effective to reduce the number of registered citizen calls. These results suggest that the continuous trapping of solids eventually impairs a gully pots hydraulic performance. This is also reflected in the estimated parameters for the Weibull distribution that show an increasing blockage propensity as the time since cleaning passes. Analysis of the non-parametric Kaplan–Meier estimate can aid sewer managers to optimise management strategies, as it allows for a quantitative assessment of the balance between proactive and reactive actions. For instance, results show that a cleaning interval between 12 and 16 months minimises the total maintenance costs for Utrecht. It should be noted that generalisation of the obtained results may be limited due to the local characteristics of the urban environment. However, the Kaplan–Meier estimator can be applied to other cases without making any assumptions about the distribution of the underlying data.
The proposed two-level Bayesian hierarchical model is able to provide valuable information on the effectiveness of lateral connection rehabilitation to improve sewer serviceability. Analysing the Standardised Call Ratio (SCR) is considered to be appropriate, as it compares changes in local call rates relative to the mean call rates for the city. Application of this model revealed a significant improvement in the level of sewer serviceability in an area that was experiencing above mean call rates. This provides evidence that inspection and rehabilitation of lateral connections was effective. Given the disproportional contribution of lateral connections to the risk of flood incidents, the findings from this study support the inclusion of these components into the inspection and rehabilitation management process. Quantifying the effectiveness of rehabilitation efforts can justify the allocation of investments. In addition, analysis of the SCR allows for the identification of areas with significant increased call rates, which can enhance the management process involved in prioritising inspections.
Disclosure statement
No potential conflict of interest was reported by the authors.
Acknowledgements
The research is performed within the Dutch ‘Kennisprogramma Urban Drainage’ (Knowledge Programme Urban Drainage). The involved parties are: ARCADIS, Deltares, Evides, Gemeente Almere, Gemeente Arnhem, Gemeente Breda, Gemeente ‘s-Gravenhage, Gemeentewerken Rotterdam, Gemeente Utrecht, GMB Rioleringstechniek, Sweco, KWR Watercycle Research Institute, Royal HaskoningDHV, Stichting RIONED, STOWA, Tauw, vandervalk+degroot, Waterboard De Dommel, Waternet and Witteveen+Bos.
References
- Ana, E.V., Bauwens, W., Pessemier, M., Thoeye, C., Smolders, S., Boonen, I., & De Gueldre, G. (2009). An investigation of the factors influencing sewer structural deterioration. Urban Water Journal, 6, 303–312. doi:10.1080/15730620902810902
- Arthur, S., Crow, H., Pedezert, L., & Karikas, N. (2009). The holistic prioritisation of proactive sewer maintenance. Water Science & Technology, 59, 1385–1396. doi:10.2166/wst.2009.134
- Ashley, R.M., Bertrand-Krajewski, J.-L., Hvitved-Jacobsen, T., & Verbanck, M. (2004). Solids in sewers: Characteristics, effects and control of sewer solids and associated pollutants. London: IWA Publishing Company.
- Ashley, R.M., Fraser, A., Burrows, R., & Blanksby, J. (2000). The management of sediment in combined sewers. Urban Water Journal, 2, 263–275. doi:10.1016/S1462-0758(01)00010-3
- Ashley, R.M., & Hopkinson, P. (2002). Sewer systems and performance indicators–into the 21st century. Urban Water Journal, 4, 123–135. doi:10.1016/S1462-0758(02)00010-9
- Bernadinelli, L., Pascutto, C., Best, N.G., & Gilks, W.R. (1997). Disease mapping with errors in covariates. Statistics in Medicine, 16, 741–752. doi:10.1002/(SICI)1097-0258(19970415)16:7<741:AID-SIM501>3.0.CO;2-1
- Butler, D., & Karunaratne, S.H.P.G. (1995). The suspended solids trap efficiency of the roadside gully pot. Water Research, 29, 719–729. doi:10.1016/0043-1354(94)00149-2
- Caradot, N., Granger, D., Chapgier, J., Cherqui, F., & Chocat, B. (2011). Urban flood risk assessment using sewer flooding databases. Water Science & Technology, 64, 832–840. doi:10.2166/wst.2011.611
- CBS. (2014). Demografische kerncijfers per gemeente 2014 [Demographic highlights per municipality in 2014]. The Hague: Centraal Bureau voor de Statistiek.
- Cherqui, F., Belmeziti, A., Granger, D., Sourdril, A., & Le Gauffre, P. (2015). Assessing urban potential flooding risk and identifying effective risk-reduction measures. Science of the Total Environment, 514, 418–425. doi:10.1016/j.scitotenv.2015.02.027
- Ciccarello, A., Bolognesi, A., Maglionico, M., & Artina, S. (2012). The role of settling velocity formulation in the determination of gully pot trapping efficiency: Comparison between analytical and experimental data. Water Science & Technology, 65, 15–21. doi:10.2166/wst.2011.775
- Clayton, D., & Kaldor, J. (1987). Empirical bayes estimates of age-standardized relative risks for use in disease mapping. Biometrics, 43, 671–681. doi:10.2307/2532003
- Conradin, F. (1989, October 22–27). Study on catch basins in Switzerland. Paper presented at the URRC ASCE Conference ‘Urban Stormwater Quality Enhancement: Source Control, Retrofitting, and Combined Sewer Technology’, Davos Platz, Switzerland.
- de Man, H., van den Berg, H.H.J.L., Leenen, E.J.T.M., Schijven, J.F., Schets, F.M., van der Vliet, J.C., van Knapen, F., & de Roda Husman, A.M. (2014). Quantitative assessment of infection risk from exposure to waterborne pathogens in urban floodwater. Water Research, 48, 90–99. doi:10.1016/j.watres.2013.09.022
- Deletic, A., Ashley, R.M., & Rest, D. (2000). Modelling input of fine granular sediment into drainage systems via gully-pots. Water Research, 34, 3836–3844. doi:10.1016/S0043-1354(00)00133-0
- Dirksen, J., Clemens, F.H.L.R., Korving, H., Cherqui, F., Le Gauffre, P., Ertl, T., Plihal, H., Müller, K., & Snaterse, C.T.M. (2013). The consistency of visual sewer inspection data. Structure and Infrastructure Engineering, 9, 214–228. doi:10.1080/15732479.2010.541265
- Duchesne, S., Beardsell, G., Villeneuve, J.P., Toumbou, B., & Bouchard, K. (2013). A survival analysis model for sewer pipe structural deterioration. Computer-Aided Civil and Infrastructure Engineering, 28, 146–160. doi:10.1111/j.1467-8667.2012.00773.x
- Efron, B. (1979). Bootstrap methods: Another look at the jackknife. The Annals of Statistics, 7(1), 1–26. doi:10.1214/aos/1176344552
- Ellis, J.B., & Harrop, D.O. (1984). Variations in solids loadings to roadside gully pots. Science of the Total Environment, 33, 203–211. doi:10.1016/0048-9697(84)90394-2
- Fenner, R.A. (2000). Approaches to sewer maintenance: A review. Urban Water Journal, 2, 343–356. doi:10.1016/S1462-0758(00)00065-0
- Fontecha, J.E., Akhavan-Tabatabaei, R., Duque, D., Medaglia, A.L., Torres, M.N., & Rodríguez, J.P. (2016). On the preventive management of sediment-related sewer blockages: A combined maintenance and routing optimization approach. Water Science & Technology, 74, 302–308. doi:10.2166/wst.2016.160
- Freni, G., La Loggia, G., & Notaro, V. (2010). Uncertainty in urban flood damage assessment due to urban drainage modelling and depth-damage curve estimation. Water Science & Technology, 61, 2979–2993. doi:10.2166/wst.2010.177
- Freni, G., Mannina, G., & Viviani, G. (2008). Uncertainty assessment of sewer sediment erosion modelling. Urban Water Journal, 5, 21–31. doi:10.1080/15730620701737033
- Gelman, A., & Rubin, D.B. (1992). Inference from iterative simulation using multiple sequences. Statistical Science, 7, 457–472. doi:10.1214/ss%2F1177011136
- Geman, S., & Geman, D. (1984). Stochastic relaxation, gibbs distributions, and the bayesian restoration of images. IEEE Transactions on Pattern Analysis and Machine Intelligence, 6, 721–741. doi:10.1109/TPAMI.1984.4767596
- Hahn, M.A., Palmer, R.N., Merrill, M.S., & Lukas, A.B. (2002). Expert system for prioritizing the inspection of sewers: Knowledge base formulation and evaluation. Journal of Water Resources Planning and Management, 128, 121–129. doi:10.1061/(ASCE)0733-9496
- Kaplan, E.L., & Meier, P. (1958). Nonparametric estimation from incomplete observations. Journal of the American Statistical Association, 53, 457–481. doi:10.1080/01621459.1958.10501452
- Karlsson, K., & Viklander, M. (2008). Trace metal composition in water and sediment from catch basins. Journal of Environmental Engineering, 134, 870–878. doi:10.1061/(ASCE)0733-9372
- Lawson, A.B. (2013). Bayesian disease mapping: Hierarchical modeling in spatial epidemiology (2nd ed.). Boca Raton, FL: CRC Press
- Li, Y., Lau, S.L., Kayhanian, M., & Stenstrom, M.K. (2005). Particle size distribution in highway runoff. Journal of Environmental Engineering, 131, 1267–1276. doi:10.1061/(ASCE)0733-9372
- Memon, F.A., & Butler, D. (2002). Assessment of gully pot management strategies for runoff quality control using a dynamic model. Science of The Total Environment, 295, 115–129. doi:10.1016/S0048-9697(02)00056-6
- Plihal, H., Kretschmer, F., Schwarz, D., & Ertl, T. (2014). Innovative sewer inspection as a basis for an optimised condition-based maintenance strategy. Water Practice and Technology, 9, 88–94. doi:10.2166/wpt.2014.010
- Plummer, M. (2003, March 20–22 ). JAGS: A program for analysis of Bayesian graphical models using Gibbs sampling. Paper presented at the 3rd International Workshop on Distributed Statistical Computing, Vienna, Austria.
- Post, J.A.B., Pothof, I.W.M., Dirksen, J., Baars, E.J., Langeveld, J.G., & Clemens, F.H.L.R. (2016). Monitoring and statistical modelling of sedimentation in gully pots. Water Research, 88, 245–256. doi:10.1016/j.watres.2015.10.021
- Pratt, C.J., Elliott, G.E.P., & Fulcher, G.A. (1987). Suspended solids discharge from highway gully pots in a residential catchment. Science of the Total Environment, 59, 355–364. doi:10.1016/0048-9697(87)90459-1
- R Core Team. (2014). R: A language and environment for statistical computing. Vienna, Austria: R Foundation for Statistical Computing.
- Ridgers, D., Rolf, K., & Stål, Ö. (2006). Management and planning solutions to lack of resistance to root penetration by modern PVC and concrete sewer pipes. Arboricultural Journal, 29, 269–290. doi:10.1080/03071375.2006.9747467
- Rodríguez, J.P., McIntyre, N., Díaz-Granados, M., & Maksimović, Č. (2012). A database and model to support proactive management of sediment-related sewer blockages. Water Research, 46, 4571–4586. doi:10.1016/j.watres.2012.06.037
- Salman, B., & Salem, O. (2012). Modeling failure of wastewater collection lines using various section-level regression models. Journal of Infrastructure Systems, 18, 146–154. doi:10.1061/(ASCE)IS.1943-555X.0000075
- Silverman, B.W. (1986). Density estimation for statistics and data analysis. Boca Raton, FL: Chapman & Hall/CRC.10.1007/978-1-4899-3324-9
- Swanson, L. (2001). Linking maintenance strategies to performance. International Journal of Production Economics, 70, 237–244. doi:10.1016/S0925-5273(00)00067-0
- Tanner, M.A., & Wong, W.H. (1983). The estimation of the hazard function from randomly censored data by the kernel method. The Annals of Statistics, 11, 989–993. doi:10.1214/aos/1176346265
- Ten Veldhuis, J.A.E. (2010). Quantitative risk analysis of urban flooding in lowland areas ( PhD thesis). Delft, Netherlands: Delft University of Technology.
- ten Veldhuis, J.A.E., Clemens, F.H.L.R., & van Gelder, P.H.A.J.M. (2011). Quantitative fault tree analysis for urban water infrastructure flooding. Structure and Infrastructure Engineering, 7, 809–821. doi:10.1080/15732470902985876
- Tobias, P.A., & Trindade, D. (2011). Applied reliability. Boca Raton, FL: Chapman & Hall/CRC.
- Weibull, W. (1951). Wide applicability. Journal of Applied Mechanics, 18, 293–297.
- Wirahadikusumah, R., Abraham, D.M., & Iseley, T. (2001). Challenging issues in modeling deterioration of combined sewers. Journal of Infrastructure Systems, 7, 77–84. doi: 10.1061/(ASCE)1076-0342